Abstract
The rapid expansion of consumer sleep devices is outpacing the validation data necessary to assess the potential use of these devices in clinical and research settings. Common sleep monitoring devices utilize a variety of sensors to track movement as well as cardiac and respiratory physiology. The variety of sensors and user-specific factors offer the potential, at least theoretically, for clinically relevant information. We describe the current challenges for interpretation of consumer sleep monitoring data, since the devices are mainly used in non-medical contexts (consumer use) although medically-definable sleep disorders may commonly occur in this setting. A framework for addressing questions of how certain devices might be useful is offered. We suggest that multistage validation efforts are crucially needed, from the level of sensor data and algorithm output, to extrapolations beyond healthy adults and into other populations and real-world environments.
Introduction
Wearable technology for health and wellness tracking is expanding rapidly in the consumer space – perhaps faster in some cases than the medical community can assess potential risks and benefits of these advances. A variety of sensor technologies have been applied specifically to monitor sleep–wake patterns. Some of these technologies claim to improve sleep, either via “sleep-coach” features or by the implication that simply tracking one’s sleep will result in discovery of patterns that can lead to sleep improvements. Consumer demand for sleep monitors is striking, with the market apparently sufficient to support numerous companies active in this space, as well as frequent new additions from a pipeline that enjoys rapid technical and computational advances.
Despite extensive published experience with the limitations of wrist actigraphy for quantifying sleep, most of the currently available monitoring devices are actigraphy-based yet purport to accurately measure sleep. To place the marketing trends and consumer demand into perspective, we note that a consumer sleep monitor with no published validation was listed among Time Magazine’s inventions of the year in 2005,Citation1 decades after wrist actigraphy had been introduced for sleep tracking. The combination of consumer demand and potential or perceived wellness benefits of sleep monitors seems to overshadow the limitations and lack of validation, as recently reviewedCitation2 (some criticisms have been voiced in the academicCitation3,Citation4 as well as legal settingsCitation5). The recently increased involvement of large technology companies like Apple and Intel (the latter acquired the Basis watch) in sleep monitoring may lend further legitimacy to the idea of consumer-facing sleep monitors.
The current landscape of marketing claims that stretch beyond the scant validation literature may contribute to the discrepancy between consumer and health care community adoption of sleep monitoring devices. Clinicians and researchers, more familiar with the extensive experience and validation of polysomnography (PSG) and actigraphy, may be hesitant to become early adopters pending rigorous validation, which may not be prioritized for devices that do not intend to make medical claims. Wellness claims are necessarily vague, and as a result, government oversight is limited from a medical use perspective. In this review, we explore the developments in consumer sleep monitoring technology, identify validation issues in this space, and speculate on potential clinical utility. That sleep conceptually straddles an increasingly blurry boundary between sleep as a medical field and sleep as “wellness” emphasizes the need for a framework to interpret consumer sleep device outputs across this spectrum.
Consumer sleep monitors: what they measure, and what they claim
The most basic type of sensor used in consumer sleep monitors is movement detection, often based on wrist actigraphy. The algorithm output of devices in this class () may subdivide sleep into “light” or “deep” phases (eg, Jawbone and Fitbit), presumably based on the degree of detected movement. Sensor technology has more recently expanded to incorporate physiological measures beyond actigraphy, such as heart rate and/or respiration patterns (). Priced similarly to some of the popular wrist movement devices, these devices contain more advanced sensing methods, some of which claim to distinguish rapid eye movement (REM) and non-REM (NREM) sleep substages. Two wrist devices monitor heart rate in addition to actigraphy and use this information to stage REM and NREM sleep (Basis, Jawbone UP3). FitBit has a model that reports heart rate, but it is not clear that this information is used in the sleep staging algorithm. Other cardiopulmonary monitors for tracking sleep can be either worn on the body (as a shirt with embedded sensors, such as the Hexoskin), or placed in the bedroom for passive data collection, such as on the mattress (eg, Beddit). The totally noncontact sensing of respiration pattern (ResMed S+) is also available and reports REM and NREM sleep stages based on stage-specific breathing patterns, which were seen even in the earliest descriptions of REM sleep.Citation6 Each provides a sleep score (usually 0–100 scale), based on some combination of features such as total sleep duration, “depth” or fragmentation; none of the scoring quality methods has been validated. The devices differ in battery charging requirements and water resistance (). illustrates example outputs from some of the devices, representing a spectrum of sensor technologies, recorded simultaneously with gold standard measurements from PSG or formal actigraphy. A visual comparison between outputs among the different consumer devices, and between each consumer device and the gold standards, highlights the need for careful evaluation of what kinds of information the monitors provide, and how that information compares and/or complements currently accepted methodologies.
Figure 1 Comparison of sleep architecture across monitoring modalities.
Abbreviations: REM, rapid eye movement; PSG, polysomnography.
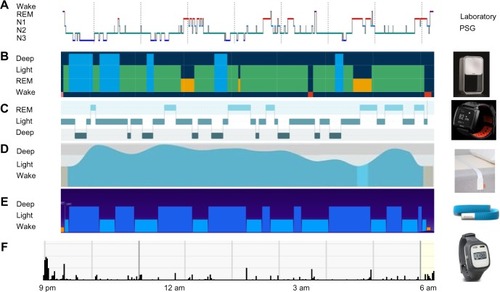
Table 1 Overview of common consumer sleep monitors
Although many of the available consumer devices offer a smart-alarm feature (), none has been validated for this purpose. Smart alarms are based on the concept that the stimulus to awaken should be delivered when one is naturally transitioning to light sleep or wakefulness to avoid potential grogginess that may be associated with awakening from deeper NREM sleep stages. Instead of experimental validation, the marketing claims seem to be relying on face validity: the intuition that an alarm should align with times when one is already practically awake. A more appropriate term might be “transitive property validation”, because what makes the idea seem valid resides in some approximately related research. For the smart alarm, the reasoning might be: sleep inertia occurs in some people when awakened from slow wave sleep, so if the device alarm targets periods of movement, which rarely occur in deep sleep, then sleep inertia can be avoided and instead one awakens feeling more energetic. A recent study of a smart-phone app that performed similarly to actigraphy for gauging sleep versus wake in healthy adults failed to demonstrate the effectiveness of the smart-alarm feature.Citation3
The common implication across the marketing of consumer sleep monitors is that tracking sleep will lead to improved sleep. The claims are not intended to be “medical” in nature, such that the validation standards normally applied to medically used devices are not required, and accordingly the marketing content often includes caveats in this regard. However, the language found on the web sites can range from fairly benign (Garmin: “monitor your rest”) to rather hyperbolic (Reston: “most accurate sleep monitor ever”) (). Information available on the internet may influence consumer confidence, and certain language can come across as scientifically or medically valid. Consider as an example that FitBit’s chief technology officer was quoted in the USA Today regarding an individual for whom their device detected 83 wake-ups, which prompted the user to get tested for obstructive sleep apnea (OSA), adding that FitBit hears such stories “all the time”.Citation7 This device is not validated for any medical purpose, and certainly not intended to screen for OSA, yet such public anecdotes (especially from company leadership) may send a different message to consumers. The mismatch between marketing content and supporting validation makes answering the seemingly straightforward question more challenging: is there any potential use for consumer sleep devices in sleep medicine?
Sleep and the blurred boundary of wellness and medicine
Motivations for using consumer sleep monitors are surely diverse and could range from simple curiosity to wellness goals like self-improvement through optimizing sleep patterns. Perhaps more concerning to physicians are the individuals with sleep-related concerns who seek some explanation or improvement via consumer devices before (or instead of) seeking medical advice. Consumers encountering the optimistic marketing claims of these sleep devices might not recognize the limitations and might even wonder why doctors do not use these devices more often in their practice. Providers, by contrast, are typically aware that even “medical-grade” actigraphy enjoys clinical utility in only limited situations,Citation8 such as assessing circadian rhythm disorder patterns in those who cannot or will not provide diary data, or verifying adequate sleep opportunity in the nights preceding a multiple sleep latency test. Providers might be skeptical of what is seen as largely unvalidated marketing claims and thus the potential utility of the devices remains questionable.
Although the wellness claims do not require regulation typical of medical devices, the practical issues raised by devices used at the interface of health care and wellness remain challenging. Primary sleep disorders have protean manifestations, and symptoms potentially attributable to poor sleep tend to be nonspecific. The boundary between sleep as wellness and sleep as a medical concern is hardly clear, and the overlap impacts interpretation of consumer device outputs. By comparison, even medically validated diagnostic technologies are at risk for false positive and false negative results. Such risks are influenced by the Bayesian triad of sensitivity, specificity, and pretest probability of the disease in question.Citation9 Likewise, the potential for false positive and false negative outcomes can be understood if we consider consumer sleep devices in this framework.
illustrates the potential for confusion regarding device performance accuracy across a simplified context often invoked to understand diagnostic testing: dichotomous health status (here, the presence or absence of an objective sleep disorder), and the dichotomous presence or absence of sleep-related symptoms. Consider an asymptomatic individual who uses a consumer sleep device, and is faced with interpreting the sleep “score” output. A poor score would be a false alarm if the person in fact had healthy sleep, while a good score would be a false reassurance if the person in fact had an occult primary sleep disorder. The asymptomatic individual might not appreciate that occult sleep disorders are not uncommon, and thus not appreciate that false negative results are possible.
Figure 2 Schematic representation of symptoms, sleep health status, and consumer monitor output.
Abbreviations: TP, true positive; TN, true negative; FP, false positive; FN, false negative.
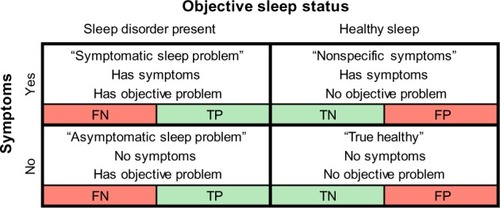
On the other hand, an individual with symptoms that could in principle be related to a sleep disorder is presented with a similar interpretation challenge. A good sleep score from a consumer device could represent false reassurance if a primary disorder is present, or it could be accurate if sleep was in fact healthy but the symptom (such as fatigue) had a nonsleep underlying cause (such as undiagnosed depression or hypothyroidism). By contrast, a poor sleep score in this setting could falsely steer the individual toward clinical sleep investigations and potentially delay diagnosis of the actual underlying problem.
The consumer knows which row to consider when interpreting their sleep score (ie, whether they have symptoms potentially linked to poor sleep), but they do not know the probability of their true sleep status being in the healthy versus affected column (). Thus, within each row, four possible interpretations exist for each individual using a sleep monitor, encompassing true and false positives, and true and false negatives. The probability of objective sleep disorder presence, known as the prior or pretest probability, is a necessary step to interpret any test result, such as the output of a consumer device. The other required pieces of information, sensitivity and specificity for discriminating healthy and affected states, are also not known, although the marketing language may lead consumers to believe that the outputs can accurately detect problems. Although a provider might have an intuition for the pretest probability, they have little validation data to navigate the uncertainty surrounding the accuracy of the device for quantifying any sleep disturbance in a clinical setting.
There are of course many other possibilities not captured by the dichotomies that are shown in . Consider an individual who uses a consumer monitor that detects snoring. Upon reviewing the monitor’s data suggesting that snoring is occurring, the individual then looks on the internet for snoring interventions, and learns that nasal spray or breathe-right strips may help. This may sound innocent enough, but the potential downside is clear: the person with snoring may or may not have OSA, and the consumer sleep monitor would not answer this important question. Perhaps of greater concern, if OSA was in fact present, an over-the-counter intervention that actually reduced the snoring would be unlikely to meaningfully reduce the OSA severity. Therefore, if the device output suggested reduced snoring, then false reassurance may occur unless the individual recognizes the potential accuracy limitations of snore detection, as well as the dissociation between snoring metrics and OSA severity metrics. How might a snore detection device be used more sensibly? Although one might use snoring as one component of a risk stratification approach, to place the issue of snore detectionCitation10 into context, consider that the entire eight question STOP-Bang screening tool,Citation11 of which snoring is just one question, has only modest screening value,Citation9 and had somewhat lower performance in other clinical studies.Citation12
Given this uncertainty, it is currently unclear how individual patients or providers might utilize data from consumer devices. One major concern is that consumers may conflate the rows and columns of , assigning symptom presence/absence as if it meant sleep disorder presence/absence. Together with possibly over-estimating device accuracy, this leads to problems at all levels of Bayesian inference when interpreting device outputs. At the population level, without knowing how many individuals fall into each of these categories, the scope of false reassurance, false concern, or true health status cannot be estimated. Important questions remain thus unanswered: How often do individuals first present to their physician seeking sleep evaluation based on consumer sleep device data? What portion of individuals who use consumer sleep monitors make a health care-related decision based on the data? What portion of the physicians (sleep specialists or otherwise) feel confident in interpreting the results of consumer devices?
Claim-validation mismatch: the many faces of “validation”
Validation is a broad concept, the details of which are useful to consider in the context of consumer sleep devices. Vernacular use of the word validity implies a sense of legitimacy such as might be achieved by testimonials, endorsement, or the “obviousness” known as face validity. We have discussed that transitive property validation is also common in the consumer space. These aspects of validation may be useful first steps, but are insufficient to address the potential utility of devices for clinical or research endeavors. describes different levels of validation to provide a framework for considering the potential utility of consumer sleep devices, and how validation can be achieved. Even when apparent validation data are available for a device, it is important to parse out the details regarding what aspect of sleep is being assessed by the sensors and the algorithms, what population was tested and in what context, and what external factors (sleep or medical disorders, alcohol, etc) might influence the performance in a clinical population. Because sleep is not a monolithic entity, any validation experiment or claim of a sleep monitor should be contextualized by specifying the device feature being assessed and the gold standard reference, in addition to other details of the study design and statistics.
Figure 3 Stages of validation for consumer sleep monitors.
Abbreviation: PSG, polysomnography.
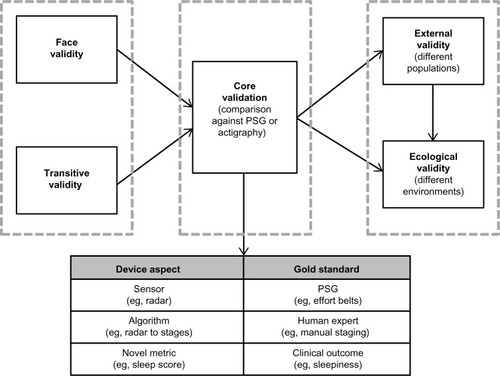
Essentially all consumer sleep devices can make claims within the minimal validation context of face validity or transitive property validity. The next step would be comparison of device performance against a gold standard, such as PSG or actigraphy. This important step should itself be subdivided, because the experimental approach could refer to the sensor itself (does it accurately measure the aspect of physiology it purports to measure), the algorithm used to map the sensed physiology to some output (such as sleep stage), and perhaps whether a novel metric is being related to a standard metric (eg, depth instead of stage). Few devices have published data in this category of validation against a gold standard in healthy individuals. Even at the sensor level, two devices ostensibly measuring the same aspect of human physiology might not be functionally equivalent in their hardware or software components. Previous studies have shown with actigraphy and pulse oximetry that the details of the particular sensors and algorithms impact device results.Citation13,Citation14 Presumably, the same issues would arise among consumer devices using a given technique, such as using wrist movement, to assess sleep–wake state.
The most important aspect of validation, however, is arguably to assess the robustness of the sensor and algorithm performance in settings beyond that of healthy adults in the sleep laboratory. External validity refers to the extent to which in-laboratory validation results can be extrapolated to populations that differed from those tested during the validation studies. For example, a laboratory validation of a consumer sleep monitor in healthy young adults might not extrapolate to device performance in an older population with objective sleep disturbance recorded by laboratory PSG. Ecological validity is a related concept, referring to the extent to which validation results can be extrapolated to different environments than those used during validation studies. For example, a laboratory validation of a consumer device in a population with objective sleep disturbance might not extrapolate to device performance when used by such patients in their homes. Further, user-specific factors such as differences in body position, tightness of the device, position of the device on the body (for wearables) or near the body (for noncontact sensors), or other behaviors might impact device outputs as well, even for devices that have been experimentally validated in certain settings, such as a controlled laboratory environment. The presence of a bed partner may impact sensor function or algorithm processing accuracy, perhaps more so if a sleep disorder is present in the partner.
Consumer sleep monitor validity: reframing the questions
Once the uncertainties regarding consumer device validation are appreciated, we can better address the question of what kinds of information, if any, could be reasonably extracted from these monitors to support clinical decision-making? The answer might depend on several patient-specific factors (type and severity of sleep disorder, comorbidities or medications, comfort level with different aspects of technology) and device-specific factors (type of sensor, algorithm accuracy for different aspects of sleep physiology and pathophysiology). In addition, user-specific factors and user-errors also play into the interpretation of consumer device data, such as how the device is worn (for wearable items) or where it is positioned on or near the bed (for the passive or noncontact monitors), or whether a bed partner is present. Even an apparently simple issue like needing to remember to start or stop recording could lead to nonrandom data loss during longitudinal home monitoring (eg, an individual might be more likely to forget to start recording when tired, or if alcohol was consumed). Consumer sleep devices that are easy to implement, or automatically start/stop recording, might allow for an increased likelihood of adherence. Recording multiple nights of data can capture night to night variability, avoid the first night effect that may occur in the laboratory setting, and facilitate pattern recognition linking sleep-related measurements with other behaviors (eg alcohol, medications, exercise).
By specifying potential goals for use of consumer sleep devices, one can develop a framework for assessing which devices, if any, could theoretically meet the goals, and thus inform how to undertake validation. Potential solutions surely will differ depending on context at the level of individual patient care decisions, versus large-scale epidemiological studies that combine self-reported health queries and objective monitoring. We will consider several categories of potential utility of consumer sleep devices ().
Table 2 Use categories
Can a consumer device replace or complement a diary of sleep and wake times?
For individuals who cannot or will not adhere to manually tracking their sleep and wake times, a device could theoretically substitute, if it was easy to use or had automated assessments of time in bed. Most currently available devices require the user to indicate when they are in bed trying to sleep, which itself requires some degree of adherence. From an epidemiology perspective, sleep duration is typically self-reported as an average or habitual estimate, and has led to speculation in regard to associations with long and short duration reports.Citation15,Citation16 Self-reported sleep–wake durations, especially in those with sleep complaints like insomnia, are well-known to contain a variety of uncertainties.Citation17 The question of how important the single facet of sleep duration may be for health is itself influenced by the nature of self-report; the technological advances of consumer sleep monitors hold promise for bringing objectivity and multi-night sampling to bear on this most basic epidemiological query.
Can a consumer device replace formal actigraphy?
The answer here depends on what aspect of actigraphy is desired. For gross patterns of time in bed versus time out of bed, nearly all of the devices on the market could accomplish this. For quantitative analysis of movements, and translating the sensor data into scoring of sleep/rest periods, wrist-worn movement devices would be preferred over cardio-pulmonary monitors, and formal validation studies would be required. This would include comparing the device sensor (accelerometer) against gold standard actigraphy, as well as the algorithm output. If application of novel metrics is based on activity counts, such as scale invariant correlationsCitation18 or transition probability metrics,Citation19 then, in addition, the capacity to export the raw movement signals would be required.
When formal actigraphy is used to track sleep patterns longitudinally in those with insomnia, perhaps to monitor therapeutic interventions, the same limitations applying to the interpretation of actigraphy data would be germane to the interpretation of a consumer device based on the wrist movement. Formal actigraphy tends to have high sensitivity and low specificity (approximately 90% and 50%, respectively) for detecting sleep.Citation20 Therefore, actigraphy can overestimate total sleep time for an insomniac, who may spend substantial time awake but not moving sufficiently to register with the algorithm. Individuals with primary underlying sleep disorders such as periodic limb movements or OSA might exhibit different performance accuracy of actigraphy analysis, yet actigraphy itself cannot detect these problems, so implementation of wrist actigraphy (by any device) should take this into consideration.
Can a consumer device be useful for insomnia management?
Although objective testing is not routinely recommended for clinical management of insomnia, recent evidence argues for the potential utility of objective sleep measurements for several reasons, and at-home multi-night tracking may be particularly useful in this population.Citation21 Epidemiology data incorporating objective testing suggest that medicalCitation22 and psychiatricCitation23 risks studied prospectively require the combination of insomnia symptoms and short objective sleep duration on PSG – suggesting that insomnia phenotyping according to objective measures may be important for risk stratification, risk-benefit assessment of hypnotics, and even prospective clinical trial design. Misperception among patients with chronic insomnia, which requires comparison of subjective report with objective measurement, could be better characterized with home monitoring to allow repeated measurements (is misperception a state or trait phenomenon?) and discover patterns. Feedback from sleep monitoring devices may be beneficial in patients with misperception.Citation24 The growing risk concerns surrounding even intermittent hypnotic use, together with the limited data supporting medical benefit of these drugs, highlight the use of nonpharmacological approaches such as cognitive-behavioral therapy.Citation25 Finally, increasing data suggesting occult sleep apnea occurs in patients with insomnia symptomsCitation26–Citation28 is a reminder that the choice of testing must consider the physiological realities beyond the clinical assessments that often channel patients to certain pathways.
Can a consumer device be useful for tracking sleep stages?
The rich experimental literature linking REM and N3 to cognitive performance has not enjoyed wide practical clinical appreciation, in part because of limited tools to augment these stages, but also because of wide individual variability. The uncertainty in the clinical validity of these experimental links is highlighted by a recent large study of healthy adults finding no relationship of episodic memory to stage architecture.Citation29 Having reliable home monitoring of sleep stage architecture would allow individuals to test possible relationships themselves through a combination of diary entries, device outputs, and perhaps performance tracking. The use of autonomic physiology to estimate sleep stages traditionally defined by electroencephalography involves trade-offs, such as the benefits of ease of use and simplifying automation, versus the risks of reduced estimation accuracy compared with the gold standard. Assuming no untreated primary disorder was present (such as occult OSA or occult periodic limb movements), an individual could in principle search for correlations over time, to answer two questions: are aspects of sleep architecture (stages, fragmentation, total duration) linked reliably to one or more aspects of daytime function (cognition, mood, etc), and are behaviors or experiences influencing sleep architecture (caffeine, exercise, alcohol, stress). Such data could be powerful across the spectrum of wellness and health.
Can a consumer device screen for sleep apnea?
Current methods of screening based on clinical features have limitations.Citation9 However, objective testing with limited-channel devices for OSA diagnosis is explicitly excluded for general screening use by the American Academy of Sleep Medicine guidelines.Citation30 Home monitoring might fill a niche here by adding an objective component to risk stratification. Analysis of single channels of data have already shown promise for quantifying sleep disordered breathing in certain settings,Citation31–Citation33 and also used to distinguish central from obstructive apnea.Citation34 Whether a combination of clinical information and a consumer wearable device could provide risk stratification is a testable hypothesis. Devices using only wrist movement would be unlikely to serve this purpose, whereas devices that recorded cardiac or respiratory parameters could be the focus of future validation.
Conclusion
It is no surprise that consumer sleep devices provide only a subset of the extensive physiology contained in laboratory PSG. Yet proper validation of these devices for specific purposes has strong potential for a variety of goals described earlier. The status quo, in which consumer devices contextualize their claims as “wellness”, can send conflicting messages, in which neither providers nor consumers can rationally navigate the device outputs (). The more widely these products enjoy consumption, the more urgently important it is for all stakeholders to engage in resolving the current state of claim-validation mismatch. Insurers are another group with potential interest in consumer devices for health tracking,Citation35 and the same validation issues are germane to this perspective as well. The question remains whether devices can add value in clinical and research domains. Formal cost-effectiveness modeling will be informed by further validation data, as many factors have important influence such as device cost, technical robustness (failure rates), accuracy for the intended use, and downstream consequences of the actions based on device output. Although these are important concepts to consider, the time and resources required to establish external and ecological validity may not be prioritized in the consumer space, where even basic validation studies are often not available. Recognizing the validation limitations may nevertheless help pave the path forward. The widespread consumption of sleep monitors is a reminder that the stakes are high, and that prioritizing validation efforts, regardless of whether certain devices meet more rigorous medical standards, will be important for all stakeholder perspectives to understand the potential risks and benefits of sleep monitoring technology in the consumer space.
Supplementary material
Table S1 Operational features of common consumer sleep monitors
Disclosure
Matt T Bianchi receives funding from the Department of Neurology, Massachusetts General Hospital, the Center for Integration of Medicine and Innovative Technology, and the Milton Family Foundation. Matt T Bianchi also has a patent pending on a home sleep monitoring device and has received travel funding from Servier, has consulting agreements with Foramis, MC10, Insomnisolv, and GrandRounds, and has provided expert testimony in sleep medicine. The other authors report no conflict of interest in this work.
References
- The most amazing inventions of 2005Time166New York200591
- KoPTKientzJAChoeEKKayMLandisCAWatsonNFConsumer sleep technologies: a review of the landscapeJ Clin Sleep Med2015piijc-00498-14
- BhatSFerrarisAGuptaDIs there a clinical role for smartphone sleep apps? comparison of sleep cycle detection by a smartphone application to polysomnographyJ Clin Sleep Med201511770971525766719
- BeharJRoebuckADomingosJSGederiECliffordGDA review of current sleep screening applications for smartphonesPhysiol Meas2013347R29R4623771214
- ComstockJ database on the internet2015 Available from: http://mobihealthnews.com/43499/class-action-lawsuit-alleges-fitbit-misled-buyers-with-inaccurate-sleep-tracking/Accessed May 17, 2015
- AserinskyEKleitmanNRegularly occurring periods of eye motility, and concomitant phenomena, during sleepScience1953118306227327413089671
- PainterKSleep-tracking gadgets raise awareness – and skepticismU S A Today2013 Available from: http://www.usatoday.com/story/news/nation/2013/03/24/sleep-tracking-devices/2007085/Accessed July 30, 2015
- MorgenthalerTILee-ChiongTAlessiCPractice parameters for the clinical evaluation and treatment of circadian rhythm sleep disorders. An American Academy of Sleep Medicine reportSleep200730111445145918041479
- BianchiMTScreening for obstructive sleep apnea: Bayes weighs inOpen Sleep J200925659
- JinHLeeLASongLAcoustic analysis of snoring in the diagnosis of obstructive sleep apnea syndrome: a call for more rigorous studiesJ Clin Sleep Med201511776577125766705
- ChungFYegneswaranBLiaoPSTOP questionnaire: a tool to screen patients for obstructive sleep apneaAnesthesiology2008108581282118431116
- BoyntonGVahabzadehAHammoudSRuzickaDLChervinRDValidation of the STOP-BANG questionnaire among patients referred for suspected obstructive sleep apneaJ Sleep Disord Treat Care201324
- WeissARJohnsonNLBergerNARedlineSValidity of activity-based devices to estimate sleepJ Clin Sleep Med20106433634220726281
- BohningNSchultheissBEilersSPenzelTBohningWSchmittendorfEComparability of pulse oximeters used in sleep medicine for the screening of OSAPhysiol Meas201031787588820505219
- BliwiseDLYoungTBThe parable of parabola: what the U-shaped curve can and cannot tell us about sleepSleep200730121614161518246971
- KurinaLMMcClintockMKChenJHWaiteLJThistedRALauderdaleDSSleep duration and all-cause mortality: a critical review of measurement and associationsAnn Epidemiol201323636137023622956
- MillerCBGordonCJToubiaLAgreement between simple questions about sleep duration and sleep diaries in a large online surveySleep Health20151133137
- HuKVan SomerenEJSheaSAScheerFAReduction of scale invariance of activity fluctuations with aging and Alzheimer’s disease: involvement of the circadian pacemakerProc Natl Acad Sci U S A200910682490249419202078
- LimASYuLCostaMDQuantification of the fragmentation of rest-activity patterns in elderly individuals using a state transition analysisSleep201134111569158122043128
- SadehAThe role and validity of actigraphy in sleep medicine: an updateSleep Med Rev201115425926721237680
- Sanchez-OrtunoMMEdingerJDInternight sleep variability: its clinical significance and responsiveness to treatment in primary and comorbid insomniaJ Sleep Res Oct2012215527534
- VgontzasANFernandez-MendozaJLiaoDBixlerEOInsomnia with objective short sleep duration: the most biologically severe phenotype of the disorderSleep Med Rev201317424125423419741
- Fernandez-MendozaJSheaSVgontzasANCalhounSLLiaoDBixlerEOInsomnia and incident depression: role of objective sleep duration and natural historyJ Sleep Res201524439039825728794
- HarveyAGTangNK(Mis)perception of sleep in insomnia: a puzzle and a resolutionPsychol Bull201213817710121967449
- EdingerJDMeansMKCognitive-behavioral therapy for primary insomniaClin Psychol Rev200525553955815951083
- KrakowBUlibarriVARomeroEPersistent insomnia in chronic hypnotic users presenting to a sleep medical center: a retrospective chart review of 137 consecutive patientsJ Nerv Ment Dis20101981073474120921864
- LichsteinKLRiedelBWLesterKWAguillardRNOccult sleep apnea in a recruited sample of older adults with insomniaJ Consult Clin Psychol199967340541010369061
- BianchiMTGoparajuBMoroMSleep apnea in patients reporting insomnia or restless legs symptomsActa Neurol Scand Epub2015514
- AckermannSHartmannFPapassotiropoulosAde QuervainDJRaschBNo associations between interindividual differences in sleep parameters and episodic memory consolidationSleep201538695195925325488
- CollopNAAndersonWMBoehleckeBClinical guidelines for the use of unattended portable monitors in the diagnosis of obstructive sleep apnea in adult patients. Portable Monitoring Task Force of the American Academy of Sleep MedicineJ Clin Sleep Med20073773774718198809
- BianchiMTLipomaTDarlingCAlameddineYWestoverMBAutomated sleep apnea quantification based on respiratory movementInt J Med Sci201411879680224936142
- GroverSSPittmanSDAutomated detection of sleep disordered breathing using a nasal pressure monitoring deviceSleep Breath200812433934518368430
- AmirOBarak-ShinarDHenryASmartFWPhotoplethysmography as a single source for analysis of sleep-disordered breathing in patients with severe cardiovascular diseaseJ Sleep Res20122119410021672069
- ThomasRJMietusJEPengCKDifferentiating obstructive from central and complex sleep apnea using an automated electrocardiogram-based methodSleep200730121756176918246985
- OlsonPWearable tech is plugging into health insuranceForbes2014 Available from: http://www.forbes.com/sites/parmyolson/2014/06/19/wearable-tech-health-insurance/Accessed July 1, 2015
- mybasis.com/ [homepage on the Internet]Basis| Heart Rate Monitor and Fitness TrackerAccessed on Oct 29, 2015
- beddit.com/features#sleepscore. [homepage on the Internet]Beddit Sleep Tracker: World’s first ambient sleep monitorAccessed on Oct 29, 2015
- fitbit.com/charge. [homepage on the Internet]Fitbit Official Site for Activity Trackers and MoreAccessed on Oct 29, 2015
- sites.garmin.com/en-US/vivo/vivofit/. [homepage on the Internet]vivofit 2 | Activity Tracker | GarminAccessed on Oct 29, 2015
- hexoskin.com. [homepage on the Internet]HexoskinAccessed on Oct 29, 2015
- jawbone.com/store/buy/up3. [homepage on the Internet.]The world’s most advanced trackerAccessed on Oct 29, 2015
- support.lark.com/hc/en-us. [homepage on the Internet.]Accessed on Oct 29, 2015
- misfit.com/products/shine. [homepage on the Internet.]Mesfit Shine | Premium Fitness + Sleep MonitorAccessed on Oct 29, 2015
- resmed.com/us/en/consumer/s-plus.html. [homepage on the Intenet.]S+ sleep monitoring device| ResMed.comAccessed on Oct 29, 2015
- indiegogo.com/projects/reston-more-than-a-sleep-monitor#/story [homepage on the Internet.]RestOnAccessed on Oct 29, 2015
- sleeprate.com/. [homepage on the Internet.]SleepRate: Sleep-Life Balance ProgramAccessed on Oct 29, 2015
- sleeptracker.com. [homepage on the Internet.]Sleeptracker®-PremiumAccessed on Oct 29, 2015
- withings.com/us/en/products/aura? [homepage on the Internet.]Withings Aura Connected Alarm ClockAccessed on Oct 29, 2015