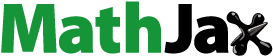
Abstract
Objective
This study aimed to identify prognostic genes for diffuse large B-cell lymphoma (DLBCL), using bioinformatic methods.
Methods
Five gene expression data sets were downloaded from the Gene Expression Omnibus database. Significance analysis of microarrays algorithm was used to identify differentially expressed genes (DEGs) from two data sets. Functional enrichment analysis was performed for the DEGs with the Database for Annotation, Visualization and Integration Discovery (DAVID). Survival analysis was performed with the Kaplan–Meier method using function survfit from package survival of R for the other three data sets. Cox univariate regression analysis was used to further screen out prognostic genes.
Results
Thirty-one common DEGs were identified in the two data sets, mainly enriched in the regulation of lymphocyte activation, immune response, and interleukin-mediated signaling pathway. Combined with 47 DLBCL-related genes acquired by literature retrieval, a total of 78 potential prognostic genes were obtained. Cases from the other three data sets were used in hierarchical clustering, and the 78 genes could cluster them into several subtypes with significant differences in survival curves. Cox univariate regression analysis revealed 45, 33, and eleven prognostic genes in the three data sets, respectively. Five common prognostic genes were revealed, including LCP2, TNFRSF9, FUT8, IRF4, and TLE1, among which LCP2, FUT8, and TLE1 were novel prognostic genes.
Conclusion
Five prognostic genes of DLBCL were identified in this study. They could not only be used for molecular subtyping of DLBCL but also be potential targets for treatment.
Introduction
Diffuse large B-cell lymphoma (DLBCL) is one of the most common types of non-Hodgkin lymphoma, which occurs primarily in older individuals. It is an aggressive tumor. R-CHOP, an improved form of cyclophosphamide, doxorubicin, vincristine, and prednisone (CHOP) with the addition of rituximab, is a standard treatment for DLBCL.
Many subtypes of the lymphoid neoplasms are established based on the World Health Organization classification system, and DLBCL is the most common type in Asians.Citation1 However, classification merely based on morphology and clinical information is difficult and thus a considerable percentage of cases are not classified. Gene expression profiling studies have attempted to distinguish heterogeneous groups of DLBCL from each other.Citation2–Citation4 For instance, by gene expression profile, two groupings of germinal center B-cell-like and the activated B-cell-like were identified as two DLBCL subtypes in the current World Health Organization classification.Citation5 The study by Lenz et alCitation6 provides genetic evidence that the DLBCL subtypes are distinct diseases that use different oncogenic pathways. Obviously, DNA microarrays provide a better understanding of the biology of DLBCL and advance the development of novel diagnostic tools.Citation7
Meanwhile, many genes with prognostic effect have been reported in DLBCL, such as BCL2Citation8 and BCL6.Citation9 Hu et alCitation10 suggested that MYC/BCL2 coexpression, rather than cell-of-origin classification, is a better predictor of prognosis in patients with DLBCL treated with R-CHOP. Additionally, Gratzinger et alCitation11 reported the prognostic value of vascular endothelial growth factor and vascular endothelial growth factor receptors in DLBCL patients treated with anthracycline-based chemotherapy. Besides, Hussain et alCitation12 found that X-linked inhibitor of apoptosis expression is a poor prognostic factor for DLBCL.
Due to the heterogeneity of DLBCL, more works are necessary to advance molecular subtyping as well as to discover the prognostic genes. In this study, two gene expression data sets were analyzed to identify differentially expressed genes (DEGs), which were regarded as potential prognostic genes for DLBCL, and to ascertain whether these genes would be used to well distinguish the subtypes of DLBCL in other three expression profile data sets.
Methods
Gene expression data
All the five gene expression data sets were downloaded from the Gene Expression Omnibus.
The data set of GSE32918Citation13,Citation14 collected gene expression profiles of 172 DLBCL samples. The platform of Illumina GPL8432 (Illumina HumanRef-8 WG-DASL v3.0) was used. It included a total of 294 sequencing data since some samples were sequenced repeatedly.
The data set of GSE10846Citation15,Citation16 included gene expression profiles of 181 clinical samples from chemotherapy-treated patients and 233 clinical samples from rituximab–chemotherapy-treated patients. The platform was Affymetrix GPL570 (Affymetrix Human Genome U133 Plus 2.0 Array). A total of 416 gene expression data were included.
The data set of GSE11318Citation6 consisted of gene expression profiles of 203 DLBCL samples, based on the platform of Affymetrix GPL570.
The data set of GSE9327Citation17 collected gene expression profiles of 36 DLBCL samples and eight reactive lymph nodes samples, which were used as controls. The platform of GPL6011 (CNIO Human Oncochip 1.0, 1.2, and 2.0) was used.
The data set of GSE30881Citation18 contained gene expression profiles of 23 DLBCL samples and ten healthy controls, in order to investigate the changes in NF-κB pathway activation. The platform was Affymetrix GPL3738 (Affymetrix Canine Genome 2.0 Array).
Pretreatment of raw data
Probes were mapped to genes according to the annotation files. For a gene corresponding to more than one probe, the average probe value was calculated as the gene expression value for the specific gene.Citation19 Subsequently, log2 conversion and quantile normalizationCitation20 were applied on the data.
A total of 4,356 and 16,454 unique genes were identified in GSE9327 and GSE30881, respectively. Both GSE10846 and GSE11318 were obtained using GPL570, and a total of 20,693 unique genes were acquired. Besides, 18,403 unique genes were identified in GSE32918.
Clinical information
The expression profiles of GSE10846 and GSE11318 provided clinical information such as age, sex, stage, lactate dehydrogenase (LDH) level, extranodal versus nodal presentation, treatment, subtype, survival time, and survival status. GSE32918 described age, sex, treatment, subtype, survival time, and survival status. According to these three data sets, we found that “stage” could well separate samples into different groups with diverse survival time while “age”, “sex”, and “treatment” could not.
Screening of DEGs
Significance analysis of microarrays algorithmCitation21 was adopted to screen out DEGs. It can reduce the false-positive rate in multiple testing via controlling false discovery rate. Relative difference (statistic d) is calculated as follows:
(1)
Statistic d measures the relative differences in gene expression levels, and it is the corrected t.
represents the average expression level of a gene under certain state,
represents the average expression level of a gene under another state, and s represents the variance of a gene.
Adjusted P-value <0.05 and log |fold change| >1.5 were set as the threshold to select the DEGs.
Functional enrichment analysis
Gene ontology enrichment analysis and Kyoto Encyclopedia of Genes and Genomes pathway enrichment analysis were performed for the DEGs with DAVIDCitation22 to examine the potential altered functions and pathways of these DEGs. False discovery rate <0.05 was set as the cutoff.
Survival analysis
Kaplan–Meier method (K–M method; product-limit method) is suitable for analysis with small sample size. The analysis procedure is as follows: 1) Put the samples in ascending order according to the survival time, rank i=1, 2, …, n. 2) List the number of surviving at the beginning of each time point (in fact, a short time). 3) Calculate the probability of death at each time point q and survival probability p (p=1−q). 4) Calculate the survival rate S(ti) for each time point, which equals to the product of each survival probability from the starting point to ti. S(ti)=p1×p2×p3 … pti. Finally, plot survival curves with survival time in abscissa and survival rate in ordinate.
Survival analysis was performed with function survfit from package survival of R.Citation23 Difference in survival curves for two groups was analyzed with log-rank method using function survdiff from package survival.Citation24
Screening of risk factors
Cox univariate regression analysis was carried out using function coxph from package survival to screen out risk factors related to survival.Citation25 The formula is as follows:
(2)
h0(t) is the basic risk function, the risk function when all covariates X1, X2, …, Xm are 0 or under standard conditions, and it is generally unknown. h (t, x) represents the risk function when each covariate X is given a fixed value, and it is proportional to h0(t). Therefore, the model is also known as the proportional hazard model. X1, X2, …, Xm are covariates while β1, β2, …, βm are regression coefficients. When the regression coefficient βi>0, that is, the risk ratio >1, it indicates that the covariate is a risk factor. The greater the covariate is, the shorter the survival time is. When the regression coefficient βi<0, that is, the risk ratio <1, it indicates that the covariate is a protective factor, so the greater the covariate is, the longer the survival time is.
Results
Differentially expressed genes and enriched biological functions
According to the aforementioned criteria, a total of 437 DEGs were identified in DLBCL from the data set GSE9327 and 1,457 DEGs from the data set GSE30881. Thirty-one overlapping genes were selected out and functional enrichment analysis was performed for these genes, which are mainly involved in the regulation of lymphocyte activation, immune response, and interleukin-mediated signaling pathway (), suggesting that the 31 DEGs were closely associated with the development of DLBCL.
Figure 1 Functional enrichment analysis result for the 31 differentially expressed genes (DEGs) (top 20 gene ontology [GO] terms ranked by the significance).
Abbreviation: IL, interleukin.
![Figure 1 Functional enrichment analysis result for the 31 differentially expressed genes (DEGs) (top 20 gene ontology [GO] terms ranked by the significance).](/cms/asset/65213368-f4ee-4244-bdde-dac1c384171e/dott_a_12199288_f0001_c.jpg)
Moreover, 47 DLBCL-related genes were acquired via literature retrieval.Citation2,Citation15,Citation26–Citation31
Survival analysis result
The 31 DEGs and 47 DLBCL-related genes were combined and a total of 78 potential prognostic genes were obtained, which were used to classify samples with diverse survival time from other three data sets.
In the data set of GSE10846, 71 out of the 78 genes were detected. Using hierarchical clustering, the 71 genes could well cluster the 416 DLBCL samples into four subtypes (). The differences in survival curves of the four subtypes were found to be significant (P=7.65e−11; ).
In the data set of GSE11318, 71 out of the 78 genes were detected. Using hierarchical clustering, the 71 genes could well classify the 203 DLBCL samples into three subtypes (). The difference in survival curves of the three subtypes was found to be significant (P=7.5e−05; ).
In the data set of GSE32918, 69 out of the 78 genes were detected. Some samples were sequenced repeatedly, and thus average expression levels were calculated as the final values. Using hierarchical clustering, the 69 genes could cluster the 172 DLBCL samples into three subtypes (). The difference in survival curves of the three subtypes was found to be significant (P=0.013; ).
Figure 2 Subtyping of diffuse large B-cell lymphoma (DLBCL) in three gene data sets using the 78 predicted and curated DLBCL-related genes.
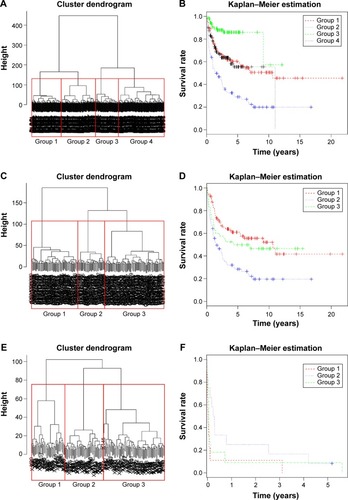
Prognostic genes
The correlation between each gene and the survival of DLBCL patients was calculated with Cox univariate regression analysis to further screen out genes with prognostic value. In the data set of GSE10846, 45 genes were found to have significant prognostic effect, while in GSE11318, 33 genes had prognostic effect, and in GSE32918, eleven genes showed prognostic value. Five prognostic genes were common among the three data sets (; ). According to the coefficient, lymphocyte cytosolic protein 2 (LCP2) and tumor necrosis factor receptor superfamily member 9 (TNFRSF9) might be related to poor prognosis while fucosyltransferase 8 (FUT8), interferon regulatory factor 4 (IRF4), and transducin-like enhancer of split 1 (TLE1) might bring in favorable prognosis.
Figure 3 Venn diagram of the prognostic genes from three gene expression data sets (GSE10846, GSE11318, and GSE21918).
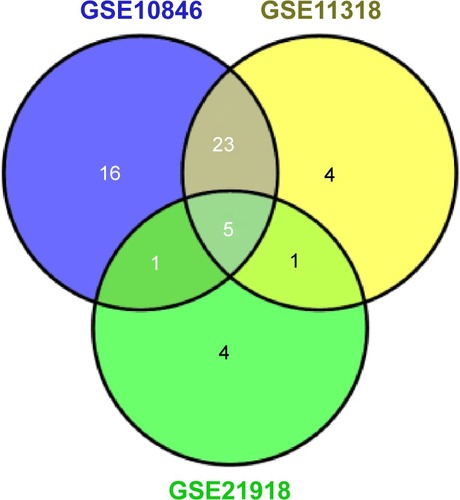
Table 1 Five common prognostic genes
Discussion
In this study, five gene expression data sets were downloaded from the Gene Expression Omnibus. Thirty-one common DEGs were identified from two gene expression data sets, mainly enriching in the regulation of lymphocyte activation, immune response, and interleukin-mediated signaling pathway, which were closely associated with the development of DLBCL. Combined with 47 DLBCL-related genes acquired by literature retrieval, 78 potential prognostic genes were obtained, which could successfully cluster the DLBCL samples from another three gene expression data sets into several subtypes with significant differences in survival. Prognostic genes were screened out via Cox univariate regression analysis, and five common genes were acquired, such as LCP2, TNFRSF9, FUT8, IRF4, and TLE1.
TNFRSF9Citation32 and IRF4Citation33 are two known prognostic genes of DLBCL. TNFRSF9 is a member of the TNF-receptor superfamily that can induce proliferation in peripheral monocytes. Alizadeh et alCitation32 indicate that expression levels of LIM domain only 2 (LMO2) and TNFRSF9 powerfully predict the overall survival in patients with DLBCL. TNFRSF9 can also serve as the target to treat DLBCL. The study by Houot et alCitation34 demonstrates that anti-CD137 therapy has a potent antilymphoma activity in a mouse model. IRF4 belongs to the interferon regulatory factor (IRF) family of transcription factors. Salaverria et alCitation35 report that translocations activating IRF4 identify a subtype of germinal center-derived B-cell lymphoma affecting predominantly children and young adults. Therefore, it may be a therapeutic target of DLBCL.Citation36
LCP2, FUT8, and TLE1 may be novel prognostic genes of DLBCL. LCP2 plays a positive role in promoting T-cell development and activation as well as mast cell and platelet function. FUT8 is an enzyme belonging to the family of fucosyltransferases. It may contribute to the malignancy of cancer cells and to their invasive and metastatic capabilities.Citation37 Chen et alCitation38 found that FUT8 is upregulated during epithelial–mesenchymal transition via the transactivation of β-catenin/lymphoid enhancer-binding factor (LEF)-1. Based on these instances, we speculated that FUT8 might exert a similar role in DLBCL and thus contributes to the metastasis of DLBCL. TLE1 is a multitasked transcriptional corepressor that acts through the acute myelogenous leukemia 1, Wnt, and Notch signaling pathways. Promoter CpG island hypermethylation-associated inactivation of TLE1 has been observed in DLBCL.Citation39 Fraga et alCitation40 further point out that TLE1 epigenetic inactivation contributes to the development of hematologic malignancies by disrupting critical differentiation and growth-suppressing pathways. However, the exact role of TLE1 in DLBCL remains to be explored. We supposed that more researches may unveil clinical applications of the three genes.
Overall, five critical genes with prognostic effect were disclosed in DLBCL via bioinformatic analysis of existing gene expression data. Two out of the five genes have been reported while the other three are novel predictors. Further researches on these genes can benefit molecular subtyping and also provide potential therapeutic targets of DLBCL.
Highlights
A set of 31 common DEGs were identified from two gene expression data sets.
Totally, 78 potential prognostic genes were suggested be used for subtyping of DLBCL.
Five prognostic genes, including three novel ones, were identified in DLBCL.
Disclosure
The authors report no conflicts of interest in this work.
References
- MortonLMWangSSDevesaSSHartgePWeisenburgerDDLinetMSLymphoma incidence patterns by WHO subtype in the United States, 1992–2001Blood2006107126527616150940
- AlizadehAAEisenMBDavisREDistinct types of diffuse large B-cell lymphoma identified by gene expression profilingNature2000403676950351110676951
- HoefnagelJJDijkmanRBassoKDistinct types of primary cutaneous large B-cell lymphoma identified by gene expression profilingBlood200510593671367815308563
- ViscoCLiYXu-MonetteZYComprehensive gene expression profiling and immunohistochemical studies support application of immunophenotypic algorithm for molecular subtype classification in diffuse large B-cell lymphoma: a report from the International DLBCL Rituximab-CHOP Consortium Program StudyLeukemia20122692103211322437443
- XuQTanCNiSIdentification and validation of a two-gene expression index for subtype classification and prognosis in diffuse large B-cell lymphomaSci Rep201551000625940947
- LenzGWrightGWEmreNCMolecular subtypes of diffuse large B-cell lymphoma arise by distinct genetic pathwaysProc Natl Acad Sci U S A200810536135201352518765795
- LossosISMorgenszternDPrognostic biomarkers in diffuse large B-cell lymphomaJ Clin Oncol2006246995100716418498
- DunleavyKWilsonWHDifferential role of BCL2 in molecular subtypes of diffuse large B-cell lymphomaClin Cancer Res201117247505750722184285
- WinterJNWellerEAHorningSJPrognostic significance of Bcl-6 protein expression in DLBCL treated with CHOP or R-CHOP: a prospective correlative studyBlood2006107114207421316449523
- HuSXu-MonetteZYTzankovAMYC/BCL2 protein coexpression contributes to the inferior survival of activated B-cell subtype of diffuse large B-cell lymphoma and demonstrates high-risk gene expression signatures: a report from The International DLBCL Rituximab-CHOP Consortium ProgramBlood2013121204021403123449635
- GratzingerDZhaoSTibshiraniRJPrognostic significance of VEGF, VEGF receptors, and microvessel density in diffuse large B cell lymphoma treated with anthracycline-based chemotherapyLab Invest2008881384717998899
- HussainARUddinSAhmedMPrognostic significance of XIAP expression in DLBCL and effect of its inhibition on AKT signallingJ Pathol2010222218019020632385
- BarransSLCrouchSCareMAWhole genome expression profiling based on paraffin embedded tissue can be used to classify diffuse large B-cell lymphoma and predict clinical outcomeBr J Haematol2012159444145322970711
- CareMACoccoMLayeJPSPIB and BATF provide alternate determinants of IRF4 occupancy in diffuse large B-cell lymphoma linked to disease heterogeneityNucleic Acids Res201442127591761024875472
- LenzGWrightGDaveSSLymphoma/Leukemia Molecular Profiling ProjectStromal gene signatures in large-B-cell lymphomasN Engl J Med2008359222313232319038878
- Cardesa-SalzmannTMColomoLGutierrezGHigh microvessel density determines a poor outcome in patients with diffuse large B-cell lymphoma treated with rituximab plus chemotherapyHaematologica2011967996100121546504
- Ruiz-VelaAAggarwalMde la CuevaPLentiviral (HIV)-based RNA interference screen in human B-cell receptor regulatory networks reveals MCL1-induced oncogenic pathwaysBlood200811131665167618032706
- MudaliarMAHaggartRDMieleGComparative gene expression profiling identifies common molecular signatures of NF-kappaB activation in canine and human diffuse large B cell lymphoma (DLBCL)PLoS One201389e7259124023754
- MaHSchadtEEKaplanLMZhaoHCOSINE: condition-specific sub-network identification using a global optimization methodBioinformatics20112791290129821414987
- FerrariFBortoluzziSCoppeANovel definition files for human GeneChips based on GeneAnnotBMC Bioinformatics2007844618005434
- LarssonOWahlestedtCTimmonsJAConsiderations when using the significance analysis of microarrays (SAM) algorithmBMC Bioinformatics2005612915921534
- DennisGJrShermanBTHosackDADAVID: database for annotation, visualization, and integrated discoveryGenome Biol2003453
- XuYGaoXWangZNonparametric method of estimating survival functions containing right-censored and interval-censored dataSheng Wu Yi Xue Gong Cheng Xue Za Zhi201431226727225039125
- JonesMPCrowleyJA general class of nonparametric tests for survival analysisBiometrics19894511571702655728
- AndersenPAGR, Cox’s regression model for counting processes, a large sample studyAnn Stat19821020
- RosenwaldAWrightGChanWCLymphoma/Leukemia Molecular Profiling ProjectThe use of molecular profiling to predict survival after chemotherapy for diffuse large-B-cell lymphomaN Engl J Med2002346251937194712075054
- ShippMARossKNTamayoPDiffuse large B-cell lymphoma outcome prediction by gene-expression profiling and supervised machine learningNat Med200281687411786909
- LossosISCzerwinskiDKAlizadehAAPrediction of survival in diffuse large-B-cell lymphoma based on the expression of six genesN Engl J Med2004350181828183715115829
- CaiYDHuangTFengKYHuLXieLA unified 35-gene signature for both subtype classification and survival prediction in diffuse large B-cell lymphomasPLoS One201059e1272620856936
- RimszaLMUngerJMTomeMELeblancMLA strategy for full interrogation of prognostic gene expression patterns: exploring the biology of diffuse large B cell lymphomaPLoS One201168e2226721829609
- WrightGTanBRosenwaldAHurtEHWiestnerAStaudtLMA gene expression-based method to diagnose clinically distinct subgroups of diffuse large B cell lymphomaProc Natl Acad Sci U S A2003100179991999612900505
- AlizadehAAGentlesAJAlencarAJPrediction of survival in diffuse large B-cell lymphoma based on the expression of 2 genes reflecting tumor and microenvironmentBlood201111851350135821670469
- RichardsKLMotsinger-ReifAAChenHWGene profiling of canine B-cell lymphoma reveals germinal center and postgerminal center subtypes with different survival times, modeling human DLBCLCancer Res201373165029503923783577
- HouotRGoldsteinMJKohrtHETherapeutic effect of CD137 immunomodulation in lymphoma and its enhancement by Treg depletionBlood2009114163431343819641184
- SalaverriaIPhilippCOschliesIMolecular Mechanisms in Malignant Lymphomas Network Project of the Deutsche KrebshilfeGerman High-Grade Lymphoma Study GroupBerlin-Frankfurt-Münster-NHL trial groupTranslocations activating IRF4 identify a subtype of germinal center-derived B-cell lymphoma affecting predominantly children and young adultsBlood2011118113914721487109
- ShafferALEmreNCRomesserPBStaudtLMIRF4: immunity. malignancy! therapy?Clin Cancer Res20091592954296119383829
- ItoYMiyauchiAYoshidaHExpression of alpha1,6-fucosyltransferase (FUT8) in papillary carcinoma of the thyroid: its linkage to biological aggressiveness and anaplastic transformationCancer Lett2003200216717214568171
- ChenCYJanYHJuanYHFucosyltransferase 8 as a functional regulator of nonsmall cell lung cancerProc Natl Acad Sci U S A2013110263063523267084
- CastellanoGTorrisiELigrestiGYin Yang 1 overexpression in diffuse large B-cell lymphoma is associated with B-cell transformation and tumor progressionCell Cycle20109355756320081364
- FragaMFBerdascoMBallestarEEpigenetic inactivation of the Groucho homologue gene TLE1 in hematologic malignanciesCancer Res200868114116412218519670