Abstract
Background
Opioid abuse in chronic pain patients is a major public health issue, with rapidly increasing addiction rates and deaths from unintentional overdose more than quadrupling since 1999.
Purpose
This study seeks to determine the predictability of aberrant behavior to opioids using a comprehensive scoring algorithm incorporating phenotypic risk factors and neuroscience-associated single-nucleotide polymorphisms (SNPs).
Patients and methods
The Proove Opioid Risk (POR) algorithm determines the predictability of aberrant behavior to opioids using a comprehensive scoring algorithm incorporating phenotypic risk factors and neuroscience-associated SNPs. In a validation study with 258 subjects with diagnosed opioid use disorder (OUD) and 650 controls who reported using opioids, the POR successfully categorized patients at high and moderate risks of opioid misuse or abuse with 95.7% sensitivity. Regardless of changes in the prevalence of opioid misuse or abuse, the sensitivity of POR remained >95%.
Conclusion
The POR correctly stratifies patients into low-, moderate-, and high-risk categories to appropriately identify patients at need for additional guidance, monitoring, or treatment changes.
Introduction
Opioid abuse in chronic pain patients is a major public health issue, with rapidly increasing addiction rates and deaths from unintentional overdose more than quadrupling since 1999Citation1 (National Institute on Drug Abuse). Prescription opioid-related overdoses killed 183,000 people in the USA between 1999 and 2016.Citation2 Furthermore, the US Department of Health and Human Services estimates that opioid abuse, misuse, or dependence plagued >1.9 million people in 2013 alone.Citation3 In addition to the significant human costs, opioid abuse and dependence result in enormous health care costs. In 2012 alone, hospitalizations for opioid abuse and dependence resulted in approximately $15 billion in inpatient charges.Citation4 If that figure is extended to associated infections, the costs increase $700 million.Citation4 Both types of hospitalizations rely primarily on Medicaid for payment, illustrating the financial burden placed on taxpayers.Citation4
The issue of prescription opioid abuse, misuse, and addiction is increasing in the national spotlight, with the White House Initiative in 2011, the US Secretary of Health and Human Services targeted initiative in 2015, and focused efforts by the Center for Disease Control and Federal Drug Administration (CDC and FDA) to curb prescription opioid abuse. The prescription opioid epidemic has been characterized by adverse patient outcomes and large increases in sales and prescriptions: a striking statistic that the USA consumes 80% of the global opioid supply, while contributing only 4.6% of the world’s population.Citation5 Furthermore, between 1997 and 2007, the average sales of opioids per person increased 402%, with the greatest increases in methadone, oxycodone, fentanyl, hydromorphone, hydrocodone, and morphine, all demonstrating >200% increases in retail sales.Citation6
However, even with judicious and appropriate use of opioids, there are risks involved. Chronic pain is the most commonly cited reason for initial opioid use in those with opioid use disorder (OUD).Citation6 It is estimated that 11.2% of the American population suffers from chronic pain.Citation7 Of these patients, many are prescribed opioids, which has contributed to the prescription drug abuse epidemic. In addition to the risk to the patient, physicians treating pain disorders increasingly find themselves subject to criminal charges or civil lawsuits following opioid abuse or overdose by patients. To treat chronic pain patients effectively, while minimizing abuse, misuse, dependence, and addiction, it is imperative that clinicians have tools that can provide guidance on identifying patients most at risk of OUD.
Common tools used to assess risk include the Opioid Risk Tool (ORT)Citation8 and the Screener and Opioid Assessment for Patients with Pain (SOAPP®),Citation9 both of which rely on environmental factors, patients’ self-reports of behavior, and personal and family history. As opioid addiction, dependence, use, and misuse are multifaceted – with factors ranging from genetics to environmental factors, such as previous substance abuse, childhood sexual abuse, age, and stressCitation10 – these tools are useful and offer some predictive value in assessing risk. Published data state that the specificity of the SOAPP Revised (SOAPP®-R) to determine aberrant drug-related behavior is 52.0%Citation9 with a sensitivity of 80%. The ORTCitation8 describes its specificity and sensitivity using a single statistical measure known as a c statistic and found that the ORT c statistic is equal to 0.82 for men and 0.85 for women. However, according to the American Society of Addiction Medicine, genetic factors account for up to half of the likelihood that an individual will develop addiction.Citation11 Therefore, these clinical questionnaires may only capture a portion of the risk factors – and they, of course, are subject to inaccuracies due to memory bias and misreporting.
Additional studies, such as studies conducted in twins,Citation12–Citation14 provide further evidence for a significant degree of genetic contribution in substance abuse. Specifically, Tsuang et alCitation14 examined the genetic contribution to addiction to alcohol, marijuana, stimulants, and opiates and concluded that genetics contributed 44% of the variance in opioid abuse. These studies and others have identified single-nucleotide polymorphisms (SNPs) in mesocorticolimbic circuits to be associated with OUD.Citation15–Citation17 These SNPs lie within four important neurochemical pathways associated with the brain reward pathways – the serotonergic, endorphinergic, GABAergic, and dopaminergic circuits. Variant genes within these cascades have been shown to be predictive of individuals that exhibit aberrant, risky behaviors, such as the abuse of psychoactive substances.Citation20–Citation22
Genetic testing of these markers to inform risks associated with opioid use is already commercially available. The Proove Opioid Risk (POR) profile is a panel test that combines known phenotypic risk factors with validated genetic markers in a patented algorithm to predict risk of OUD with a high degree of sensitivity. The interplay between environment and genetics is widely acknowledged.Citation12,Citation23,Citation24 Therefore, an evaluation of both phenotypic and genetic risk is necessary to achieve predictive accuracy. In fact, a previous study evaluating the POR with both the ORT and SOAPP®-R demonstrated that there was a significant positive correlation among the three tests, but that the POR detected OUD risk determination with higher specificity than either ORT or SOAPP®-R.Citation25
The objective of this study was to evaluate the POR algorithm, which was developed in an independent discovery cohort of patients, to establish validated predictive accuracy, and to provide additional evidence to support the performance characteristics of the algorithm.
Patients and methods
Study population
This multicenter, observational study (study protocols 1JAN15-20CR and 1JAN15-14CR) was reviewed, approved, and overseen by Solutions IRB, an institutional review board licensed by the US Department of Health and Human Services, Office for Human Research Protections. All participants signed informed consent forms prior to data collection.
The study population () consisted of 258 patients diagnosed with OUD (defined as the International Statistical Classification of Diseases and Related Health Problems [ICD]-9 series code 304, equivalent to ICD-10 series code F11.20) and 650 control patients. OUD patients were identified solely using ICD codes. OUD diagnosis was independently conducted by patients’ medical professionals at their respective clinics. In addition to diagnostic coding, inclusion criteria for OUD patients involved confirmation of present experience of chronic noncancer pain, consumption of opioid medication as part of a pain-management plan, and fluency in English. In this study, OUD is the diagnostic term for OUD, rather than the physiological state of opioid-dependence alone. Control patients were those who were prescribed opioids for chronic pain and were diagnosed with lower back pain (ICD-9 series code 724.2, equivalent to ICD-10 series code M54.5), and no other diagnoses, no history of depression, anxiety, other mental health issues, or history of illicit and prescription drug abuse or misuse. Subjects were 18–89 years of age and predominantly of Caucasian ethnicity. Patients were enrolled from 24 study sites across the USA by site addiction specialists, including experts in internal medicine, family medicine, pain management, orthopedics, neurology, osteopathy, podiatry, mental health, and physical medicine and rehabilitation.
Table 1 Patient demographics
Data collection
Genomic DNA was isolated from buccal swabs obtained from each patient using a proprietary DNA isolation technique and DNA isolation kit (Macherey Nagel GmbH & Co, KG, Duren, Germany), according to the manufacturer’s instructions. Genotyping was performed using predesigned TaqMan® assays (Applied Biosystems, Foster City, CA, USA). Allele-specific fluorescence signals were distinguished by measuring end point 6-FAM or VIC fluorescence intensities at 508 and 560 nm, respectively, and genotypes were generated using Genotyper® Software V 1.3 (Applied Biosystems). The DNA elution buffer was used as a negative control, and K562 cell line DNA (Promega Corporation, Madison, WI, USA) was included in each batch of samples tested as positive control.
Phenotypic information was also collected, including whether patients had a personal history of alcoholism, personal history of illegal drug abuse, personal history of prescription drug abuse, family history of alcoholism, family history of illegal drug abuse, family history of prescription drug abuse, mental health disorders and/or depression, and whether or not they were 16–45 years old. This information was collected in a paper questionnaire that asked patients to give yes or no answers to the phenotypic factors indicated above.
The POR algorithm
A POR score and its associated risk stratification were calculated for each subject. The POR algorithm is a patent-protected, validated measure of opioid risk.Citation25 In short, it combines phenotypic and genotype information to calculate a risk score that correlates with high-, moderate-, or low-risk stratification of opioid dependence,Citation25 such that a score of 1–11 is associated with low-risk, 12–23 with moderate-risk, and ≥24 with high-risk of OUD. Low-risk denotes the subject is at low risk of OUD and the clinician may proceed with prescription opioid therapy; moderate-risk suggests the clinician should proceed with caution and may want to consider more routine urine drug testing and possibly limit the duration of opioid therapy; and high-risk suggests the physician may want to consider an alternative analgesic to improve patient outcomes, consider more routine urine drug testing, limit the duration of opioid therapy, consider titrating the patient off opioid therapy, maintain vigilant awareness of patient outcomes, and possibly consider medically-assisted treatment for detoxification.
The genetic markers used in the algorithm include 11 different SNPs that have been implicated in opioid abuse, misuse, dependence, or addiction (). Risk alleles for each SNP are weighted more heavily in an additive genetic model, and an overall higher panel score summed across SNPs represents an increased risk of OUD. This approach, which focuses on validated genetic variants, as opposed to comprehensive next-generation sequencing, is the preferred approach of many in the field.Citation26 The phenotypic factors tested include age (whether or not they were 16–45 years old),Citation27,Citation28 personal history of alcohol abuse,Citation23,Citation29 personal history of illegal drug abuse,Citation8,Citation30 personal history of prescription drug abuse,Citation31 and personal history of other mental health diseases including attention deficit disorder,Citation32 obsessive compulsive disorder,Citation33 bipolar disorder,Citation24 and schizophrenia.Citation34
Table 2 Proove Opioid Risk test panel markers
Statistical analyses
A Mann–Whitney U test was used to determine the statistical significance of the difference between the POR scores of the OUD group and control group. A cross-tab analysis was performed to assess the diagnostic performance of the POR as a comprehensive algorithm for the evaluation of OUD risk. Statistical analyses (implemented in R v3.2.5) included measures of sensitivity (the percentage of OUD patients correctly identified by POR scores), specificity (the percentage of control patients correctly identified by POR scores), positive likelihood ratio (PLR; the likelihood of identifying OUD patients using the POR), and negative likelihood ratio (NLR; the likelihood of identifying controls using the POR). For the risk stratification portion of the analysis, POR scores of OUD patients and controls were divided into low-, moderate-, and high-risk categories. To establish the exact POR scoring parameters for each category, POR scores were compared in OUD patients vs controls.
Results
Distribution of POR scores
The overall distribution of POR scores between patients in the OUD group (n=258) and the patients in the control group (n=650) is shown in . The mean POR score for the controls was 17.6 (median =17), with standard deviation of 5.07, whereas the mean POR score for patients with diagnosed OUD was 25.5 (median =26), with standard deviation of 9.05, demonstrating that the POR-predicted risk is increased in patients with diagnosed OUD (p=7.97×10−34).
Figure 1 Distribution of POR scores by OUD status.
Abbreviations: OUD, opioid use disorder; POR, Proove Opioid Risk; SD, standard deviation.
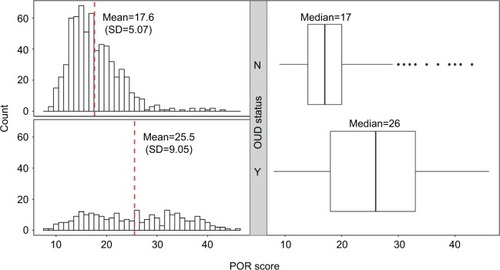
POR algorithm performance
To assess the performance of the POR for diagnosis of OUD, sensitivities and specificities were calculated across all possible POR scores (Table S1). The area under the curve (AUC) of the receiver operating characteristic (ROC) curve provides information about the accuracy of the test, where an AUC of 1 is equal to 100% accuracy and an AUC of 0.5 is equal to random chance. The AUC of the POR was 0.757 (95% confidence interval: 0.718–0.797), indicating that the POR accurately identified patients in this cohort >75% of the time (). The sensitivity of the POR score increased as OUD risk increased, with a sensitivity of 95.3% at a POR score of at least 12 (ie, at moderate and high risk of OUD). At different prevalence rates of OUD, 8% (prevalence of OUD in the general population), 28% (prevalence of OUD in the cohort), and 50% (a balanced prevalence rate), the POR algorithm performs equally well ().
Figure 2 ROC curve of POR predicting OUD status.
Abbreviations: AUC, area under the curve; OUD, opioid use disorder; POR, Proove Opioid Risk; ROC, receiver operating characteristic.
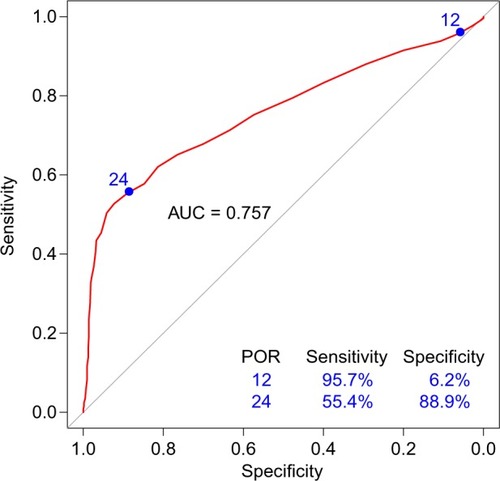
Figure 3 Performance of POR across different prevalence rates of OUD.
Abbreviations: OUD, opioid use disorder; POR, Proove Opioid Risk.
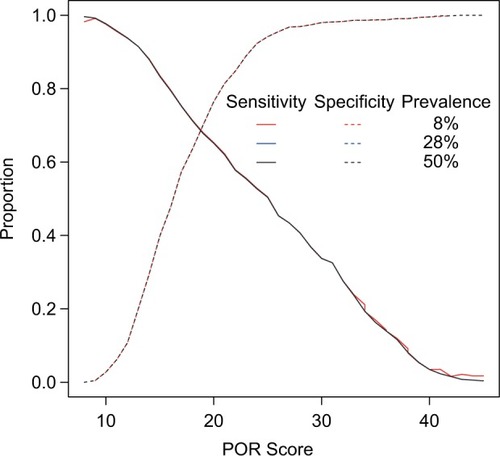
Odds of diagnosed OUD
In this cohort, when compared to controls, OUD patients identified by the POR algorithm to be at moderate risk had on average 4.17 increased odds of OUD, whereas those in the high-risk category had on average 16.5 increased odds of OUD ().
Figure 4 ORs of OUD in each POR risk stratification.
Abbreviations: OR, odds ratio; OUD, opioid use disorder; POR, Proove Opioid Risk.
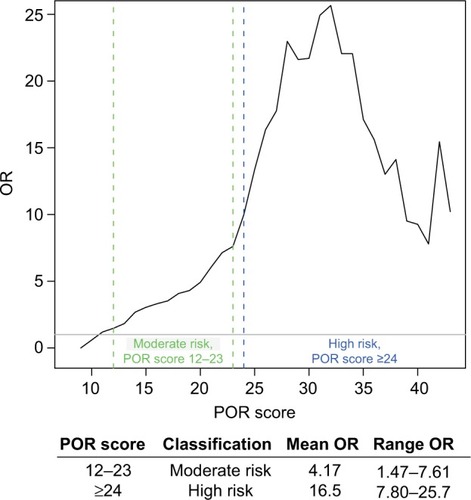
Likelihood of OUD
The PLR demonstrates the likelihood that a person with OUD would receive a positive POR test result, while the opposite is true for the NLR, which indicates the likelihood that a person with the condition would receive a negative test result. These ratios are based on the sensitivity and specificity of the test and do not change based on the prevalence of the disorder of interest. shows small (<2) increases in the likelihood of OUD for the POR scores of 8–19, moderate (2–10) increases in the likelihood of OUD for the POR scores of 20–26, and large (>10) increases in the likelihood of OUD for the POR scores of 27 and up except for the scores of 39–41, which had moderate likelihoods.
Figure 5 PLRs and NLRs of OUD across different prevalence rates.
Abbreviations: NLRs, negative likelihood ratios; OUD, opioid use disorder; PLRs, positive likelihood ratios; POR, Proove Opioid Risk.
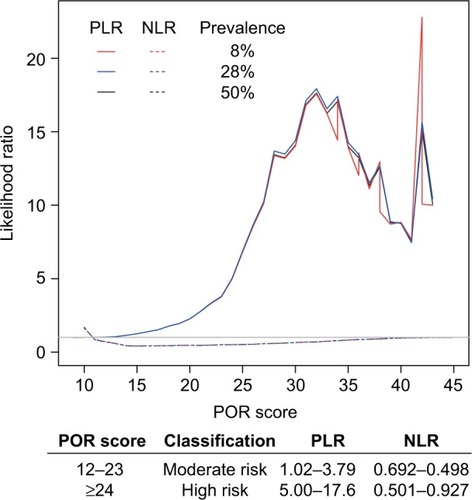
Discussion
The POR algorithm incorporates genetics and clinical factors to accurately predict a person’s risk of OUD. A previous discovery study demonstrated that the POR predicts risk of OUD with greater specificity than either the ORT or the SOAPP®.Citation25 This study confirms the utility of the POR, with an entirely new cohort of patients. In fact, the odds ratios calculated demonstrate a greatly increased rate of OUD for patients in the moderate- or high-risk category. Those in the moderate-risk category demonstrated an increased 4.17 odds of OUD (ranging from 1.47 to 7.61), and those in the high-risk category had an average of 16.5 increased odds of OUD (ranging from 3.79 to 17.6). Furthermore, based on the ROC curve, the POR is a good model for OUD, with an AUC of >75%. This statistic represents the accuracy of the test and demonstrates that it is capable of stratifying patients into different risk groups.
POR is used as decision tool to understand and act on risks of opioid-associated aberrant behaviors. For patients being evaluated for risk factors associated with a diagnosis of OUD, one would expect this tool to perform with high specificity, so there are few false negatives. The POR algorithm showed high specificity in this validation cohort, with high-risk scores being >90% specific. In contrast, for patients already prescribed opioids or those being screened for opioid use, this tool should capture the patients most at risk so appropriate cautionary measures can be taken. For this scenario, the POR tool should perform with high sensitivity, which may result in false positives, but is less likely to miss patients who are at risk. The POR algorithm showed increasing sensitivity with lower scores, with at least 90% sensitivity for scores ≤14, which indicates that patients of moderate-risk scores should be treated with caution. The high sensitivity of moderate-risk scores and the high specificity at high-risk scores validate the profile’s ability to capture, with good sensitivity, patients who should be monitored more closely but do not label patients inappropriately as high risk.
An algorithm that reliably predicts patients most at the risk of opioid addiction is a valuable tool in a clinician’s arsenal. By stratifying risk, physicians can appropriately identify those patients at higher risk of OUD and then more safely prescribe opioids in most of the population, which is low risk. This knowledge, as displayed by the POR algorithm, should lead to more rational decision-making by allowing patients access to necessary medication management and avoid exposure to those at elevated risk. The POR algorithm gives physicians the information they need about their most susceptible patients, which not only protects the patient but also protects the physician’s practice as governmental and law enforcement agencies elevate scrutiny on opioid prescribing and the physician. With the dramatic impact of the opioid abuse epidemic, the POR algorithm provides an evidence-based decision-making tool to improve clinical outcomes, reduce deaths and abuse, and potentially reduce health care costs.
Limitations
The limitations of this study include the wide age range of study participants and the reliance on ICD code for diagnosis of OUD. Future studies will include additional objective measures of drug use, including urine drug screening.
Conclusion
This study serves as an additional validation of the POR algorithm in identifying patients at the risk of OUD and concurrently identifying those patients at the lower risk of OUD, for whom opioid treatment may be a good option. There is a delicate balance between managing pain for chronic pain patients and preventing OUD. This algorithm provides clinicians with a tool to identify patients most at risk, reducing stress to the physician, increasing physician confidence in opioid prescribing, and reducing risk to the patient. The precision medicine approach of the POR can be used clinically to address the prescription opioid epidemic to guide health care decisions that increase patient and physician safety while decreasing health care costs.
Acknowledgments
We would like to thank the patients who participated in this study, without whom this study would not be possible. Proove Biosciences provided support for this study. The article has been presented at International Conference on Opioids, June 5–7, 2016, Boston, MA, USA.
Supplementary material
Table S1 Sensitivities and specificities of the POR algorithm using different cutoffs of POR scores to predict OUD
Disclosure
AB, SK, BM, and CL are the employees of Proove Biosciences. JB is a former employee of Proove Biosciences. MS and SR are on the Medical Advisory Board of Proove Biosciences. The authors report no other conflicts of interest in this work.
References
- NoraDVolkowMD webpage on the InternetAmerica’s Addiction to Opioids: Heroin and Prescription Drug Abuse2014 [cited August 30 2016]. Available from: https://www.drugabuse.gov/about-nida/legislative-activities/testimony-to-congress/2016/americas-addiction-to-opioids-heroin-prescription-drug-abuseAccessed March 6, 2017
- Center for Disease Control and PreventionPrescription Opioid Overdose Data https://www.cdc.gov/drugoverdose/data/overdose.html2017Accessed Mar 29, 2017
- SAMHSAResults from the 2013 National Survey on Drug Use and Health: Summary of National FindingsRockville, MDSAMHSA20147HHS (Publication No. SMA 14863, NSDUH Series H-48)
- RonanMVHerzigSJHospitalizations related to opioid abuse/dependence and associated serious infections increased sharply, 2002–12Health Aff (Millwood)201635583283727140989
- ManchikantiLFellowsBAilinaniHPampatiVTherapeutic use, abuse, and nonmedical use of opioids: a ten-year perspectivePain Physician201013540143520859312
- GriffinMLMcDermottKAMcHughRKFitzmauriceGMJamisonRNWeissRDLongitudinal association between pain severity and subsequent opioid use in prescription opioid dependent patients with chronic painDrug Alcohol Depend201616321622127161860
- NahinRLEstimates of pain prevalence and severity in adults: United States, 2012J Pain201516876978026028573
- WebsterLRWebsterRMPredicting aberrant behaviors in opioid-treated patients: preliminary validation of the Opioid Risk ToolPain Med20056643244216336480
- ButlerSFBudmanSHFernandezKCFanciulloGJJamisonRNCross-validation of a screener to predict opioid misuse in chronic pain patients (SOAPP-R)J Addict Med200932667320161199
- EdlundMJSteffickDHudsonTHarrisKMSullivanMRisk factors for clinically recognized opioid abuse and dependence among veterans using opioids for chronic non-cancer painPain2007129335536217449178
- ASAM [webpage on the Internet]Definition of Addiction Quality & Practice; ASAM Talks About What Addiction Is, Does, and Is Caused By2017 Available from: http://www.asam.org/quality-practice/definition-of-addictionAccessed March 6, 2017
- KendlerKSNealeMCSullivanPCoreyLAGardnerCOPrescottCAA population-based twin study in women of smoking initiation and nicotine dependencePsychol Med199929229930810218922
- TsuangMTLyonsMJHarleyRMGenetic and environmental influences on transitions in drug useBehav Genet199929647347910857252
- TsuangMTBarJLHarleyRMLyonsMJThe Harvard Twin Study of Substance Abuse: what we have learnedHarv Rev Psychiatry20019626727911600486
- Schwantes-AnTHZhangJChenLSAssociation of the OPRM1 variant rs1799971 (A118G) with non-specific liability to substance dependence in a collaborative de novo meta-analysis of European-Ancestry CohortsBehav Genet201646215116926392368
- GratacosMGonzálezJRMercaderJMde CidRUrretavizcayaMEstivillXBrain-derived neurotrophic factor Val66Met and psychiatric disorders: meta-analysis of case-control studies confirm association to substance-related disorders, eating disorders, and schizophreniaBiol Psychiatry200761791192217217930
- IsazaCHenaoJBeltránLGenetic variants associated with addictive behavior in Colombian addicted and non-addicted to heroin or cocaineColomb Med (Cali)2013441192524892317
- HaerianBSHaerianMSOPRM1 rs1799971 polymorphism and opioid dependence: evidence from a meta-analysisPharmacogenomics201314781382423651028
- LiDZhaoHKranzlerHRGenome-wide association study of copy number variations (CNVs) with opioid dependenceNeuropsychopharmacology20154041016102625345593
- NobleEPD2 dopamine receptor gene in psychiatric and neurologic disorders and its phenotypesAm J Med Genet B Neuropsychiatr Genet2003116b110312512497624
- CaoBJRodgersRJDopamine D4 receptor and anxiety: behavioural profiles of clozapine, L-745,870 and L-741,742 in the mouse plus-mazeEur J Pharmacol19973352–31171259369363
- NelsonECLynskeyMTHeathACAssociation of OPRD1 polymorphisms with heroin dependence in a large case-control seriesAddict Biol201419111112122500942
- JangKLLivesleyWJVernonPAAlcohol and drug problems: a multivariate behavioural genetic analysis of co-morbidityAddiction1995909121312217580819
- KhazaalYGex-FabryMNalletAAffective temperaments in alcohol and opiate addictionsPsychiatr Q201384442943823456370
- FarahJRLeeCKantorovichSSmithGAMeshkinBBrentonAEvaluation of a Predictive Algorithm that Detects Aberrant Use of Opioids in an Addiction Treatment CentreJ Addict Res Ther20178216
- LauschkeVMIngelman-SundbergMRequirements for comprehensive pharmacogenetic genotyping platformsPharmacogenomics201617891792427248710
- ClelandCMRosenblumAFongCMaxwellCAge differences in heroin and prescription opioid abuse among enrolees into opioid treatment programsSubst Abuse Treat Prev Policy201161121635762
- SprouleBBrandsBLiSCatz-BiroLChanging patterns in opioid addiction: characterizing users of oxycodone and other opioidsCan Fam Physician2009551686969.e169.e519155373
- EdlundMJSullivanMSteffickDHarrisKMWellsKBDo users of regularly prescribed opioids have higher rates of substance use problems than nonusers?Pain Med20078864765618028043
- Institute of Medicine Committee on Advancing Pain Research, C and Education, The National Academies Collection: Reports funded by National Institutes of HealthRelieving Pain in America: A Blueprint for Transforming Prevention, Care, Education, and ResearchWashington, DCNational Academies Press (US) National Academy of Sciences2011
- ComptonWMVolkowNDAbuse of prescription drugs and the risk of addictionDrug Alcohol Depend200683suppl 1S4S716563663
- DaigreCRonceroCGrau-LópezLAttention deficit hyperactivity disorder in cocaine-dependent adults: a psychiatric comorbidity analysisAm J Addict201322546647323952892
- FontenelleLFOostermeijerSHarrisonBJPantelisCYücelMObsessive-compulsive disorder, impulse control disorders and drug addiction: common features and potential treatmentsDrugs201171782784021568361
- KernAMAkermanSCNordstromBROpiate dependence in schizophrenia: case presentation and literature reviewJ Dual Diagn201410525725392062
- TassinJPUncoupling between noradrenergic and serotonergic neurons as a molecular basis of stable changes in behavior induced by repeated drugs of abuseBiochem Pharmacol2008751859717686465
- KranzlerHRModesto-LoweVVan KirkJNaltrexone vs. nefazodone for treatment of alcohol dependence. A placebo-controlled trialNeuropsychopharmacology200022549350310731624
- KoksSVasarEDeramciclane (Egis)Curr Opin Investig Drugs200232289294
- WadeAGCrawfordGMNemeroffCBCitalopram plus low-dose pipamperone versus citalopram plus placebo in patients with major depressive disorder: an 8-week, double-blind, randomized study on magnitude and timing of clinical responsePsychol Med201141102089209721349239
- BrousseGVorspanFKsoudaKCould the inter-individual variability in cocaine-induced psychotic effects influence the development of cocaine addiction? Towards a new pharmacogenetic approach to addictionsMed Hypotheses201075660060420801583
- Fernàndez-CastilloNRibasésMRonceroCCasasMGonzalvoBCormandBAssociation study between the DAT1, DBH and DRD2 genes and cocaine dependence in a Spanish samplePsychiatr Genet201020631732020505554
- JiNShuaiLChenYDopamine beta-hydroxylase gene associates with stroop color-word task performance in Han Chinese children with attention deficit/hyperactivity disorderAm J Med Genet B Neuropsychiatr Genet2011156B673073621761554
- CubellsJFSunXLiWLinkage analysis of plasma dopamine beta-hydroxylase activity in families of patients with schizophreniaHum Genet2011130563564321509519
- NymanESSulkavaSSoronenPInteraction of early environment, gender and genes of monoamine neurotransmission in the aetiology of depression in a large population-based Finnish birth cohortBMJ Open201111e000087
- Le FollBGalloALe StratYLuLGorwoodPGenetics of dopamine receptors and drug addiction: a comprehensive reviewBehav Pharmacol200920111719179847
- NavarroJFLunaGGarcíaFPedrazaCEffects of L-741,741, a selective dopamine receptor antagonist, on anxiety tested in the elevated plus-maze in miceMethods Find Exp Clin Pharmacol2003251454712690706
- GrossNBDunckerPCMarshallJFStriatal dopamine D1 and D2 receptors: widespread influences on methamphetamine-induced dopamine and serotonin neurotoxicitySynapse201165111144115521584865
- HanDHCraving for alcohol and food during treatment for alcohol dependence: modulation by T allele of 1519T>C GABAAalpha6Alcohol Clin Exp Res20083291593159918616664
- CarlezonWAJrBéguinCKnollATCohenBMKappa-opioid ligands in the study and treatment of mood disordersPharmacol Ther2009123333434319497337
- GelernterJGueorguievaRKranzlerHRVA Cooperative Study #425 Study GroupOpioid receptor gene (OPRM1, OPRK1, and OPRD1) variants and response to naltrexone treatment for alcohol dependence: results from the VA Cooperative StudyAlcohol Clin Exp Res200731455556317374034
- PeerboomsOLvan OsJDrukkerMMTHFR in Psychiatry GroupMeta-analysis of MTHFR gene variants in schizophrenia, bipolar disorder and unipolar depressive disorder: evidence for a common genetic vulnerability?Brain Behav Immun20112581530154321185933
- CeladaPPuigMAmargós-BoschMAdellAArtigasFThe therapeutic role of 5-HT1A and 5-HT2A receptors in depressionJ Psychiatry Neurosci200429425226515309042