Abstract
Background
Electronic health record (EHR) databases provide rich, longitudinal data on interactions with healthcare providers and can be used to advance research into respiratory conditions. However, since these data are primarily collected to support health care delivery, clinical coding can be inconsistent, resulting in inherent challenges in using these data for research purposes.
Methods
We systematically searched existing international literature and UK code repositories to find respiratory disease codelists for asthma from January 2018, and chronic obstructive pulmonary disease and respiratory tract infections from January 2020, based on prior searches. Medline searches using key terms provided in article lists. Full-text articles, supplementary files, and reference lists were examined for codelists, and codelists repositories were searched. A reproducible methodology for codelists creation was developed with recommended lists for each disease created based on multidisciplinary expert opinion and previously published literature.
Results
Medline searches returned 1126 asthma articles, 70 COPD articles, and 90 respiratory infection articles, with 3%, 22% and 5% including codelists, respectively. Repository searching returned 12 asthma, 23 COPD, and 64 respiratory infection codelists. We have systematically compiled respiratory disease codelists and from these derived recommended lists for use by researchers to find the most up-to-date and relevant respiratory disease codelists that can be tailored to individual research questions.
Conclusion
Few published papers include codelists, and where published diverse codelists were used, even when answering similar research questions. Whilst some advances have been made, greater consistency and transparency across studies using routine data to study respiratory diseases are needed.
Introduction
Electronic health record (EHR) databases include rich, longitudinal data on an individual’s interactions with health care providers. They comprise part of the clinical information systems which health care providers use during clinical consultations across primary, secondary and tertiary care. From these systems, data can then be extracted to enhance patient care through clinical research, healthcare planning, decision-making, and clinical audit. These routine data have been used to make significant advances in research into the epidemiology, burden, and natural history of respiratory disease, leading to improved prevention, detection and management, and to inform health service planning and policy.Citation1,Citation2 The scale of these data facilitates a wide range of research with high statistical power due to the in-depth variety of variables recorded and the number of patients contributing to the data, particularly as they are increasingly linked with other data sources.Citation28 However, EHRs are primarily populated to support health care delivery rather than research. This gives rise to challenges, including high volume and irregularly collected,Citation2 informatively observed (where data collection is driven by clinical requirements), missing, and incorrectly coded data.Citation3,Citation4
To study a health condition in EHR databases, an operational definition based on clinical codes is often used. Clinical codes are alphanumerical codes ascribed to specific clinical events or descriptions. Numerous code systems exist, and each diagnosis can have multiple clinical codes associated with it. Therefore, in order to search for a particular diagnosis, multiple clinical codes are required, constituting a clinical codelist.Citation5 The choice of codes requires clinical and epidemiological expertise and knowledge about data quality and provenance in addition to knowledge about the databases being interrogated.Citation6 However, there is often significant variation in the clinical codes used to define respiratory conditions.Citation7,Citation8 This can result in considerable differences in study findings,Citation9 such as incidence and prevalence across studies and limits the generalisability and comparability of findings.Citation10,Citation11 As an example, Mukherjee et al examined UK asthma prevalence and reported that annual prevalence of clinician-reported-and-diagnosed asthma was 5.7% (3.6 M individuals) when derived from primary care databases and 6.8% (4.3 M individuals) when derived from the financial incentive-based Quality and Outcomes Framework in UK primary care, whereas annual prevalence of patient-reported clinician-diagnosed-and-treated asthma was 9.6% (6.0 M individuals) derived from national health surveys.Citation12 Therefore, it is important that standardised codelists exist to support research reproducibility, translation of findings between institutions and reduce duplication of work.Citation13
Previously published codelists for respiratory diseases have included specific codes relating to study-specific research questions, and few validation studies have been published.Citation14,Citation15 We sought to respond to this knowledge gap by developing a systematically derived collection of published codes from EHRs for three common respiratory disease categories: asthma, chronic obstructive pulmonary diseaseCitation1 and selected respiratory infections (Box S1). We aimed to amalgamate all codelists into one document and from that produce a recommended list for each disease, which can be used by researchers to identify relevant respiratory-related codes. We describe our methodology for this work in detail to allow researchers to replicate this methodology as appropriate to ensure transparency and reproducibility of codes using EHRs.Citation16
Methods
We systematically searched the literature and existing code repositories to identify all codes relating to asthma, COPD, and respiratory infections. We used a similar approach to previously published respiratory-related validation studies and built on this work.Citation7,Citation14–Citation17 Reviewers were split into three groups to search for codes related to asthma, COPD, and respiratory infections, respectively. Each group comprised at least three epidemiological researchers and one clinician researcher to evaluate disease codes.
Search for Codes Published in the Literature
The Medline database was used because of its comprehensive coverage of clinical medicine research. Searches were performed using key search terms for asthma, COPD, and respiratory infections separately () and abstracts and full text articles were screened by at least two researchers for each disease. We included full-text studies that reported codelists for asthma, COPD, and respiratory infections which were published in January 2020 (), published in English language. These dates were chosen in order to identify up-to-date codes that could be added to existing systematic reviews and codelists already available in the Health Data Research UK (HDR UK) Phenotype Library.Citation18 Our objective was to identify which codelists are being used in research, rather than comment on validity; therefore, the risk of bias analysis was not performed. Supplementary material from the included studies was also reviewed to ensure all codes were identified. Specific codelists of interest included: Read version 2 codes, Clinical Terms version 3 (CTV3), SNOMED CT codes or Clinical Practice Research Datalink (CPRD) medcodeid codes, International Classification of Primary Care (ICPC) codes, International Classification of Diseases (ICD) 9, ICD 10, and ICD 11, and UK Biobank self-diagnosis codes.
Table 1 Search Terms Used to Identify EHR-Related Articles on Asthma, COPD and Respiratory Infections
Figure 1 Study selection, PRISMA diagram.
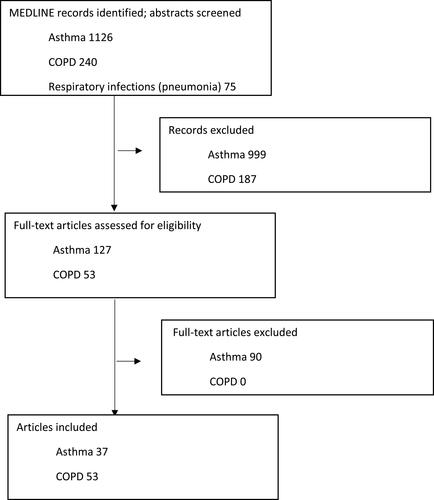
For asthma, studies that were published from 1 January 2018 were also included to capture work published since the last primary care validation study.Citation6 For COPD and respiratory infections, we searched full-texts from the 1st January 2020. We excluded codes related to SARS-CoV-2 infections because of the rapidly evolving evidence base in this area. There were no restrictions on study design, or age of populations studied. Studies that met the search criteria were screened by at least two researchers using a reference manager (Covidence, Zotero & Excel) for the three diseases separately and codelists that were published were compiled into a Microsoft Excel spreadsheet.
Search for Codes Published in Code Repositories
In addition to a literature search, we searched existing UK code repositories: CALIBER HDR UK Phenomics portal, the Cambridge University repository, LSHTM Data Compass, QReseach, Oxford-RCGP RSC, Manchester Clinical Codes, and OpenSAFELY. Relevant code lists for asthma, COPD, and respiratory infections were added to the list of codes identified from the literature search.
Once all codes were complied, recommended lists of codes for asthma, COPD, and respiratory infections were created based on validation studies, previous publications and multidisciplinary clinical expertise and consensus. The recommended lists of codes were labelled “BREATHE recommended codes” for diagnosis codes and were further categorised into phenotypes as appropriate. Phenotype lists were created by two non-clinical authors, and a second review was performed by a clinical author. Asthma-related phenotypes included incident and prevalent asthma and exacerbation of asthma. COPD-related phenotypes included emphysema, chronic bronchitis, incident COPD, prevalent COPD, and exacerbations of COPD. Finally, respiratory infections included 20 different types of infection (supplement).
Results
For asthma, 1126 articles were identified, of which 37 (3%) included published asthma codes and were therefore included. The COPD search retrieved 240 articles of which 53 (22%) included published COPD codes. Respiratory infection pneumonia search retrieved 75 articles for pneumonia where four (5%) of the identified articles included published codes, 15 articles for aspergillosis where four (5%) of identified pneumonia articles included published codes, one (1%) of acute bronchitis articles included published codes, and no articles on aspergillosis included published codes.
In terms of codes identified in repositories, 12 codelists were identified for asthma from Cambridge University,Citation19 Keele University,Citation20 LSHTM Data Compass,Citation21 Manchester clinical codes,Citation22 NHS England,Citation23 OpenSAFELY,Citation24 and UK Biobank.Citation25 Twenty-three codelists were identified for COPD from the Cambridge University repository, LSHTM Data Compass, Manchester Clinical Codes, and OpenSAFELY. In total, 64 codelists were identified for respiratory infections (pneumonia: 21, acute bronchitis: 12, aspergillosis: 0) from the Manchester Clinical Codes, CALIBER HDR UK Phenomics portal, OpenSAFELY, LSHTM Data Compass and Oxford-RCGP RSC repositories).
illustrates the total number of codes published in the included articles for each disease. Most diagnosis codes for asthma were Read v2, whereas the majority of diagnosis codes for all other diseases were SNOMED CT codes for the corresponding CPRD medcodeid codes. For all diseases, very few Biobank and ICPC codes were found.
Figure 2 Number of codes according to code set terms published in included articles for asthma, COPD, and respiratory infections.
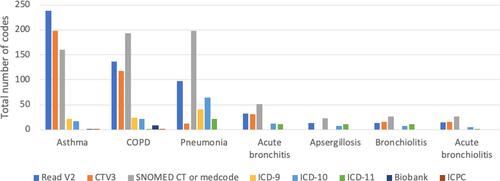
All codelists for asthma, COPD, and respiratory infections can be found on the HDR UK Phenomics portal: https://phenotypes.healthdatagateway.org/about/breathe/#collections.
Discussion
We undertook a systematic literature search of articles that published codelists along with their manuscript and searched codelist repositories to create a comprehensive list of codes used in previous studies related to specific respiratory diseases. From this, we derived a recommended list for each disease for future use either to use as they are or as a starting point for derivation of a list for future work.
Relatively few published papers include, or reference published codelists. The majority of included studies used asthma codelists, which are likely to relate to worldwide asthma prevalence being more than twice that of COPD.Citation26 Of all codelists identified in the literature and repositories, the range of codes used to define specific disorders varied and it was common for research groups to reuse their own codes in each study. Codelists for a variety of databases are continuously updated and made available to the wider scientific community to allow up-to-date and transparent epidemiological research. Codes are added and removed from specific databases (such as SNOMED CT codes) over time and researchers should be aware of this and update their codelists as needed. Other nuances of these codes are that SNOMED CT codes and Read V2 codes do not always directly map across and independent code searches for each type of code should be conducted separately in the database being used for a study in order to identify all possible codes. Researchers also need to be aware that local SNOMED CT codes and Read V2 codes exist, so not all code browsers may include all possible codes. Overall, our work highlights the importance of systematic search strategies and clinical input to identify codes relevant to specific diseases.
Limitations and Use of Codes for Research
Not only do authors often not publish codelists with their work but there are also few validation studies of codelists. To date, our team has created inclusive codelists for COPD, asthma, and respiratory infections that can be used to find these respiratory diseases within UK datasets of routinely collected electronic health records, including sources such as the CPRD GOLD and Aurum, Hospital Episode Statistics, and the SAIL Databank. These codes are relatively broad and cover a range of phenotypes as well as incident and prevalent disease codes.
Researchers must be cautious when using these codes to identify specific populations of individuals with these diseases and the choice of codes will depend on researcher’s specific research question. For example, researchers should only consider incident codes when identifying an incident disease population or if the study group wish to identify, for example, emphysema, a specific emphysema codelist should be used rather than a more general COPD codelist. Furthermore, EHRs are primarily maintained to support health care rather than for research and specific codes may be preferred by clinicians, some codes may not be coded correctly, and some may not be used at all.Citation27 A study examining the usage of disease codes in primary care found in two million consultations performed over a seven-year period, 50% of EHRs were populated with only eight codes out of the 352 (2.3%) possible codes, and in 95% of cases only 36 codes out of 352 (10.2%) were used. Twenty-one percent of all possible allergy codes were never used.Citation28 This highlights the challenges of using EHR data and the importance of creating robust codelists to identify all possible events. The choice of codes for the same clinical condition may also need to be reviewed locally and tailored to the population or dataset as coding practices and data quality may differ between UK regions. In addition, these codes may have limited utility outside the UK and knowledge of local healthcare systems is essential to appreciate why certain codes are used and when.
In addition to the use of specific codes for case definition, other important parameters should be considered depending on the clinical condition and database. This is because specific codes for various conditions may be underused by health care providers and captured by other variables in the database such as prescriptions, tests (such as spirometry to diagnose COPD) and symptoms. One example is the Quality and Outcomes Framework (QOF) indicator for asthma, AST001, (currently suspended in 2021) which uses a 12-month lookback period for prescriptions (in addition to every diagnosis) to identify individuals with active/treated asthma. Similarly, when identifying patients with exacerbations of COPD, other parameters such as prescriptions for respiratory-related oral corticosteroids and antibiotics and symptoms should be used in addition to exacerbation and lower respiratory tract infection codes to identify all possible events and patients.
Transparency of Codes Used for Research
Transparency in research is vital and increasingly expected by funding organisations. Whilst initiatives such as RECORD have helped to increase transparency in reporting of studies undertaken using observational routinely collected data and have led to an extension of STROBE for this purpose, journals do not often mandate that codes or methodologies used for deriving codes for use in routine sources of data are published. It is important for studies to disclose the codelists used to allow the methods to be fully understood, findings interpreted clearly and for analysis to be replicated. One way in which to do this is to include or reference exact lists of codes in published manuscripts rather than only including vague code ranges. We aim to build on this work and create codelists for specific phenotypes (as well as incident and prevalent codes) for asthma, COPD, and respiratory infections with input from respiratory clinicians. These codes could be used to identify sub-populations of patients with respiratory diseases (such as severe asthma) or specific disease-related events (such as an exacerbation of COPD).
Conclusions
We have compiled codelists with the intention of helping researchers find the most up-to-date codes relevant to their study, which will ultimately help comparative respiratory research. Our standardised codelists for respiratory diseases address these issues by creating comprehensive lists that can be used to research respiratory disease, leading to new and clinically important research insights to improve respiratory health. Since lists of codes vary by research questions, these lists of codes might need to be tailored to the exact research question being addressed and can be seen as a starting point for defining respiratory diseases in EHRs. More transparency in reporting is needed, as are validation studies for phenotypes that have not yet been validated, given these data are only going to be continued to be used more frequently and by more people.
Author Contributions
JKQ conceptualised the study and all authors contributed to study design, searching the literature and collating and deriving recommended codelists. CM, HW and MM drafted the original manuscript, with critical revision of the manuscript by all authors. All authors approved the final manuscript. All authors made a significant contribution to the work reported, whether that is in the conception, study design, execution, acquisition of data, analysis and interpretation, or in all these areas; took part in drafting, revising or critically reviewing the article; gave final approval of the version to be published; have agreed on the journal to which the article has been submitted; and agree to be accountable for all aspects of the work. The corresponding author attests that all listed authors meet authorship criteria and that no others meeting the criteria have been omitted. The corresponding author is also the guarantor for this manuscript and accepts full responsibility for the work, had access to all the data and was responsible for the decision to publish.
Acknowledgments
SAIL team for creating the website pages.
Disclosure
CM, HW, MM, LD, AM, CI, MA, EV, EOR, ATW, PWS have nothing to declare. JKQ reports grants from AUK-BLF, The Health Foundation, MRC, grants and personal fees from AZ, BI, GSK, Bayer, grants from Chiesi, outside the submitted work. AS reports grants from AUK-BLF and HDR UK.
Additional information
Funding
References
- Esteban C, Quintana JM, Garcia-Gutierrez S, et al. Determinants of change in physical activity during moderate-to-severe COPD exacerbation. Int J COPD. 2016;11:251–261. doi:10.2147/COPD.S79580
- Anandan C, Simpson CR, Fischbacher C, Sheikh A. Exploiting the potential of routine data to better understand the disease burden posed by allergic disorders. Clin Exp Allergy. 2006;36(7):866–871. doi:10.1111/j.1365-2222.2006.02520.x
- Goldstein BA. Five analytic challenges in working with electronic health records data to support clinical trials with some solutions. Clin Trials. 2020;17(4):370–376. doi:10.1177/1740774520931211
- Whittaker H, Quint JK. Using routine health data for research: the devil is in the detail. Thorax. 2020;75(9):714–715. doi:10.1136/thoraxjnl-2020-214821
- Introduction to codelists; OpenSAFELY documentation. Available from: https://docs.opensafely.org/codelist-intro/. Accessed January 14, 2022.
- O’Malley KJ, Cook KF, Price MD, Wildes KR, Hurdle JF, Ashton CM. Measuring diagnoses: ICD code accuracy. Health Serv Res. 2005;40(5p2):1620–1639. doi:10.1111/j.1475-6773.2005.00444.x
- Quint JK, Müllerova H, DiSantostefano RL, et al. Validation of chronic obstructive pulmonary disease recording in the clinical practice research datalink (CPRD-GOLD). BMJ open. 2014;4(7):e005540–e. doi:10.1136/bmjopen-2014-005540
- Simpson CR, Anandan C, Fischbacher C, Lefevre K, Sheikh A. Will systematized nomenclature of medicine-clinical terms improve our understanding of the disease burden posed by allergic disorders? Clin Exp Allergy. 2007;37(11):1586–1593. doi:10.1111/j.1365-2222.2007.02830.x
- Tate AR, Dungey S, Glew S, Beloff N, Williams R, Williams T. Quality of recording of diabetes in the UK: how does the GP’s method of coding clinical data affect incidence estimates? Cross-sectional study using the CPRD database. BMJ open. 2017;7(1):e012905–e. doi:10.1136/bmjopen-2016-012905
- Nissen F, Quint JK, Morales DR, Douglas IJ. How to validate a diagnosis recorded in electronic health records. Breathe. 2019;15(1):64–68. doi:10.1183/20734735.0344-2018
- Scott P, Dunscombe R, Evans D, Mukherjee M, Wyatt J. Learning health systems need to bridge the ‘two cultures’ of clinical informatics and data science. J Innov Health Inform. 2018;25(2):126–131. doi:10.14236/jhi.v25i2.1062
- Mukherjee M, Stoddart A, Gupta R, et al. The epidemiology, healthcare and societal burden and costs of asthma in the UK and its member nations: analyses of standalone and linked national databases. BMC Med. 2016;14. doi:10.1186/s12916-016-0657-8
- Al Sallakh MA, Vasileiou E, Rodgers SE, Lyons RA, Sheikh A, Davies GA. Defining asthma and assessing asthma outcomes using electronic health record data: a systematic scoping review. Eur Respir J. 2017;49(6):1700204.
- Nissen F, Quint JK, Wilkinson S, Mullerova H, Smeeth L, Douglas IJ. Validation of asthma recording in electronic health records: a systematic review. Clin Epidemiol. 2017;9:643–656. doi:10.2147/CLEP.S143718
- Rothnie KJ, Müllerová H, Hurst JR, et al. Validation of the recording of acute exacerbations of COPD in UK Primary Care Electronic Healthcare Records. PLoS One. 2016;11(3):e0151357–e. doi:10.1371/journal.pone.0151357
- Jayatunga W, Stone P, Aldridge RW, Quint JK, George J. Code sets for respiratory symptoms in electronic health records research: a systematic review protocol. BMJ open. 2019;9(3):e025965–e. doi:10.1136/bmjopen-2018-025965
- Pikoula M, Quint JK, Nissen F, Hemingway H, Smeeth L, Denaxas S. Identifying clinically important COPD sub-types using data-driven approaches in primary care population based electronic health records. BMC Med Inform Decis Mak. 2019;19(1). doi:10.1186/s12911-019-0805-0
- Health Data Research UK (HDRUK) Phenotype Library. Available from: https://phenotypes.healthdatagateway.org/phenotypes/. Accessed December 01, 2021.
- University of Cambridge Research Methods Hub, Code lists. Available from: https://www.phpc.cam.ac.uk/pcu/research/research-groups/crmh/cprd_cam/codelists/. Accessed December 01, 2021.
- University of Keele Medical Record Data Research, Code Lists. Available from: https://www.keele.ac.uk/mrr/codelists/. Accessed December 01, 2021.
- London School of Hygiene and Tropical Medicine (LSHTM) data compass. Available from: https://datacompass.lshtm.ac.uk/. Accessed December 01, 2021.
- University of Manchester, Clinical Codes. Available at https://clinicalcodes.rss.mhs.man.ac.uk/. Accessed December 01, 2021.
- NHS Digital Clinical Classifications. Available from: https://digital.nhs.uk/services/terminology-and-classifications/clinical-classifications. Accessed December 01, 2021.
- OpenSAFELY Code. Available from: https://www.opensafely.org/code/. Accessed December 01, 2021.
- UK Biobank Primary Care Linked Data. Available from: https://biobank.ndph.ox.ac.uk/showcase/showcase/docs/primary_care_data.pdf. Accessed December 01, 2021.
- Soriano JB, Kendrick PJ, Paulson K, et al. Prevalence and attributable health burden of chronic respiratory diseases, 1990–2017: a systematic analysis for the Global Burden of Disease Study 2017. Lancet Respir Med. 2020;8(6):585–596.
- Jordan K, Porcheret M, Croft P. Quality of morbidity coding in general practice computerized medical records: a systematic review. Fam Pract. 2004;21(4):396–412. doi:10.1093/fampra/cmh409
- Mukherjee M, Wyatt JC, Simpson CR, Sheikh A. Usage of allergy codes in primary care electronic health records: a national evaluation in Scotland. Allergy. 2016;71(11):1594–1602. doi:10.1111/all.12928