Abstract
Purpose
To evaluate, in a proof-of-concept study, a decision aid that incorporates hypothetical choices in the form of a discrete-choice experiment (DCE), to help patients with early rheumatoid arthritis (RA) understand their values and nudge them towards a value-centric decision between methotrexate and triple therapy (a combination of methotrexate, sulphasalazine and hydroxychloroquine).
Patients and Methods
In the decision aid, patients completed a series of 6 DCE choice tasks. Based on the patient’s pattern of responses, we calculated his/her probability of choosing each treatment, using data from a prior DCE. Following pilot testing, we conducted a cross-sectional study to determine the agreement between the predicted and final stated preference, as a measure of value concordance. Secondary outcomes including time to completion and usability were also evaluated.
Results
Pilot testing was completed with 10 patients and adjustments were made. We then recruited 29 patients to complete the survey: median age 57, 55% female. The patients were all taking treatment and had well-controlled disease. The predicted treatment agreed with the final treatment chosen by the patient 21/29 times (72%), similar to the expected agreement from the mean of the predicted probabilities (68%). Triple therapy was the predicted treatment 24/29 times (83%) and chosen 20/29 (69%) times. Half of the patients (51%) agreed that completing the choice questions helped them to understand their preferences (38% neutral, 10% disagreed). The tool took an average of 15 minutes to complete, and median usability scores were 55 (system usability scale) indicating “OK” usability.
Conclusion
Using a DCE as a value-clarification task within a decision aid is feasible, with promising potential to help nudge patients towards a value-centric decision. Usability testing suggests further modifications are needed prior to implementation, perhaps by having the DCE exercises as an “add-on” to a simpler decision aid.
Background
In early rheumatoid arthritis (RA), one preference-sensitive decision is the choice between triple therapy, a combination of 3 medications (methotrexate, sulphasalazine and hydroxychloroquine), versus methotrexate alone as initial treatment. There is moderate quality evidence from randomized trials that triple therapy is superior to methotrexate alone for disease control in the short term,Citation1–Citation3 but it involves more pills that may be overly burdensome for patients. In an elegant illustration of the preference-sensitive nature of this decision, Fraenkel et al asked a trained patient panel to vote on a recommendation after reviewing the same evidence summary used in the American College of Rheumatology (ACR) Guidelines.Citation4 The patient panel recommended triple therapy, opposite from the physician-dominated panel and official ACR Guidelines, which recommended methotrexate.Citation1,Citation4 The difference was in how physicians and patients viewed the trade-offs. Both the patient and physician panel agreed that this was a “conditional”, preference-sensitive recommendation.Citation4
Findings from a previous study we conducted support the judgements of the ACR patient panel regarding triple therapy.Citation5 We first measured the preferences of patients with early RA for the risks-benefit trade-offs of alternative treatments using a discrete-choice experiment (DCE),Citation6 a quantitative stated preference method that asks patients to make choices between hypothetical treatment options. We then used these results to apply value weights to outcomes from a network meta-analysisCitation2,Citation3 and other considerations, including dosing and rare risks. In doing so, we estimated most patients (78%) would prefer triple therapy.Citation5 We also found important variability in preferences, with a subgroup of patients being more risk averse, again highlighting the need to tailor the choice of treatment to patients’ preferences, and supporting the need for shared decision-making.
Patient decision aids are now well established as effective tools to facilitate shared decision-making, improve knowledge about treatment options and reduce decisional conflict across a range of medical decisions.Citation7 Decisional conflict reflects the uncertainty patients feel when making a decision, and can be measured using various validated tools, including the decisional conflict scale,Citation8 or the simplified 4-question SURE (Sure of myself; Understand information; Risk-benefit ratio; Encouragement) scale.Citation9 Multiple prior decision aids for rheumatoid arthritis have been developed, and have shown improvements in decisional conflict and other measures of decision quality.Citation7,Citation10,Citation11 Most of the prior decision aids have focused on a decision between various biologic therapies, or between no treatment versus a single DMARD. We are not aware of other decision aids developed for the choice between methotrexate and triple therapy as initial treatment.
One key element of high-quality decision aids is that they promote choices of options that are congruent with a patients’ informed values.Citation12 Consequently, value-clarification exercises are often included to help patients clarify and communicate their personal values.Citation13 These value clarification methods vary widely, and there is no consensus as to how best to structure these tasks.Citation14 Simply completing value clarification tasks may not be sufficient, as patients may not be able to map how their values match to the options presented. Interestingly, in a 2016 systematic review of methods for value clarification, explicitly showing people the implications of their stated values was one of the most promising design features for improving readiness and decision quality.Citation15 By presenting patients with the implications of their values in a user-friendly way, it may serve as a “nudge” to help overcome decision-making biases that can occur.Citation16
Discrete-choice experiments are an appealing option for a value clarification task, as they provide a quantitative method of estimating the relative importance of each treatment attribute (eg risks, benefits, dosing), which can then be related back to the actual treatment choices. A challenge, however, is implementing this within a decision-aid, as real-time data analysis is often not feasible, and DCEs often require multiple choice tasks (eg 10–15) per patient. The objective of this study was to evaluate the use of a DCE in value clarification tasks as a “nudge” to assist patients in making a value-centric decision for the choice between methotrexate and triple therapy as initial treatment. We incorporated a novel matching algorithm approach, whereby we used data from our prior DCECitation6 to estimate each patient’s preferred treatment, based on their responses to the DCE choices within the decision aid. The study was designed as an initial proof-of-concept study to see if the DCE could be useful in a predictive fashion, with a view towards future formal testing.
Methods
Overview of Decision-Aid Platform
To develop our tool, we used a previously developed decision-aid platform, the Dynamic Computer Interactive Decision Application (DCIDA).Citation17 DCIDA follows principles of high-quality decision aids,Citation18 including discussion of the treatment decision and options, value clarification tasks, and next steps to prepare for decision-making. The usability of the DCIDA platform has been previously demonstrated in 20 RA patients through in-person think-aloud interviews combined with eye-tracking.Citation17
Similar to the original DCIDA platform,Citation17 our decision aid began with a description of the disease (RA) and treatment options. Patients then completed demographic questions (age, gender, disease duration) and patient-reported outcomes for the purposes of this study: the health-assessment questionnaire disability index (HAQ-DI), a validated measure of functional limitation with scores ranging from 0 (no functional limitation) to 3 (severe disability),Citation19 and the patient global assessment of disease activity, a validated measure of rheumatoid arthritis disease activity ranging from 0 (disease inactive) to 10 (disease very active). Next, patients completed the value clarification exercises, which were modified from the original DCIDA platform to include DCE exercises, as described in detail below. Finally, we collected the SURE (Sure of myself; Understand information; Risk-benefit ratio; Encouragement) scale, a validated measure of decisional conflict,Citation9 the System Usability Scale (SUS), a validated scale that measures user friendliness of software,Citation20 and a Likert scale question asking patients whether completing the choice tasks helped the patient understands his or her preferences. Scores on the system usability scale range from 0 to 100, with mean scores of 52 reflecting “OK” usability, 73 for “Good” usability and 86 for “Excellent” usability.Citation20
Value Clarification Tasks
We used our prior discrete-choice experiment (DCE) as the basis for the value clarification tasks.Citation6 In the original DCE, patients were asked to choose 1 of 3 hypothetical treatment options that varied across 8 different attributes. The attributes included treatment benefits, harms, and dosing options relevant to a choice between common early RA treatment options including methotrexate, triple therapy and anti-TNF therapy. For the purposes of this study, the DCE included the same attributes and levels, but patients only completed 6 tasks where they chose between 2 hypothetical treatments (). As with our published DCE, the choice tasks for the decision tool were generated using a “balanced overlap” design, using Sawtooth Software (Orem, USA). All patients completed the same set of choice tasks, which was one of the 100 generated designs, chosen at random.
Estimation of Patients’ Preferred Treatment
To display a patient’s preferred treatment, we used a matching algorithm. For each of the 64 possible response profiles (eg AAAAA, AAAAAB, … BBBBBB) to the choice tasks [6 tasks with 2 choices each = 64 (26) possible response profiles], we calculated the corresponding probability of preferring triple therapy versus methotrexate. This calculation was conducted in two stages, and utilized data from our prior DCE, which had the same inclusion criteria as this current study (RA diagnosis less than 2 years).Citation6
In the first stage, we estimated how each patient from our prior study (n=152) would be expected to respond to the 6 modified choice tasks. This was done using “scenario analyses”, which are common with DCEs. For each of the 6 choice tasks, we assumed patients would prefer the treatment with the highest overall “value”, which was calculated by summing up the part-worth utilities of the attributes that defined it. These part-worth utilities reflect the relative importance of each attribute level, and were previously calculated using a hierarchical Bayesian model.Citation5 Thus, for each individual patient in our prior study (n=152) it was possible to re-estimate their expected pattern of responses to the modified choice tasks for this current study. By conducting these analyses multiple times (eg 10,000) using values that were sampled from their distributions, we were able to estimate the probability each patient would choose each of the 64 possible response profiles.
In the second stage, we mapped the expected pattern of responses for the 6 choice tasks to the probability that the patient would choose triple therapy versus methotrexate, which had been previously calculated.Citation5 Again, these calculations were based on scenario analyses, with the attributes of triple therapy and methotrexate defined by the outcomes from a network meta-analysisCitation2,Citation3 and other considerations (ie, dosing, rare risks). We then calculated the weighted average of the probability of choosing triple therapy for each response profile (see Appendix A for an example calculation). This allowed us to develop a matching algorithm, where for each of the 64 possible response patterns to the 6 choice tasks, there was a corresponding probability of choosing triple therapy. Once patients completed their choice tasks, the corresponding probability of choosing triple therapy that matched their response pattern was presented back to them.
The Nudge
We explored different approaches for how best to display the information in order to nudge patients to choose options that best met their preferences. Ultimately, we chose to display the information as a pre-selected default option (where the preferred option would be selected), alongside the strength of preference as a probability (). Patients were then asked whether they agree with the selected option and were asked to make a final choice of treatment.
Pilot Testing
The pilot testing of our decision aid was modeled on the prior development of the DCIDA platform.Citation17 First, we conducted a series of 1-on-1 sessions with patients with early RA (<2 years since diagnosis) recruited from rheumatology clinics in Calgary, Alberta. A research assistant obtained signed informed consent for the pilot testing and a review of their medical charts. The initial portion of the session was unstructured; participants were encouraged to “think aloud” as they worked through the decision aid in a simulated physician–patient interaction. Following the think-aloud portion, participants were asked if they had any specific questions to clarify issues that may have been identified during prior sessions (eg, wording of questions, comprehension difficulty, page layout, page navigation, preference for different formats). Participants were also asked their overall thoughts on using the tool and whether they would be likely to find the tool useful.
The research coordinator took field notes during each session and all interviews were audio-recorded and transcribed verbatim. Similar to our prior work,Citation17 the field notes and content of the interviews were analyzed after each session by the same staff member to identify usability issues, which were classified into critical (prevents further use) or general. General issues became critical if they occurred with 2 different users. When a critical issue was identified, further interviewing was interrupted, and the tool was modified prior to resuming. The sessions continued until no new usability issues were identified (saturation of responses).
Proof-of-Concept Study
Once we were satisfied regarding the usability of the tool, a proof-of-concept study was conducted in patients with early RA (<2 years since diagnosis). Patients who had been consented to be contacted for future studies were recruited from Rheum4U, an ongoing prospective registry of RA patients in Calgary, Alberta.Citation21 Eligible patients were sent an e-mail invitation to participate in the study, along with the informed consent document explaining the study. Implied consent was obtained through completion of the survey. In addition to data collected for the study, we obtained consent to link participant’s responses to medication use data collected in the registry. Participants who did not respond were sent up to 2 reminders at 1 and 2 weeks.
Analysis
Characteristics of patients in both the pilot testing and proof-of-concept study were summarized using descriptive statistics. For the proof-of-concept testing, our primary outcome of interest was the percent agreement between the patient’s final treatment choice versus the predicted choice. This was used as an estimate of value concordance, one of the key constructs of decision quality.Citation12 As our predictions were probabilistic, we did not expect perfect agreement. We, therefore, compared the observed percent agreement to the expected percent agreement, which was calculated as the mean value of the predicted probabilities across all patients.
Results for the other outcomes were summarized descriptively. Scores for the SURE scale were calculated as a simple sum of the “yes” responses across the 4 questions and summarized as the proportion of patients with each score. The interpretation of these ranges from 0 (extremely high decisional conflict) to 4 (no decisional conflict).Citation9 Scores for the SUS were calculated using scoring guidelines for the tool.Citation20 The scores range from 0 to 100, with scores >52 indicating “OK” usability and scores >72 indicating “Good” usability.Citation20 Products should typically aim for usability scores >70, and scores <50 are considered unacceptable.Citation20
Finally, we evaluated the association between participant characteristics and their stated preference and other secondary outcomes through logistic regression, with a P-value of <0.05 indicating statistical significance. The secondary outcomes were dichotomized for these analyses: SURE score equal to 4 (no decisional conflict) versus less than 4; SUS equal to or above the median value versus below; Strongly agree/agree versus strongly disagree/disagree/neutral for the Likert question “completing the choices helped me understand my preferences”.
Ethics Approval
This study was conducted according to the principles outlined in the Declaration of Helsinki with informed consent obtained from all patients. The study was approved by the University of Calgary Conjoint Health Research Ethics Board (#REB15-1665)
Results
Pilot Testing
The pilot testing was completed with 10 patients (6 male, median age 54). Patients found completing 6 tasks to be feasible number. Early participants reported task comprehension issues, included difficulty understanding what they were required to do on each page, and the hypothetical nature of the choice tasks. These were addressed by modifying the text to include more clear instructions, and further recruitment for testing was then completed. Participants also found it easier to complete the choice tasks first (), prior to viewing the page outlining the attributes associated with the actual treatment options (). Other usability issues included issues with scrolling, font size, and navigating between the pages. These were corrected in an iterative fashion to finalize the decision aid. Most of the participants were satisfied with their overall experience of using the tool, and some communicated an interest in using something similar again. They liked the ability to personalize their responses and receive an individualized summary. Others remained unclear about its potential benefits.
Proof-of-Concept Study
E-mail invitations were sent to 73 participants in the Rheum4U registry who met eligibility criteria for the study and had agreed to be contacted for future research. Of these, 33 started the survey and 29 completed (40% response rate). Characteristics of the participants who completed the survey are summarized in . The participants had a median age of 57 and 55% were female, similar to the 44 participants who did not complete the survey (median age 52, 64% female). The disease duration of the patients who completed the survey was short at a median of 1.2 years (). Patient global scores were low (median 2.0) and HAQ-DI scores indicated low levels of functional disability (median 0.2). Most patients had taken or were taking methotrexate (93%) and hydroxychloroquine (82%), with fewer having taken sulphasalazine (36%).
Table 1 Participant Characteristics
Table 2 Comparison of Predicted versus Stated Preferences
DCE Choices and Treatment Predictions
All 29 patients completed all of the 6 choice tasks. The responses to the choice tasks clustered on 19 of the 64 unique profiles, with one particular profile being chosen by 4 participants (). The comparison of patients’ predicted versus stated treatment choices is summarized in . Overall, the predicted treatment agreed with the stated choice for 21/29 patients (72%). This was similar to the expected agreement of 68%, calculated as the mean of the prediction probabilities that were displayed to patients. Triple therapy was the predicted treatment 24/29 times (83%) and chosen 20/29 (69%) times (). Prior or current use of triple therapy was not associated with patients’ stated preference for triple therapy (logistic regression P-value = 0.12).
Figure 3 Comparison of predicted versus stated preferences across all possible response profiles. The predicted probabilities are those displayed to the patient in the decision tool for each of the 64 possible response profiles. Probabilities above the 50% hashed line are anticipated to prefer triple therapy, while those below the hashed line prefer methotrexate. The actual response profile chosen by each patient is shown at the bottom of the figure, along with the agreement (yes/no) with the prediction.
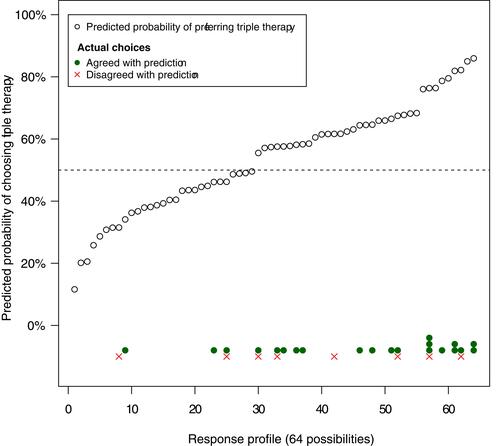
Secondary Outcomes
Scores on the SURE scale indicated no decisional conflict for the majority of patients (62%) (). The median score on the system usability scale was 55 (25th, 75th percentile: 16, 91), indicating “ok” to “good” usability.Citation20 About half of the patients (51%) agreed or strongly agreed that completing the choice questions helped them understand their preferences, 11 (38%) were neutral and 3 (10%) disagreed or strongly disagreed. The patients who were neutral or disagreed/strongly disagreed to this question (N=14) had statistically significant lower usability ratings than patients who agreed or strongly agreed [median (25th, 75th percentiles) SUS scores: 50 (36, 54) versus 70 (58, 83), logistic regression P-value = 0.01]. These 14 patients were also statistically more likely to be older [median (25th, 75th percentiles) age: 61 (55, 66) years versus 55 (37, 60) years, logistic regression P-value = 0.048]. There were no other statistically significant associations between patient characteristics and any of the secondary outcomes (data not shown).
Table 3 Post-Tool Decisional Conflict Ratings
On average, the tool took 15 minutes (median) to complete (25th, 75th percentile: 7, 73), excluding 4 patients who had completion times between 22 hours and 9 days, who had clearly left the survey page and returned to it at another sitting. Amongst these 4 patients, who were still included in the overall analyses, 2 had chosen triple therapy as their final treatment preference, and 2 had chosen methotrexate.
Discussion
We have developed an online decision aid that embeds a DCE as a value-clarification task to help nudge patients towards a value-centric choice. The tool utilizes a database of existing patient preferences to “map” a given individual to a preference profile, and then predicts which option would likely match their profile. Importantly, since this prediction may not capture the intricacies of an individual’s preference profile, it provides only a nudge to what option might be best, and does not preclude an individual choosing a different option.Citation16 In our proof-of-concept study, we found that the patients chose their predicted treatment 72% of the time, similar to the expected agreement. Furthermore, many patients felt the tool helped to understand their preferences. The tool took on average 15 minutes to complete, similar to other decision tools, suggesting it could be feasible to incorporate into a clinical encounter. Together, these results suggest that the tool may be a useful approach for some patients and merits further evaluation.
While explicit values clarification methods as a whole have been shown to encourage value-congruent decision-making,Citation7,Citation22 many different approaches have been used, with few evaluated on whether or not they encourage value congruent decisions.Citation14,Citation15 The approaches used have tended to either require patients to answer numerous, cognitively challenging questions in order to develop accurate, individual-level predictions, making them unfeasible for use in the real world, or have used too few questions to enable robust individual level estimates.Citation23–Citation26 Eight prior studies have used conjoint analysis as value clarification methods.Citation27 All eight of these used adaptive conjoint analysis, a variation on a DCE, whereby the choice tasks presented to each patient are altered (adapted) based on the real-time analysis of a participant’s prior responses. A strength of our approach is that it allowed individual-level predictions of patients’ treatment preferences without the need for real-time data analysis, and with the patient only having to answer a few choice tasks. This may provide a more feasible approach for incorporating DCEs into the design of a decision aid. It also simplified the design considerations, as we were not bound to include a given number of choice tasks. Understanding the impact of the number of choice tasks would be important future work. As with any of the other approaches for incorporating conjoint analysis tasks, prior data from a similar population of patients are required to make individual-level predictions, which is a limitation, particularly if the treatment landscape changes.
Another challenge with decision aids is how best to incorporate and display second-order uncertainty (imprecision) regarding risks and benefits. For example, while the chance of remission with triple therapy was estimated at 61% from the NMA, there is some imprecision around this estimate (eg a 95% credible interval).Citation2,Citation3 While this imprecision in estimates should rationally be incorporated into people’s decisions, it is challenging for most people to understand. A previous review found few decision aids describe imprecision in estimates or risk and benefits,Citation28 and those that have studied the impact show they can promote pessimistic appraisals of risks and avoidance of decision-making, a set of responses known as ambiguity aversion.Citation29 This is why we chose to display only the point estimates to patients in the DCE. A strength of our approach is that the modelling exercises we used to derive the treatment predictions factored in the imprecision to the underlying calculations by averaging over multiple samples.
Limitations of our study should be borne in mind. The number of patients in both the pilot and proof-of-concept testing was small, although the DCIDA decision-aid platform our decision tool was built from has been previously user-tested.Citation17 The usability scores in the proof-of-concept testing suggest room for improvement. Not surprisingly, lower usability scores were seen in the patients who felt that the tool did not help them understand their preferences. There are several possible reasons for the low usability scores in some patients. Patients in the proof-of-concept testing were recruited at random from a registered database and completed the tool at home, so additional issues not detected in the pilot testing may have occurred. Additionally, the prior study that we drew the DCE exercises was designed to capture a range of treatments including biologic therapy. As such, several of the attributes in the DCE were not relevant to the choice between triple therapy and methotrexate. Including these additional attributes may have added to the complexity of the tasks and affected the usability. Patients’ preferences for their involvement in shared decision-making also vary. It is unlikely that one decision-aid type or method will be suitable for all patients. We believe that these more in-depth value clarification tasks may be most useful as an “add-on” option within a simpler decision aid for patients with a greater desire for shared decision-making. Further development is planned to evaluate this.
While our study demonstrated the predictive accuracy of the DCE in relation to a patient’s stated preference, further studies would be required to demonstrate that the value-clarification tasks impact the actual choices patients make. This would require a controlled study comparing the choices patients made with and without the decision tool. Having patients complete the tool at the time of decision would also help overcome the hypothetical bias in our study. In our survey, patients were asked to complete the survey as if their disease were active. Patients were also already on treatment, with most taking methotrexate. This may have skewed patients’ responses towards triple therapy, which presented a clear alternative to the status quo. However, the DCE exercises in our decision tool were unlabeled and included multiple different treatment options. Thus, the fact that triple therapy was the predicted treatment preference 20/29 times (69%), based on the patients’ responses to the DCE, suggests that patients were still selecting the treatment with the greatest benefit, regardless of the specific treatments presented.
Our results, while subject to the limitations of a small proof-of-concept study, also have clinical implications when viewed within a larger body of evidence.Citation30 The results of this study agree with those from our prior DCECitation5,Citation6 and the work of Fraenkel et alCitation4 showing many patients may prefer triple therapy over methotrexate monotherapy. In practice, the use of triple therapy varies widely and is largely driven by the physician. In a large multicenter Canadian cohort, the use of triple therapy as initial varied from 1% to 52% between sites.Citation31 Our results suggest that implementing a decision-aid could result in higher rates of triple therapy use, closer to the highest-use site. This has implications for patients, as initial use of triple therapy versus methotrexate monotherapy has been associated with improved outcomes, and for society, as triple therapy has been associated with cost-effectiveness and improved worker productivity.Citation2,Citation3,Citation32 These are potential benefits, beyond those related to improved decision-making (eg decisional conflict), which have been consistently demonstrated with decision aids.Citation7
Conclusion
In summary, as a proof-of-concept, our study used DCE-based choice tasks to relate a patient’s preferences to the actual treatment choices available. We demonstrated feasibility and predictive accuracy of the value clarification exercise – a first step to evaluating the usefulness of this approach. Given that explicitly showing people the implications of their stated values are one of the most promising design features of value-clarification tasks,Citation15 we believe a DCE is a promising approach that merits further evaluation. Specifically, we believe these tasks may be best implemented as an “add-on” within a simpler decision-aid, to appeal to a diverse range of decision-making styles. Uptake of decision aids is also a major barrier, and implementation studies, ideally tied to a broader suite of preference-sensitive decisions would also be important future work.
Author Contributions
All authors made substantial contributions to conception and design, acquisition of data, or analysis and interpretation of data; took part in drafting the article or revising it critically for important intellectual content; gave final approval of the version to be published; and agree to be accountable for all aspects of the work.
Disclosure
GH: Supported by a Canadian Institute for Health Research (CIHR) New Investigator Salary Award.
DM: Received salary support from the Arthur J.E. Child Chair Rheumatology Outcomes Research and a Canada Research Chair, Health Systems and Services Research (2008–18).
LL: Supported by the Canada Research Chair Program, Harold Robinson/Arthritis Society Chair in Arthritic Diseases, and the Michael Smith Foundation for Health Research Scholar Award.
The authors report no other conflicts of interest in this work.
Additional information
Funding
References
- Singh JA, Saag KG, Bridges SL Jr, et al. 2015 American College of Rheumatology guideline for the treatment of rheumatoid arthritis. Arthritis Rheum. 2016;68(1):1–26. doi:10.1002/art.39480
- Hazlewood GS, Barnabe C, Tomlinson G, Marshall D, Devoe DJ, Bombardier C. Methotrexate monotherapy and methotrexate combination therapy with traditional and biologic disease modifying anti-rheumatic drugs for rheumatoid arthritis: a network meta-analysis. Cochrane Database Syst Rev. 2016;8:CD010227.
- Hazlewood GS, Barnabe C, Tomlinson G, Marshall D, Devoe D, Bombardier C. Methotrexate monotherapy and methotrexate combination therapy with traditional and biologic disease modifying antirheumatic drugs for rheumatoid arthritis: abridged Cochrane systematic review and network meta-analysis. BMJ. 2016;353:i1777. doi:10.1136/bmj.i1777
- Fraenkel L, Miller AS, Clayton K, et al. When patients write the guidelines: patient panel recommendations for the treatment of rheumatoid arthritis. Arthritis Care Res (Hoboken). 2016;68(1):26–35. doi:10.1002/acr.22758
- Hazlewood GS, Bombardier C, Tomlinson G, Marshall D. A Bayesian model that jointly considers comparative effectiveness research and patients’ preferences may help inform GRADE recommendations: an application to rheumatoid arthritis treatment recommendations. J Clin Epidemiol. 2018;93:56–65. doi:10.1016/j.jclinepi.2017.10.003
- Hazlewood GS, Bombardier C, Tomlinson G, et al. Treatment preferences of patients with early rheumatoid arthritis: a discrete-choice experiment. Rheumatology (Oxford). 2016;55(11):1959–1968. doi:10.1093/rheumatology/kew280
- Stacey D, Legare F, Lewis K, et al. Decision aids for people facing health treatment or screening decisions. Cochrane Database Syst Rev. 2017;4:CD001431.
- O’Connor AM. Validation of a decisional conflict scale. Med Decis Making. 1995;15(1):25–30. doi:10.1177/0272989X9501500105
- Legare F, Kearing S, Clay K, et al. Are you SURE?: assessing patient decisional conflict with a 4-item screening test. Can Fam Physician. 2010;56(8):e308–314.
- Pablos JL, Jover JA, Roman-Ivorra JA, et al. Patient Decision Aid (PDA) for patients with rheumatoid arthritis reduces decisional conflict and improves readiness for treatment decision making. Patient. 2019; 13(1):57–69.
- Fraenkel L, Matzko CK, Webb DE, et al. Use of decision support for improved knowledge, values clarification, and informed choice in patients with rheumatoid arthritis. Arthritis Care Res (Hoboken). 2015;67(11):1496–1502. doi:10.1002/acr.22659
- Sepucha KR, Borkhoff CM, Lally J, et al. Establishing the effectiveness of patient decision aids: key constructs and measurement instruments. BMC Med Inform Decis Mak. 2013;13(Suppl 2):S12. doi:10.1186/1472-6947-13-S2-S12
- Fagerlin A, Pignone M, Abhyankar P, et al. Clarifying values: an updated review. BMC Med Inform Decis Mak. 2013;13(Suppl 2):S8. doi:10.1186/1472-6947-13-S2-S8
- Witteman HO, Scherer LD, Gavaruzzi T, et al. Design features of explicit values clarification methods: a systematic review. Med Decis Making. 2016;36(4):453–471. doi:10.1177/0272989X15626397
- Witteman HO, Gavaruzzi T, Scherer LD, et al. Effects of design features of explicit values clarification methods: a systematic review. Med Decis Making. 2016;36(6):760–776. doi:10.1177/0272989X16634085
- Blumenthal-Barby JS, Cantor SB, Russell HV, Naik AD, Volk RJ. Decision aids: when ‘nudging’ patients to make a particular choice is more ethical than balanced, nondirective content. Health Aff (Millwood). 2013;32(2):303–310. doi:10.1377/hlthaff.2012.0761
- Bansback N, Li LC, Lynd L, Bryan S. Development and preliminary user testing of the DCIDA (dynamic computer interactive decision application) for ‘nudging’ patients towards high quality decisions. BMC Med Inform Decis Mak. 2014;14(1):62. doi:10.1186/1472-6947-14-62
- Elwyn G, O’Connor A, Stacey D, et al. Developing a quality criteria framework for patient decision aids: online international Delphi consensus process. BMJ. 2006;333(7565):417. doi:10.1136/bmj.38926.629329.AE
- Fries JF, Spitz PW, Young DY. The dimensions of health outcomes: the health assessment questionnaire, disability and pain scales. J Rheumatol. 1982;9(5):789–793.
- Bangor A, Kortum PT, Miller JT. An empirical evaluation of the system usability scale. Int J Hum Comput Interact. 2008;24(6):574–594. doi:10.1080/10447310802205776
- Barber CEH, Sandhu N, Rankin JA, et al. Rheum4U: development and testing of a web-based tool for improving the quality of care for patients with rheumatoid arthritis. Clin Exp Rheumatol. 2018 37(3):385–92.
- Munro S, Stacey D, Lewis KB, Bansback N. Choosing treatment and screening options congruent with values: do decision aids help? Sub-analysis of a systematic review. Patient Educ Couns. 2016;99(4):491–500. doi:10.1016/j.pec.2015.10.026
- Matheis-Kraft C, Roberto KA. Influence of a values discussion on congruence between elderly women and their families on critical health care decisions. J Women Aging. 1997;9(4):5–22. doi:10.1300/J074v09n04_02
- Montgomery AA, Fahey T, Peters TJ. A factorial randomised controlled trial of decision analysis and an information video plus leaflet for newly diagnosed hypertensive patients. Br J Gen Pract. 2003;53(491):446–453.
- O’Connor AM, Wells GA, Tugwell P, Laupacis A, Elmslie T, Drake E. The effects of an ‘explicit’ values clarification exercise in a woman’s decision aid regarding postmenopausal hormone therapy. Health Expect. 1999;2(1):21–32. doi:10.1046/j.1369-6513.1999.00027.x
- Sheridan SL, Griffith JM, Behrend L, Gizlice Z, Cai J, Pignone MP. Effect of adding a values clarification exercise to a decision aid on heart disease prevention: a randomized trial. Med Decis Making. 2010;30(4):E28–39. doi:10.1177/0272989X10369008
- Weernink MGM, van Til JA, Witteman HO, Fraenkel L, IJzerman MJ. Individual value clarification methods based on conjoint analysis: a systematic review of common practice in task design, statistical analysis, and presentation of results. Med Decis Making. 2018;38(6):746–755. doi:10.1177/0272989X18765185
- Bansback N, Bell M, Spooner L, Pompeo A, Han PKJ, Harrison M. Communicating uncertainty in benefits and harms: a review of patient decision support interventions. Patient. 2017;10(3):311–319. doi:10.1007/s40271-016-0210-z
- Ellsberg D. Risk, ambiguity, and the Savage axioms. Q J Econ. 1961;75(4):643–669. doi:10.2307/1884324
- Durand C, Eldoma M, Marshall DA, Hazlewood GS. Patient preferences for disease modifying anti-rheumatic drug treatment in rheumatoid arthritis: a systematic review. J Rheumatol. 2019;47(2):176–87.
- Harris JA, Bykerk VP, Hitchon CA, et al. Determining best practices in early rheumatoid arthritis by comparing differences in treatment at sites in the Canadian Early Arthritis Cohort. J Rheumatol. 2013;40(11):1823–1830. doi:10.3899/jrheum.121316
- de Jong PH, Hazes JM, Buisman LR, et al. Best cost-effectiveness and worker productivity with initial triple DMARD therapy compared with methotrexate monotherapy in early rheumatoid arthritis: cost-utility analysis of the tREACH trial. Rheumatology (Oxford). 2016;55(12):2138–2147. doi:10.1093/rheumatology/kew321