Abstract
Background
Pervasive health misinformation on social media affects people’s health. Fact-checking health information before it is shared is an altruistic behavior that effectively addresses health misinformation on social media.
Purpose
Based on the influence of presumed media influence (IPMI), this study serves two purposes: The first is to investigate factors that influence social media users’ decisions to fact-check health information before sharing it in accordance with the IPMI model. The second is to explore different predictive powers of the IPMI model for individuals with different levels of altruism.
Methods
This study conducted a questionnaire survey of 1045 Chinese adults. Participants were divided into either a low-altruism group (n = 545) or a high-altruism group (n = 500) at the median value of altruism. A multigroup analysis was conducted with R Lavaan package (Version 0.6–15).
Results
All of the hypotheses were supported, which confirms the applicability of the IPMI model in the context of fact-checking health information on social media before sharing. Notably, the IPMI model yielded different results for the low- and high-altruism groups.
Conclusion
This study confirmed the IPMI model can be employed in the context of fact-checking health information. Paying attention to health misinformation can indirectly affect an individual’s intention to fact-check health information before they share it on social media. Furthermore, this study demonstrated the IPMI model’s varying predictive powers for individuals with different altruism levels and recommended specific strategies health-promotion officials can take to encourage others to fact-check health information.
Introduction
With the global digitization and proliferation of the Internet, the general public has increasingly utilized various web portals and websites to seek out and share health information.Citation1 More than 70% of adults search for health-related information.Citation2 While social media has become a significant source of information,Citation3,Citation4 these platforms are often blamed for the spread of misinformation,Citation5,Citation6 which severely affects the dissemination of health-related information,Citation7 because of the voluminous amount of unregulated user-generated content.Citation8,Citation9
Health misinformation is defined as false health-related claims that lack scientific evidence.Citation1 A recent study suggested that misinformation spreads faster and more easily on social media than accurate information.Citation10 Because information people find online has been shown to impact their health-related decision-making,Citation11 including lifestyle changes, treatment compliance, professional treatment delays, and being prescribed the wrong medicine.Citation12–17 Health misinformation on social media has particularly serious consequences for an individual’s quality of life, and can even place their life in jeopardy. Therefore, the prevalence of health misinformation on social media must be addressed. Fact-checking is highlighted as a solution.Citation18
In the age of social media, individuals have become accustomed to sharing information online. However, the content they share is not always accurate. Due to the absence of journalistic gatekeeping functions and deficient algorithms, social media users share information based on their subjective and biased interpretations.Citation19 Information considered a high level of “share worthiness” spreads rampantly on social media like a highly contagious virus, regardless of whether it is true or false.Citation20 Fact-checking information reduces the likelihood of believing misinformation,Citation21 and individuals who question the accuracy of online information have been shown to make better decisions about sharing content,Citation22,Citation23 which underscores the importance of fact-checking.
The few studies that investigated predictors for fact-checking information before sharing it on social media were based on the theory of planned behavior and the theory of reasoned action, both of which focus on the factors of oneself.Citation19,Citation24,Citation25 Considering the necessity of fact-checking health-related information before sharing it on social media, this must be explored further.
Accordingly, this study employs the Influence of Presumed Media Influence (IPMI) model,Citation26 which focuses on factors related to the presumed influence of media on others and expands plausible predictors of fact-checking before sharing on social media. The study participants were divided into low- and high-altruism groups to explore how individuals with different levels of altruism influence the effect of the IPMI model in the context of health misinformation.
This study explores the effects of media attention toward health misinformation on intentions toward fact-checking and the underlying mechanism. Specifically, individuals’ self-attention to health misinformation on social media leads to the presumed others’ exposure to health misinformation, and in turn, the presumed influence of health misinformation on others forms. The presumed influence of health misinformation on others affects the subsequent intentions to fact-check before sharing mediated by attitudes and personal norms based on the IPMI theory. The mechanism by which media attention to fact-checking before sharing affects personal behavior is based on the consideration of other people’s exposure to the health misinformation, which then leads to the perception of their influence. This process extends the effect of media attention beyond the individual and contributes to personal behavior change. In addition, this study explores the two-stage process, from individuals’ self-attention toward health misinformation to the presumed influence on others, and then to individuals’ fact-checking before sharing.
Literature Review
The IPMI model is derived from the third-person effect (TPE) hypothesis, which consists of two components: (1) The presumed media influence on others, and (2) The influence of presumed media influence.Citation27 According to the persuasive press inference,Citation28 the IPMI model posits that individuals tend to assume that others view or pay attention to media content that is similar to what they have been viewing, and this assumption strongly influences their attitudes and behaviors.Citation27
When individuals presume the influence of mass-media content on others, they react to the anticipated consequences of media influence.Citation27 In contrast to the TPE model, which relies on the discrepancy between presumed media influences on others and oneself to drive behavioral outcomes,Citation29 the IPMI model is based on the premise that behavioral outcomes can be solely driven by the presumed media influence on others,Citation27,Citation30 because this presumed influence excludes the presumed influence on oneself. The IPMI model is more accurate due to less interference from such factors as self-esteem and self-superiority.Citation31
The potential behavioral outcomes of presumed media influence are classified into three categories: prevention, coordination, and normative influence.Citation32,Citation33 Prevention refers to the behaviors relating to stopping or limiting the dissemination of the obviously harmful message.Citation32,Citation33 Though prior studies based on the IPMI model have explored prevention in the aspects of supporting censorship and legislation for misinformation,Citation31,Citation34 few studies focus on prevention in terms of fact-checking.
While the IPMI model was initially employed in the mass-communication context,Citation26 it can also accommodate the social-media context. Social media is a form of mass communication because it allows users to disseminate information to a substantial audience in a timely manner, thereby enabling top-down and one-to-many communication.Citation35 Moreover, social media can facilitate the sharing and reception of bottom-up information generated by ordinary people.Citation36 An earlier study investigated people’s intentions toward COVID-19 misinformation censorship and correction comprising interpersonal communication and examined the IPMI in the context of social media.Citation37 The IPMI model, which was developed in the pre-social media age, can fit the context of this current study.
Presumed Exposure and Presumed Influence
The IPMI model proposes a two-stage process where media indirectly influences people’s attitudes and behaviors due to perceived media influence on others.Citation27 In line with persuasive press inference,Citation28 the first stage of the IPMI model assumes a positive relationship between an individual’s personal attention and presumed others’ exposure to mass communication.Citation26,Citation27 Past studies have proposed a cognitive explanation of presumed media influence, specifically that people are naïve social scientists who attempt to explain how the world works and strive to have accurate perceptions of society,Citation32,Citation38 and they make subjective inferences about others’ exposure to similar media content based on their own attention.Citation26,Citation27
Several studies have examined the positive relationship between individuals’ attention and presumed others’ exposure. One study, for example, examined direct and indirect media influences on pro-environmental behaviors and observed a positive relationship between an individual’s attention and presumed others’ exposure to media information.Citation30 Studies on HIV prevention and on consumers’ willingness to pay for plant-based meat both established this same positive relationship.Citation39,Citation40 Thus, the first hypothesis is proposed:
H1: Individuals’ attention to health misinformation on social media is positively related to presumed others’ exposure to health misinformation on social media.
People tend to overestimate social media’s reach and level-of-exposure, which leads to the presumed influence on others.Citation28,Citation38,Citation41 Eveland et al suggested that presumed others’ exposure is positively related to the presumed media influence on others. Prior studies have examined the positive relationship between presumed others’ exposure and the presumed influence on others in various contexts, including anti-smoking messages,Citation42 pro-environmental behavior,Citation30 e-cigarette YouTube videos,Citation43 and HIV prevention.Citation39 A study on COVID-19 concluded that presumed others’ attention to COVID-19 mis- and disinformation on social media positively related to the presumed influence of misinformation on others.Citation37 If this is extended to the present study context, it can be reasonably assumed that presumed others’ exposure to health misinformation on social media is positively related to the presumed influence of health misinformation on others. Thus, Hypothesis 2 is as follows:
H2: Presumed others’ exposure to health misinformation on social media is positively related to the presumed influence of health misinformation on social media on others.
Influence of Presumed Influence
Attitude
The IPMI posits that individuals’ attitudes will be shaped by assumed media content’s influence on others.Citation27,Citation44 Attitude is a type of reaction based on positive or negative evaluations of an attitudinal object.Citation45 The attitudinal object bringing about a positive outcome leads to individuals’ positive evaluation.Citation46 When people perceive that others are susceptible to media influence, they form notions of what others are thinking and doing according to their perceptions of the media content to which they assume others are exposed.Citation28 This, in turn, motivates people to adjust their own attitudes and behaviors to better align themselves with others.Citation42,Citation47 A plausible explanation for this adjustment is humans’ innate desire to build and maintain relations by presenting themselves like everyone else.Citation48
Gunther and Storey posited the relationship between an individual’s attitude and the presumed influence on others.Citation27 Several studies conducted in distinctive contexts have detected a positive relationship. For example, Ho et al observed that the presumed influence of media messages on exercise and a healthy diet on others has a positive effect on their attitudes toward physical activity.Citation49 Wen et al found that the young people’s presumed influence of cosmetic surgery-related media on peers was positively associated with their attitudes to cosmetic surgery.Citation50 In the context of misinformation, a study about combating fake scientific news verified that scientists’ presumed harm to other scientists and the general public is positively related to their attitudes toward thwarting fake science news.Citation34 In light of these factors, the third hypothesis is proposed:
H3: Individuals’ presumed influence of health misinformation on social media on others is positively related to their attitude toward fact-checking health information before they share it on social media.
Attitude can shape behavioral intentions and guide behaviors.Citation51,Citation52 Because people are motivated to behave in a manner that will help them to attain their goals,Citation53 individuals are more likely to perform behavior they have positively evaluated. A study employed the theory of planned behavior to examine the collective impact of awareness, knowledge about fake news, the fear of missing out, and sadism related to an individual’s intention to fact-check news before sharing it on social media concluded that an individual’s attitude toward verifying news stories before sharing them is a significant predictor of their intention toward fact-checking before sharing.Citation54 Apuke et al reported a positive relationship between Nigerian social media users’ attitudes toward fact-checking information before sharing it and their intention to fact-check before sharing as well.Citation55 Based on these findings, the fourth hypothesis is as follows:
H4: An individual’s attitude toward fact-checking health information before sharing it on social media is positively related to intention toward fact-checking health information before sharing it on social media.
Personal Norms
An individual’s perception of the media’s influence on others shapes their normative perceptions.Citation56 A person forms their perception of norms by observing and talking with other people in their daily life.Citation57 This is due to people’s innate desire to develop appropriate behavioral modes by observing their social surroundings and adjusting their opinions and behaviors to conform to that of the perceived majority.Citation58,Citation59 Specific to the present study, people change their perceived social norms according to how they assume others are influenced by the media. Prior studies have found that social norms play a key explanatory role in the IPMI model.Citation60,Citation61
Personal norms are essentially internalized social norms,Citation62,Citation63 intrinsic moral compulsions to behave in a certain manner that refers to the adoption of specific behaviors to fulfill moral obligations.Citation64,Citation65 Personal norms are activated when an individual develops an awareness of consequences and assumes an ascription of responsibility.Citation65 “Awareness of consequence” refers to individuals’ tendency to associate their actions with the benefit to others, and “ascription of responsibility” describes individuals’ personal feelings about whether or not they are responsible for the consequences of their behaviors.Citation65 Someone’s personal norms toward a certain behavior allow them to envision a legitimate reason to perform it in order to avoid the guilt of violating their personal norms.Citation66–68
The IPMI model is premised on the assumption that people presume negative media content has a stronger impact on others than on themselves.Citation26 Davison noted that people presume media content that promotes undesirable behaviors will harm others.Citation29 Because presumptive harm is equal to an individual’s awareness of consequences, they are motivated to behave in a manner that will mitigate the adverse influence and eradicate the harmful source. When an individual presumes something is adversely influencing others, an ascription of responsibility can be potentially motivated, and in turn personal norms are activated. In terms of information valence, people tend to believe that others are more susceptible to negative information than they are.Citation69 This can cause them to develop a strong sense of social responsibility and be accountable for themselves to ensure that others are not further harmed.
A few studies have incorporated personal norms into the IPMI model. A study integrated personal norms into the IPMI model to predict scientists’ intentions to intervene in fake news.Citation34 Ho et al employed personal norms as a mediator between the presumed influence of health-related messaging and people’s intentions to adopt a healthy lifestyle.Citation49 This is a plausible merger because people adjust their social norms according to the influence they presume the media has on others and adopt acceptable modes of behavior.Citation70,Citation71 Including personal norms in the IPMI model provides an alternative mechanism to explain the indirect effects of the media on behavior.Citation47
Studies on this topic have observed that individuals consider the influence of social media on others when addressing misinformation, and people are motivated to discuss misinformation on social media when they believed the misinformation could result in negative consequences to others.Citation72–74 When an individual presumes health misinformation is influencing others, their awareness of possible consequences is activated. The prevalence of social media has led ordinary people to believe they can proactively protect others instead of relying on the government or a particular social media platform to censor information. Hence, this study posits the fifth and sixth hypotheses:
H5: Individuals’ presumed the influence of health misinformation on social media on others is positively related to their personal norms toward fact-checking health information before sharing it on social media.
H6: Individuals’ personal norms toward fact-checking health information before sharing it on social media are positively related to intention toward fact-checking health information before sharing it on social media.
Altruism
Altruism is a psychological trait that compels people to help others without seeking or receiving an external reward, which serves as an essential motivation to voluntarily help others.Citation75 Altruistic behavior is premised on “the sense that one knows what is best for others” or “concern for the well-being of others”.Citation76 According to Davison, the third-person perception of the TPE model could potentially result in people advocating for socially relevant actions if they perceive presumed media influence is harmful to others.Citation29 As it relates to the present study, because fact-checked information has been specifically prepared to be shared with others, fact-checking health information before sharing it on social media takes others’ benefits into account and is therefore an altruistic behavior.
The IPMI model was utilized in the present study to investigate respondents’ intention to the altruistic behavior of fact-checking health information before sharing it on social media. As past studies have shown, the presumed detrimental influence of health mis- and disinformation on others is the driving force that compels some people to take decisive action on misinformation on social media.Citation72–74 The IPMI model is premised on the belief that humans are intrinsically altruistic, while the TPE hypothesis is based on an individual’s perception of the media’s influence on others.Citation26,Citation27 Specific to the present study, Gunther et al posited that those who perceive the strong influence of mass media on others may react to this perception by associating this presumed influence on others and the corresponding behavior that inevitably occurs.Citation26
It should be noted that the IPMI model may yield different results among individuals with different levels of altruism. Prior studies have found that different levels of altruism resulted in different behaviors. For example, one study reported that individuals with high-level altruism had stronger perceived reciprocal benefits, and they are more concerned about helping others and sharing knowledge without expecting any reward.Citation77 An earlier study about climate change showed that people with higher altruism were shown to perceive more control and were more willing to sacrifice in pro-environmental behaviors.Citation78
In the context of the present study, someone with low levels of altruism may not consider benefits to others or alter their behavior according to assumptions of media’s presumed influence on others, which could render different results in the IPMI model than highly altruistic people. Accordingly, this study explored the differences between individuals with low- and high-levels of altruism within the IPMI model in the context of fact-checking health information before sharing it on social media. Hence, the following research question is proposed:
RQ: Does the IPMI differ among people with different levels of altruism?
and present the full hypothesized model.
Methods
Data Collection
An online survey was conducted in China by disseminating a questionnaire link on WeChat between September 10 and September 21, 2022. The survey was designed in English and translated into Chinese. To ensure the translation quality, the questionnaire was then translated back into English and checked against the original English-language form. A pilot survey was initially conducted among 94 participants to verify the rationality of this questionnaire.
Convenient and snowball sampling strategies were both employed in this study. The participants received informed consent, and they could only complete the questionnaires after they affirmed their consent, and their participation was entirely voluntary. While 1371 surveys were returned, only 1045 valid questionnaires remained after data cleaning. Questionnaires that did not meet the regular time for completion, or questionnaires having the same responses were excluded. All data were anonymous, and the participants were guaranteed confidentiality.
Sample
Of the final 1045 respondents, 532 (50.9%) were male. The participants ranged from 18–75 years of age, with an average age of 43.47 years (SD = 15.09). Of the respondents, 460 (44.0%) were 18–40 years of age, 500 (47.8%) were 41–65 years of age, and 85 (8.1%) were older than 65 years of age. Regarding their level-of-education, 73 (6.9%) completed junior middle school or below, 98 (9.4%) graduated from senior high school, 413 (39.5%) had a vocational education, 376 (36.0%) completed an undergraduate degree, and 85 (8.1%) respondents held a master’s degree or above. The median monthly household income was 5000–10,000 CNY. Of the respondents, 146 (14.0%) earned less than 5000 CNY per month, 473 (45.3%) earned 5000–9999 CNY each month, and 425 (40.7%) earned 10,000 CNY or more every month.
Measurements
Control Variables
The following demographic variables were used as control variables in this study: Age, gender, education level, and monthly household income.
Attention to Health Misinformation on Social Media
The respondents’ attention to health misinformation on social media was measured by a single item adapted from Shi et al rated on a 7-point Likert scale, ranging from 1 (none at all) to 7 (an extreme amount).Citation37 The item was “How much attention have you paid to health misinformation on social media?” (M = 5.13, SD = 1.63).
Presumed Other’s Exposure to Health Misinformation on Social Media
Presumed others’ exposure to health misinformation on social media was measured with four items adapted from Liao et al rated on a 7-point Likert scale (ie, 1 = not at all; 7 = very frequently).Citation30 The items measured the respondents’ perception of (1) Their family members’; (2) Their friends’; and (3) The general public’s exposure to health misinformation on social media (M = 4.98; SD = 1.29).
Presumed Influence of Health Misinformation on Social Media on Others
Presumed influence of health misinformation on social media on others was measured by four items adapted from Liao et al rated on a 7-point Likert scale (ie, 1 = no influence; 7 = a lot of influence).Citation30 The items measured the extent to which respondents presumed the influence of health misinformation on social media on: (1) Their family members; (2) Their friends; and (3) The general public (M = 5.04; SD = 1.26).
Altruism
The respondents’ levels of altruism were measured by five items adapted from Apuke and Omar and Fang and Chiu rated on a 7-point Likert scale (ie, 1 = strongly disagree; 7 = strongly agree).Citation25,Citation79 The items were: (1) When I have the opportunity, I love assisting others; (2) When I have the opportunity, it feels right to assist others to resolve their issues; (3) When I have the opportunity, I want to motivate and inspire others; (4) When I have the opportunity, I want to offer information to others; and (5) When I have the opportunity, I want to admonish others (M = 5.16; SD = 1.40).
Attitude
The respondents’ attitudes toward fact-checking health information before sharing it on social media was measured by six 7-point Likert semantic differential-scale items adapted from Poliakoff and Webb.Citation80 The items were: I think it is a/an (1) Bad–good; (2) Unenjoyable–enjoyable; (3) Pointless–worthwhile; (4) Unpleasant–pleasant; (5) Foolish–wise; and (6) Harmful–beneficial thing to fact-check before sharing health information on social media. A higher score represented a more positive evaluation of fact-checking health information before sharing it on social media (M = 5.21; SD = 1.35).
Personal Norms
Personal norms related to fact-checking health information before sharing it on social media were measured by six items adapted from Steg et al rated on a 7-point Likert scale (ie, 1 = strongly disagree; 7 = strongly agree).Citation81 The items were: (1) I feel personally obliged to fact-check before sharing health information on social media as often as possible; (2) I feel morally obliged to fact-check before sharing health information on social media, regardless of what others do; (3) I feel guilty if I do not fact-check before sharing health information on social media; (4) People like me should do everything they can to fact-check before sharing health information on social media; (5) I feel obliged to fact-check before sharing health information on social media in my daily behavior; and (6) I would be a better person if I fact-checked before sharing health information on social media (M = 5.26; SD = 1.42).
Fact-Checking Before Sharing
The respondents’ intention to fact-check health information before sharing it on social media was measured by six items adapted from Yu and Shen rated on a 7-point Likert scale (ie, 1 = strongly disagree; 7 = strongly agree).Citation82 The items were: I fact-check health information on social media before sharing it in the following ways: (1) I check other major news outlets; (2) I ask friends/family members; (3) I use search engines; (4) I check social media (ie, Weibo, WeChat); and (5) I consult other sources (M = 5.20; SD = 1.30).
Data Analysis
Data analysis was conducted with R Lavaan package (Version 0.6–15).Citation83 The measurement model was initially assessed using Confirmatory Factor Analysis (CFA) with factor loading and intercept constrained to be equal.
This study used Structural Equation Modeling (SEM) to test model fit and the relationship between variables. The demographic variables, age, gender, education level, and monthly household income were used as control variables in the model. The measurement model fit and the SEM model fit were assessed based on the following criteria: (1) The relative Chi-square ratio (χ2/df) should not exceed 5,Citation84 (2) The Root Mean Square Error of Approximation (RMSEA) value yielded should fall below 0.08,Citation85 and (3) The values obtained for both the Comparative Fit Index (CFI) and (4) Tucker–Lewis Index (TLI) should exceed 0.90.Citation86 Items with factor loadings under 0.60 were dropped in order to ensure that the resulting items had good reliability.Citation87
To explore if the IPMI differed among people with different levels of altruism, this study divided the respondents into a low-altruism group (n = 545) and a high-altruism group (n = 500) at the median value of altruism, based on previous studies.Citation88–90 A multigroup comparison analysis was conducted with factor loading and intercept constrained to be equal.
Results
Results indicated satisfactory model fit indices and statistics (χ2 = 1826.982, df = 536, χ2/df = 3.408; CFI = 0.904; TLI = 0.902; RMSEA= 0.068). and showed results for the structural equation model with the statistical information for the low- and high-altruism groups.
Figure 3 Structural equation model with path coefficients and factor loadings for the low-altruism group (n = 545, ***p<0. 001).
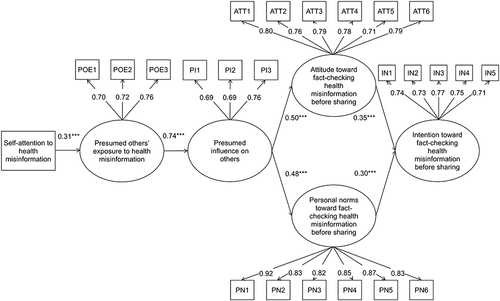
Figure 4 Structural equation model with path coefficients and factor loadings for the high-altruism group (n = 500, ***p<0. 001).
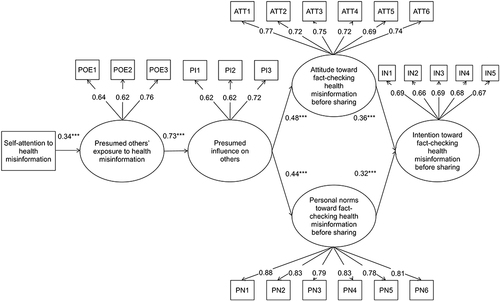
The structural equation model for the low-altruism group explained 26.2% of the variances. As it relates to the IPMI model, the regression model results demonstrated a positive relationship between these respondents’ attention to social media health misinformation and presumed others’ exposure to health misinformation on social media (β = 0.31; p < 0.001), which supported H1a. A positive relationship was observed between presumed others’ exposure to health misinformation on social media and the presumed influence of health social media misinformation on others (β = 0.74; p < 0.001), which supported H2a. A positive relationship was detected between the presumed influence of social media health misinformation on others and their attitude toward fact-checking health information before sharing it on social media (β = 0.50; p < 0.001), which supported H3a. There was a positive relationship between their attitude toward fact-checking health information before sharing it on social media and their intention to fact-check before sharing (β = 0.35; p < 0.001), which supported H4a. A positive relationship also existed between the presumed influence of social media health misinformation on others and their personal norms toward fact-checking health information before sharing it on social media (β = 0.48; p < 0.001), which supported H5a. Finally, there was a positive relationship between their personal norms toward fact-checking health information before sharing it on social media and their intention to fact-check before sharing (β = 0.30; p < 0.001), which supported H6a.
The structural equation model explained 28.1% of the variances in the high-altruism group. The results in this group supported Hypotheses 1b–6b. While the relationships between self-attention and presumed others’ exposure (β = 0.34; p < 0.001), between their attitude and intention (β = 0.36; p < 0.001), between their personal norms and intention (β= 0.32; p < 0.001) were higher among individuals with high-altruism, the relationships between presumed others’ exposure and the presumed media influence (β = 0.73; p < 0.001), between presumed media influence and their attitude (β = 0.48; p < 0.001), between presumed media influence on others and their personal norms (β = 0.44; p < 0.001) were higher in low-altruism group.
Discussion
This study investigated two questions. First, the IPMI model was implemented to examine the media’s influence on someone’s intention to fact-check health information before sharing it on social media. Second, the study participants were divided into low- and high-altruism groups to determine whether the IPMI model would vary according to different levels of altruism. All hypotheses proposed according to IPMI were supported, which confirms the applicability of the IPMI model in the context of fact-checking health information before sharing it on social media.
While the structural equation model for the low-altruism group explained 26.2% of the variances, the model explained 28.1% of the variances among the high-altruism group. The predictive power of the model for high-altruism group was higher. It is plausible that because the IPMI theory is premised on the belief that humans are intrinsically altruistic, individuals with high-altruism are in more alignment with the premise. Therefore, the structural equation model explaining the variances was higher for the high-altruism group.
The perceptual component of the IPMI model was supported for both low- and high-altruism groups, which is consistent with previous studies.Citation39,Citation40 However, prior studies focus on prevention—one of three consequent categories of the presumed media influence—in the aspects of supporting for censorship and legislation, which expects others to tackle with misinformation.Citation34,Citation37 The present study explored fact-checking health information before sharing it, which is a self-behavior to prevent people from misinformation. This extends the dimension of “prevention”.
In the context of fact-checking health information on social media, the present study demonstrated that users who pay more attention to health misinformation assume that others are also exposed to such content, and a positive relationship between presumed others’ exposure and the presumed influence of health misinformation on others was observed. This can be explained by the persuasive press inference theory and the extrapolation effect, which asserts that people pay attention to mass media and subsequently generate perceptions of a range of media content that other individuals assume represent media content on a broader, more general scale, and the media content is then expected to reach and influence a broader, more general audience.Citation28
The low-altruism group results are distinct from those of the high-altruism group for the perceptual components of the IPMI model. While the variable correlation between self-attention and presumed others’ exposure to health misinformation on social media was higher in high-altruism group (βlow = 0.31; βhigh = 0.34), the variable correlation between presumed others’ exposure and to health misinformation and presumed influence of health misinformation on others was higher in low-altruism group (βlow = 0.74; βhigh = 0.73). It is plausible that because individuals with higher altruism are more likely to perspective-taking,Citation91,Citation92 they are more considerate of other’s feelings or thoughts. Therefore, when high-altruism individuals pay attention to health misinformation on social media, they tend to assume that others are also exposure to health misinformation. Nonetheless, altruistic individuals are more likely to be optimistic and look for the bright side of things.Citation93,Citation94 Even though high-altruism individuals take notice of health misinformation, and assume that others are exposed to health misinformation, their optimistic mindset contributes to their perception that media had less of an influence on others.
Moreover, the behavioral component of the IPMI model was supported for both low- and high-altruism groups as well, which findings are in line with past studies.Citation40,Citation49 The respondents’ attitudes and personal norms mediated the relationships between the presumed media influence on others and their intention to fact-check health information before sharing it on social media. This is because the presumed media influence of health misinformation on others shapes an individual’s favorable or unfavorable opinions about fact-checking health information before sharing it on social media, which in turn predicts their tendency to fact-check health information before sharing it. Furthermore, an individual’s personal norms are formed after they receive and internalize media information that heightens their awareness of the consequences of their actions, highlights their responsibility to perform certain behaviors, and subsequently drives their behavior through feelings of guilt and shame.
The behavioral components of the IPMI model also yielded different results for low- and high-altruism groups. Presumed media influence on others was more related to the low-altruism group’s attitude (βlow = 0.50; βhigh = 0.48) and personal norms (βlow = 0.48; βhigh = 0.44) toward fact-checking health information before sharing it on social media. This could be because highly altruistic individuals might have an inherently high attitude and personal norms to fact-check health information before sharing it on social media, and indicates that the presumed influence of health misinformation on others may not be a strong predictor for an individual’s attitude and personal norms.
The positive relationship between the high-altruism group’s attitude toward health misinformation and their intention to fact-check health information before sharing it on social media was higher (βlow = 0.35; βhigh = 0.36). The positive relationship between the high-group’s personal norms to fact-checking health information before sharing it on social media and their intention to do the same was also higher (βlow = 0.30; βhigh = 0.32). A plausible explanation is that individuals with higher altruism are inclined to care for others’ welfare.Citation95 When they believe that fact-checking health information contributes to positive outcomes, such as benefiting and protecting others, they are more likely to fact-check health information on social media before sharing it. Similarly, when high-altruism individuals believe that they have personal norms toward fact-checking health information, they are more willing to fact-check before sharing.
Implications
The present study offers the following theoretical implications. Notably, the application context of the IPMI model was extended. Previous studies employed the IPMI model and focused on censoring and correcting health misinformation, while this study investigated the model in the context of fact-checking health information before sharing it on social media. Furthermore, this study explored the use of the IPMI model among individuals with different personality traits, examining the predictive power of the IPMI model in both low- and high-altruism groups yielded different results for different levels of altruism. Finally, this study integrated personal norms into the IPMI model, thereby providing an alternative mechanism to explain the media’s indirect effect on various behaviors.
The present study also yields two practical implications. Because an individual’s attention to health misinformation on social media could prompt them to fact-check information before sharing it, health officials could take appropriate measures to make social media users’ awareness of misinformation on social media. It is also worth noting that the varying results of the use of the IPMI model that were due to people’s different characteristics suggesting that health officials could evaluate individual users’ personalities and develop targeted interventions about fact-checking based on their character traits.
Limitations and Future Study
Certain limitations in this study should be addressed in future research. Like many studies that employ the IPMI model, this study used cross-sectional survey data to test the hypotheses, in which causality could not be inferred. It is therefore advisable to employ longitudinal survey data in future studies, which could further advance the theoretical framework. Moreover, this study employed self-reported data, which were more likely to be affected by a social-desirability bias,Citation96 because the respondents might have given socially acceptable answers they deemed would be “appropriate”, rather than truthful answers, to present a more positive self-image. Importantly, the sampling was mostly from the same region of China, which may have resulted in regional limitations by ignoring the different socioeconomic characteristics across the nation. Finally, this study only investigated the influence of different levels of altruism on the IPMI model, perhaps future research could evaluate the IPMI model among low- and high-egoistic groups.
Conclusion
Given the prevalence of health misinformation on social media, the IPMI model was investigated in the context of fact-checking health information before sharing it on social media, which is an effective strategy to address health misinformation. In the present study, the IPMI model revealed that an individual’s attention to health misinformation positively affected their intention to fact-check health information before sharing it on social media through a series of mediation processes, which confirms the model’s applicability in the context of fact-checking misinformation. This study also explored the IPMI model’s predictive power in relation to different personality traits. The findings revealed higher predictive power of the model for high-altruism group, which suggests particular strategies health officials could follow to encourage social media users to fact-check health information.
Ethical Statement
The Ethical Committee of the School of History and Culture of Shandong University approved the study (No.2022006). The study was conducted in line with the Helsinki Declaration. All participants provided informed consent for the research. The participants were assured of the confidentiality of the information and their participation was voluntary and anonymous. Only questionnaires fully completed by adult respondents were included in the analysis.
Disclosure
The author reports no conflicts of interest in this work.
References
- Chou WYS, Oh A, Klein WMP. Addressing health-related misinformation on social media. JAMA. 2018;320(23):2417. doi:10.1001/jama.2018.16865
- Li HOY, Bailey A, Huynh D, Chan J. YouTube as a source of information on COVID-19: a pandemic of misinformation? BMJ Glob Health. 2020;5(5):e002604. doi:10.1136/bmjgh-2020-002604
- Lin HC, Chang CM. What motivates health information exchange in social media? The roles of the social cognitive theory and perceived interactivity. Inf Manage. 2018;55(6):771–780. doi:10.1016/j.im.2018.03.006
- Niknam F, Samadbeik M, Fatehi F, Shirdel M, Rezazadeh M, Bastani P. COVID-19 on Instagram: a content analysis of selected accounts. Health Policy Technol. 2021;10(1):165–173. doi:10.1016/j.hlpt.2020.10.016
- Garrett RK. Social media’s contribution to political misperceptions in U.S. presidential elections. PLoS One. 2019;14(3):e0213500. doi:10.1371/journal.pone.0213500
- Vraga EK, Bode L, Tully M. Creating news literacy messages to enhance expert corrections of misinformation on Twitter. Commun Res. 2022;49(2):245–267. doi:10.1177/0093650219898094
- Le HT, Nguyen DN, Beydoun AS, et al. Demand for health information on COVID-19 among Vietnamese. Int J Environ Res Public Health. 2020;17(12):4377. doi:10.3390/ijerph17124377
- Garrett L. COVID-19: the medium is the message. Lancet. 2020;395(10228):942–943. doi:10.1016/S0140-6736(20)30600-0
- Zarocostas J. How to fight an infodemic. Lancet. 2020;395(10225):676. doi:10.1016/S0140-6736(20)30461-X
- Vosoughi S, Roy D, Aral S. The spread of true and false news online. Science. 2018;359(6380):1146–1151. doi:10.1126/science.aap9559
- Thapa DK, Visentin DC, Kornhaber R, West S, Cleary M. The influence of online health information on health decisions: a systematic review. Patient Educ Couns. 2021;104(4):770–784. doi:10.1016/j.pec.2020.11.016
- Bert F, Gualano MR, Brusaferro S, et al. Pregnancy e-health: a multicenter Italian cross-sectional study on internet use and decision-making among pregnant women. J Epidemiol Community Health. 2013;67(12):1013–1018. doi:10.1136/jech-2013-202584
- Erku DA, Belachew SA, Abrha S, et al. When fear and misinformation go viral: pharmacists’ role in deterring medication misinformation during the “infodemic” surrounding COVID-19. Res Soc Adm Pharm. 2021;17(1):1954–1963. doi:10.1016/j.sapharm.2020.04.032
- Lu X, Zhang R, Wu W, Shang X, Liu M. Relationship between internet health information and patient compliance based on trust: empirical study. J Med Internet Res. 2018;20(8):e9364. doi:10.2196/jmir.9364
- Lu X, Zhang R, Wu W, Shang X, Sun L, Zhu X. Is the internet different from traditional mass media in promoting patient compliance with mature treatments? Telemed E Health. 2020;26(1):69–77. doi:10.1089/tmj.2018.0292
- Wang Y, McKee M, Torbica A, Stuckler D. Systematic literature review on the spread of health-related misinformation on social media. Soc Sci Med. 2019;240:112552. doi:10.1016/j.socscimed.2019.112552
- Xie B, Wang M, Feldman R, Zhou L. Internet use frequency and patient-centered care: measuring patient preferences for participation using the health information wants questionnaire. J Med Internet Res. 2013;15(7):e2615. doi:10.2196/jmir.2615
- Walter N, Cohen J, Holbert RL, Morag Y. Fact-checking: a meta-analysis of what works and for whom. Polit Commun. 2020;37(3):350–375. doi:10.1080/10584609.2019.1668894
- Khan ML, Idris IK. Recognise misinformation and verify before sharing: a reasoned action and information literacy perspective. Behav Inf Technol. 2019;38(12):1194–1212. doi:10.1080/0144929X.2019.1578828
- Trilling D, Tolochko P, Burscher B. From newsworthiness to shareworthiness: how to predict news sharing based on article characteristics. Journal Mass Commun Q. 2017;94:38–60. doi:10.1177/1077699016654682
- Gottfried JA, Hardy BW, Winneg KM, Jamieson KH. Did fact-checking matter in the 2012 presidential campaign? Am Behav Sci. 2013;57(11):1558–1567. doi:10.1177/0002764213489012
- Pennycook G, McPhetres J, Zhang Y, Lu JG, Rand DG. Fighting COVID-19 misinformation on social media: experimental evidence for a scalable accuracy-nudge intervention. Psychol Sci. 2020;31(7):770–780. doi:10.1177/0956797620939054
- Pennycook G, Epstein Z, Mosleh M, Arechar AA, Eckles D, Rand DG. Shifting attention to accuracy can reduce misinformation online. Nature. 2021;592(7855):590–595. doi:10.1038/s41586-021-03344-2
- Alwreikat A. Sharing of misinformation during COVID-19 pandemic: applying the theory of planned behavior with the integration of perceived severity. Sci Technol Libr. 2022;41(2):133–151. doi:10.1080/0194262X.2021.1960241
- Apuke OD, Omar B. Fake news and COVID-19: modelling the predictors of fake news sharing among social media users. Telemat Inform. 2021;56:101475. doi:10.1016/j.tele.2020.101475
- Gunther AC, Bolt D, Borzekowski DLG, Liebhart JL, Dillard JP. Presumed influence on peer norms: how mass media indirectly affect adolescent smoking. J Commun. 2006;56(1):52–68. doi:10.1111/j.1460-2466.2006.00002.x
- Gunther AC, Storey JD. The influence of presumed influence. J Commun. 2003;53(2):199–215.
- Gunther AC. The persuasive press inference: effects of mass media on perceived public opinion. Commun Res. 1998;25(5):486–504. doi:10.1177/009365098025005002
- Davison WP. The third-person effect in communication. Public Opin Q. 1983;47(1):1–15. doi:10.1086/268763
- Liao Y, Ho SS, Yang X. Motivators of pro-environmental behavior: examining the underlying processes in the influence of presumed media influence model. Sci Commun. 2016;38(1):51–73. doi:10.1177/1075547015616256
- Chen L, Liu Y, Jiang X, Fu L, Zhu Y. How does media attention affect parental response behaviors to telecommunication fraud? Based on the influence of presumed media influence model. Curr Psychol. 2022;41(4):1728–1739. doi:10.1007/s12144-022-02882-w
- Tal-Or N, Tsfati Y, Gunther AC. The influence of presumed media influence: origins and implications of the third-person perception. In: Nabi RL, Oliver MB, editors. The SAGE Handbook of Media Processes and Effects. California: SAGE Publications Ltd; 2009:99–112.
- Tal-Or N, Tsfati Y. Influence of presumed media influence. In: Jan VB, editor. The International Encyclopedia of Media Psychology. Hoboken: Wiley-Blackwell; 2020:129–137.
- Ho SS, Goh TJ, Leung YW. Let’s nab fake science news: predicting scientists’ support for interventions using the influence of presumed media influence model. Journalism. 2022;23(4):910–928. doi:10.1177/1464884920937488
- Carr CT, Hayes RA. Social media: defining, developing, and divining. Atl J Commun. 2015;23(1):46–65. doi:10.1080/15456870.2015.972282
- Chen Z, Su CC, Chen A. Top-down or bottom-up? A network agenda-setting study of Chinese nationalism on social media. J Broadcast Electron Media. 2019;63(3):512–533. doi:10.1080/08838151.2019.1653104
- Shi J, Chen L, Tsang SJ. Integrating interpersonal communication into the influence of presumed media influence model: understanding intentions to censor and correct COVID-19 misinformation on social media. J Broadcast Electron Media. 2022;66(3):464–483. doi:10.1080/08838151.2022.2109638
- Eveland WP Jr, Nathanson AI, Detenber BH, McLeod DM. Rethinking the social distance corollary: perceived likelihood of exposure and the third-person perception. Commun Res. 1999;26(3):275–302. doi:10.1177/009365099026003001
- Hong Y. Extending the influence of presumed influence hypothesis: information seeking and prosocial behaviors for HIV prevention. Health Commun. 2021:1–14. doi:10.1080/10410236.2021.1975902
- Ho SS, Chuah ASF, Koh ELQ, Ong L, Kwan VQY. Understanding public willingness to pay more for plant-based meat: environmental and health consciousness as precursors to the influence of presumed media influence model. Environ Commun. 2022;16(4):520–534. doi:10.1080/17524032.2022.2051576
- Gunther AC, Christen CT. Projection or persuasive press? Contrary effects of personal opinion and perceived news coverage on estimates of public opinion. J Commun. 2002;52(1):177–195. doi:10.1111/j.1460-2466.2002.tb02538.x
- Paek HJ, Gunther AC. How peer proximity moderates indirect media influence on adolescent smoking. Commun Res. 2007;34(4):407–432. doi:10.1177/0093650207302785
- Cho H, Shen L, Peng L. Examining and extending the influence of presumed influence hypothesis in social media. Media Psychol. 2021;24(3):413–435. doi:10.1080/15213269.2020.1729812
- Tsfati Y, Cohen J. The influence of presumed media influence on democratic legitimacy: the case of Gaza settlers. Commun Res. 2005;32(6):794–821. doi:10.1177/0093650205281057
- Eagly AH, Chaiken S. The Psychology of Attitudes. 1st ed. California: Harcourt brace Jovanovich college publishers; 1993.
- Maio GR, Haddock G, Verplanken B. The Psychology of Attitudes and Attitude Change. 3rd ed. California: SAGE Publications Ltd; 2018.
- Tal-Or N, Cohen J, Tsfati Y, Gunther AC. Testing causal direction in the influence of presumed media influence. Commun Res. 2010;37(6):801–824. doi:10.1177/0093650210362684
- Wood W. Attitude change: persuasion and social influence. Annu Rev Psychol. 2000;51(1):539–570. doi:10.1146/annurev.psych.51.1.539
- Ho SS, Lee EWJ, Ng K, Leong GSH, Tham THM. For fit’s sake: a norms-based approach to healthy behaviors through influence of presumed media influence. Health Commun. 2016;31(9):1072–1080. doi:10.1080/10410236.2015.1038772
- Wen N, Chia SC, Hao X. Does gender matter? Testing the influence of presumed media influence on young people’s attitudes toward cosmetic surgery. Sex Roles. 2017;76(7):436–447. doi:10.1007/s11199-016-0680-4
- Ajzen I, Fishbein M. Attitudinal and normative variables as predictors of specific behavior. J Pers Soc Psychol. 1973;27(1):41. doi:10.1037/h0034440
- Ajzen I, Fishbein M. Attitude-behavior relations: a theoretical analysis and review of empirical research. Psychol Bull. 1977;84(5):888. doi:10.1037/0033-2909.84.5.888
- Fishbein M. An investigation of the relationships between beliefs about an object and the attitude toward that object. Hum Relat. 1963;16(3):233–239. doi:10.1177/001872676301600302
- Pundir V, Devi EB, Nath V. Arresting fake news sharing on social media: a theory of planned behavior approach. Manag Res Rev. 2021;44(8):1108–1138. doi:10.1108/MRR-05-2020-0286
- Apuke OD, Omar B, Tunca EA. Effect of fake news awareness as an intervention strategy for motivating news verification behaviour among social media users in Nigeria: a quasi-experimental research. J Asian Afr Stud. 2022. doi:10.1177/00219096221079320
- Rimal RN, Real K. How behaviors are influenced by perceived norms: a test of the theory of normative social behavior. Commun Res. 2005;32(3):389–414. doi:10.1177/0093650205275385
- Kincaid DL. From innovation to social norm: bounded normative influence. J Health Commun. 2004;9(S1):37–57. doi:10.1080/10810730490271511
- Festinger L. A theory of social comparison processes. Hum Relat. 1954;7(2):117–140. doi:10.1177/001872675400700202
- Bandura A. Social Foundations of Thought and Action: A Social Cognitive Theory. 1st ed. New Jersey: Prentice Hall; 1985.
- Ho SS, Poorisat T, Neo RL, Detenber BH. Examining how presumed media influence affects social norms and adolescents’ attitudes and drinking behavior intentions in rural Thailand. J Health Commun. 2014;19(3):282–302. doi:10.1080/10810730.2013.811329
- Yanovitzky I, Stryker J. Mass media, social norms, and health promotion efforts: a longitudinal study of media effects on youth binge drinking. Commun Res. 2001;28(2):208–239. doi:10.1177/009365001028002004
- Ajzen I. The theory of planned behavior. Organ Behav Hum Decis Process. 1991;50(2):179–211. doi:10.1016/0749-5978(91)90020-T
- Fishbein M. Attitude and the prediction of behavior. In: Fishbein M, editor. Readings in Attitude Theory and Measurement. New York: Wiley; 1967:477–492.
- Parker D, Manstead ASR, Stradling SG. Extending the theory of planned behaviour: the role of personal norm. Br J Soc Psychol. 1995;34(2):127–138. doi:10.1111/j.2044-8309.1995.tb01053.x
- Schwartz SH. Normative influences on altruism. In: Leonard B, editor. Advances in Experimental Social Psychology. Massachusetts: Academic Press; 1977:221–279.
- Baumeister RF, Vohs KD, Nathan DeWall C, Zhang L. How emotion shapes behavior: feedback, anticipation, and reflection, rather than direct causation. Personal Soc Psychol Rev. 2007;11(2):167–203. doi:10.1177/1088868307301033
- Massi Lindsey LL. Anticipated guilt as behavioral motivation: an examination of appeals to help unknown others through bone marrow donation. Hum Commun Res. 2005;31(4):453–481.
- Thøgersen J. Social norms and cooperation in real-life social dilemmas. J Econ Psychol. 2008;29(4):458–472. doi:10.1016/j.joep.2007.12.004
- Weinstein ND. Unrealistic optimism about future life events. J Pers Soc Psychol. 1980;39(5):806. doi:10.1037/0022-3514.39.5.806
- Eveland WP, Glynn CJ, Donsbach W, Traugott M. Theories on the perception of social reality. In: Eveland WP Jr, Carroll JG, editors. The SAGE Handbook of Public Opinion Research. California: SAGE Publications Ltd; 2008:155–163.
- Paluck EL. Reducing intergroup prejudice and conflict using the media: a field experiment in Rwanda. J Pers Soc Psychol. 2009;96(3):574. doi:10.1037/a0011989
- Arif A, Robinson JJ, Stanek SA, et al. A closer look at the self-correcting crowd: examining corrections in online rumors. Proceedings of the 20th ACM Conference on Computer-Supported Cooperative Work and Social Computing; 2017 February 25–March 1; New York, NY, USA: Association for Computing Machinery; 2017.
- Sun Y, Chia SC, Lu F, Oktavianus J. The battle is on: factors that motivate people to combat anti-vaccine misinformation. Health Commun. 2022;37(3):327–336. doi:10.1080/10410236.2020.1838108
- Tandoc EC, Lim D, Ling R. Diffusion of disinformation: how social media users respond to fake news and why. Journalism. 2020;21(3):381–398. doi:10.1177/1464884919868325
- Mowen JC, Sujan H. Volunteer behavior: a hierarchical model approach for investigating its trait and functional motive antecedents. J Consum Psychol. 2005;15(2):170–182. doi:10.1207/s15327663jcp1502_9
- McLeod DM, Eveland WP Jr, Nathanson AI. Support for censorship of violent and misogynic rap lyrics: an analysis of the third-person effect. Commun Res. 1997;24(2):153–174. doi:10.1177/009365097024002003
- Moghavvemi S, Sharabati M, Klobas JE, Sulaiman A. Effect of trust and perceived reciprocal benefit on students’ knowledge sharing via Facebook and academic performance. Electron J Knowl Manag. 2018;16(1):23–35.
- Knez I. Is climate change a moral issue? Effects of egoism and altruism on pro-environmental behavior. Curr Urban Stud. 2016;4(2):157–174. doi:10.4236/cus.2016.42012
- Fang YH, Chiu CM. In justice we trust: exploring knowledge-sharing continuance intentions in virtual communities of practice. Comput Hum Behav. 2010;26(2):235–246. doi:10.1016/j.chb.2009.09.005
- Poliakoff E, Webb TL. What factors predict scientists’ intentions to participate in public engagement of science activities? Sci Commun. 2007;29(2):242–263. doi:10.1177/1075547007308009
- Steg L, Dreijerink L, Abrahamse W. Factors influencing the acceptability of energy policies: a test of VBN theory. J Environ Psychol. 2005;25(4):415–425. doi:10.1016/j.jenvp.2005.08.003
- Yu W, Shen F. Does fact-checking habit promote COVID-19 knowledge during the pandemic? Evidence from China. Public Health. 2021;196:85–90. doi:10.1016/j.puhe.2021.05.005
- Rosseel Y. Lavaan: an R package for structural equation modeling. J Stat Softw. 2012;48:1–36. doi:10.18637/jss.v048.i02
- Wheaton B, Muthen B, Alwin DF, Summers GF. Assessing reliability and stability in panel models. Sociol Methodol. 1977;8:84–136. doi:10.2307/270754
- MacCallum RC, Browne MW, Sugawara HM. Power analysis and determination of sample size for covariance structure modeling. Psychol Methods. 1996;1(2):130. doi:10.1037/1082-989X.1.2.130
- Hu L, Bentler PM. Cutoff criteria for fit indexes in covariance structure analysis: conventional criteria versus new alternatives. Struct Equ Model Multidiscip J. 1999;6(1):1–55. doi:10.1080/10705519909540118
- Stevens JP. Applied Multivariate Statistics for the Social Sciences. 2nd ed. Hilldale: Erlbaum; 1992.
- Bati GF, Singh VK. Are you altruistic? Your mobile phone could tell. Proceedings of 2017 IEEE Smart World Ubiquitous Intelligence and Computing, Advanced and Trusted Computed, Scalable Computing and Communications, Cloud and Big Data Computing, Internet of People and Smart City Innovation Conference; 2017 Apr 4–8; San Francisco, NY, USA: IEEE; 2018.
- Johnson RC, Danko GP, Darvill TJ, et al. Cross-cultural assessment of altruism and its correlates. Pers Individ Dif. 1989;10(8):855–868. doi:10.1016/0191-8869(89)90021-4
- Nakao T, Tokunaga S, Takamura M, Nashiwa H, Hayashi S, Miyatani M. Altruistic people show no self-reference effect in memory. J Gen Psychol. 2012;139(1):29–41. doi:10.1080/00221309.2011.642027
- McAuliffe WHB, Forster DE, Philippe J, McCullough ME. Digital altruists: resolving key questions about the empathy–altruism hypothesis in an internet sample. Emotion. 2018;18(4):493–506. doi:10.1037/emo0000375
- Underwood B, Moore B. Perspective-taking and altruism. Psychol Bull. 1982;91(1):143–173. doi:10.1037/0033-2909.91.1.143
- Baek YM, Mee KE, Bae Y. My privacy is okay, but theirs is endangered: why comparative optimism matters in online privacy concerns. Comput Hum Behav. 2014;31:48–56. doi:10.1016/j.chb.2013.10.010
- Mukherjee S. Consumer altruism and risk taking: why do altruistic consumers take more risks? Int Rev Public Nonprofit Mark. 2021;19(3). doi:10.1007/s12208-021-00323-1
- Dahl A, Paulus M. From interest to obligation: the gradual development of human altruism. Child Dev Perspect. 2019;13(1):10–14. doi:10.1111/cdep.12298
- Johnson TP, Fendrich M, Hubbell A. A validation of the Crowne-Marlowe social desirability scale. Proceedings of the 57th Annual meeting of the American Association for Public Opinion Research; 2002 May 15–16; Florida, USA: Oxford University Press; 2002.