Abstract
Introduction
Money source influences risk-taking behaviors. Although studies consistently indicated that individuals demonstrate a higher propensity to make risky investments when utilizing non-labor income as opposed to labor income, explanations as to why non-labor income leads to continuously blowing money into risky investments are scarce.
Methods
The current study leverages a computational modeling approach to compare the differences in the dynamic risk investment process among individuals endowed with income from different sources (ie, non-labor income vs labor income) to understand the shaping force of higher risk-taking propensity in individuals with non-labor income. A total of 103 participants were recruited and completed the Balloon Analogue Risk Task (BART) with an equal monetary endowment, either as a token for completion of survey questionnaires (representing labor income) or as a prize from a lucky draw game (representing non-labor income).
Results
We found that individuals endowed with non-labor income made more risky investments in BART compared to those with labor income. With computational modeling, we further identified two key differences in the dynamic risk investment processes between individuals endowed with labor and those with non-labor income. Specifically, individuals endowed with non-labor income had a higher preset expectation for risk-taking and displayed desensitization towards losses during risk investments, in contrast to individuals with labor income.
Discussion
This study contributed to a better understanding of the psychological mechanisms of why individuals make more risk-taking behaviors with non-labor income, namely higher preset expectations of risk-taking and desensitization towards losses. Future research could validate these findings across diverse samples with varying backgrounds and adopt different manipulations of labor and non-labor income to enhance the external validity of our study.
Introduction
The field of economics has widely recognized that investment decisions are not solely based on the evaluation of costs and benefits. Money sources influence how people spend money.Citation1,Citation2 Individuals receive a wide variety of non-labor income (eg, lottery gains, stock market booms, inheritance, gift coupons, etc.) apart from labor income, which constitutes more than one-third of personal income in the family households.Citation3 From anecdotes of lottery winners ending up blowing all their money by irrational splashes to unplanned and excessive hedonic purchasesCitation4,Citation5 and even addictive gamblingCitation6,Citation7 observed among lottery winners, we know that a blessing of fortune is sometimes a curse in disguise, propelling individuals to make successive risky investments till it is all blown away. Previous studies have identified a generally higher propensity for making one-time investments with non-labor income compared to labor income.Citation8–10 However, these studies have not specifically examined the impact of labor income on long-term investment tendencies. Specifically, they have not explored whether individuals persistently engage in risky investments using non-labor income until all their funds are depleted, a phenomenon frequently observed in anecdotal accounts mentioned above. By delving deeper into this subject and considering that excessive risk-taking may imply impaired inhibitive control, as suggested by previous studies,Citation11 the current study aims to illustrate and empirically test the dysfunctional negative feedback mechanism that prevents individuals from stopping their risk-taking behavior in response to losses within the dynamic process of risky investment involving non-labor income.
The control theory naturally fits well with the dynamics of continuous risk-taking investments upon receipt of non-labor income until splashing it all away. The dynamic process of money splashing closely resembles a self-reinforcing feedback loop in the control theory, which constitutes two elements, namely the presence of an initial perturbance and a mal-functioning feedback system that exacerbates the magnitude of the initial perturbation.Citation12 In the case of a higher risk-taking propensity towards non-labor income, the initial perturbation is individuals’ initial preset higher expectations on risk-taking investments. The malfunctioning feedback system is flawed in the sense of an attenuated negative feedback mechanism. This prevents individuals from resisting risk-taking upon observation of negative consequences. In the next sections, we will review evidence in support of the existence of the two elements, respectively.
On the one hand, multiple theories and empirical evidence support the existence of an initially elevated perturbation to risk-taking tendency upon endowment of a non-labor income. The mental account theory and the house money effect both posit that money sources influence risk-taking propensity via money organizational structures (ie, labor income into regular earnings or say the in-house part and non-labor income into windfall gains or say the extra part in addition to house money).Citation7,Citation13,Citation14 Empirical studies along with this theory discovered enriched psychological implications associated with “windfall gains”, including less perceived easiness of money acquisition, less anticipation about the money, less planning on money usage, and discounted subjective value.Citation1,Citation7 These together imply that individuals may possess a schematized cognition towards non-labor income, which is strong and highly structured around multiple aspects spanning from money acquisition, money storage, and money valuation, to usage planning. The automatic activation of a strong schema might in turn push us towards a psychological preparedness to easily spend away non-labor income, which constitutes the initial elevated perturbation to risk-taking tendency upon receiving a non-labor income.
On the other hand, the Quasi-Hedonic Editing HypothesisCitation14 pointed to a lack of negative feedback mechanisms in dynamic risky investments with non-labor income, which increases individuals’ risk tolerance upon observation of a loss consequence in the midst of investment.Citation15–17 The Quasi-Hedonic Editing Hypothesis posits that the reference point at which investors psychologically distinguish gains from losses would be elevated into the gain domain upon receipt of non-labor income, such that losses are no longer net losses but a reduction of net gains.Citation14,Citation18 As individuals tend to integrate smaller losses with larger gains to maximize overall happiness,Citation14 they cancel small losses in each trial, leading to a desensitization to losses in the dynamic investment process. This subsequently precludes individuals from reducing their investment levels after experiencing losses. Therefore, we expect a malfunctioned negative feedback mechanism associated with investing non-labor income, which constitutes the shaping force of a persistent inclination towards higher risk-taking. To summarize, incorporating control theories to account for a mal-functioning of dynamic control in risk investments with non-labor income, we propose that non-labor income would elicit (1) an immediate higher preset expectation on the risk-taking propensity upon receipt of non-labor income and (2) engagement in a dynamic cycle of risk-taking behavior with desensitization towards loss outcomes (indexed by decreased learning rate towards loss outcomes) during the investment process.
To provide a direct empirical test on our hypothesized dynamic regulatory process, we leverage the Balloon Analogue Risk Task (BART) to capture individuals’ risk-taking propensities in dynamic risk decision-makingCitation19 and incorporate the computational modeling approach to depict their dynamic risk decisions beyond analyzing traditional indices of risk-taking indicators. BART is a prevalent measure of risk-taking propensities in dynamic investments.Citation19,Citation20 BART coupled with computational modeling could capture how individuals differ in risk-taking at the beginning and how they learn from past gain or loss outcomes to decide future risk investmentsCitation21,Citation22 and thus enable a direct test on our hypothesized dynamic mechanisms of how non-labor income leads to higher risk investments. BART also has high ecological validity with a moderate correlation with real-life risk behaviors,Citation19,Citation23,Citation24 especially gambling behavior, which is one major harmful avenue through which individuals splash non-labor income. In this study, we incorporated well-established computational models from previous studies as candidate models, including the Bayesian sequential risk-taking (BSR),Citation25 the Target model,Citation21 the Scaled Target Learning (STL) model and its extension, and the Scaled Target Learning with Decay (STL-D).Citation22 Within the optimal model, by intergroup comparisons on key parameters depicting risk-taking propensities at the beginning and sensitivity (ie, learning rates) towards losses, we can have a direct test on how labor versus non-labor income elicit different preset risk-taking expectation at the beginning and elicit difference learning processes towards negative investment outcomes in the midst of dynamic investment.
In brief, in the current study, we investigate how income sources (ie, the non-labor versus the labor income) influence individuals’ dynamic risky decision-making process within the BART paradigm. We hypothesize that individuals with non-labor income show persistently higher risk preference across time in the dynamic risk investment process than those with labor income. Within a computational modeling approach, we further hypothesize that individuals with non-labor income would preset a higher target number of pumps and have a decreased learning rate towards loss in BART, which might be the underlying reason why they show persistent higher risk preference.
Methods
Participants
We selected a target sample size of 51 per group to ensure adequate power to detect a medium-sized effect (effect size Cohen’s d = 0.5, Type I error α = 0.05, power 1-β = 0.8)Citation26 based on a G*power calculation. However, in consideration of potential emotional issues, such as moderate-to-severe levels of depression or anxiety symptoms based on self-report in college students,Citation27 we implemented a participant exclusion rate of 20%. Additionally, considering a 20% failure rate in the manipulation of experimental psychology paradigm,Citation28 we ultimately recruited 172 participants from a local university through campus postings. This rigorous recruitment process was designed to ensure both an adequate number of participants for valid manipulations and that they were free from potential emotional issues. The age of the participants ranged from 17 to 26 (Mage = 20.92, SDage = 1.86). All participants did not have a reported history of mental disorders or substance use.
Participants completed the Beck Depression Rating Scale (BDI),Citation29 Patient Health Questionnaire-9 (PHQ-9),Citation30 dimension of neuroticism in NEO Personality Inventory (NEO-PI-N),Citation31 Trait Anxiety Inventory (TAI),Citation32 and the Barratt Impulsiveness Scale (BIS)Citation33 before the experiment to exclude outliers in the personality and mood (anxiety or depression) measures. We also used the Positive and Negative Affect Schedule (PANAS)Citation34 to compare the arousal of positive emotion and negative emotion after the manipulation of mental account.
For our final sample who had completed all of the questionnaires, we excluded individuals with potential mental disorders, extreme outliers, and those misclassifying endowment sources. Eventually, 103 participants were included in the analyses. Information on sample exclusion is available in Supplementary Material S1.
Manipulation of Sources of Income
We manipulated the sources of income by randomly assigning participants to one of the two experimental conditions, ie, the labor income condition and the non-labor income condition. Participants in the two groups received different cover stories about the sources of the same amount of endowment. For the labor income group, ¥15 of the endowment was given to them immediately as a token for completing the questionnaire. For the non-labor income group, a gift was given as a token for completing the questionnaire as well. This step aimed to prevent their potential mental account of subsequent lucky prizes into labor income. Then, participants in the non-labor income group were invited to play a lucky draw game. They were told that in this game, they had a small chance (10%) to win a large prize (¥15) and a large chance (90%) to gain nothing. To facilitate the manipulation of a windfall gain, a confederate would draw together with the participant. Participants would see themselves win a large prize and the confederate win nothing. Upon hearing an announcement “Congratulations! You win ¥15!”, participants would immediately receive the money via WeChat or AliPay.
Immediately after receiving the money, participants were asked to complete the PANAS scale and were told that they could use the ¥15 as principal to complete the BART task. Participants were informed that they could win more money if they played well but could lose money if they played badly. They can also avoid the BART task by giving up pumping up any balloons. Participants first pumped two balloons for exercise before the formal experiment to familiarize themselves with the task procedure. For the manipulation check, participants were asked to report their perceived reason why they got the ¥15, which they could choose from “Labor income”, “Non-labor income” or “Other Reason but Not Listed in the Choices”. After the manipulation check, 10% of the amount of money obtained in BART was given as an additional bonus ().
Balloon Analogue Risk Task
We administered a 30-trial BART task adapted from Lejuez’s study.Citation19 The task was programmed in E-prime 2.0. Participants were told that their goal was to earn as much money as possible. In each pump, participants had to choose between “pumping” or “stop pumping” by pressing one of two buttons (). If participants chose to pump, the balloon had a certain probability of explosion. If the balloon did not explode, ¥0.05 would be added to the temporary reserve. If the balloon exploded, participants would lose all the money from the temporary reserve. If participants stop pumping before the balloon explodes (they can even give up pumping at the beginning of any balloon to avoid potential loss). The next trial would start either after the balloon explodes or after participants stop pumping. A new balloon would appear on the screen, and the temporary reserve would be reset to zero at the beginning of each trial. The maximum number of pumps allowed for each balloon was 128. The conditional probability of explosion after each pump, if the prior pumps did not explode, was equally set to 1/128. Thus, the probability of explosion would increase as the balloon got bigger, and the balloon would explode for certain after 128 pumps. At the end of 30 trials, participants would collect all the money from their permanent reserve as a part of their compensation.
Analytic Strategies
Beyond the manipulation check, we also used the PANAS scale to verify the effectiveness of the manipulation of mental accounts. We hypothesize that participants in the non-labor income group who won the lucky draw showed higher positive emotions than those in the labor income group. To test this hypothesis, we used independent sample t-tests to compare positive emotions between groups.
Further, to test the hypothesis that participants in the non-labor income group showed higher risk preference than those in the labor income group, we used independent sample t-tests to compare between-group differences in risk preference. Four behavioral indicators related to risk decision-making in BART were analyzed: (1) adjusted pumps or the average number of pumps of win balloons (AP); (2) the number of pop-balloons (NP); (3) the average number of pumps of win-balloons immediately following a win (AP+); (4) the average number of pumps of win-balloons immediately following a loss (AP-). To explore how the effect of explosion outcomes on the last balloon influenced the risk preference in the next balloon, we used a linear mixed model to investigate the main effects and interaction effects between the source of the money, the number of trials, and feedback from the last trial on the number of pumps in each balloon. Learning was indicated by the main effect of feedback from the last trial on the number of pumps.
To formally compare the differences in the dynamic risk investment process between individuals with different mental accounts, we built four candidate computational models (Bayesian Sequential Risk-taking Model, Target Model, Scaled Target Learning Model, and Scaled Target Learning with Decay Model) in Rstan (R version 4.1.3). Models were fit separately for participants with different mental accounts. Settings of prior distributions and ranges of estimated parameters were shown in Supplementary Material S2. Model comparisons were indicated by leave-one-out cross-validation (LOO) information criteria, a common method to estimate out-of-sample predictive accuracy from Bayesian models.Citation35 After selecting the optimal model, we used a hierarchical Bayesian approach to simultaneously acquire group and individual-level parameter estimation with hierarchical Bayesian estimators in Rstan. We then performed between-group parameter comparison for different mental accounts using the posterior distribution of different parameters within 89% of the highest density intervals (HDIs).Citation36
We also performed model recovery and parameter recovery to validate the robustness of parameter estimation in the optimal model. The details of model recovery and parameter recovery are shown in the Supplementary Materials S3 and S4 separately. For model recovery, we first used the original parameter estimates for each individual to simulate their pumping process in each opportunity. We then calculated the Pearson correlation between the number of pumps in each opportunity in the original data and the simulated data to check if the simulated pump of each trial in BART could capture the key characteristics of participants’ original responses. For parameter recovery, we first used individual-level parameter estimates to simulate the pumping process of each individual and derive parameter estimates for each individual from the simulated data. We then calculated the Pearson correlation between original and recovered parameter estimates across different participants. We repeated the above procedure 20 times to derive the mean (SD) Pearson correlation indices for each parameter to evaluate the stability of parameter estimation.
In the following part of this section, we will outline the formalism of the computational models. If these specifics are not of interest to the reader, they can proceed to Results.
Formalism of Computational Models
Bayesian Sequential Risk-Taking Model (BSR)
BSR model assumes that individuals calculate an optimal number of pumps for each trial based on their perceived probability of explosion. The perceived probability of explosion is updated in a Bayesian Observer manner, determined by the number of successful () versus the total pumps (
) occurred before trial k (f1) (Wallsten et al, 2005). Using this probability as evidence, participants then determine a target number of pumps (
) for the upcoming trial (f2). BSR model further assumes that the probability that participants will pump on opportunity l on trial k is determined by
and behavioral consistency, denoted as β (f3) (Ji et al, 2021). Free parameters in this model include (1) initial beliefs about the probability of balloon explosion (
), with 0<α<µ; (2) risk-taking propensity (γ); (3) behavioral consistency (β).
In the kth trial, the update process is:
The target number of pumps to make on trial k is:
And the actual probability that the participants will pump on trial k for a given pump opportunity l (k = 1, 2, …) is calculated as:
Target Model
The Target model assumes that participants select a target number of pumps () before the first trial at the very beginning (Wallsten et al, 2005). Then, participants adjust the target number of pumps of the current trial (
) down if the balloon prior to trial k explodes, and up if it does not (f4). Parameters that characterize the size of the adjustment (ie, learning rate) are vwin after a win, and vloss after a loss. α controls how rapidly the adjustment decreases with experience.Citation22 The target number of pump is adjusted on each trial according to the following formula:
Free parameters include: (1) the initial target number of pumps (); (2) sensitivity for wins (vwin): (3) sensitivity for losses (vloss); (4) decay parameter (α); (5) behavioral consistency (β). The probability of stopping pumping in each pump is the same as (f3).
Scaled Target Learning (STL) Model
The Scaled Target Learning (STL) model is similar to the Target Model except that it provides an alternative rule about how the target number of pumps () is updated based on the outcome in the previous trial (f5). The separate learning rates for wins and losses (ie, vwin and vloss) reflect an individual’s different sensitivity to win and loss outcomes. nmax is the total number of pumps allowed in each trial (ie, 128). Because ωk is scaled by nmax when fitting STL-D, so that the value of ωk is between 0 and 1. Free parameters include (1) the initial target number of pumps (
); (2) sensitivity for wins (vwin): (3) sensitivity for losses (vloss); (4) behavioral consistency (β).
The probability of stop pumping in each pump is the same as (f3).
Scaled Target Learning with Decay (STL-D) Model
Both STL model and STL-D model describe learning as adjustments to the target number of pumps in reaction to outcomes in the task. A critical advancement to the STL-D model compared to STL model is that it assumes participants make adjustments to the target number of pumps denoted by α, with the size of adjustment smaller as k increases (f6) (Zhou et al, 2021):
Free parameters include: (1) the initial target number of pumps (); (2) sensitivity for wins (vwin): (3) sensitivity for losses (vloss); (4) discount parameter (α); (5) behavioral consistency (β). The probability of stopping pumping at each pump is the same as (f3).
Results
Data from 103 participants (Mage = 21.02, SDage = 1.91) were included in the analysis. There were no significant between-group differences in demographics, personality, anxiety or depression measurements between the labor income group and the non-labor income group (). Independent sample t-tests suggested that participants in the non-labor income group showed higher positive emotion than those in the labor income group (t(101)= 2.36, 95% CI = [1.05, 12.11], p < 0.05), while there was no significant difference in the negative emotion between the two groups (t(101)= 0.57, 95% CI = [−3.86, 6.97], p = 0.57) based on the PANAS scale, suggesting the effectiveness of the manipulation of mental accounts.
Table 1 Group Differences in the Demographic Variables (M ± SD)
Independent sample t-tests suggested that individuals in non-labor income showed higher risk preference convergently across four behavioral indicators (): (1) adjusted pumps (AP): t(101)= 3.43, 95% CI = [2.76, 10.33], p < 0.001, Cohen’s d = 0.68; (2) number of pop-balloons (NP): t(101)= 2.04, 95% CI = [0.03, 2.20], p = 0.044, Cohen’s d = 0.41; (3) mean number of pumps of win-balloons immediately following a win (AP+): t(101)= 3.47, 95% CI = [2.99, 10.95], p < 0.001, Cohen’s d = 0.69; (4) mean number of pumps of win-balloons immediately following a pop (AP-): t(99)= 3.60, 95% CI = [3.20, 11.04], p < 0.001, Cohen’s d = 0.72.
Table 2 Group Comparisons of BART Indicators Between Labor Income Group and Non-Labor Income Group (M ± SD)
To trial-by-trial explore how the manipulation of the source of income and feedback from the last trial in BART would influence the current trial, we used a linear mixed model to examine the main and interactional effects between the source of income, the number of trials, and feedback (ie, the explosion outcomes of the last trial) on the number of pumps of the current trial. We found significant main effects of the source of income (F(1110) = 12.04, 95% CI = [−9.18, −2.55], p < 0.001), the number of trials (F(29,2873) = 2.31, p < 0.001), and feedback (F(12,884) = 23.85, 95% CI = [1.45, 3.38], p < 0.001). The three-way interaction among the source of income, the number of trials, and feedback (F(29,2876) = 1.18, 95% CI = [−1.62, 2.25], p = 0.234), as well as the second-order interactions (between the source of income and the number of trials: F(29,2873) = 1.36, p = 0.096; between the source of income and feedback: F(12,884) = 0.10, 95% CI = [−1.62, 2.25], p = 0.749; between the number of trials and feedback: F(29,2876) = 1.07, p = 0.363), were found to be non-significant in the linear mixed model analysis.
The post hoc test showed that the number of pumps in the current trial in the non-labor income group was significantly higher than the number of pumps in the current trial in the labor income group. The number of pumps in the current trial following a win was also significantly higher than the number of pumps in the current trial following a loss (t(3088)= 2.88, p < 0.01, ). In addition to reverifying the previous conclusion that participants in the non-labor income group showed higher risk preference than participants in the labor income group, these results also implied that participants learn from past outcomes to adjust the number of pumps in the current trial based on outcomes from the last trial for both groups of participants. Meanwhile, we did not find a significant interaction between the source of income and the number of trials. The result showed that in the non-labor income group, the number of pumps was higher than in the labor income group, and this higher risk-taking propensity persisted with the evolvement of time ().
Figure 2 The main effects of feedback from the last trial on the number of pumps in the current trial (A) Comparison of the number of pumps between the non-labor income group and labor income group across trials (B).
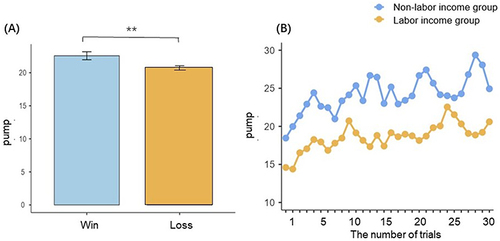
Model comparisons of four candidate models (ie, BSR, Target, STL, and STL-D) between experimental conditions (ie, labor income and non-labor income) were shown in . For the labor income group, STL-D had the numerically smallest LOOIC. For the non-labor income group, BSR had the numerically smallest LOOIC and had no significant difference with STL-D for goodness-of-fit by model comparisons (Mean Difference < SE). As STL-D captured the learning rate for winning and losing trials, which could reflect our interested psychological processes of how individuals learn from gains and losses underlying risk-taking behavior, we selected the STL-D model as the optimal model for parameter comparison across conditions. Model recovery showed that the simulated data from the optimal model STL-D could capture key characteristics of the original data (Supplementary Materials S3). Parameter recovery showed stable parameter estimation of STL-D, indicated by the high correlation between original and recovered parameters (Supplementary Materials S4).
Table 3 Mean (SE) of LOOICs for Different Candidate Models and Model Comparison Results
Descriptive statistics and 89% CI for the group-level estimation of each parameter were shown in . We found a credible between-group difference of learning rate in loss trial (vloss) (89% HDI: (0.03, 0.19)), target number of pumps before the first trial () (89% HDI: (−0.11, −0.01)), and the inverse temperature (β) (89% HDI: (0.03, 0.24)) between the labor income and the non-labor income group. These results indicated that participants in the non-labor income group (1) preset a larger target number of pumps (
) at the beginning of the game; (2) were less sensitive to feedback of losses (vloss); (3) acted more randomly, in which pump probability was less dependent on the target number of pumps (β).
Table 4 Parameter Estimation and Between-Group Comparison Results (M ± SD)
Discussion
The current study uncovers that the source of money affects the dynamic risk investment process. Individuals made a persistently higher level of risk investments with money from non-labor sources than from labor sources. Computational model analyses further revealed that individuals (1) preset a higher targeted number of pumps at the very beginning of the game upon money endowment (indicated by a higher ), (2) had a decreased learning rate towards losses (indicated by a smaller vloss), and (3) displayed more random decision-making (indicated by a smaller β) compared to individuals in the labor income group. These results suggested that individuals tended to splash non-labor income via continuous risky investments across time. Our study offered a direct empirical test on our proposed mechanism underlying this persistent higher risky propensity over non-labor income, ie, higher preset risk-taking propensity at the beginning and the malfunctioning of the negative feedback loop in the dynamic control process.
Elevated risk-taking propensity in individuals endowed with non-labor income through traditional risk-taking indicators of BART
Our findings suggest that individuals endowed with non-labor income showed a higher risk preference convergently across four behavioral indicators compared to those endowed with labor income. These indicators are widely used to assess risk-taking tendencies and have been correlated with various risk-related behaviors. For example, AP has been shown to be positively correlated with substance use and other health-related risk behaviors,Citation19 such as smokingCitation37 and alcohol consumption.Citation38 AP+ and AP- have been used as effective indices of risk preference stability and sensitivity to risky decision feedback, leading to the detection of decision-making biases in suicide attempters with major depressive disorder.Citation39
The decision differences between individuals in the labor income and non-labor income groups become more apparent through trial-by-trial analysis. By examining the influence of the source of income and feedback from the previous trial on the current trial using a linear mixed model, we identified the presence of a learning effect in BART. Participants adjusted their strategies based on the outcome of the previous trials, reducing the number of pumps in the current trial after balloon bursts and vice versa. However, individuals in the non-labor income group, particularly during loss trials, exhibited a dampened learning effect, maintaining their higher risk-taking propensity over time. This finding aligns with previous observations in individuals with high overall risk scores, where risk-takers tend to respond increasingly to successive balloons even after an explosion.Citation40 Moreover, a previous study also reported reduced feedback-related negativity (FRN) of individuals with higher risk preference in response to the balloons that exploded during decision-making.Citation41 This altered FRN implies a diminished sensitivity to negative feedback, further supporting the alignment of these findings with our finding.
Computational modeling
Our study represents a novel contribution by employing computational modeling to directly investigate the malfunctioning negative feedback mechanism underlying individuals’ persistent elevated risk-taking behavior in the presence of non-labor income. To the best of our knowledge, this is the first study to employ such an approach, highlighting the unique and innovative aspect of our research. Our finding that individuals with non-labor income showed higher risk preference in dynamic risk decision-making and had higher target number of pumps () were in line with our hypotheses and with existing studies in static decision-making.Citation7,Citation42 The higher values of
indicate that participants with non-labor income have more willingness to take risks than those with labor income even at the beginning of the BART. Previous consumption decision-making studies have also found that money in the windfall gains account that has an irresponsibly higher marginal propensity to consumeCitation4,Citation7 than other accounts even before consumption. Findings from multi-period gambling also show that individuals have the strongest willingness to take risks immediately after a large win.Citation43
Moreover, computational modeling showed that the vloss of participants in the non-labor income group was significantly lower than that in the labor income group. That is, individuals with non-labor income were less sensitive to the negative feedback in BART. This finding could be backed up by the edition rule elicited by the Quasi-Hedonic Editing Hypothesis.Citation14 The reference point for participants in our labor income group is likely to be 0, whereas the reference point could increase to 15 (ie, the number of gains from the lucky draw) for the non-labor income group, representing a large gain at the beginning. To maximize happiness, the non-labor income group is less likely to exhibit loss aversion because losses fewer than 15 are no longer “losses” in their mind in the process of integration with larger gains, and thus this results in a desensitization to losses. Until the winnings are completely depleted, losses are canceled out.Citation14,Citation44 The increased target number of pumps () and desensitization towards losses (νloss) found in our experimental study provides clear support for the Quasi-Hedonic Editing Hypothesis. Further, the desensitization towards losses retains subsequent risk-taking at a continuously high level.
Outside of our proposed malfunctioning control mechanisms, we additionally found that participants in the non-labor income group based their actions less on the target number of pumps and more at random and consequently had less rationality in decisions, as indicated by their lower behavioral consistency parameter β. Further, because the target number of pumps is primarily based on learning from the previous trial, the β can also be interpreted as a segregation of outcomes between trials.Citation21,Citation45 In other words, participants with lower β values will base their actions less on the target number of pumps they had in mind but on the outcome of a particular trial.
Theoretical and practical implications
Our study has both theoretical and practical implications. In the theory setting, we provided direct empirical support to our proposed malfunctioning of control in the dynamic investment of non-labor income. That is, non-labor income leads to an initial perturbance (ie, a higher prior expectation of risky investment) and a malfunctioned negative feedback loop to cool down this initial perturbance (ie, desensitization towards loss outcomes in the investment process, which indexed by a lower learning rate towards losses). These two elements together constitute the fundamental propeller of individuals’ continuous risk-taking behaviors. With dynamics of how non-labor income elicits continuous risk investment understood, our study can shed important light on how to prevent individuals from immediately splashing non-labor income away, guiding them toward more rational investment and consumer behaviors. Effective intervention could target at cooling down consumption or risky investment immediately upon individuals receiving the non-labor income, ameliorating loss desensitization, and enhancing investment rationality in the long term. This intervention, in some circumstances, could also prevent individuals from gambling addiction with non-labor income, as individuals with gambling disorders exhibited similar malfunctioning of control, indicated by desensitization to losses in the gambling process (Beck et al, 2009; FitzGerald et al, 2009; Romanczuk-Seiferth et al, 2005).
Limitations
The present study has the following limitations. A key limitation of this study is that our experiment only involves undergraduate students. A considerable proportion of participants were excluded due to failure in the manipulation of mental accounts. This insusceptibility to manipulation may be due to certain sample characteristics, ie, higher educational background.Citation46 Future studies could replicate our results using non-student samples to eliminate the influence of higher educational background on mental account manipulations and test the generalizability of our results. Second, the success rate of manipulation of income sources to strictly labor versus non-labor sources is limited revealed by a direct manipulation check. This could also possibly be resulted from individual differences in money source attribution or a lack of effort expenditure in the questionnaire completion task. Future studies could pay participants for completion of tasks involving a larger amount of labor.
Conclusion
To deepen our understanding of the influence of non-labor income on higher risk-taking propensity, the current study employs computational modeling to compare the dynamic risk investment processes among individuals with different income sources. In the theoretical setting, our study provides compelling empirical evidence supporting the proposed psychological mechanism underlying the continuous risk-taking behavior associated with investing non-labor income over time. Specifically, we found that this behavior can be attributed to an initial heightened level of targeted risk investments, a desensitization towards investment losses, and a reduced level of rationality in the investment process. These findings contribute to a deeper understanding of the psychological factors driving risk-taking behavior in individuals with non-labor income. In the practical setting, windfall gains are a common occurrence, ranging from receiving a coupon to winning the lottery, and they often constitute a significant portion of our income. Our study delivers an important message to the public: to retain windfall gains and prevent them from being quickly depleted, it is crucial to consciously counter our automatic tendency towards casual expenditure upon receiving the money and to constantly remind ourselves of the potential for significant investment losses. By raising awareness of these risks, individuals can make more informed financial decisions and better manage their windfall gains. It is important to note that the convenience sampling principle we employed in participant recruitment may have some limitations in terms of generalizability. To enhance the broader applicability of our findings, future research should aim to validate our results across diverse samples with varied backgrounds and explore different types of labor and non-labor income. By doing so, we can gain a more comprehensive understanding of the factors influencing continuous risk investment associated with non-labor income.
Preregistration of Analysis Plans
We did not preregister the research with or without an analysis plan in an independent, institutional registry.
Preregistration of Studies
We did not preregister the research in an independent, institutional registry.
Data Sharing Statement
Data for this research project will be available upon reasonable request to the corresponding author.
Ethics Approval
All procedures performed in studies involving human participants were in accordance with the ethical standards of the institutional and/or national research committee and with the 1964 Helsinki Declaration and its later amendments or comparable ethical standards. This study was reviewed and given ethical clearance by the Institutional Review Board of Southwest University (IRB NO.H23171).
Consent to Participate
Before participation, all participants provided written informed consent.
Consent to Publish
Participants signed informed consent regarding publishing their data and photographs.
Disclosure
The authors declare no conflicts of interest in this work.
Acknowledgments
We would like to thank all the participants and the supports from all the research assistants. This work was supported by Innovative Practical Training Program for College Students, Institute of Psychology, Chinese Academy of Sciences (project number D202101) and the National Natural Science Foundation of China (project number 72033006 and 82171535), which provided support for the data collection. The manuscript has been posted as a preprint on PsyChinaXiv to ensure timely access for the academic community to our research findings (DOI: 10.12074/202309.00151V1). This paper has been uploaded as a preprint: https://chinaxiv.org/abs/202309.00151.
Additional information
Funding
References
- Gajewski JF, Meunier L, Ohadi S. Do sources of money matter in risk-taking behaviour? Appl Econ. 2022;54(4):443–466. doi:10.1080/00036846.2021.1963412
- Thaler RH. Mental accounting matters. J Behav Decis Mak. 1999;12(3):183–206. doi:10.1002/(SICI)1099-0771(199909)12:3
- Lawson MM, Rasker R, Gude PH. The importance of non-labor income: an analysis of socioeconomic performance in western counties by type of non-labor income. J Reg Anal Policy. 2014;44(2):175–190.
- Kim EL, Tanford S. The windfall gain effect: using a Surprise discount to stimulate add-on purchases. Int J Hosp Manag. 2021;95:102918. doi:10.1016/j.ijhm.2021.102918
- Seol M, Kim Y. Hedonic consumption and consumer’s choice under the windfall gains. 한국산업정보학회논문지 [Journal of Korea Society of Industrial Information Systems]. 2022;27(2):83–100.
- Abbott M, Binde P, Clark L, et al. Conceptual framework of harmful gambling: an international collaboration revised September 2015. Gambling Research Exchange Ontario (GREO); 2015. Available from: https://prism.ucalgary.ca/items/3cd090fd-a0be-464a-83be-77b660e2e8b3. Accessed October 24, 2023.
- Arkes HR, Joyner CA, Pezzo MV, Nash JG, Siegel-Jacobs K, Stone E. The psychology of windfall gains. Organ Behav Hum Decis Process. 1994;59(3):331–347. doi:10.1006/obhd.1994.1063
- Antonides G, Ranyard R. Mental accounting and economic behaviour. Econ Psychol. 2017;3:123–138.
- Henderson PW, Peterson RA. Mental accounting and categorization. Organ Behav Hum Decis Process. 1992;51(1):92–117. doi:10.1016/0749-5978(92)90006-S
- Zhang CY, Sussman AB. Perspectives on mental accounting: an exploration of budgeting and investing. Financ Plan Rev. 2018;1:1–2.
- Magar EC, Phillips LH, Hosie JA. Self-regulation and risk-taking. Personal Individ Differ. 2008;45(2):153–159. doi:10.1016/j.paid.2008.03.014
- Sullivan M Cultural Anthropology: a Contemporary Perspective; 1981. Available from: https://www.jstor.org/stable/3317238. Accessed November 3, 2023.
- Shefrin HM, Thaler RH. Mental accounting, saving, and self-control. Adv Behav Econ. 2004;3:395–428.
- Thaler RH, Johnson EJ. Gambling with the house money and trying to break even: the effects of prior outcomes on risky choice. Manage Sci. 1990;36(6):643–660. doi:10.1287/mnsc.36.6.643
- Bailey NT, Ramalingam A, Stoddard BV. Experimental (re-) analysis of the house-money effect in a public goods game. J Econ Sci Assoc. 2023;9(1):1–14. doi:10.1007/s40881-022-00122-2
- Clark J. House money effects in public good experiments. Exp Econ. 2002;5(3):223–231. doi:10.1023/A:1020832203804
- Harrison GW. House money effects in public good experiments: comment. Exp Econ. 2007;10(4):429–437. doi:10.1007/s10683-006-9145-x
- Kahneman D, Tversky A. Prospect theory: an analysis of decision under risk. In: Handbook of the Fundamentals of Financial Decision Making: Part I. World Scientific; 2013:99–127.
- Lejuez CW, Read JP, Kahler CW, et al. Evaluation of a behavioral measure of risk taking: the Balloon Analogue Risk Task (BART). J Exp Psychol Appl. 2002;8(2):75. doi:10.1037/1076-898X.8.2.75
- Lasagna CA, Pleskac TJ, Burton CZ, McInnis MG, Taylor SF, Tso IF. Mathematical modeling of risk-taking in bipolar disorder: evidence of reduced behavioral consistency, with altered loss aversion specific to those with history of substance use disorder. Comput Psychiatry. 2022;6(1). doi:10.5334/cpsy.61
- Wallsten TS, Pleskac TJ, Lejuez CW. Modeling behavior in a clinically diagnostic sequential risk-taking task. Psychol Rev. 2005;112(4):862. doi:10.1037/0033-295X.112.4.862
- Zhou R, Myung JI, Pitt MA. The scaled target learning model: revisiting learning in the balloon analogue risk task. Cognit Psychol. 2021;128:101407. doi:10.1016/j.cogpsych.2021.101407
- Aklin WM, Lejuez CW, Zvolensky MJ, Kahler CW, Gwadz M. Evaluation of behavioral measures of risk taking propensity with inner city adolescents. Behav Res Ther. 2005;43(2):215–228. doi:10.1016/j.brat.2003.12.007
- Lejuez CW, Aklin WM, Jones HA, et al. The balloon analogue risk task (BART) differentiates smokers and nonsmokers. Exp Clin Psychopharmacol. 2003;11(1):26. doi:10.1037/1064-1297.11.1.26
- Pleskac TJ. Decision making and learning while taking sequential risks. J Exp Psychol Learn Mem Cogn. 2008;34(1):167. doi:10.1037/0278-7393.34.1.167
- Faul F, Erdfelder E, Lang AG, Buchner A. G* Power 3: a flexible statistical power analysis program for the social, behavioral, and biomedical sciences. Behav Res Methods. 2007;39(2):175–191. doi:10.3758/BF03193146
- Andrews B, Wilding JM. The relation of depression and anxiety to life‐stress and achievement in students. Br J Psychol. 2004;95(4):509–521. doi:10.1348/0007126042369802
- Oppenheimer DM, Meyvis T, Davidenko N. Instructional manipulation checks: detecting satisficing to increase statistical power. J Exp Soc Psychol. 2009;45(4):867–872. doi:10.1016/j.jesp.2009.03.009
- Beck AT, Steer RA, Brown GK Beck Depression Inventory. Harcourt Brace Jovanovich New York; 1987. Available from: http://www.med.upenn.edu/cbti/assets/user-content/documents/Beck%20Depression%20Inventory.pdf. Accessed January 25, 2024.
- Löwe B, Kroenke K, Herzog W, Gräfe K. Measuring depression outcome with a brief self-report instrument: sensitivity to change of the Patient Health Questionnaire (PHQ-9). J Affect Disord. 2004;81(1):61–66. doi:10.1016/S0165-0327(03)00198-8
- Costa PT, McCrae RR. The Revised Neo Personality Inventory (Neo-Pi-r). Sage Publications, Inc; 2008.
- Spielberger CD, Gonzalez-Reigosa F, Martinez-Urrutia A, Natalicio LF, Natalicio DS. The State-Trait Anxiety Inventory. Rev Interam Psicol J Psychol. 1971;5:3–4.
- Patton JH, Stanford MS, Barratt ES. Factor structure of the Barratt impulsiveness scale. J Clin Psychol. 1995;51(6):768–774. doi:10.1002/1097-4679(199511)51:6<768::AID-JCLP2270510607>3.0.CO;2-1
- Watson D, Clark LA, Tellegen A. Development and validation of brief measures of positive and negative affect: the PANAS scales. J Pers Soc Psychol. 1988;54(6):1063. doi:10.1037/0022-3514.54.6.1063
- Vehtari A, Gelman A, Gabry J. Practical Bayesian model evaluation using leave-one-out cross-validation and WAIC. Stat Comput. 2017;27:1413–1432. doi:10.1007/s11222-016-9696-4
- McElreath R. Statistical Rethinking: A Bayesian Course with Examples in R and Stan. Chapman and Hall/CRC; 2020.
- Lejuez CW, Aklin WM, Bornovalova MA, Moolchan ET. Differences in risk-taking propensity across inner-city adolescent ever-and never-smokers. Nicotine Tob Res. 2005;7(1):71–79. doi:10.1080/14622200412331328484
- Skeel RL, Pilarski C, Pytlak K, Neudecker J. Personality and performance-based measures in the prediction of alcohol use. Psychol Addict Behav. 2008;22(3):402. doi:10.1037/0893-164X.22.3.402
- Liu Q, Zhong R, Ji X, et al. Decision‐making biases in suicide attempters with major depressive disorder: a computational modeling study using the balloon analog risk task (BART). Depress Anxiety. 2022;39(12):845–857. doi:10.1002/da.23291
- Robles E, Emery NN, Vargas PA, et al. Patterns of responding on a balloon analogue task reveal individual differences in overall risk-taking: choice between guaranteed and uncertain cash. J Gen Psychol. 2014;141(3):207–227. doi:10.1080/00221309.2014.896781
- Xu S, Wang H, Wang C. Paying out one versus paying out all trials and the decrease in behavioral and brain activity in the Balloon Analogue Risk Task. Psychophysiology. 2020;57(3):e13510. doi:10.1111/psyp.13510
- Heilman CM, Nakamoto K, Rao AG. Pleasant surprises: consumer response to unexpected in-store coupons. J Mark Res. 2002;39(2):242–252. doi:10.1509/jmkr.39.2.242.19081
- Hsu YL, Chow EH. The house money effect on investment risk taking: evidence from Taiwan. Pac Basin Finance J. 2013;21(1):1102–1115. doi:10.1016/j.pacfin.2012.08.005
- Strahilevitz MA, Loewenstein G. The effect of ownership history on the valuation of objects. J Consum Res. 1998;25(3):276–289. doi:10.1086/209539
- Barkan R, Danziger S, Ben-Bashat G, Busemeyer JR. Framing reference points: the effect of integration and segregation on dynamic inconsistency. J Behav Decis Mak. 2005;18(3):213–226. doi:10.1002/bdm.496
- Lee J, Soberon-Ferrer H. Consumer vulnerability to fraud: influencing factors. J Consum Aff. 1997;31(1):70–89. doi:10.1111/j.1745-6606.1997.tb00827.x