Abstract
Purpose
Psychosocial determinants influence healthcare workers’ compliance with surgical site infection (SSI) preventive interventions. In order to design needs-based interventions promoting compliance, such determinants must first be assessed using valid and reliable questionnaire scales. To compare professional groups without bias, the scales must also be measurement-equivalent. We examine the validity/reliability and measurement equivalence of four scales using data from physicians and nurses from outside the university sector. Additionally, we explore associations with self-reported SSI preventive compliance.
Participants and Methods
N = 90 physicians and N = 193 nurses (response rate: 31.5%) from nine general/visceral or orthopedic/trauma surgery departments in six non-university hospitals in Germany participated. A written questionnaire was used to assess the compliance with SSI preventive interventions and the determinants of compliance based on the Capability-Opportunity-Motivation-Behavior-Model. Psychometric testing involved single- and multiple-group confirmatory factor analyses, and explorative analyses used t-tests and multiple linear regression.
Results
The scales assessing individual determinants of compliance (capability, motivation, and planning) were found to be reliable (each Cronbach’s α ≥ 0.85) and valid (each Root-Mean-Square-Error of Approximation ≤ 0.065, each Comparative-Fit-Index = 0.95) and revealed measurement equivalence for physicians and nurses. The scale assessing external determinants (opportunity) did not demonstrate validity, reliability, or measurement equivalence. Group differences were found neither in compliance (p = 0.627) nor determinants (p = 0.192; p = 0.866; p = 0.964). Capability (β = 0.301) and planning (β = 0.201) showed associations with compliance for nurses only.
Conclusion
The scales assessing motivation, capability, and planning regarding SSI preventive compliance provided reliable and valid scores for physicians and nurses in surgery. Measurement equivalence allows group comparisons of scale means to be interpreted without bias.
Introduction
Healthcare worker (HCW) non-compliance remains a key barrier in the successful implementation of evidence-based infection prevention and control (IPC) interventions. For example, although hand hygiene compliance (HHC) is consistently higher among nurses than physicians, it is still suboptimal.Citation1–3 Thus, attempts were made to understand possible reasons for (non-)compliance using behavioral science approaches, among others.Citation4,Citation5 One promising strategy to increase the effectiveness of interventions, such as training or workflow modifications, is to tailor implementation to HCWs and their working environment.Citation6,Citation7 Therefore, psychosocial determinants that influence compliance should be identified to inform design of interventions.
For instance, two studiesCitation8,Citation9 have developed questionnaire items to measure such determinants on HCWs’ HHC using the Capability-Opportunity-Motivation-Behavior (COM-B) model.Citation10 According to this model, there are three constructs that influence behavior. First, “capability” denotes physical and psychological capacities, such as knowledge and skills, to engage in and execute a given behavior or activity.Citation10 Regarding surgical site infection (SSI) prevention, examples are to know how to correctly wear a surgical mask and to be able to implement surgical hand disinfection according to guidelines. Second, “opportunity” points to social and physical environmental factors, which may represent facilitators or barriers to given behaviors or activities.Citation10 SSI prevention-related examples are the support by leaders and superiors, and the availability of, or lack thereof, hand sanitizer dispensers. Third there is “motivation”, ie, reflective and automatic processes such as analytical decision-making and goal setting or emotional and habitual responses that energize and direct given behaviors or activities.Citation10 In the present context, examples are setting goals to correctly disinfecting one’s hands before every aseptic procedure and being socially acknowledged for compliant aseptic dressing change.
Both studies used pooled data from physicians and nurses to assess the psychometric properties of the newly developed questionnaires. A scale score is considered reliable if it is as unbiased as possible, meaning, if measurement errors are as small as possible. Usually Cronbach’s α is used as an indicator of reliability. Validity, defined as whether the items really measure the underlying, non-measurable constructs, is usually examined by confirmatory factor analysis (CFA). Validity is observed, for instance, if highly motivated HCWs do display high mean scores on the “motivation” scale.
However, CFA results of pooled data from different subgroups, such as physicians and nurses, do not reveal whether these groups understand questionnaire items in an equivalent manner. As an extension to validity testing, measurement equivalence (ME; synonym: measurement invariance) ensures that item comprehension is comparable across subgroups and that underlying constructs are interpreted the same in each subgroup.Citation11 For instance, if there is ME, a nurse and a physician with the same level of motivation would respond similarly to items measuring “motivation”. Thus, ME strictly speaking is a prerequisite for ensuring that comparisons of means are unbiased and reflect reality.Citation12,Citation13 ME is usually tested by multiple-group confirmatory factor analysis (MGCFA), an extension of CFA.Citation14
To explore the ME of physicians and nurses in COM factors, this study follows up on a previous study in which the COM-B model was applied to compliance with surgical site infection (SSI) preventive interventions of orthopedic physicians in a tertiary care university clinic.Citation15 In this previous study, exploratory factor analysis of 12 items on individual COM determinants produced three scales covering the constructs of “capability”, “motivation”, and “planning”. Another exploratory factor analysis of six items on environmental determinants resulted in one scale, “opportunity”.Citation15 In the present analysis, we determine the validity, reliability, and ME of these scalesCitation15 using data from physicians and nurses from outside the university sector. Thus, we go beyond the former studyCitation15 by testing the scales not only among physicians, but nurses as well, conducting confirmatory, not only exploratory analyses, and using a new sample approximately five times as large. Additionally, we explore associations between valid, reliable, and cross-group equivalent scale scores and self-reported SSI prevention compliance.
Materials and Methods
From March to October 2019, a cross-sectional survey was conducted as part of the baseline data collection of the WACH trial (“Wundinfektionen und Antibiotikaverbrauch in der Chirurgie” [“Wound Infections and Antibiotic Use in Surgery”]) using a self-administered written questionnaire.Citation16 The WACH study used the behavioral approach of a previous university hospital studyCitation17 to promote compliance with SSI preventive intervention measures in six non-university hospitals.Citation16 The study was approved by the Ethics Committee of the Medical Faculty of the University of Leipzig (vote no. 034/18-ek) and the ethics committees of the medical associations of the participating federal states. The baseline survey was approved by the staff councils of all participating hospitals.
The IPC team at each hospital distributed the questionnaire to physicians and nurses working in either the general/visceral surgery, orthopedic/trauma surgery, or anesthesia department. The two surgical specialties were selected on the basis of epidemiological considerations, ie, prevalence in Germany.Citation18 Questionnaires were anonymous, ie, included no unique identifier, were accompanied by a data privacy statement which included all necessary assurances, and were returned via devices such as boxes placed across departments in which they could be dropped. Reminders were verbally communicated to clinicians by the IPC team for as often as they judged it appropriate. The duration of data collection ranged from 14 to 97 days depending on the size of the hospital, the number of participating departments, and the work capacity of the IPC team. Informed consent was obtained from all participants.
The questionnaire included 94 items addressing compliance with 26 clinical SSI preventive interventions, COM determinants, socio-demographics, and awareness of in-house implementation strategies (not reported here). The COM determinants were surveyed using 18 items on a 7-point-Likert-scale (“strongly disagree“ to “strongly agree”). In accordance with the previous exploratory factor analysis (EFA),Citation15 the number of items measuring ‘capability’, “opportunity”, “motivation”, and “planning” were six, six, four, and two, respectively (for items, see Table S1). The SSI preventive intervention measures for which self-reported compliance was assessed were selected in accordance with national recommendations (see Table S2). For each of the interventions, participants were asked to indicate in what percentage of the opportunities in which an intervention is indicated they actually performed the intervention compliantly. Participants could select ”not applicable” if they felt an intervention was outside their area of responsibility. The overall compliance rate for all interventions combined was calculated as in:Citation15 summing the compliance rate for each intervention divided by the number of interventions for which responsibility was indicated.
Participants indicated their gender, age, and specialty. Based on the latter including such information, participants were categorized as physician or nurse. Age was recorded in categories (<18, 18–30, 31–40, 41–50, 51–60, >60 years) to comply with data protection regulations.
IBM SPSS Statistics v27 was used for t-tests and regressions, and R’s lavaan-package v0.6–12 for CFA and MGCFA. To account for missing data and multivariate normality assumptions, a robust full-information-maximum-likelihood estimate was used.Citation19,Citation20 Two models were tested based on the earlier EFA:Citation15 one with the three individual determinants “capability”, “motivation”, and “planning”, and one with the environmental determinants (“opportunity”).
CFA was used to test for construct validity with a good model fit determined by Root-Mean-Square-Error of Approximation (RMSEA) ≤ 0.08,Citation21 Standardized Root Mean Square Residual (SRMR) ≤ 0.08,Citation22 and Comparative-Fit-Index (CFI) ≥ 0.9.Citation23 The reliability of the scales was tested using Cronbach’s α, with values > 0.7 being considered acceptable.Citation20
In case of satisfactory model fit in CFA, MGCFA was used to test for ME across physicians and nurses. To perform MGCFA, the assumption of sufficient model fit must be met in both groups, therefore stratified CFAs were performed beforehand.Citation12
ME is not dichotomous but has three hierarchical levels.Citation11,Citation12 During the steps of MGCFA, the model is increasingly constrained, ie forced to be equal across both groups.Citation12 Configural equivalence is the lowest level and achieved when the model fit is good despite the number of constructs and the pattern of items loading on constructs being the same in both groups.Citation12 The next higher levels, metric and scalar equivalence, are confirmed if the respective model fit does not decrease compared with the respective less constrained model, with ∆CFI < 0.01 as cut-off.Citation11,Citation12 For testing metric equivalence, factor loadings, that is the correlation between item and construct, are constrained, implying that the constructs’ relationships within the model can be compared across groups.Citation11,Citation12 For testing scalar equivalence, item intercepts are additionally constrained, implying that cross-group comparisons of constructs’ means are possible.Citation11,Citation12
Based on results of CFA and MGCFA, further explorative analyses were conducted. To compare physicians and nurses, t-tests were performed for the overall compliance rate for all interventions, COM scales with ME, and the number of SSI preventive interventions participants considered to be responsible for (“responsibility”). Multiple hierarchical linear regressions were performed to examine associations between COM scales with ME and overall compliance in order to provide first indications of their criterion-related validity. In the first regression model in each case, ie, for physicians and for nurses, the variables “responsibility” (as the number of SSI preventive interventions which participants had rated to fall into their field of responsibility) and participants’ specialty (surgery vs anesthesiology) were entered as confounders. The scale “motivation” was added in the second model, followed by “capability” in the third model, respectively. As an additional factor that had emerged in the earlier study,Citation15 “planning” was added in the final model.
Results
N = 336 of 1,068 HCWs participated (response rate: 31.5%; broken down by hospitals in ascending order: 19.9%, 23.1%, 41.5%, 41.8%, 49.6%, and 52.7%). For N = 18 participants the information on specialty was missing, implying response rates of 28.4% for physicians (by hospital: 17.9%, 27.7%, 41.0%, 26.9%, 20.8%, and 66.7%) and 30.4% for nurses (17.9%, 20.3%, 35.8%, 40.0%, 65.8%, and 47.2%). Furthermore, N = 3 physicians and N = 32 nurses were excluded because they answered less than 20% of the COM items. The analysis sample consisted of N = 90 physicians and N = 193 nurses (). More than a three-quarters majority of nurses were women, while among physicians, they did not represent a majority. Younger age groups (30 year or less) were more strongly represented among nurses than physicians. Surgical specialists among physicians and ward nurses represented the majority in their respective professional group. Finally, most respondents worked at a orthopedics/trauma surgery department, both overall and among professional groups.
Table 1 Sample Description by Gender, Age, Specialty and Department
In line with the confirmatory approach pursued in the present study, only the one-factor model of environmental determinants and the three-factor model of individual determinants were tested. CFA revealed insufficient model fit (Χ² = 86.6, df = 9, p < 0.001, RMSEA = 0.175, SRMR = 0.067, CFI = 0.83), precluding consideration of this scale in further analysis. For the three-factor model of individual determinants, CFA revealed an excellent model fit (Χ² = 111.3, df = 51, p < 0.001, RMSEA = 0.065, SRMR = 0.039, CFI = 0.95), with reliability for “capability” (Cronbach’s α = 0.90), “motivation” (α = 0.85), and “planning” (α = 0.89; see ). Criteria for MGCFA were met, thus ME testing was performed. MGCFA revealed both metric and scalar equivalence, as the decline in model fit was tolerable (∆CFI = −.006 and ∆CFI = 0.005, respectively) ().
Table 2 CFA and MGCFA Results for Measurement Equivalence of the Scale “Opportunity”, and of the Scales “Capability”, “Motivation”, and “Planning” (N = 283)
Figure 1 Standardized solution for confirmatory factor analysis on three-factor model of individual determinants of compliance with surgical site infection prevention (N=283).
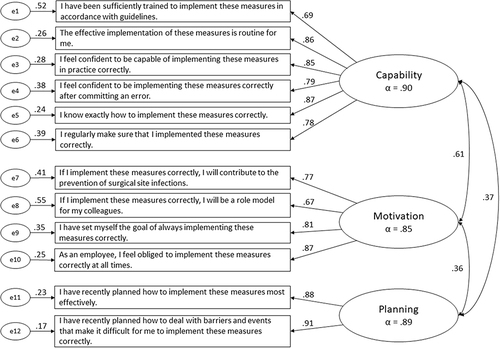
Physicians and nurses did not differ in self-reported compliance or individual determinants (capability, motivation, and planning), but physicians reported being responsible for a significantly higher number of interventions (p < 0.001) ().
Table 3 Results of T-tests for Comparing Physicians and Nurses in Regard to Overall Self-Reported Surgical Site Infection Preventive Compliance (in %), Capability, Motivation, and Planning (Scales of 1–7, Respectively), and the Number of Preventive Interventions for Which Responsibility Was Stated (Maximum Possible Score: 26)
On linear regression (), for physicians, the final model explained 6% of the variance in self-reported compliance (F(5;79) = 2.08, p = 0.077). Being a surgeon (vs being an anesthetist) was the regressor with the highest standardized coefficient (β = 0.196), ie, surgeons reported higher compliance than anesthetists. However, as for all others, ie, the numerically positive associations of motivation, capability, and planning, this effect was not statistically significant (p = 0.123).
Table 4 Results of Hierarchical Multiple Linear Regressions for Physicians and Nurses: Self-Reported Overall Surgical Site Infection Preventive Compliance by Surgical vs Anesthesiological Background, Number of Preventive Interventions Self-Reportedly Being Responsible for, and Individual Determinants of Compliance (Capability, Motivation, and Planning)
For nurses, the first model (“specialty” and “responsibility”) explained 10.2% of the variance. The final model explained 25.4% of the variance (F(5;164)=12.52, p < 0.001) and “capability” (β = 0.301, p = <0.001) and “planning” (β = 0.201, p = 0.006) showed the strongest associations. That is, both of these COM-B factors were positively linked to self-reported SSI preventive compliance.
Discussion
The scales “motivation”, “capability”, and “planning”, which were created to assess the individual determinants of compliance with SSI preventive interventions,Citation15 proved to be valid and reliable for both physicians and nurses. Neither validity nor reliability could be demonstrated for the scale to assess the environmental determinants. Due to the sample recruitment in six hospitals, the data have a hierarchical structure. Presumably, the assessment of the environmental determinants was influenced more by hospital-specific characteristics than that of the individual determinants. This could have led to the participants’ responses to the environmental determinants being more inconsistent across all hospitals. However, due to the sample size, the CFA could not be conducted in subgroups per hospital. As previous studies have emphasized the importance of environmental determinants of IPC compliance,Citation24–27 developing valid and reliable measures of environmental determinants remains an important task.
Whereas in original COM-B terminology, planning represents a facet of motivation,Citation10 CFA results replicated “planning” as an independent construct.Citation15 The contents of these two items, i.e, action and coping planning, represent cognitions oriented toward the implementation of behavior, and seem to stand out as a specific determinant of (at least self-reported) behavior, especially given the large number of SSI preventive interventions. In addition, the mean values for “planning“ for physicians and nurses were by far the lowest of all the scales (see and S1). It appears that HCWs have high intentions to comply with SSI prevention guidelines but do not adequately plan how to translate this into behavior. To help HCWs overcome this intention-behavior gap,Citation28,Citation29 tailored implementation strategies may specifically address action and coping planning to promote compliance. In general, we emphasize that this result should not be considered as an extension of the COM-B model, even though the labels “motivation” and ”capability” have been retained for the other factors representing individual determinants.
Unlike previous studies,Citation24–27,Citation30 physicians and nurses in this sample did not differ in psychosocial determinants of compliance. By identifying ME between physicians and nurses, comparisons of scale means between these groups are unbiased and can be meaningfully interpreted. This raises the question of whether the differences found in previous studies are spurious.Citation31 In other words, if questionnaires with no or inadequate ME have been used, any differences found in the determinants of compliance may be due to different understandings of the items and may not reflect true differences in the underlying construct. On the other hand, existing differences may not be found due to a lack of, or insufficient, ME. This supports the call for greater attention to issues of construct validity in IPC research,Citation32 not only in measuring compliance but also in measuring the determinants of compliance.
Although physicians and nurses did not differ in self-reported compliance rate, differences were found in the regressions modeling self-reported compliance. For physicians, the explained variance of compliance was <10% and no regressor showed a significant relationship. In contrast, for nurses, the final model explained over 25% of the variance, and “planning“ and “capability” showed a significant association. Considering that self-reported compliance was similar in both groups and that observed compliance is consistently lower among physicians,Citation1–3,Citation33 these differences in determinants predicting compliance can be interpreted in three different ways. First, the determinants may be less predictive of physicians’ self-reported compliance because their overestimation is greater than that of nurses,Citation33 and thus these self-reports do not reflect compliance but rather overestimation. Second, the individual facets of the COM-B model may simply be not that relevant to physicians’ compliance, at least when measured with the scales used here. This would be consistent with the limited success of previous research in promoting physician IPC compliance,Citation1–3,Citation7,Citation17 and raising the question of which factors are relevant to physicians. Third, there is the possibility that things are more chaotic than theory suggests, that is highly context-specific and/or dependent on unknown factors. This would be supported by previous regression results for physicians in a university orthopedic hospitalCitation15 being similar to those for nurses in this non-university sample. The “chaos” might highlight the need to tailor any intervention to promote compliance to the situation “on the ground” in an even more careful way. This could be demonstrated by studies linking data on psychosocial determinants to observed compliance at the individual level of each HCW. However, such designs face ethical and data protection barriers.
In terms of practice, this questionnaire may be used as a survey tool to provide data that can guide healthcare managers and practitioners in tailoring interventions to promote implementation, and thus in how to optimize, SSI preventive compliance.
This study has several strengths, including being confirmatory not only by its statistical approach but also by replicating previous analysesCitation15 and, to our knowledge, being the first study examining the ME of psychosocial determinants of compliance with IPC interventions.
Nevertheless, the study has some limitations. To begin with, the survey response rate in the present study was just above 30%, which was lower than the global average of 53% among surgical physicians reported in an overview paper on survey response rates in surgery.Citation34 This led to a nearly undersized sample for the MGCFA among physicians. Probably questionnaire length, as well as the lack of tangible incentives and questionnaire personalization,Citation35 affected the response rate. The earlier study achieved a response rate of 73% among surgical physicians, likely because healthcare professionals in a university hospital demonstrate a higher affinity for research and the study received exceptional support from the medical director.Citation15 While efforts should be made to increase response rates, there is a declining trend in response rates among surgical physicians,Citation35 and the current rate is at least within one standard deviation of the 2019 mean.Citation35
Second, the 18 COM items represent only a sample of facets of all compliance determinants. For example, the Theoretical Domains Framework, the most integrative and granular classification of theoretical constructs relevant to behavior change, maps 84 constructs into 14 domains.Citation36 Nevertheless, all of these 84 constructs can be mapped onto the COM components.Citation36 The present items therefore reflect the need for a concise survey instrument. The selection of the most relevant facets was based on the best available evidence and the expertise of the transdisciplinary WACH study group.
Third, the items used to collect the determinants referred to all preventive interventions within the participant’s area of responsibility. It could be argued that this may bias the relationship between the determinants and the overall compliance score. Models such as the COM-B model were developed to identify determinants of individual behaviors in order to design behavior change interventions. However, different determinants may be important for different behaviors.Citation10 While this argument certainly holds validity, a key trend in SSI prevention has been the use of bundles,Citation37–39 ie, sets of “…evidence-based interventions that, when implemented together, will result in significantly better outcomes than when implemented individually”.Citation40, Compliance is therefore increasingly defined and measured on the basis of more than one intervention. In this sense, the operationalization of SSI prevention compliance used in this study may be seen as a kind of “subjective bundle” approach. However, we acknowledge that such bundles may need unbundling to describe specific interventions to promote compliance.
Fourth, no specific direction of causality could be established because of the cross-sectional design of this observational study. Thus, the use of the scales in longitudinal studies is necessary to assess their causal structure and sensitivity to change.
Fifth, we did not contrast women vs men in our analyses, simply because it is not these groups that define target groups for interventions to promote implementation of SSI prevention, but physicians and nurses are. Additionally, both dimensions are correlated, which would have left N = 33 male nurses for any gender-sensitive analysis. Studies with even larger samples are needed to disentangle any differences or similarities between same-sex physicians and nurses.
Finally, the data were collected before the COVID-19 pandemic. Physicians and nurses may now differ in self-reported compliance and COM factors. However, the results of CFA and MGCFA, ie, the evidence base for the validity, reliability, and ME of the scales, should be robust because these statistical analyses are based on the structure of the item responses, not the item scores themselves.
Conclusion
In summary, this study provides scales to assess capability, motivation, and action and coping planning as individual determinants of compliance with SSI preventive interventions. As scales with proven ME among physicians and nurses, they allow a valid interpretation of group comparisons, at least for the original German version. Thus, they contribute to the understanding of how and why healthcare professions (do not) differ in IPC compliance. In conclusion, the study contributes to physicians’ IPC compliance as a current key topic in this areaCitation7 and meets the demand for “…easy-to-use, valid tools to provide data on the factors that influence the…behavior of healthcare professionals”.Citation8
Abbreviations
COM-B, Capability-Opportunity-Motivation-Behavior Model; CFI, Comparative-Fit-Index; CFA, confirmatory factor analysis; EFA, exploratory factor analysis; HCW, healthcare workers; HH, hand hygiene; IPC, infection prevention and control; ME, measurement equivalence; MGCFA, multiple group confirmatory factor analysis; RMSEA, Root-Mean-Square-Error of Approximation; SD, standard deviation; SRMR, Standardized Root Mean Square Residual; SSI, surgical site infection; WACH, Wundinfektionen und Antibiotikaverbrauch in der Chirurgie (Surgical Site Infections and Antibiotic Use in Surgery) = acronym and name of the study.
Author Contributions
All authors made a significant contribution to the work reported, whether that is in the conception, study design, execution, acquisition of data, analysis and interpretation, or in all these areas; took part in drafting, revising, or critically reviewing the article; gave final approval of the version to be published; have agreed on the journal to which the article has been submitted; and agree to be accountable for all aspects of the work.
Disclosure
The authors declare no conflicts of interest regarding this work.
Acknowledgments
Preliminary results were presented as a poster on September 6, 2022, at the 74th Annual Meeting of the German Society for Hygiene and Microbiology in Berlin, Germany, and on September 13, 2023, at the 7th International Conference on Prevention & Infection Control (ICPIC) in Geneva, Switzerland. A previous version of this paper has been uploaded to Research Square as a preprint: https://www.researchsquare.com/article/rs-3199535/v2.
Data Sharing Statement
The data is available from the corresponding author upon reasonable request.
Additional information
Funding
References
- Erasmus V, Daha TJ, Brug H, et al. Systematic review of studies on compliance with hand hygiene guidelines in hospital care. Infect Control Hosp Epidemiol. 2010;31(3):283–294. doi:10.1086/650451
- Lambe KA, Lydon S, Madden C, et al. Hand hygiene compliance in the ICU: a systematic review. Crit Care Med. 2019;47(9):1251–1257. doi:10.1097/CCM.0000000000003868
- Bredin D, O’Doherty D, Hannigan A, et al. Hand hygiene compliance by direct observation in physicians and nurses: a systematic review and meta-analysis. J Hosp Infect. 2022;130:20–33. doi:10.1016/j.jhin.2022.08.013
- Edwards R, Charani E, Sevdalis N, et al. Optimisation of infection prevention and control in acute health care by use of behaviour change: a systematic review. Lancet Infect Dis. 2012;12(4):318–329. doi:10.1016/S1473-3099(11)70283-3
- Srigley JA, Corace K, Hargadon DP, et al. Applying psychological frameworks of behaviour change to improve healthcare worker hand hygiene: a systematic review. J Hosp Infect. 2015;91(3):202–210. doi:10.1016/j.jhin.2015.06.019
- Baker R, Camosso-Stefinovic J, Gillies C, et al. Tailored interventions to address determinants of practice. Cochrane Database Syst Rev. 2015;2015(4):CD005470. doi:10.1002/14651858.CD005470.pub3
- Lotfinejad N, Peters A, Tartari E, et al. Hand hygiene in health care: 20 years of ongoing advances and perspectives. Lancet Infect Dis. 2021;21(8):e209–21. doi:10.1016/S1473-3099(21)00383-2
- Lydon S, Greally C, Tujjar O, et al. Psychometric evaluation of a measure of factors influencing hand hygiene behaviour to inform intervention. J Hosp Infect. 2019;102(4):407–412. doi:10.1016/j.jhin.2019.02.003
- Zheng S, Yang Q, Wang X, et al. Capability, opportunity, motivation, and hand hygiene behavior in healthcare workers: a structural equation modeling. Psychol Res Behav Manag. 2022;15:2219–2228. doi:10.2147/PRBM.S373287
- Michie S, van Stralen MM, West R. The behaviour change wheel: a new method for characterising and designing behaviour change interventions. Implement Sci. 2011;6:42. doi:10.1186/1748-5908-6-42
- Steenkamp J-EM, Baumgartner H. Assessing measurement invariance in cross-national consumer research. J Consum Res. 1998;25(1):78–90. doi:10.1086/209528
- Somaraju AV, Nye CD, Olenick J. A review of measurement equivalence in organizational research: what’s old, what’s new, what’s next? Organ Res Methods. 2022;25(4):741–785. doi:10.1177/10944281211056524
- Vandenberg RJ, Lance CE. A review and synthesis of the measurement invariance literature: suggestions, practices, and recommendations for organizational research. Organ Res Methods. 2000;3(1):4–70. doi:10.1177/109442810031002
- Gana K, Broc G. Structural Equation Modeling with Lavaan. London: Wiley; 2019.
- Tomsic I, Ebadi E, Gossé F, et al. Determinants of orthopedic physicians’ self-reported compliance with surgical site infection prevention: results of the WACH-trial’s pilot survey on COM-B factors in a German university hospital. Antimicrob Resist Infect Control. 2021;10:67. doi:10.1186/s13756-021-00932-9
- von Lengerke T, Hartlep I, Schipper P, et al. SSI-preventive compliance after tailored interventions for infection prevention and control teams: results of the cluster-randomized controlled WACH-trial in six non-university centres. Antimicrob Resist Infect Control. 2021;10(Suppl 1):130.
- von Lengerke T, Ebadi E, Schock B, et al. Impact of psychologically tailored hand hygiene interventions on nosocomial infections with multidrug-resistant organisms: results of the cluster-randomized controlled trial PSYGIENE. Antimicrob Resist Infect Control. 2019;8:56. doi:10.1186/s13756-019-0507-5
- Postoperativer Wundinfektionen P. Empfehlung der Kommission für Krankenhaushygiene und Infektionsprävention (KRINKO) beim Robert Koch-Institut [Commission for Hospital Hygiene and Infection Prevention (KRINKO) at the Robert Koch Institute]. Bundesgesundheit Gesundheitsf Gesundheits. 2018;61(4):448–473. German. doi:10.1007/s00103-018-2706-2
- Rosseel Y. Lavaan: an R package for structural equation modeling. J Stat Softw. 2012;48(2):1–36. doi:10.18637/jss.v048.i02
- Hair JF, Black WC, Babin BJ, et al. Multivariate Data Analysis. 7th. Upper Saddle River, NJ: Pearson Prentice Hall; 2014.
- Browne MW, Cudeck R. Alternative ways of assessing model fit. In: Bollen KA, Long JS, editors. Testing Structural Equation Models. Newbury Park, CA: Sage; 1993:136–162.
- Hu LT, Bentler PM. Cutoff criteria for fit indexes in covariance structure analysis: conventional criteria versus new alternatives. Struct Equation Model. 1999;6(1):1–55. doi:10.1080/10705519909540118
- Bentler PM. Comparative fit indexes in structural models. Psychol Bull. 1990;107(2):238–246. doi:10.1037/0033-2909.107.2.238
- Shah N, Castro-Sánchez E, Charani E, et al. Towards changing healthcare workers’ behaviour: a qualitative study exploring non-compliance through appraisals of infection prevention and control practices. J Hosp Infect. 2015;90(2):126–134. doi:10.1016/j.jhin.2015.01.023
- von Lengerke T, Lutze B, Graf K, et al. Psychosocial determinants of self-reported hand hygiene behaviour: a survey comparing physicians and nurses in intensive care units. J Hosp Infect. 2015;91(1):59–67. doi:10.1016/j.jhin.2015.04.018
- Ibrahim MAB, Chow C, Poh BF, et al. Differences in psychosocial determinants of hand hygiene between health care professional groups: insights from a mixed-methods analysis. Am J Infect Control. 2018;46(3):253–260. doi:10.1016/j.ajic.2017.09.014
- Gilbert GL, Kerridge I. The politics and ethics of hospital infection prevention and control: a qualitative case study of senior clinicians’ perceptions of professional and cultural factors that influence doctors’ attitudes and practices in a large Australian hospital. BMC Health Serv Res. 2019;19(1):212. doi:10.1186/s12913-019-4044-y
- Sheeran P, Webb TL. The intention-behavior gap. Soc Pers Psychol Compass. 2016;10:503–518. doi:10.1111/spc3.12265
- Zhang C, Zhang R, Schwarzer R, et al. A meta-analysis of the health Action process approach. Health Psychol. 2019;38(7):623–637. doi:10.1037/hea0000728
- Lutze B, Chaberny IF, Graf K, et al. Intensive care physicians’ and nurses’ perception that hand hygiene prevents pathogen transmission: belief strength and associations with other cognitive factors. J Health Psychol. 2017;22(1):89–100. doi:10.1177/1359105315595123
- Millsap RE. Statistical Approaches to Measurement Invariance. New York: Routledge; 2011.
- Neo JRJ. Construct validity—Current issues and recommendations for future hand hygiene research. Am J Infect Control. 2017;45(5):521–527. doi:10.1016/j.ajic.2017.01.028
- Lamping J, Tomsic I, Stolz M, et al. Do task and item difficulty affect overestimation of one’s hand hygiene compliance? A cross sectional survey of physicians and nurses in surgical clinics of six hospitals in Germany. Antimicrob Resist Infect Control. 2022;11:147. doi:10.1186/s13756-022-01188-7
- Meyer VM, Benjamens S, El Moumni M, et al. Global overview of response rates in patient and health care professional surveys in surgery. Ann Surg. 2022;275(1):75. doi:10.1097/SLA.0000000000004078
- Edwards PJ, Roberts I, Clarke MJ, et al. Methods to increase response to postal and electronic questionnaires. Cochrane Database Syst Rev. 2023;2023:MR000008. doi:10.1002/14651858.MR000008.pub5
- Atkins L, Francis J, Islam R, et al. A guide to using the theoretical domains framework of behaviour change to investigate implementation problems. Implement Sci. 2017;12(1):77. doi:10.1186/s13012-017-0605-9
- Wolfhagen N, Boldingh QJJ, Boermeester MA, et al. Perioperative care bundles for the prevention of surgical-site infections: meta-analysis. Br J Surg. 2022;109(10):933–942. doi:10.1093/bjs/znac196
- Avsar P, Patton D, Sayeh A, et al. The impact of care bundles on the incidence of surgical site infections: a systematic review. Adv Skin Wound Care. 2022;35(7):386–393. doi:10.1097/01.ASW.0000831080.51977.0b
- Zywot A, Lau CSM, Stephen Fletcher H, et al. Bundles prevent surgical site infections after colorectal surgery: meta-analysis and systematic review. J Gastrointest Surg. 2017;21(11):1915–1930. doi:10.1007/s11605-017-3465-3
- Resar R, Griffin FA, Haraden C, et al. Using care bundles to improve health care quality. IHI Innovation Series white paper. Cambridge, Massachusetts: Institute for Healthcare Improvement; 2012. Available from: https://www.ihi.org/education/IHIOpenSchool/Courses/Documents/CourseraDocuments/01_IHIUsingCareBundlesWhitePaper2012.pdf. Accessed July 24, 2023.