Abstract
Purpose
Burden of comorbidities appears to be related to clinical outcomes in hospitalized patients. Clinical stratification of admitted patients could be obtained calculating a comorbidity score, which represents the simplest way to identify the severity of patients’ clinical conditions and a practical approach to assess prevalent comorbidities. Our aim was to validate a modified Elixhauser score for predicting in-hospital mortality (IHM) in internal medicine admissions and to compare it with a different one derived from clinical data previously used in a similar setting, having a good prognostic accuracy.
Patients and Methods
A single-center retrospective study enrolled all patients admitted to internal medicine department between January and June 2016. A modified Elixhauser score was calculated from chart review and administrative data; moreover, a second prognostic index was calculated from chart review only. Comorbidity scores were compared using c-statistic.
Results
We analyzed 1614 individuals without selecting the reason for admission, 224 (13.9%) died during hospital stay. Deceased subjects were older (83.3±9.1 vs 78.4±13.5 years; p<0.001) and had higher burden of comorbidities. The modified Elixhauser score calculated by administrative data and by chart review and the comparator one was 18.13±9.36 vs 24.43±11.27 vs 7.63±3.3, respectively, and the c-statistic was 0.758 (95% CI 0.727–0.790), 0.811 (95% CI 0.782–0.840) and 0.740 (95% CI 0.709–0.771), respectively.
Conclusion
The new modified Elixhauser score showed a similar performance to a previous clinical prognostic index when it was calculated using administrative data; however, its performance improved if calculation was based on chart review.
Introduction
Prevalence of people living with multimorbidity is increasing,Citation1 especially in high-income countries during the last years.Citation2 Multimorbidity is defined as the detection of two or more chronic conditions in a single individual,Citation3 and individuals with combinations of different conditions require a comprehensive management approach.Citation4 Although multimorbidity increases risk of deathCitation5,Citation6 and both number and different combinations of conditions have been reported to rise mortality,Citation6–Citation8 its definition is still a matter of debate.Citation9,Citation10 Despite these evidences, health-care systems are organized for managing single diseases rather than their combinations because of their focus on specialization.Citation11 In the last decade, our research group published a series of papers using administrative databases in order to demonstrate the relationship between in-hospital mortality (IHM) and comorbidity in different conditions such as myocardial infarction, pulmonary embolism, stroke, urinary tract infections, renal transplantation, chronic obstructive pulmonary disease and acute esophageal variceal bleeding.Citation12–Citation19 Subsequently, we decided to modify an existing score in order to adapt it to internal medicine patients, and, in 2017, we obtained a new index of comorbidity based on internal medicine International Classification of Diseases codes and derived from Elixhauser index,Citation20 to predict risk for IHM in patients admitted to internal medicine wards.Citation21
In order to obtain information on comorbidity health care professionals could use medical chart reviews or administrative data. The first method is time consuming and laborious, whilst analysis of administrative databases is fast and cheap. On the other hand, the number of comorbidities identified with administrative databases is lower than those detected by chart review. Aim of this study was to validate this score comparing results derived from chart review and administrative data in a consecutive cohort of individuals admitted to internal medicine. Moreover, a prognostic index calculated from clinical data previously tested in internal medicine patients was used as a comparator score.
Patients and Methods
Population and Administrative Data Source
This retrospective study was conducted in agreement with the declaration of Helsinki stated in 1975, and revised in 2013, and it was approved by the local institutional committees for human research (Comitato Etico Azienda Ospedaliera Universitaria di Ferrara, approvazione 100,893).
The study included all hospital admissions for different causes along a 6-month period from January to June 2016, recorded in the database of the University Hospital St. Anna of Ferrara, Region Emilia-Romagna (RER) of Italy, and maintained by the Centre for Health Statistics. Since 1999, our hospital started to use an electronic database to store all the discharge hospital records (DHR) of hospitalized patient. These DHR report, gender, date of birth, date and department of hospital admission and discharge, vital status at discharge, length of stay, charge details, main and up to 6 accessory discharge diagnoses, and the most important diagnostic procedures, based on the International Classification of Diseases, 9th Revision, Clinical Modification (ICD-9-CM). Moreover, authors evaluated all medical charts and recorded clinical data and comorbidity after patients’ discharge. In agreement with national dispositions by law in terms of privacy, the health authorities removed patient names, exact addresses, and other potential identifiers from the database provided for this study.
The St. Anna University Hospital is a 660-bed teaching hospital with all facilities, excluding only cardiothoracic surgery. The majority of admissions are from the province of Ferrara, they are approximately 27,000 yearly, and the hospital is the hub center of the area. The province of Ferrara (≈ 350,000 inhabitants, mean age 47.3 years, 25.9% >65 years) is served by one Teaching Hospital (hub center) and three community hospitals (spoke centers about 200 beds/each). Local economy is mainly agriculture-based, and in minor part industrial. The annual flow of patients by the emergency department (ED) is approximately 90,000, mainly elderly subjects due to the fact that the area is characterized by a high percentage of elderly subjects, and approximately 3000 subjects are aged more than 90 years. The Department of Medicine consists of four Internal Medicine units, two Infectious Disease units, and one each of Geriatrics, and Gastroenterology (165 total beds, 24/24 h and 7/7 days open to the ED admissions). About one-third of all hospital admissions are directed to the Department of Medicine. The great part of medical and nursing staff is permanent, covering also festive days or holidays.
Comorbidity Score Calculation
We calculated a modified Elixhauser index, a novel score recently proposed by our group in order to take into due account the comorbidity burden.Citation21 For development of the score, we included hospital admissions for any cause in the department of internal medicine. At the time of the score development, more than 75,000 admissions aged 72 years were analyzed, and administrative data were used following guidelines suggested by Quan et al.Citation22 The points assigned to each condition selected in the score ranged from 0 to 16, and the possible range of the score varied between 0 and 89. The following conditions were considered for score calculation: age (0–60 years points=0; 61–70 years points=3; 71–80 years points=7; 81–90 years points 11; ≥91 years points 16), gender (male points=2), presence of renal diseases (points=1), neurological disorders (points=3), lymphoma (points=4), solid tumor with metastasis (points=4), ischemic heart disease (points=5), congestive heart disease (points=5), coagulopathy (points=8), fluid and electrolyte disorders (points=8), liver disease (points=10), weight loss (points=11), and metastatic cancer (points=12).Citation21 The risk of IHM became significant when the score was >40, overcoming the value of 60%. In this comparison study, the modified Elixhauser score was calculated using both administrative data recorded at the time of discharge and electronic medical charts. Authors evaluated all medical charts and recorded comorbidity according to the modified Elixhauser score and according to a previous prognostic index derived from clinical data proposed by Cei et al in 2015 in elderly medical patients.Citation23 For the calculation of this latter index male gender (point=1), dependency in activities of daily living (1–4 ADLs points=2; 5 ADLs points=5), congestive heart failure (points=2), solitary or hematologic cancer (points=3), metastatic cancer (points=8), serum creatinine >3 mg/dl (points=2), serum album <3 g/dl (points=2) or between 3 and 3.4 g/dl (point=1) were evaluated.Citation23
Statistical Analysis
Analysis was limited to hard clinical indicators: fatal (IHM) and nonfatal (patient discharged alive) outcome. We performed a descriptive analysis of all data collected, and results were expressed as absolute numbers, percentages, and mean ± SD. Univariate analysis was performed to define the difference between survivors and deceased subjects; a statistical analysis was performed using the Chi-square test, Student t test, and Mann–Whitney test as appropriate. We compared models using receiver operating characteristics (ROC) curve scores (c-statistics). The probability of risk of death assigning is the c-statistics, if the value is 0.5 the model is not a good predictor, while a value of 1 suggests a perfect discrimination between deceased and survivor individuals. Values lower than 0.7 are considered poor predictors and those between 0.7 and 0.8 could be reasonable. When the value is greater than 0.8 prediction is good. The area under the receiver-operator characteristic curve (AUC) and 95% confidence intervals were reported.
SPSS 13.0 for Windows (SPSS Inc., Chicago, IL, 2004) was used for statistical analyses.
Results
During the study period, 1962 individuals were admitted in the medical department (average 10.8 patients per day) of whom 348 were excluded because data were incomplete. Totally, 1614 subjects were analyzed, 43.3% were male and mean age was 79.1±13.1 years. IHM was recorded in 224 patients (13.9%). Main characteristics of the population are reported in , while prevalence of the different conditions considered in order to calculate the different scores using both administrative data recorded at the time of discharge and electronic medical charts is reported in . The number of comorbidities derived from electronic medical charts was higher than comorbidities derived from administrative data recorded at the time of discharge (3787 vs 1875), and modified Elixhauser score calculated from electronic medical charts had an average value of 5 points higher than modified Elixhauser score calculated from administrative data recorded at the time of discharge. Age and comorbidity scores were higher in deceased than in survivors ( and ). The modified Elixhauser score calculated by administrative data and by chart review and the comparator one was 18.13±9.36 vs 24.43±11.27 vs 7.63±3.3, respectively, and the c-statistic was 0.758 (95% CI 0.727–0.790), 0.811 (95% CI 0.782–0.840) and 0.740 (95% CI 0.709–0.771), respectively. AUC of the different scores are reported in . Values of modified Elixhauser score calculated by administrative data between 44 and 53 suggested a risk of IHM between 50% and 75%, whilst when the calculated value was higher than 53 risk of IHM was higher than 75% ().
Figure 1 Receiver operating characteristics (ROC) analysis reporting the area under the receiver-operator characteristic curve of the different scores evaluated.
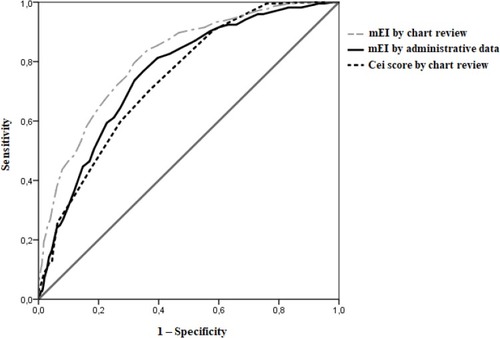
Figure 2 Relationship between values of modified Elixhauser score calculated by administrative data and risk of in-hospital mortality (IHM).
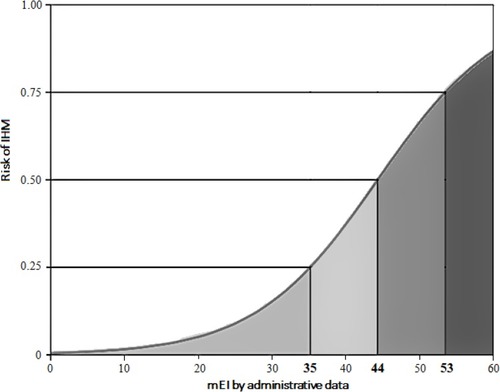
Table 1 Main Characteristics of the 1,614 Individuals Investigated
Table 2 Comparison of Prevalence of the Different Conditions Considered in Order to Calculate the Different Scores Using Both Electronic Medical Charts and Administrative Data Recorded at the Time of Discharge
Table 3 Main Characteristics of Deceased and Survivors
Table 4 Comparison of Prevalence of the Different Conditions Considered in Order to Calculate the Different Scores Using Electronic Medical Charts and Administrative Data Recorded at the Time of Discharge in Deceased and Survivors
Discussion
In this paper, we aimed to validate a modified Elixhauser score developed in 2017 by our groupCitation21 and we found that its performance could be considered reasonable if calculated using administrative data recorded at the time of discharge and good if calculated using electronic medical charts. Moreover, using electronic medical charts its performance was better than a prognostic index derived from clinical data and evaluated by Cei et al in 2015 in a similar setting;Citation23 the reason for comparing the modified Elixhauser score with the one evaluated by Cei et al is due to the setting, ie, Italian internal medicine wards, and the good performance reported by Cei et al that was 0.81 (95% CI 0.78–0.84).Citation23 We found that our modified Elixhauser score performed similarly to Cei et alCitation23 clinical prognostic index, and when it was calculated using clinical data derived by charts review its prognostic performance improved due to the higher number of comorbid conditions detected reviewing every single case.
We previously evaluated the usefulness of the modified Elixhauser score on risk factors for IHM in internal medicine wards patients with infectious diseases. We included all hospital admissions recorded in the database of the local hospital and ICD-9-CM codes were selected to identify infections, development of sepsis, and to calculate the score. The modified Elixhauser score was independently associated with IHM and the receiver operating characteristic (ROC) analysis showed that the area under the curve was 0.724 for detection of IHM.Citation24
In elderly population, acute medical condition requiring hospitalizations contributes to decline of health status,Citation25 and adjustment for comorbidities could be useful for risk prediction of negative outcomes. The use of comorbidities in risk adjustment for health outcomes research is frequently necessary to explain some of the observed variations. Medical chart reviews to obtain information on comorbidities is time consuming and laborious. Administrative databases have provided an alternative for health services researchers to obtain comorbidity information, however, the rates obtained from databases are still a matter of debate.
In 2003, de Groot et alCitation26 systematically reviewed available methods to measure comorbidity and to assess their validity and reliability. Content, concurrent, predictive and construct validity, and the reliability were assessed. The authors found that Charlson Comorbidity Index (CCI) was the most extensively studied score for predicting mortality, while Cumulative Illness Rating Scale (CIRS) addressed all relevant body systems without using specific diagnoses, Index of Coexisting Disease (ICED) measured disease severity and disability, Kaplan Index was specifically developed for use in diabetes research. All were considered valid and reliable methods to be used in clinical research.Citation26 Subsequently in 2012, it was carried out a systematic review in order to identify the best performing comorbidity indices for short-term and long-term mortality.Citation27 Authors analyzed 54 articles and found that the Deyo variant of CCI was the most commonly referred comparator followed by the Elixhauser measure. Deyo variant of CCI represents the CCI adaptation for ICD-9-CM.Citation28 Comorbidity predicted in a better way long-term than short-term mortality and Elixhauser seemed to be the best predictor for this outcome. In the case of short-term mortality, recalibration giving empirical weights was reported to be more important than the choice of comorbidity measure.Citation27 In fact, our modified Elixhauser score was developed weighting differently conditions impacting survival of patients during admission.Citation21
As expected, chart review data turns out to perform better in predicting outcome of patients than administrative information. Similar performance was showed in 1992 by Hannan et alCitation29 that compared the ability of a clinical and administrative database in New York State to predict IHM and to assess hospital performance for coronary artery bypass graft surgery. They found that clinical data were better than administrative ones in predicting mortality.Citation29 Moreover, in 1999 Kieszak et alCitation30 compared CCI calculated from medical record data and ICD-9-CM in order to determine how well inpatient and 30-day mortality, length of stay, and complications were predicted among Medicare beneficiaries hospitalized for carotid endarterectomy. Using logistic regression, CCI derived from clinical chart review was found to be a significant predictor for all clinical outcomes, after controlling for age, gender, and neurologic and medical risk factors. In 2006 Martins et alCitation31 compared the predictive capacity of the original CCI, the CCI with new assigned diagnostic codes and estimated weights, and a new developed comorbidity index in a Brazilian population; the c-statistic was 0.72 for the original CCI, and increased to 0.74 for the CCI with new weights and 0.76 for the new index. The c-statistic increases in all the comorbidity indices with the utilization of more diagnostic information.Citation31 In 2007 Luthi et alCitation32 compared cross-sectionally the CCI derived from a rapid single-day chart review with the same index derived from administrative data (ICD-10-CM) to determine how well each predicted IHM and nosocomial infection. Authors evaluated 890 adult patients hospitalized from acute care wards in Switzerland and found that CCI derived from administrative data provided a higher c-statistic compared with single-day chart review for IHM (0.863 vs 0.795) and for nosocomial infection (0.645 vs 0.614). They concluded that CCI derived from administrative data was superior to the index derived from rapid single-day chart review.Citation32
In administrative database analysis, an important issue that should be taken into account is the lower number of comorbidities identified compared to those recognized by charts review. In our study, we detected 1875 comorbidities using administrative records and 3787 using charts review. In 2011, Chong et alCitation33 quantified retrospectively and cross-sectionally the agreement between administrative data and medical charts review in determining the presence of comorbidities. Moreover, authors examined which factors were associated with under- or over-reporting of comorbidities using administrative data. They analyzed patients aged ≥55 years, hospitalized for pneumonia at 3 acute care hospitals. They reported that prevalence of almost all comorbidities obtained using administrative data was lower than that obtained using medical charts review. Agreement between comorbidities obtained from medical charts and administrative data varied widely. Factors associated with over-reporting of comorbidities were increased length of hospital stay, disease severity, and IHM. On the contrary, those associated with under-reporting were number of comorbidities, age, and hospital admission in the previous 3 months. They concluded that the validity of using secondary diagnoses detected from administrative data as an alternative to medical charts for identification of comorbidities is related to age, number of comorbidities, hospital admission in the previous 3 months, severity of illness, length of hospitalization, and IHM.Citation33 In the same year, Christensen et alCitation34 examined the performance of comorbidity scores in predicting mortality of intensive care unit (ICU) patients. They evaluated CCI alone and in combination with other readily available administrative data and three physiology-based scores (acute physiology and chronic health evaluations [APACHE] II, simplified acute physiology score [SAPS] II, and SAPS III) in predicting short- and long-term mortality following intensive care. Data for CCI calculation, age and gender, surgical/medical status, social factors, mechanical ventilation and renal replacement therapy, primary diagnosis, and complete follow-up for 1-year mortality were obtained from administrative databases. When CCI was combined with other administrative data c-statistics was 0.75 for IHM, 0.75 for 30-day mortality, and 0.72 for 1-year mortality. Difference between c-statistics of physiology-based systems and the CCI combined with other administrative data was not significant. Authors concluded that CCI combined with administrative data could predict short- and long-term mortality for ICU patients in a similar way as physiology-based scores.Citation34 Quan et alCitation35 re-evaluated the CCI and reassigned weights to each condition by identifying and tested predictivity of mortality within 1 year after hospital discharge. Authors applied the updated index and weights to administrative databases from 6 different countries and tested for their ability to predict IHM. C-statistics for detecting IHM mortality between the new score and the original CCI were 0.825 and 0.808, respectively, in Australia, 0.828 and 0.825 in Canada, 0.878 and 0.882 in France, 0.727 and 0.723 in Japan, 0.831 and 0.836 in New Zealand, and 0.869 and 0.876 in Switzerland.Citation35
Limitations
Several limitations should be mentioned. First of all, this is a single-center retrospective study, therefore results could not be generalizable. Secondly, we did not differentiate between conditions existing before admission and complications developed during hospitalization; moreover, we did not take into consideration admission diagnosis. Third, the modified Elixhauser index did not include all cardiovascular diseases, nor a very common illness such as chronic obstructive pulmonary disease, that could be frequently encountered in patients admitted to internal medicine wards.Citation36,Citation37 Outcome of these conditions is highly related to comorbidity.Citation36,Citation37 Fourth, our outcome was IHM, we had no information about out-of-hospital mortality. Originally, the score used in this study was developed from administrative data that are claimed for financial reasons and not for research, therefore data quality could be different in different health care organization and hospital setting.Citation38 Performance of clinical physicians at the time of coding and financial incentives such as diagnosis-related groups or healthcare-related groups could be different between hospitals depending on gaps in clinical information and the billing pressure could alter the quality rating of administrative data, as underlined by our comparison with data obtained from chart review. We believe that errors of omission were the leading cause of different performances of modified Elixhauser score calculated by administrative data and by chart review.Citation39 Interrater reliability could not be assessed because only one author reviewed medical chart, besides we compared scores with different number of items (13 vs 9). On the other hand, both scores had been tested in similar Italian hospital settings.
Conclusion
Physicians mainly focus their attention on diagnosis and treatment, however nowadays, due to the aging of the population, prognosis is becoming a major responsibility, therefore, risk stratification has to be taken into account in everyday clinical practice. Comorbidity is often considered as a complication in specialized clinical conditions, however, it could be that associations of different chronic illnesses determine the outcome of different treatment.Citation40–Citation42 Diagnostic and therapeutic strategies should be influenced by prognosis, especially in the case of shortage of resources.
Our study allowed validation and comparison of a new prognostic score derived from Elixhauser index. Calculation was based on both chart review and administrative data in order to identify the quoad vitam prognosis of patients admitted to internal medicine wards. The prognostic power of such a score increased when it was calculated by chart review instead of using administrative data due to the higher number of comorbidity that could be identified. However, when it was calculated by using administrative data its prognostic efficiency was similar to a previous index calculated in a similar clinical setting by direct assessment of the patient’s comorbidities.
Acknowledgments
We are indebted to Mr Mauro Pasin from Hypertension Centre, University Hospital St. Anna, Ferrara, Italy for his valuable and precious collaboration. This work has been supported, in part, by a research grant from the Ministry of Education, University and Research (MIUR), [FFABR 2017 (Fondo Finanziamento Attività di Base della Ricerca) - Prof Fabio Fabbian 2017].
Disclosure
The authors declare that there are not any potential conflicts of interests that are directly or indirectly related to the data presented in the paper.
References
- Wolff JL, Starfield B, Anderson G. Prevalence, expenditures, and complications of multiple chronic conditions in the elderly. Arch Intern Med. 2002;162(20):2269–2276. doi:10.1001/archinte.162.20.226912418941
- Uijen AA. van de Lisdonk EH. Multimorbidity in primary care: prevalence and trend over the last 20 years. Eur J Gen Pract. 2008;14(sup1):28–32. doi:10.1080/1381478080243609318949641
- van den Akker MBF, Knottnerus JA. Comorbidity or multimorbidity: what’s in a name? A review of literature. Eur J Gen Practice. 1996;(2):65–70.
- Gijsen R, Hoeymans N, Schellevis FG. Causes and consequences of comorbidity: a review. J Clin Epidemiol. 2001;54:661–674. doi:10.1016/S0895-4356(00)00363-211438406
- Willadsen TG, Siersma V, Nicolaisdóttir DR, et al. Multimorbidity and mortality: a 15-year longitudinal registry-based nationwide Danish population study. J Comorb. 2018;8:1–9. doi:10.1177/2235042X1880406329492397
- Nunes PBFT, Mielke GI, Thumé E. Multimorbidity and mortality in older adults: a systematic review and meta-analysis. Arch Gerontol Geriatr. 2016;67:130–138. doi:10.1016/j.archger.2016.07.00827500661
- Menotti A, Mulder I, Nissinen A. Prevalence of morbidity and multimorbidity in elderly male populations and their impact on 10-year all-cause mortality: the FINE study (Finland, Italy, Netherlands, Elderly). J Clin Epidemiol. 2001;54:680–686. doi:10.1016/S0895-4356(00)00368-111438408
- Ferrer A, Formiga F, Sanz H. Multimorbidity as specific disease combinations, an important predictor factor for mortality in octogenarians: the Octabaix study. Clin Interv Aging. 2017;12:223–231. doi:10.2147/CIA.S12317328184153
- Diederichs C, Berger K, Bartels DB. The measurement of multiple chronic diseases—a systematic review on existing multimorbidity indices. J Gerontol A Biol Sci Med Sci. 2011;66:301–311. doi:10.1093/gerona/glq20821112963
- Willadsen TG, Bebe A, Køster-Rasmussen R, et al. The role of diseases, risk factors and symptoms in the definition of multimorbidity - a systematic review. Scand J Prim Health Care. 2016;34(2):112–121. doi:10.3109/02813432.2016.115324226954365
- Tinetti ME, Fried T. The end of the disease era. Am J Med. 2004;116(3):179–185. doi:10.1016/j.amjmed.2003.09.03114749162
- Fabbian F, Pala M, De Giorgi A, et al. In-hospital mortality in patients with renal dysfunction admitted for myocardial infarction: the Emilia-Romagna region of Italy database of hospital admissions. Int Urol Nephrol. 2013;45(3):769–775. doi:10.1007/s11255-012-0250-922828743
- Fabbian F, Gallerani M, Pala M, et al. In-hospital mortality for pulmonary embolism: relationship with chronic kidney disease and end-stage renal disease The hospital admission and discharge database of the Emilia Romagna region of Italy. Intern Emerg Med. 2013;8:735–740.23247683
- Fabbian F, Gallerani M, Pala M, et al. Association between in-hospital mortality and renal dysfunction in 186,219 patients hospitalized for acute stroke in the Emilia-Romagna region of Italy. Angiology. 2014;65(10):906–910. doi:10.1177/000331971351201724277911
- Fabbian F, De Giorgi A, López-Soto PJ, et al. Is female gender as harmful as bacteria? Analysis of hospital admissions for urinary tract infections in elderly patients. J Womens Health. 2015;24(7):587–592. doi:10.1089/jwh.2014.5140
- Fabbian F, De Giorgi A, Manfredini F, et al. Impact of comorbidity on outcome in kidney transplant recipients: a retrospective study in Italy. Intern Emerg Med. 2016;11(6):825–832. doi:10.1007/s11739-016-1438-227003820
- Fabbian F, De Giorgi A, Tiseo R, et al. Neoplasms and renal transplantation: impact of gender, comorbidity and age on in-hospital mortality. A retrospective study in the region Emilia-Romagna of Italy. Eur Rev Med Pharmacol Sci. 2018;22:2266–2272.29762828
- Fabbian F, De Giorgi A, Manfredini F, et al. Impact of renal dysfunction on in-hospital mortality of patients with severe chronic obstructive pulmonary disease: a single-center Italian study. Int Urol Nephrol. 2016;48(7):1121–1127. doi:10.1007/s11255-016-1272-527020445
- Fabbian F, Fedeli U, De Giorgi A, et al. Sex and acute oesophageal variceal bleeding-related in-hospital mortality: a 15-year retrospective study. Eur Rev Med Pharmacol Sci. 2019;23(2):811–817. doi:10.26355/eurrev_201901_1689530720189
- Elixhauser A, Steiner C, Harris DR, Coffey RM. Comorbidity measures for use with administrative data. Med Care. 1998;36(1):8–27. doi:10.1097/00005650-199801000-000049431328
- Fabbian F, De Giorgi A, Maietti E, et al. A modified Elixhauser score for predicting in-hospital mortality in internal medicine admissions. Eur J Intern Med. 2017;40:37–42. doi:10.1016/j.ejim.2017.02.00228187963
- Quan H, Sundararajan V, Halfon P, et al. Coding algorithms for defining comorbidities in ICD-9-CM and ICD-10 administrative data. Med Care. 2005;43(11):1130–1139. doi:10.1097/01.mlr.0000182534.19832.8316224307
- Cei M, Mumoli N, Vitale J, Dentali F. A prognostic index for 1-year mortality can also predict in-hospital mortality of elderly medical patients. Intern Emerg Med. 2015;10:575–579. doi:10.1007/s11739-015-1196-625633232
- Fabbian F, De Giorgi A, Boari B, et al. Infections and internal medicine patients: could a comorbidity score predict in-hospital mortality? Medicine (Baltimore). 2018;97(42):e12818. doi:10.1097/MD.000000000001281830334978
- Clegg A, Young J, Iliffe S, Rikkert MO, Rockwood K. Frailty in elderly people. Lancet. 2013;381:752–762. doi:10.1016/S0140-6736(12)62167-923395245
- de Groot V, Beckerman H, Lankhorst GJ, Bouter LM. How to measure comorbidity. A critical review of available methods. J Clin Epidemiol. 2003;56:221–229.12725876
- Sharabiani MT, Aylin P, Bottle A. Systematic review of comorbidity indices for administrative data. Med Care. 2012;50:1109–1118. doi:10.1097/MLR.0b013e31825f64d022929993
- Deyo RA, Cherkin DC, Ciol MA. Adapting a clinical comorbidity index for use with ICD-9-CM administrative databases. J Clin Epidemiol. 1992;45(6):613–619. doi:10.1016/0895-4356(92)90133-81607900
- Hannan EL, Kilburn H Jr, Lindsey ML, Lewis R. Clinical versus administrative data bases for CABG surgery. Does it matter? Med Care. 1992;30:892–907. doi:10.1097/00005650-199210000-000021405795
- Kieszak SM, Flanders WD, Kosinski AS, Shipp CC, Karp H. A comparison of the Charlson comorbidity index derived from medical record data and administrative billing data. J Clin Epidemiol. 1999;52:137–142. doi:10.1016/S0895-4356(98)00154-110201654
- Martins M, Blais R. Evaluation of comorbidity indices for inpatient mortality prediction models. J Clin Epidemiol. 2006;59:665–669. doi:10.1016/j.jclinepi.2005.11.01716765268
- Luthi JC, Troillet N, Eisenring MC, et al. Administrative data outperformed single-day chart review for comorbidity measure. Int J Qual Health Care. 2007;19:225–231. doi:10.1093/intqhc/mzm01717599922
- Chong WF, Ding YY, Heng BH. A comparison of comorbidities obtained from hospital administrative data and medical charts in older patients with pneumonia. BMC Health Serv Res. 2011;11:105. doi:10.1186/1472-6963-11-10521586172
- Christensen S, Johansen MB, Christiansen CF, Jensen R, Lemeshow S. Comparison of Charlson comorbidity index with SAPS and APACHE scores for prediction of mortality following intensive care. Clin Epidemiol. 2011;3:203–211. doi:10.2147/CLEP.S2024721750629
- Quan H, Li B, Couris CM, et al. Updating and validating the Charlson comorbidity index and score for risk adjustment in hospital discharge abstracts using data from 6 countries. Am J Epidemiol. 2011;173:676–682. doi:10.1093/aje/kwq43321330339
- Scrutinio D, Passantino A, Guida P, et al. Prognostic impact of comorbidities in hospitalized patients with acute exacerbation of chronic heart failure. Eur J Intern Med. 2016;34:63–67. doi:10.1016/j.ejim.2016.05.02027263064
- Spannella F, Giulietti F, Cocci G, et al. Acute exacerbation of chronic obstructive pulmonary disease in oldest adults: predictors of in-hospital mortality and need for post-acute care. J Am Med Dir Assoc. 2019;20(7):893–898. doi:10.1016/j.jamda.2019.01.12530826270
- Lash TL, Mor V, Wieland D, Ferrucci L, Satariano W, Silliman RA. Methodology, design, and analytic techniques to address measurement of comorbid disease. J Gerontol a Biol Sci Med Sci. 2007;62(3):281–285. doi:10.1093/gerona/62.3.28117389725
- Iezzoni LI. Assessing quality using administrative data. Ann Intern Med. 1997;127:666–674. doi:10.7326/0003-4819-127-8_Part_2-199710151-000489382378
- Karnad A, Pannelay A, Boshnakova A, Lovell AD, Cook RG. Stroke prevention in Europe: how are 11 European countries progressing toward the European Society of Cardiology (ESC) recommendations? Risk Manag Healthc Policy. 2018;11:117–125. doi:10.2147/RMHP.S16343930197544
- Shen J, Xin W, Li Q, Gao Y, Zhang J. A grading system for the prediction of unilateral chronic subdural hematoma recurrence after initial single burr hole evacuation. Risk Manag Healthc Policy. 2019;12:179–188. doi:10.2147/RMHP.S22214431802959
- Liu Y, Ye S, Xiao X, et al. Machine learning for tuning, selection, and ensemble of multiple risk scores for predicting Type 2 diabetes. Risk Manag Healthc Policy. 2019;12:189–198. doi:10.2147/RMHP.S22576231807099