Abstract
Purpose
To identify more objectively predictive factors of severe outcome among patients hospitalized for coronavirus disease 2019 (COVID-19).
Patients and Methods
A retrospective cohort of 479 hospitalized patients diagnosed with COVID-19 in Hunan Province was selected. The prognostic effects of factors such as age and laboratory indicators were analyzed using the Kaplan–Meier method and Cox proportional hazards model. A prognostic nomogram model was established to predict the progression of patients with COVID-19.
Results
A total of 524 patients in Hunan province with COVID-19 from December 2019 to October 2020 were retrospectively recruited. Among them, 479 eligible patients were randomly assigned into the training cohort (n = 383) and validation cohort (n = 96), at a ratio of 8:2. Sixty-eight (17.8%) and 15 (15.6%) patients developed severe COVID-19 after admission in the training cohort and validation cohort, respectively. The differences in baseline characteristics were not statistically significant between the two cohorts with regard to age, sex, and comorbidities (P > 0.05). Multivariable analyses included age, C-reactive protein, fibrinogen, lactic dehydrogenase, neutrophil-to-lymphocyte ratio, urea, albumin-to-globulin ratio, and eosinophil count as predictive factors for patients with progression to severe COVID-19. A nomogram was constructed with sufficient discriminatory power (C index = 0.81), and proper consistency between the prediction and observation, with an area under the ROC curve of 0.81 and 0.86 in the training and validation cohort, respectively.
Conclusion
We proposed a simple nomogram for early detection of patients with non-severe COVID-19 but at high risk of progression to severe COVID-19, which could help optimize clinical care and personalized decision-making therapies.
Introduction
The global pandemic of coronavirus disease 2019 (COVID-19) started in December 2019 and has brought huge challenges not only for medical institutes but also for research organizations worldwide, given that doctors and scientists knew very little about this novel coronavirus.Citation1 According to the reports from the World Health Organization (WHO), over 266 million confirmed cases and 5.2 million deaths have been reported globally, up to December 31, 2021. China’s lockdown measures slowed down the growth rate and increased the doubling time of COVID-19 cases. Their successful experience in tackling COVID-19 has become an effective model for other countries.Citation2,Citation3 The huge burden for medical resources and global economics has been inevitable because of the COVID-19 pandemic.Citation4–Citation6
The etiology of COVID-19 involves the entry of the severe acute respiratory syndrome coronavirus-2 (SARS-CoV-2) into human cells via binding to angiotensin-II converting enzyme (ACE2).Citation7 The viral particle penetrates human cells with the help of host cell receptors and endosomes, and TMPRSS2, a serine protease, facilitates the entry,Citation8 resulting in various immune or inflammatory responses.Citation9,Citation10 It has been reported that SARS-CoV-2 infection increases the expression of inflammatory cytokines, and the levels positively correlate with the viral load.Citation10 Patients with COVID-19 usually have symptoms of fever, shortness of breath, fatigue, and a cough;Citation11,Citation12 nausea and vomiting are rare.Citation12 About three quarters show pneumonia, some patients will go on to develop acute respiratory distress syndrome, and a few of them will show rapid worsening of symptoms and die of organ failure.Citation12
The wide spectrum of clinical features ranges from asymptomatic infections to mild, moderate, and eventually severe COVID-19.Citation9,Citation13,Citation14 However, it is worthy to note that the clinical spectrum of COVID-19 seems to harbor regional differences,Citation15 with fever and dyspnea being more common in Wuhan than in other regions of China.Citation16 Severe cases usually manifest dyspnea, hypoxia, diarrhea, and dyspnea, thereby requiring hospitalization in the intensive care unit (ICU) to ensure comprehensive treatment. Patients at this stage of the disease are very likely to die if not treated in a timely manner and/or their physical conditions are terrible.
To provide evidence for coping with this disease, many previous studies have focused on the risk factors predicting the prognosis.Citation17–Citation20 Patients with older age; male sex; and comorbidities including hypertension, diabetes, cardiovascular disease, chronic kidney disease, chronic pulmonary disease, and cancer have been found to be associated with worse outcomes than others.Citation1,Citation13,Citation21–Citation23 Among these factors, age is the most strongly associated with poor prognosis of COVID-19 because of the different immune response associated with age.Citation9 The above comorbidities can influence survival in patients with COVID-19;Citation24 however, the use of appropriate drugs to treat these comorbidities may also influence the outcome. For instance, although no evidence yet shows that chemotherapy can increase the risk of COVID-19,Citation25 there is emerging evidence that cancer immunotherapy can improve the COVID-19 outcomes.Citation26 This can be explained by mitochondrial restoration of T cells upon exposure to anti-programmed cell death protein-1 (anti-PD-1) antibodies.Citation27–Citation29
With the huge amount of data being generated rapidly by infected people, it is recommended to make good use of this data to develop medical treatments more effectively.Citation8 Thus, we need to identify indicators to predict the progression of COVID-19. Given the serious consequences of developing severe COVID-19, researchers have attempted to study the factors associated with severe COVID-19 and have consistently observed that lymphopenia, high levels of serum IL-6, IL-1β, and TNF-α were associated with severe COVID-19.Citation20,Citation21,Citation30,Citation31 Moreover, researchers have also attempted to build prognosis models to predict the probability of a patient’s progression to severe COVID-19, upon admission.
A prognostic nomogram model has been shown to be an effective tool, to predict a clinical outcome of interest, and this model performs better than other options, such as probability tables and risk groupings.Citation32 Thus, nomograms have been widely used in studies on clinical personalized decision-making.Citation33–Citation36
However, only a few studies have considered laboratory indicators at admission as prognosis factors for severe COVID-19 in a relatively large sample.Citation37–Citation39 The different spectrum of COVID-19 in different regions may also reveal the distinct etiological model of progression. Therefore, in this study, we aimed to explore associations between age and laboratory findings in patients with severe COVID-19 in Hunan Province, China, and develop a nomogram to predict the probability of progression to severe COVID-19.
Materials and Methods
Study Design and Participants Enrollment
This is an observational study. Medical records of hospitalized COVID-19 patients diagnosed in 26 hospitals in Hunan province were collected. All patients were diagnosed with COVID-19 by positive high-throughput sequencing or real-time reverse-transcription polymerase-chain-reaction (RT-PCR) assay using nasal and pharyngeal swab specimens. The inclusion criteria for our study were as follows: (a) clinically and laboratory-confirmed SARS-CoV-2 infection; (b) age ≥18; (c) complete baseline clinical information, and follow-up data with confirmed clinical outcome; and (d) without severe pneumonia at the time of admission. Finally, 479 patients were eligible for this study. The data were cross-checked by experienced respiratory clinicians.
This study was approved by the Independent Ethical Committee of the Institute of Clinical Pharmacology, Central South University (CTXY-140007-2). Informed consent was obtained in accordance with the World Medical Association Declaration of Helsinki.
Data Collection
The clinical information of patients with COVID-19 was extracted from the hospital management information system of each hospital. Baseline characteristics in terms of age; sex; and comorbidities including diabetes, hypertension, liver disease, renal disease, cardiovascular disease, and cancer were collected. Routine laboratory tests such as blood tests for complete blood count, neutrophil-to-lymphocyte ratio (NLR); biochemical tests for total protein, lactic dehydrogenase, aspartate transaminase (AST), creatine kinase muscle–brain isoform (CK-MB), total bilirubin, creatinine, albumin, globulin, and albumin-to-globulin ratio; coagulation function indices for D-dimer, fibrinogen, activated partial thromboplastin time (APTT), prothrombin time (PT), thrombin time (TT); and infection-related indicators for C-reactive protein (CRP) and uric acid were performed after admission within 2 days and were included as predictive factors in the study.
Definition of Outcome
The outcome of the prediction model was the progression to severe COVID-19 after admission. Severe COVID-19 in this study included severe and critical pneumonia. The diagnostic criteria of severe or critical COVID-19Citation40 were as follows: 1) respiratory distress (respiratory rate, ≥30 cycles per minute); 2) oxygen saturation ≤93% or arterial partial pressure of oxygen (PaO2)/oxygen concentration FiO2 ≤300 mmHg at the resting state; 3) respiratory failure requiring mechanical ventilation; 4) shock; and 5) other organ failure requiring monitoring and treatment in the ICU. Severe illness was determined if one of the above criteria was met.
The follow-up time was calculated from the first day of hospitalization to the date of development of severe COVID-19 or discharge from hospital or death (for the period January 31st to March 14th, 2020).
Statistical Analysis and Nomogram Construction
Statistical analysis was performed using R software (version 3.6.2, R Foundation for Statistical Computing; http://www.r-project.org/). Continuous variables were expressed as the mean ± standard deviation or median with interquartile range (IQR). Categorical variables were expressed as frequencies and proportions.
The 479 patients with COVID-19 were randomly assigned at a ratio of 8:2 into two cohorts—the training cohort and validation cohort. The difference of the indicator of interest between the groups was compared with t-test for continuous variables and chi-square test (or Fisher’s exact test) for categorical variables. A univariate Cox regression model was employed to explore the association between each indicator and progression to severe COVID-19, and a multivariate Cox regression model was applied to determine the risk factors for designing the nomogram. Estimates derived from Cox regressions were presented as hazard ratios and 95% confidence intervals (CI). Kaplan–Meier time-to-event analyses were employed to test the differences of survival between age groups or among quartiles of laboratory indicators with Log rank tests, and Kaplan–Meier survival plots were drawn for the indicators of interest.
Age was classified into binary variables (0 for <65 years, 1 for ≥65 years), and laboratory finds were classified into quartiles modeled as continuous variables (<25% quartile = 1; ≥25% and <50% quartile = 2; ≥50% quartile and <75% quartile = 3; ≥75% quartile = 4). Parameters with statistical significance (p < 0.05) in the univariate Cox regression analysis were selected as candidates for risk score development of severity. A final model selection was performed via a stepwise selection process with the Akaike information criterion. Subsequently, variables identified in the final model were used to construct the nomogram based on the risk score with their coefficients:
where n stands for the number of prognostic variables in the model; Valuei is the original value of the variablei; Coei is the estimated coefficient of the Valuei in the Cox regression model.
The apparent performance of the nomogram was elucidated by discrimination and calibration. The discriminatory power of the nomogram was estimated by Harrell’s C-index (C-index). Calibration of the nomogram in this study was executed by the Hosmer–Lemeshow goodness-of-fit test accompanied by visual calibration curves. The total scores of each patient were calculated based on the nomogram. Area under the receiver operating characteristic curve (AUC) was used to evaluate the performance of the risk score in distinguishing individuals with severe COVID-19 from those with non-severe COVID-19. A two-sided P-value <0.05 was considered to be statistically significant.
Results
A total of 524 patients aged ≥18 years and non-severe COVID-19 were screened in this study. In all, 45 patients were excluded because of incomplete information. Finally, 479 patients were included in our study: 383 patients in the training cohort and 96 patients in the validation cohort (). As presented in , there were 245 (51.1%) male and 234 (48.9%) female patients, respectively. The mean age of patients was 46.6±14.8 years for the training cohort and 46.4±14.2 for the validation cohort. Further, 68 (17.8%) and 15 (15.6%) patients developed severe COVID-19 during the follow-up period after admission in the training cohort and validation cohort, respectively. The differences of baseline characteristics were not statistically significant between the above two cohorts with regard to age, sex, or comorbidities (P > 0.05) (). In stratification analysis, mean age and history of hypertension in patients who developed severe illness was significantly higher than in those who did not develop severe illness (P < 0.05). In the training cohort, the proportion of male patients was significantly higher among the severe patients’ category than those non-severe cases (, P < 0.05).
Table 1 Baseline Characteristics of Patients with COVID-19 in the training and Validation Cohorts
Figure 1 Study flowchart detailing which samples of patients with COVID-19 were utilized at each phase of statistical analysis.
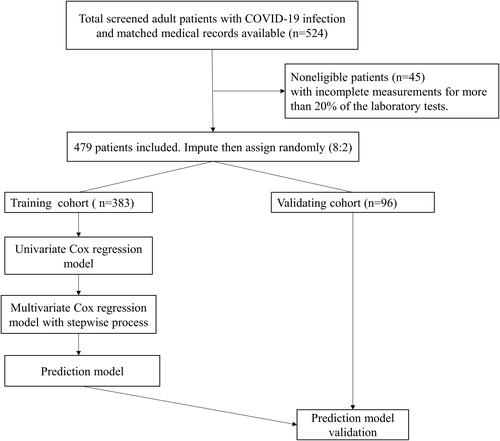
Laboratory indicators were stratified as quartiles. As shown in , univariate Cox regression analysis revealed that eosinophils, albumin, lymphocytes, albumin-to-globulin ratio, and total protein showed a protective effect against severe COVID-19, while TT, globulin, CK-MB, creatinine, APTT, fibrinogen, ALT, neutrophils, D-dimer, AST, urea, NLR, CRP, and lactic dehydrogenase were statistically significant risk factors for progression to severe COVID-19 (, all P < 0.05).
Figure 2 Prognostic associations of laboratory findings in the training dataset.
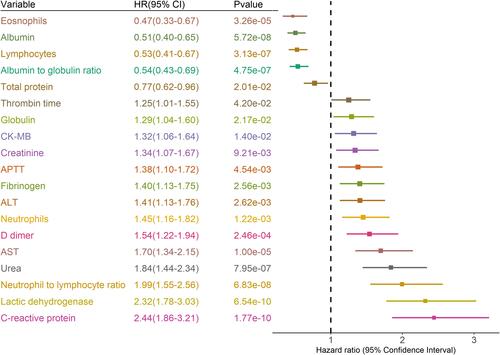
The results of multivariate Cox regression model showed independent predicted factors for the severe outcome. Independent predictive factors associated with severe COVID-19 were observed for age, albumin-to-globulin ratio, CRP, fibrinogen, lactic dehydrogenase, eosinophils, NLR, and urea (). Kaplan–Meier survival plots for these prognostic factors are shown in . As compared with age <65 years, age ≥65 years was associated with an increased risk of severe COVID-19, with a HR of 3.05 (95% CI: 1.79–5.18).
Figure 3 Kaplan-Meier survival plots for prognostic factors.
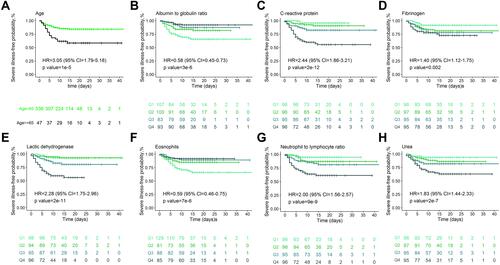
Based on the final multivariable model, a nomogram was established by assigning a weighted score to each of the factors associated with severe COVID-19, which could predict the probability of severe COVID-19 in an individual patient (). The total score was calculated as follows: 0.97367×age (≥65)-1.18836×albumin to globulin ratio+0.00647×CRP-9.74641×eosinophils+0.00374×fibrinogen+0.00402×lactic dehydrogenase+0.09469×urea+0.02773×NLR.
Figure 4 Prognostic nomogram for predicting the severe illness-free survival probability of patients with COVID-19.
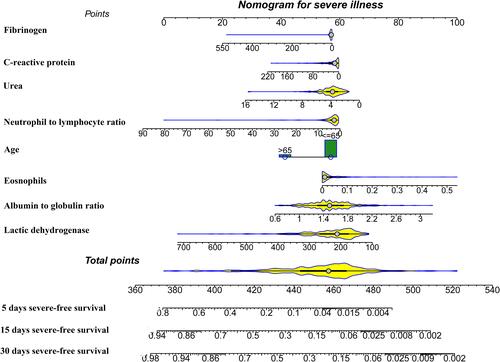
To calculate the 5-day, 15-day, and 30-day overall survival probability, we first determined each factor based on the points scale at the top of the nomogram and then summed the points of each factor. Finally, the overall survival probabilities of 5-day, 15-day, and 30-day were obtained based on the bottom point scale of the nomogram ().
Our nomogram exhibited excellent discrimination in estimating the probability of progression to severe COVID-19, with a C-index of 0.81 (95% CI: 0.73–0.89). In addition, the calibration plot for severe COVID-19 probability showed good agreement between the prediction by nomogram and actual observation in the training cohort as referred to the 5-day, 15-day, and 30-day overall survival probability ().
Figure 5 Calibration curves of the nomogram predicting severe illness-free survival in patients with COVID-19.
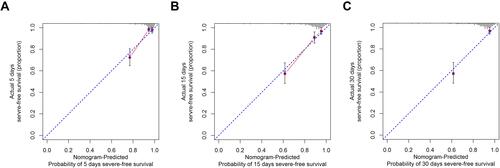
Furthermore, as displayed in , our proposed nomogram showed good discrimination for predicting severe COVID-19 (AUC = 0.81 in the training cohort; AUC = 0.86 in the validation cohort). Based on their risk scores, patients were stratified into the high and low risk groups for developing severe COVID-19 according to the median value of the score. Patients with high risk showed a significantly worse OS in the training cohort and validation cohort, with an HR of 7.22 (95% CI: 3.57, 14.6) and 16.45 (95% CI: 2.16, 125.3), respectively, than those with low risk.
Figure 6 Receiver operating characteristic curves and severe illness-free survival curves for high and low severity risk groups for the risk prediction model.
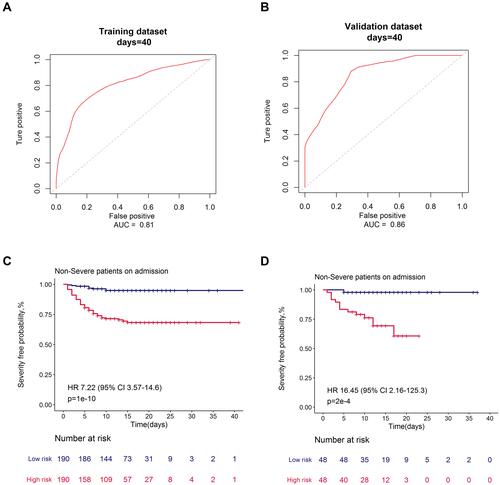
Discussion
In this study, we attempted to reveal the clinical characteristics and influential factors for progression to severe outcome in patients with laboratory-confirmed COVID-19 in the real-world setting, based on a provincial cohort by developing and validating a nomogram. Our nomogram comprised eight indicators including age and laboratory indices at admission such as albumin-to-globulin ratio, CRP, fibrinogen, lactic dehydrogenase, eosinophils, NLR, and urea. This nomogram proved to be a stable and powerful model to predict the progression of patients with non-severe COVID-19 to severe COVID-19, with a C-index of 0.81 (95% CI: 0.73–0.89). Patients with higher score would have a higher risk of progression to severe COVID-19. By October 31, 2020, the Hunan National Health Commission had reported 1020 patients with laboratory-confirmed COVID-19 in the Hunan Province. Our current cohort represents 47.0% of reported patients from multi-centers and harbors the efficiency to explore factors including laboratory indicators and age of subjects with COVID-19 who progress to severe illness. Early prediction of severe COVID-19 could help clinicians to identify patients at high-risk of progression to severe illness, and then appropriately allocate critical care resources in public health emergencies.
The proportion of patients with confirmed COVID-19 progressing into severe COVID-19 was 17.3% in the current cohort, which is lower than the earlier reports from a large community isolation center in Wuhan, China,Citation41–Citation43 Huangshi, China,Citation34 or North-Eastern France.Citation44 This difference could be attributed to two aspects. First, the mean age of our cohort was 46 years, which was significantly lower than that of the other two studies. We observed that patients with age >65 years exhibited a much higher risk of progression to severe COVID-19 than those aged <65 years. Reports from previous studies have revealed that the probability of a fatal outcome for patients with COVID-19 increases with advanced age,Citation34,Citation43 which is consistent with our study results. Furthermore, SARS-CoV-2 utilizes the host ACE2 receptor to enter human cells. Researchers have found that ACE2 expression was significantly upregulated with age in patients with severe COVID-19, but not in those with non-severe COVID-19,Citation45 and increased in the lungs of patients with comorbidities associated with severe COVID-19.Citation46 Second, different clinical spectrum according to regions may also explain this difference.Citation15
Predicting models were widely used in the diagnosis and prognosis of COVID-19, especially to predict mortality and severe/critical COVID-19.Citation47 However, most of these models would not be appropriate to apply in medical practices because of bias in sample selection, data collection, and data analysis.Citation47 Common predictors of severe COVID-19 were most studied for comorbidities such as diabetes and hypertension, and age together with laboratory bioindicators.Citation34,Citation42,Citation43 However, because age is the most important factor in predicting prognosis and elderly patients usually have underlying chronic diseases, it is concerning that collinearity may potentially influence the accuracy and stability of the model, especially in studies with a small sample size. In the current study, we mainly included objective factors of age and laboratory indicators to predict the outcome of severe COVID-19 to ensure the accuracy of data source in an emergency situation, covering nearly 50% of patients diagnosed in Hunan province in a real-world setting with 100% confirmed endpoints.
Several previous studies have reported that age and a list of laboratory findings could predict the progression of COVID-19,Citation34,Citation42,Citation43 which proved that a nomogram consisting of these factors might be an effective tool to manage patients with COVID-19. A study conducted by Cai et alCitation43 found that a nomogram model incorporating age, CRP, and D-dimer could help identify patients with high risk of progression to severe illness. Although the sample size of this study was relatively small, the nomogram showed great power with an AUC of 0.807 and 0.902 in the treatment and validation cohorts, respectively. Another study revealed that age together with lymphocyte count and pulmonary opacity score could serve as components of a predictive model, and the model was validated in a cohort with 105 patients from Huangshi, Hunan Province.Citation34 Unlike the above studies, Yang et alCitation42 focused on the survival of patients with severe COVID-19. The researchers first identified 271 patients with severe COVID-19 from three independent hospitals; then, the hospital with the largest sample of patients with severe COVID-19 was treated as the training cohort, the other two hospitals were considered as the validation cohorts. The nomogram constructed with age (≥70 years), dyspnea (breathing rrate >0/min), lymphocyte count (<1.0×109/L), and interleukin-6 (>10 pg/mL), exhibited good consistence with the actual observation of predicting survival in those patients.Citation42
In our study, we observed higher risk of progression to severe COVID-19 for higher level of CRP, fibrinogen, lactic dehydrogenase, NLR, and urea, and a protective effect of albumin-to-globulin ratio and eosinophils. Our results were in line with a few previous studies.Citation48
CRP is a marker reflecting systemic inflammation, and elevated serum levels of CRP are associated with severe disease due to bacterial or viral infections. As for patients with COVID-19, higher CRP level was associated with increased risk of critical illness and mortality than lower CRP level.Citation43,Citation49–Citation51 Thus, CRP is recommended as a promising biomarker to predict prognosis of patients with COVID-19.Citation52 In a recent prospective cohort study, 1006 subjects with symptoms of COVID-19 were recruited, based on a SARS-CoV-2 PCR oropharyngeal swab test and measurement of CRP level at baseline.Citation53 The results showed that elevated CRP was associated with much higher probability of being hospitalized with an odds ratio of 4.21 (95% CI: 2.38–7.43); however, SARS-CoV-2 positivity alone was not associated with the probability of being hospitalized.Citation53 CRP level has a prognostic value in the population of patients with COVID-19-related symptoms. Lactic dehydrogenase is an enzyme involved in energy production, usually serves as an indicator of acute or chronic tissue damage, and is considered an inflammatory marker. Similar to CRP, lactic dehydrogenase was also observed to be inversely correlated with the respiratory performance indicator of PaO2/FiO2.Citation50
Although lymphocyte count can serve as a predictor of disease progression,Citation34 NLR is a simpler and more reliable parameter of immune-inflammatory response, neuroendocrine stress, and illness severity than lymphocyte count.Citation54 NLR is also a well-established predictor for disease severity and mortality of patients with COVID-19.Citation51,Citation54,Citation55 Moreover, NLR together with CRP has been recognized as an effective and reliable predictor of severe COVID-19.Citation51
Plasma concentration of fibrinogen can increase rapidly under pathological conditions such as infection and inflammation.Citation56 In the current study, we found that high level of fibrinogen at admission significantly increased the risk of developing severe COVID-19. The results from a meta-analysis showed that the mean fibrinogen value at admission was significantly higher in patients with severe COVID-19 than in those with non-severe disease,Citation57 which suggests that high fibrinogen level can also serve as a marker of poor prognosis in patients with COVID-19.
Albumin level usually reflects an individual’s nutritional status, and low serum albumin level has been shown to be an independent risk factor of poor outcome in critically ill patients. An increased globulin level may represent a chronic inflammatory response in a patient. Thus, the albumin-to-globulin ratio is a comprehensive indicator to reflect a person’s nutritional status as well as inflammatory response.Citation58 This ratio seems to be more accurate than each value separately and could help identify patients with COVID-19 who are at high risk of developing acute respiratory distress syndrome.Citation58 The increased ratio was associated with a 42% decreased risk of developing severe COVID-19 in our study. It was reported that a reduced albumin-to-globulin ratio is considered a risk factor of COVID-19 severity for patients with cancer.Citation44
Eosinophils are potent proinflammatory cells. Zhang et alCitation59 reported that eosinopenia was present in more than half the patients, and blood eosinophil counts correlated positively with lymphocyte counts in severe/non-severe patients with. The eosinopenia associated with COVID-19 is more likely a secondary phenomenon and whether the eosinopenia directly contributes to the disease course remains to be elucidated.Citation60 Eosinophil expansion and activation has been reported to be stimulated by type 2 cytokines, especially IL-5. In patients with severe COVID-19, the blockade of eosinophil activation likely contrasts harmful immunity.Citation61 Granulocytic markers, particularly neutrophils, eosinophils, and basophils, are enriched in active COVID-19 and can be used to distinguish between patients with mild and severe illness.Citation62 This granulocytic signature can be served as a biomarker to identify COVID-19 and its severity.Citation62
Serum urea is a biomarker of dehydration and the main parameter reflecting kidney function. Serum urea was found to be an independent factor regarding the need for oxygen therapy in patients with COVID-19.Citation63 Hachim et al found that urea levels (>6.5 mmol/L) could positively predict ICU admission in patients with COVID-19.Citation64 SARS-CoV-2 infection can lead to passive reabsorption of urea by activating the renin-angiotensin-aldosterone system.Citation65 Thus, serum urea is recommended as a simple but key biomarker for detecting severe COVID-19 accompanied by dehydration or renal failure.Citation63
Our study has some limitations. First, this is a retrospective cohort study and data were collected from the medical record system in a real-world setting; therefore, inherent bias could not be avoided given the study design. However, we mainly studied the objective indicators associated with fatal outcome in patients with COVID-19. Hence, our results could withstand scrutiny. Second, patients with severe COVID-19 at admission were excluded. Although we enrolled about 50% patients with confirmed COVID-19 in Hunan province mainly from the scheduled hospitals during the pandemic in China, selection bias could not be avoided. Third, use of drugs such as glucocorticoids are reported to affect the NLR valueCitation66 to an extent. However, we did not factor the effect of medication in the current study, because it is difficult to obtain these details from patients and ensure its accuracy. Nevertheless, the enrolled patients with COVID-19 were from the same region in Hunan province, and we believe that this relatively large sample will provide more insights into the etiology of progression to severe COVID-19.
Conclusion
We constructed an early predictive nomogram model by incorporating age and several laboratory indicators such as CRP, fibrinogen, lactic dehydrogenase, NLR, urea, albumin-to-globulin ratio, and eosinophil count that could reflect the basic condition of patients with COVID-19 at admission. Our model has been effectively validated and can be conveniently employed to predict the risk of developing severe COVID-19 in a patient, thereby helping to identify patients with a high risk of developing severe COVID-19 at an early stage.
Acknowledgments
This study was granted by Hunan Province Enterprise Science and Technology Commissioner Program (No. 2021GK5005).
Disclosure
The authors declare no conflicts of Interest.
References
- Wu Z, McGoogan JM. Characteristics of and important lessons from the coronavirus disease 2019 (COVID-19) outbreak in China: summary of a report of 72 314 cases from the Chinese center for disease control and prevention. JAMA. 2020;323(13):1239–1242. doi:10.1001/jama.2020.2648
- Lau H, Khosrawipour V, Kocbach P, et al. The positive impact of lockdown in Wuhan on containing the COVID-19 outbreak in China. J Travel Med. 2020;27(3). doi:10.1093/jtm/taaa037.
- Xu W, Wu J, Cao L. COVID-19 pandemic in China: context, experience and lessons. HPT. 2020;9(4):639–648. doi:10.1016/j.hlpt.2020.08.006
- Ahrenfeldt LJ, Nielsen CR, Möller S, Christensen K, Lindahl-Jacobsen R. Burden and prevalence of risk factors for severe COVID-19 in the ageing European population - a SHARE-based analysis. Zeitschrift fur Gesundheitswissenschaften. 2021;1–10. doi:10.1007/s10389-021-01537-7
- Hsiang W, Forman H, Jain S, et al. COVID-19 testing capabilities at urgent care centers in states with greatest disease burden. F1000Res. 2020;9:328. doi:10.12688/f1000research.23203.2
- Yang J, Chen X, Deng X, et al. Disease burden and clinical severity of the first pandemic wave of COVID-19 in Wuhan, China. Nat Commun. 2020;11(1):5411. doi:10.1038/s41467-020-19238-2
- Wu F, Zhao S, Yu B, et al. A new coronavirus associated with human respiratory disease in China. Nature. 2020;579(7798):265–269. doi:10.1038/s41586-020-2008-3
- Nittari G, Pallotta G, Amenta F, Tayebati SK. Current pharmacological treatments for SARS-COV-2: a narrative review. Eur J Pharmacol. 2020;882:173328. doi:10.1016/j.ejphar.2020.173328
- García LF. Immune response, inflammation, and the clinical spectrum of COVID-19. Front Immunol. 2020;11:1441. doi:10.3389/fimmu.2020.01441
- Gutiérrez-Chamorro L, Riveira-Muñoz E, Barrios C, et al. SARS-CoV-2 infection modulates ace2 function and subsequent inflammatory responses in swabs and plasma of COVID-19 patients. Viruses. 2021;13(9):1715. doi:10.3390/v13091715
- Chang D, Lin M, Wei L, et al. Epidemiologic and clinical characteristics of novel coronavirus infections involving 13 patients outside Wuhan, China. JAMA. 2020;323(11):1092–1093. doi:10.1001/jama.2020.1623
- Chen N, Zhou M, Dong X, et al. Epidemiological and clinical characteristics of 99 cases of 2019 novel coronavirus pneumonia in Wuhan, China: a descriptive study. Lancet. 2020;395(10223):507–513. doi:10.1016/S0140-6736(20)30211-7
- Grasselli G, Zangrillo A, Zanella A, et al. Baseline characteristics and outcomes of 1591 patients infected with SARS-CoV-2 admitted to ICUs of the Lombardy Region, Italy. JAMA. 2020;323(16):1574–1581. doi:10.1001/jama.2020.5394
- Baj J, Karakuła-Juchnowicz H, Teresiński G, et al. COVID-19: specific and non-specific clinical manifestations and symptoms: the current state of knowledge. J Clin Med. 2020;9(6):1753. doi:10.3390/jcm9061753
- Kim SY, Kim DW. Does the clinical spectrum of coronavirus disease 2019 (COVID-19) show regional differences?. Clin Exp Otorhinolaryngol. 2020;13(2):83–84. doi:10.21053/ceo.2020.00612
- Park JH, Jang W, Kim SW, et al. The clinical manifestations and chest computed tomography findings of coronavirus disease 2019 (COVID-19) patients in China: a proportion meta-analysis. Clin Exp Otorhinolaryngol. 2020;13(2):95–105. doi:10.21053/ceo.2020.00570
- Parohan M, Yaghoubi S, Seraji A, Javanbakht MH, Sarraf P, Djalali M. Risk factors for mortality in patients with Coronavirus disease 2019 (COVID-19) infection: a systematic review and meta-analysis of observational studies. Aging Male. 2020;23(5):1416–1424. doi:10.1080/13685538.2020.1774748
- Zheng Z, Peng F, Xu B, et al. Risk factors of critical & mortal COVID-19 cases: a systematic literature review and meta-analysis. J Infect. 2020;81(2):e16–e25. doi:10.1016/j.jinf.2020.04.021
- Alizadehsani R, Alizadeh Sani Z, Behjati M, et al. Risk factors prediction, clinical outcomes, and mortality in COVID-19 patients. J Med Virol. 2021;93(4):2307–2320. doi:10.1002/jmv.26699
- Zhao C, Bai Y, Wang C, et al. Risk factors related to the severity of COVID-19 in Wuhan. Int J Med Sci. 2021;18(1):120–127. doi:10.7150/ijms.47193
- Attaway AH, Scheraga RG, Bhimraj A, Biehl M, Hatipoğlu U. Severe covid-19 pneumonia: pathogenesis and clinical management. BMJ. 2021;372:n436. doi:10.1136/bmj.n436
- Fadavi P, Houshyari M, Yousefi Kashi AS, et al. Review on the oncology practice in the midst of COVID-19 crisis: the challenges and solutions. APJCP. 2021;22(1):19–24. doi:10.31557/APJCP.2021.22.1.19
- Rakhsha A, Azghandi S, Taghizadeh-Hesary F. Decision on chemotherapy amidst covid-19 pandemic: a review and a practical approach from Iran. Infect Chemother. 2020;52(4):496–502. doi:10.3947/ic.2020.52.4.496
- Zhou Y, He Y, Yang H, et al. Development and validation a nomogram for predicting the risk of severe COVID-19: a multi-center study in Sichuan, China. PLoS One. 2020;15(5):e0233328. doi:10.1371/journal.pone.0233328
- Jee J, Foote MB, Lumish M, et al. Chemotherapy and COVID-19 outcomes in patients with cancer. Am J Clin Oncol. 2020;38(30):3538–3546. doi:10.1200/JCO.20.01307
- Várnai C, Palles C, Arnold R, et al. Mortality among adults with cancer undergoing chemotherapy or immunotherapy and infected with COVID-19. JAMA Netw Open. 2022;5(2):e220130. doi:10.1001/jamanetworkopen.2022.0130
- Akbari H, Taghizadeh-Hesary F, Bahadori M. Mitochondria determine response to anti-programmed cell death protein-1 (anti-PD-1) immunotherapy: an evidence-based hypothesis. Mitochondrion. 2022;62:151–158. doi:10.1016/j.mito.2021.12.001
- Taghizadeh-Hesary F, Akbari H, Bahadori M. Anti-mitochondrial therapy: a potential therapeutic approach in oncology. Preprints; 2022:2022010171.
- Chamoto K, Chowdhury PS, Kumar A, et al. Mitochondrial activation chemicals synergize with surface receptor PD-1 blockade for T cell-dependent antitumor activity. Proc Natl Acad Sci U S A. 2017;114(5):E761–E770. doi:10.1073/pnas.1620433114
- Tan L, Wang Q, Zhang D, et al. Lymphopenia predicts disease severity of COVID-19: a descriptive and predictive study. Signal Transduct Target Ther. 2020;5(1):33. doi:10.1038/s41392-020-0148-4
- Montiel-Cervantes LA, Medina G, Pilar Cruz-Domínguez M, et al. Poor survival in covid-19 associated with lymphopenia and higher neutrophile-lymphocyte ratio. IMAJ. 2021;23(3):153–159.
- Shariat SF, Capitanio U, Jeldres C, Karakiewicz PI. Can nomograms be superior to other prediction tools? BJU Int. 2009;103(4):492–497. doi:10.1111/j.1464-410X.2008.08073.x
- Yang L, Gu D, Wei J, et al. A radiomics nomogram for preoperative prediction of microvascular invasion in hepatocellular carcinoma. Liver Cancer. 2019;8(5):373–386. doi:10.1159/000494099
- Liu S, Luo H, Lei Z, et al. A nomogram predicting severe COVID-19 based on a large study cohort from China. Am J Emerg Med. 2021;50:218–223. doi:10.1016/j.ajem.2021.08.018
- Nguyen Y, Corre F, Honsel V, et al. A nomogram to predict the risk of unfavourable outcome in COVID-19: a retrospective cohort of 279 hospitalized patients in Paris area. Ann Med. 2020;52(7):367–375. doi:10.1080/07853890.2020.1803499
- Zhang Y, Wu L, Yang J, Zhou C, Liu Y, Nomogram-Based A. Prediction for severe pneumonia in patients with coronavirus disease 2019 (COVID-19). Infect Drug Resist. 2020;13:3575–3582. doi:10.2147/IDR.S261725
- Zeng Z, Ma Y, Zeng H, et al. Simple nomogram based on initial laboratory data for predicting the probability of ICU transfer of COVID-19 patients: multicenter retrospective study. J Med Virol. 2021;93(1):434–440. doi:10.1002/jmv.26244
- Chen H, Chen R, Yang H, et al. Development and validation of a nomogram using on admission routine laboratory parameters to predict in-hospital survival of patients with COVID-19. J Med Virol. 2021;93(4):2332–2339. doi:10.1002/jmv.26713
- Xia Y, Zhang Y, Yuan S, et al. A nomogram to early predict isolation length for non-severe COVID-19 patients based on laboratory investigation: a multicenter retrospective study in Zhejiang Province, China. Clin Chim Acta. 2021;512:49–57. doi:10.1016/j.cca.2020.11.019
- Wei PF.National Health Commission. Diagnosis and treatment protocol for novel coronavirus pneumonia (Trial version 7). Chin Med J. 2020;133(9):1087–1095. doi:10.1097/CM9.0000000000000819
- Chen S, Sun H, Heng M, et al. Factors predicting progression to severe COVID-19: a competing risk survival analysis of 1753 patients in community isolation in Wuhan, China. Eng. 2021. doi:10.1016/j.eng.2021.07.021
- Yang Y, Zhu XF, Huang J, et al. Nomogram for prediction of fatal outcome in patients with severe COVID-19: a multicenter study. Mil Med Res. 2021;8(1):21. doi:10.1186/s40779-021-00315-6
- Cai L, Zhou X, Wang M, et al. Predictive nomogram for severe covid-19 and identification of mortality-related immune features. J Allergy Clin Immunol Pract. 2021;9(1):177–184.e173. doi:10.1016/j.jaip.2020.10.043
- Kaeuffer C, Le Hyaric C, Fabacher T, et al. Clinical characteristics and risk factors associated with severe COVID-19: prospective analysis of 1045 hospitalised cases in North-Eastern France, March 2020. Euro surveillance. 2020;25(48). doi:10.2807/1560-7917.ES.2020.25.48.2000895.
- Baker SA, Kwok S, Berry GJ, Montine TJ. Angiotensin-converting enzyme 2 (ACE2) expression increases with age in patients requiring mechanical ventilation. PLoS One. 2021;16(2):e0247060. doi:10.1371/journal.pone.0247060
- Pinto BGG, Oliveira AER, Singh Y, et al. ACE2 expression is increased in the lungs of patients with comorbidities associated with severe COVID-19. J Infect Dis. 2020;222(4):556–563. doi:10.1093/infdis/jiaa332
- Shamsoddin E. Can medical practitioners rely on prediction models for COVID-19? A systematic review. Evid Based Dent. 2020;21(3):84–86. doi:10.1038/s41432-020-0115-5
- Gong J, Ou J, Qiu X, et al. A tool for early prediction of severe coronavirus disease 2019 (COVID-19): a multicenter study using the risk nomogram in Wuhan and Guangdong, China. Clin Infect Dis. 2020;71(15):833–840. doi:10.1093/cid/ciaa443
- Smilowitz NR, Kunichoff D, Garshick M, et al. C-reactive protein and clinical outcomes in patients with COVID-19. Eur Heart J. 2021;42(23):2270–2279. doi:10.1093/eurheartj/ehaa1103
- Poggiali E, Zaino D, Immovilli P, et al. Lactate dehydrogenase and C-reactive protein as predictors of respiratory failure in CoVID-19 patients. Clin Chim Acta. 2020;509:135–138. doi:10.1016/j.cca.2020.06.012
- Xu R, Cui J, Hu L, et al. Development and validation of a simplified nomogram predicting individual critical illness of risk in COVID-19: a retrospective study. J Med Virol. 2021;93(4):1999–2009. doi:10.1002/jmv.26551
- Sahu BR, Kampa RK, Padhi A, Panda AK. C-reactive protein: a promising biomarker for poor prognosis in COVID-19 infection. Clin Chim Acta. 2020;509:91–94. doi:10.1016/j.cca.2020.06.013
- Boyesen EO, Balsby IM, Henriksen M, et al. Triage strategies based on C-reactive protein levels and SARS-CoV-2 tests among individuals referred with suspected COVID-19: a prospective cohort study. J Clin Med. 2021;11(1):201. doi:10.3390/jcm11010201
- Zahorec R, Hulin I, Zahorec P. Rationale use of neutrophil-to-lymphocyte ratio for early diagnosis and stratification of COVID-19. Bratisl Lek Listy. 2020;121(7):466–470. doi:10.4149/BLL_2020_077
- Li X, Liu C, Mao Z, et al. Predictive values of neutrophil-to-lymphocyte ratio on disease severity and mortality in COVID-19 patients: a systematic review and meta-analysis. Critical Care. 2020;24(1):647. doi:10.1186/s13054-020-03374-8
- Luyendyk JP, Schoenecker JG, Flick MJ. The multifaceted role of fibrinogen in tissue injury and inflammation. Blood. 2019;133(6):511–520. doi:10.1182/blood-2018-07-818211
- Nugroho J, Wardhana A, Mulia EP, et al. Elevated fibrinogen and fibrin degradation product are associated with poor outcome in COVID-19 patients: a meta-analysis. Clin Hemorheol Microcirc. 2021;77(2):221–231. doi:10.3233/CH-200978
- Feketea GM, Vlacha V. The diagnostic significance of usual biochemical parameters in coronavirus disease 19 (COVID-19): albumin to globulin ratio and CRP to albumin ratio. Front med. 2020;7:566591. doi:10.3389/fmed.2020.566591
- Zhang J-J, Dong X, Cao Y-Y, et al. Clinical characteristics of 140 patients infected with SARS-CoV-2 in Wuhan, China. Allergy. 2020;75(7):1730–1741. doi:10.1111/all.14238
- Xie G, Ding F, Han L, Yin D, Lu H, Zhang M. The role of peripheral blood eosinophil counts in COVID-19 patients. Allergy. 2021;76(2):471–482. doi:10.1111/all.14465
- Pala D, Pistis M. Anti-IL5 drugs in COVID-19 patients: role of eosinophils in SARS-CoV-2-induced immunopathology. Front Pharmacol. 2021;12:622554. doi:10.3389/fphar.2021.622554
- Vitte J, Diallo AB, Boumaza A, et al. A granulocytic signature identifies COVID-19 and its severity. J Infect Dis. 2020;222(12):1985–1996. doi:10.1093/infdis/jiaa591
- Goto N, Wada Y, Ikuyama Y, et al. The usefulness of a combination of age, body mass index, and blood urea nitrogen as prognostic factors in predicting oxygen requirements in patients with coronavirus disease 2019. J Infect Chemother. 2021;27(12):1706–1712. doi:10.1016/j.jiac.2021.08.009
- Hachim MY, Hachim IY, Naeem KB, Hannawi H, Salmi IA, Hannawi S. D-dimer, troponin, and urea level at presentation with COVID-19 can predict ICU admission: a single centered study. Front med. 2020;7:585003. doi:10.3389/fmed.2020.585003
- Cheng Y, Luo R, Wang K, et al. Kidney disease is associated with in-hospital death of patients with COVID-19. Kidney Int. 2020;97(5):829–838. doi:10.1016/j.kint.2020.03.005
- Bedel C, Korkut M. Neutrophil-to-lymphocyte ratio and Covid-19. Shock. 2021;56(5):874. doi:10.1097/SHK.0000000000001712