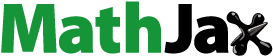
Abstract
Adequate control of comorbidity has long been recognized as a critical challenge in clinical epidemiology. Comorbidity scales reduce information about coexistent disease to a single index that is easy to comprehend and statistically efficient. These are the main advantages of an index over incorporating each disease into an analysis as an individual variable. Many study populations have a low prevalence of subjects with high comorbidity scores, so it is common to combine subjects with some score above a threshold into a single open-ended category. This paper examines the impact of collapsing comorbidity scores into these categories. It shows analytically and by synthetic example that collapsing the high-end categories of a comorbidity scale changes the pattern of effect of comorbidity. Furthermore, collapsing the high-end categories biases analyses that control for comorbidity as a confounder or analyze modification of an exposure’s effect by comorbidity. Each of these results specific to comorbidity scoring derives from more general epidemiologic principles. The appeal of collapsing categories to facilitate interpretation and statistical analysis may be offset by misleading results. Analysts should assure the uniformity of outcome risk in collapsed categories, informed by judgment and possibly statistical testing, or use analytic methods, such as restriction or spline regression, which can achieve similar goals without sacrificing the validity of results.
Introduction
A recent US National Institute on Aging Task Force defined comorbidity as “the co-occurrence of preexisting age-related health conditions (eg, disability, anemia, impairments, urinary incontinence) or diseases (eg, diabetes, heart disease, hypertension) in reference to an index disease (eg, cancer, Parkinson’s disease, diabetes).”Citation1 Adequate measurement and analytic control of comorbidity has long been recognized as a critical challenge in clinical epidemiology.Citation2 The aforementioned task force has reviewed the methodology of measurement of comorbidity,Citation3 including the nosology of disease classificationCitation4 and strategies to include disease severity in comorbidity scales.Citation5
Collapsing comorbid diseases into a single scale provides an index that is easy to comprehend and statistically efficient, which are the main advantages of an index over incorporating each disease into an analysis as an individual variable.Citation6 A simple sum of the number of comorbid diseases treats each disease equivalently, thereby ignoring differences in the severity of the component diseases and differences in the severity of the disease state in different patients. Weighting schemes have been proposed and implemented to address each of these shortcomings.Citation5,Citation7 Whether summing diseases included in the index or weighting them by severity, all comorbidity schemes inevitably misclassify study subjects with respect to the idealized true scale of comorbidity.Citation3 The impact of this misclassification on the analytic results depends on whether comorbidity is the exposure of interest, study outcome, a confounder, or modifier.Citation3
In most study populations, the prevalence of subjects with high comorbidity scores is low. It is common, therefore, to combine subjects with some score above a threshold into a single open-ended category. For example, the Charlson Index can theoretically range from 0 to 33 but was collapsed into categories of 0, 1–2, 3–4, and ≥5 in its initial presentation.Citation7 Similar examples, particularly examples of collapsing the scores in the highest categories, are easy to find, even in this author’s own work.Citation8 The rationale for collapsing these categories is the same as the rationale for using an index of comorbid diseases: ease of comprehension and statistical efficiency. The effect of collapsing these categories is also the same as the effect of collapsing disparate comorbid diseases: introduction of classification errors. In this paper, we show analytically and by synthetic example that collapsing the high-end categories of a comorbidity scale changes the estimate(s) of effect(s) of comorbidity and biases analyses that control for comorbidity as a confounder or analyze modification of an exposure’s effect by comorbidity.
Methods and results
To depict the bias introduced by collapsing categories of a comorbidity scale, we created a scale with a strictly monotonically increasing risk of the outcome (ri) with each increase in the ordinal comorbidity scale (indexed by i), and a strictly monotonically decreasing prevalence (pi) of the comorbidity value with each increase in the ordinal comorbidity scale. depicts this synthesized data. While the data are synthetic, we note that the risks of an outcome in the scale categories and the prevalence of the scale categories correspond well with values one might observe. For example, one might anticipate similar data if the population was an older population (say 70-years-old and older), if the index of comorbidity was the Charlson Index, and the outcome was a three-year risk of death.
Table 1 Depiction of the prevalence of comorbidity index categories (pi) and the risk of an outcome (ri) within the categories
Collapsing categories changes the estimate of comorbidity’s effect
The prevalence of the comorbidity categories decreases as the ordinal value increases. The prevalence of comorbidity category 4 is only 5%. In many data sets, the number of persons with this value would be small, and the number of cases of some outcome within that category even smaller. Analysts might be tempted to collapse category i = 4 with category i = 3, for example, to avoid sparse data problems or to improve the precision of the estimate of association in the highest comorbidity category. The effect of this collapse is to set the risk for the combined category to a weighted average of the two individual categories. More generally, collapsing a set of the upper-end categories ranging from i = v to the maximum (i = 4, in this example) generates a weighted average risk:
depicts the risk ratios (RRC =x vs C = 0) estimated from the synthetic data when the high-end categories are collapsed together. The collapsed categories range from some value v, which can equal 1, 2, 3, or 4 to the maximum (4, in this example). Setting v = 4 therefore corresponds to the case in which there is no collapse. Note that collapsing categories does not introduce a bias; the estimate of risk and therefore risk ratio within each category is an accurate depiction of the effect in that category. With each additional combination, the risk in the highest category becomes more heavily weighted with the low-risk comorbidity categories because these low risk categories are more prevalent. When v = 1, which corresponds to a comparison of any comorbidity (collapsing categories 1 to 4) with no comorbidity (category i = 0), the risk ratio equals 12. This risk ratio is about five-fold lower than the risk ratio in the highest comorbidity category (i = 4, in which the risk ratio equals 60) and about five-fold higher than the risk ratio in the lowest category with any comorbidity (i = 1, in which the risk ratio equals 2.7). The risk ratio of 12 is not, in fact, a very good estimate of the effect of comorbidity in any of the most finely divided categories. Collapsing comorbidity categories can therefore diminish the ability to discern important patterns that are more apparent when categories are not collapsed.
Table 2 Risk ratios associating the presence of comorbidity, compared with the absence of comorbidity, with the outcome within collapsed categories (ranging from v to 4)
Collapsing categories biases the relative risk due to confounding by comorbidity toward the null
Comorbidity data are frequently collected to control for confounding by underlying health indications. That is, comorbid diseases are likely to be more prevalent among patients with high risk conditions (eg, another health indicator such as frailty or disability) and likely also to be related to the outcome under study (eg, all-cause mortality). A scale of comorbid disease is therefore often a potential confounder and a candidate for analytic adjustment.
To examine the effect of collapsing comorbid categories when the comorbidity scale is used for analytic adjustment, we postulated a second dichotomous variable (E indexed by k = 0 or 1 within categories of the comorbidity scale) whose association with the outcome is of primary interest. We assumed that the prevalence of E = 1 depends on the category of the comorbidity scale, as depicted in . We assumed, however, that the risk of the outcome did not depend on the category of E within strata of the comorbidity scale. That is, after adjustment for the most finely divided comorbidity categories, the risk ratio associating E = 1 compared with E = 0 would be null (RRE = 1 vs E = 0 = 1).
Table 3 Depiction of the prevalence of comorbidity index categories within categories of E (pi, k)
The crude risk in categories of E is the weighted average of the risks in , where now the weights correspond with the prevalence of comorbidity within category of E, as shown in . That is
The risk equals 0.125 in E = 1 and 0.032 in E = 0, which yields a crude RRE = 1 vs E = 0 of 3.90. The substantial departure of this crude risk ratio from the true null association is entirely due to confounding by comorbidiy. The relative risk due to confounding (RRc), which equals the ratio of the crude and adjusted estimates, provides a measure of the direction and magnitude of this confounding, and in this case RRc = 3.9/1 = 3.9. To resolve the confounding, one can calculate the standardized risk ratio (sRRE = 1 vs E = 0), where the standard weights equal the prevalence of the comorbidity categories in E = 1 (these weights are pi, 1). That is:
when comorbidity categories are collapsed, the standardized risk in the denominator uses the weighted average risk in the collapsed category (rv…4, 0, where the weights come from the unexposed group) and the sum of corresponding weights in the exposed category (sum from v to 4 of pi,1).
The resulting sRR is incompletely adjusted for confounding by comorbidity. depicts the sRRE = 1 vs E = 0 and RRc for this scenario, and in a second scenario with the true sRRE =1 vs E = 0 = 1.5. In both cases, collapsing the upper-end categories of the comorbidity scales yields incomplete control for confounding by comorbidity. The result is a bias of RRc toward the null, which can give rise to the appearance of an association between E and the outcome when the true association is null (scenario 1), can give rise to the appearance of a stronger association than is truly present (scenario 2), or can give rise to an underestimate of the true association if the true RRc < 1 and the true association between E and the outcome is causal.
Table 4 Depiction of the true sRRE =1 vs E = 0 and RRc (v = 4) and biased sRRE =1 vs E = 0 and RRc (v < 4) when comorbidity categories are collapsed
Collapsing categories biases estimates of interaction unpredictably
Some analyses examine the interaction between comorbid disease and a second variable. These analyses investigate whether the effect of the exposure depends on the comorbidity category. Often the analysis compares the effect of the exposure in those with the highest comorbidity category to the effect of the exposure in those with the lowest comorbidity category. For example, one might calculate the interaction contrast (IC),Citation9 which measures the departure of risk in those with the high risk category of the exposure (E = 1) and comorbidity (I = 4) from the risk expected given (a) the independent effect of the exposure in those without comorbidity (r0, 1 – r0, 0), (b) the independent effect of higher comorbidity in those without the exposure (r4, 0 – r0, 0), (c) the risk in those with the low risk category of the exposure (E = 0) and comorbidity (I = 0). This concept simplifies to the risk difference in those with high comorbidity less the risk difference in those without comorbidity. That is:
A second measure of interaction is the ratio of the risk ratios, which we will call effect measure modification (EMM). That is:
When the highest categories of comorbidity are collapsed, however, r4,1 will be replaced with rv…4,1 and r4,0 will be replaced with rv…4,0. The result is an unpredictable bias in the estimates of the interaction between the exposure and comorbidity. In scenario 1, the exposure has no effect, so ri,1 – ri,0 = 0 and ri,1/ri,0 = 1. Therefore, IC must equal 0 and EMM must equal 1. As depicted in , the collapsed categories (v < 4) all yield IC > 0 and EMM > 1, suggesting an interaction between E and comorbidity that does not exist. Furthermore, as v increases, the bias of IC decreases but the bias of EMM increases. In scenario 2, both the exposure and comorbidy affect the outcome. Collapsing the comorbidity categories can overestimate IC (when v = 3) or underestimate IC (when v ≤ 2). On the other hand, EMM is most overestimated in scenario 2 when v = 1.
Table 5 Depiction of the true IC and EMM (v = 4) and biased IC and EMM (v < 4) when comorbidity categories are collapsed
Discussion
The common practice of collapsing the highest categories of comorbidity into a single category has the advantages of increasing the prevalence of subjects in the highest category of comorbidity, thereby improving the ease of comprehension and the statistical efficiency of the analysis. These advantages, however, come at the price of misclassification of subjects. The impact of this misclassification depends on how the comorbidity variable is used in the analysis.
When comorbidity is an exposure or predictor of the outcome in the analysis, then the misclassification changes the pattern of the outcome response as a function of the “dose” of comorbidity. This result should be expected; miscategorization of dose – and in particular combining categories with dissimilar outcome risks – yields misleading dose-response patterns.Citation10 Better analytic solutions are to collapse only adjacent comorbidity categories with similar risksCitation6 or to use more sophisticated dose-response modeling, such as spline regression.Citation10 The similarity of risks in adjacent categories is best left to judgment, perhaps informed by statistical testing, because of the poor power to detect important differences by statistical testing alone.Citation11
When comorbidity is a candidate confounder in the analysis, then the misclassification biases the relative risk due to confounding toward the null (assuming independent and nondifferential classification errors). The result is residual confounding of the association between the exposure of interest and the outcome. This result should also be expected; independent and nondifferential misclassification of a confounder is known to yield residual confounding.Citation12 Importantly, misclassification resulting from crude categorization of even a covariate that has been well-measured on a continuous scale can result in substantial bias.Citation13 As above, better analytic solutions are to collapse only adjacent comorbidity categories with similar risks,Citation6 to use spline regression,Citation10,Citation14 or to include the comorbidity index as a single linear term in regression modeling.Citation14 Restricting the study sample to subjects with comorbidity scores below the threshold where category collapsing will improve comprehensibility and statistical efficiency is also an alternative, although this restriction may reduce the generalizability of study results.Citation15
When comorbidity is a candidate modifier in the analysis, then the misclassification can give rise to the appearance of interaction when no interaction exists, can mask true interaction, and can bias the estimate of interaction.Citation3 Different combinations of these possibilities may appear depending on whether interaction is assessed as departure from additive or multiplicative effects, both of which have been proposed as important considerations in the examination of comorbidity.Citation1,Citation4 This result should also be expected; independent and nondifferential misclassification of a modifier is known to affect analyses of interaction unpredictably.Citation12 For most analyses of interaction, the best analytic solution is to restrict the analysis and inference to a category of comorbidity with uniform risk for the outcome.
The appeal of collapsing categories of comorbidity to facilitate interpretation and statistical analysis is often offset by misleading results. At a minimum, analysts should assure the uniformity of outcome risk in collapsed categories before collapsing them. Often times, more appropriate analytic methods can achieve similar goals without sacrificing the validity of the study’s results.
Disclosure
The author reports no conflicts of interest in this work.
References
- YancikRErshlerWSatarianoWHazzardWCohenHJFerrucciLReport of the National Institute on Aging Task Force on ComorbidityJ Gerontol200762A275280
- FeinsteinARThe pre-therapeutic classification of co-morbidity in chronic diseaseJ Chron Dis197023455468
- LashTLMorVWielandDFerrucciLSatarianoWSillimanRAMethodology, design, and analytic techniques to address measurement of comorbid diseaseJ Gerontol200762A281285
- KarlamanglaATinettiMGuralnikJStudenskiSWetleTReubenDComorbidity in older adults: Nosology of impairment, diseases, and conditionsJ Gerontol200762A296300
- BoydCMWeissCOHalterJHanKCErshlerWBFriedLPFramework for evaluating disease severity measures in older adults with comorbidityJ Gerontol200762A286295
- SchneeweissSMaclureMUse of comorbidity scores for control of confounding in studies using administrative databasesInt J Epidemiol20002989189811034974
- CharlsonMEPompeiPAlesKLMacKenzieCRA new method of classifying prognostic comorbidity in longitudinal studies: development and validationJ Chron Dis1987403733833558716
- LashTLThwinSHortonNJGuadagnoliESillimanRAMultiple informants: a new method to assess breast cancer patients’ comorbidityAm J Epidemiol200315724925712543625
- GreenlandSLashTLRothmanKJConcepts of interactionRothmanKJGreenlandSLashTLModern Epidemiology3rd editionPhiladelphia, PALippincott, Williams and Wilkins2008
- GreenlandSDose-response and trend analysis in epidemiology: alternatives to categorical analysisEpidemiology199563563657548341
- GreenlandSRothmanKJIntroduction to stratified analysisRothmanKJGreenlandSLashTLModern Epidemiology3s editionPhiladelphia, PALippincott, Williams and Wilkins2008
- GreenlandSThe effect of misclassification in the presence of covariatesAm J Epidemiol19801125645697424903
- BrennerHA potential pitfall in control of covariates in epidemiologic studiesEpidemiology1997968719430271
- BrennerHBlettnerMControlling for continuous confounders in epidemiologic researchEpidemiology199784294349209859
- CharlsonMEHorwitzRIApplying results of randomised trials to clinical practice: Impact of losses before randomizationBr Med J1984289128112846437520