Abstract
Aim: To determine whether dynamic DNA methylation changes in the human placenta can be used to predict gestational age. Materials & methods: Publicly available placental DNA methylation data from 12 studies, together with our own dataset, using Illumina Infinium Human Methylation BeadChip arrays. Results & conclusion: We developed an accurate tool for predicting gestational age of placentas using 62 CpG sites. There was a higher predicted gestational age for placentas from early onset preeclampsia cases, but not term preeclampsia, compared with their chronological age. Therefore, early onset preeclampsia is associated with placental aging. Gestational age acceleration prediction from DNA methylation array data may provide insight into the molecular mechanisms of pregnancy disorders.
Keywords::
The correlation between the chronological and DNA methylation gestational age of each placenta in the (A) training dataset and the (B) validation dataset. (C) A heatmap visualizing the gradual changes in DNA methylation in each of the 62 CpG sites (rows) across gestation in all samples (columns). The samples have been ordered by increasing gestational age and the probes have been ordered by the increasing magnitude of correlation with gestational age. The dataset heatmap represents the origin of each sample.
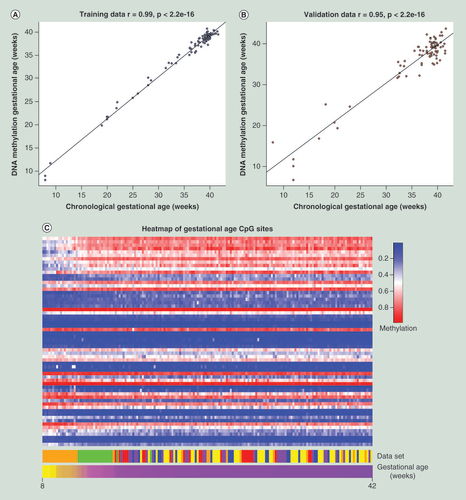
Placentas from pregnancies complicated by early-onset PE (A) show a higher DNAm GA compared with their Chron GA. (B) Placentas from late-onset PE do not show any difference between the chronological and DNA methylation gestational age. Error bars are represented as standard deviations.
Chron GA: Chronological gestational age; DNAm GA: DNA methylation gestational age.
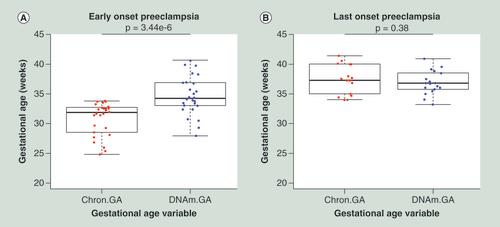
DNA methylation is a heritable epigenetic process that can regulate important genetic mechanisms and processes such as gene expression, X chromosome inactivation (XCI) [Citation1], cellular identity [Citation2] and genomic imprinting [Citation3]. DNA methylation is the covalent attachment of a methyl group to a cytosine ring by a DNA methyl transferase [Citation4]. In this article we focus on cytosine-5 DNA methylation within CpG dinucleotides as opposed to other methylated cytosines such as CHG and CHH. Recently, DNA methylation levels for 353 CpG sites have been used to measure the epigenetic age, defined as the predicted age by DNA methylation, of a variety of human tissues, which has a high correlation (r = 0.92) with the actual age [Citation5]. Moreover, another study found that accelerated aging [Citation5], defined as the difference between the estimated epigenetic age and the actual known age, is associated with maternal characteristics in pregnancy such as smoking, weight, BMI, selenium and cholesterol in peripheral blood [Citation6]. The placenta is unique compared with other tissues, with the exception of cancer tissues [Citation7,Citation8] and a human fetal fibroblast cell line (IMR90) [Citation9], in that it has been shown that it has low levels of genome-wide CpG methylation [Citation10,Citation11]. Despite this, overall placental genome CpG methylation has been observed to increase during gestation [Citation12]. However, precisely what DNA methylation changes occur during gestation and how these changes relate to pregnancy success is unknown.
Poor placental function, due to impaired placentation has been proposed to be a cause of preeclampsia (PE) [Citation13], which is characterized by high-maternal blood pressure and proteinuria [Citation14]. Adversities during pregnancy may cause epigenetic changes and altered fetal development outcomes [Citation15], which may be orchestrated by the placenta [Citation16]. Differential DNA methylation in the placenta has been shown to occur in pregnancy complications [Citation17] including PE [Citation18–21], gestational diabetes mellitus [Citation18,Citation22,Citation23] and intrauterine growth restriction [Citation24]. DNA methylation is critical for optimal placental and fetal development. For example, genomic imprinting regulates expression of IGF2 and H19, which are both required for proper placental development. Hypomethylation within the imprinting control region of IGF2 has been shown to be associated with reduced fetal growth [Citation25,Citation26] whereas loss of imprinting of H19 has been reported to be associated with PE and with small for gestational age infants [Citation27].
Previous studies have reported altered placental gene expression in human pregnancy complications including PE [Citation28–32], gestational diabetes mellitus [Citation31] and fetal growth restriction [Citation28,Citation33]. However, little is known whether changes in DNA methylation and gene expression overlap in the placenta. The relationship between global placental gene expression and DNA methylation is conflicting as one study has reported a general trend between the increase in DNA methylation and decrease in gene expression levels during gestation [Citation12], whereas another study using matched samples has reported no overlap between gene expression and DNA methylation changes between placentas from PE and uncomplicated pregnancies [Citation21]. In addition, genes within partially methylated domains in the term placenta have been reported to be repressed [Citation10].
In this study, we assembled a large dataset of publicly available placental tissue DNA methylation data, which has been measured using the Illumina Infinium HumanMethylation BeadChip arrays (Illumina). We sought to determine the precise changes in DNA methylation that occur in the placenta across gestation and determined whether DNA methylation data can be used to predict the gestational age of a placenta. Finally, we investigated what happens to the predicted gestational age in placentas from preeclamptic pregnancies. We hypothesized that the gestational age of the placenta can be estimated by its DNA methylation levels and that pregnancy complications such as PE would be characterized by accelerated placental aging. Our computational analysis of DNA methylation data reveals accurate predictions of the gestational age of the placenta. Moreover, gestational age prediction of the placenta may identify novel mechanisms in pregnancy complications, such as those associated with placental aging.
Materials & methods
Quantification of the DNA methylation levels
Quantification of the DNA methylation level of each CpG site that is annotated in either the Illumina Infinium HumanMethylation27 or 450 BeadChip (Illumina) was performed using standard techniques. The publicly available human placental datasets () were obtained from the Gene Expression Omnibus using the GEOquery Bioconductor package. Briefly, DNA methylation levels of each CpG site from each dataset were quantified by the β-value. The β-value is calculated by taking the ratio of the two fluorescent signals (methylated and unmethylated signals) in studies that provided the two fluorescent signals.
Differential methylation analysis
Differential methylation analysis was performed using the 450k datasets for which we had data for 469,017 probes. Normalization was performed using the Beta MIxture Quantile dilation (BMIQ) method [Citation34], which corrects for the probe design bias in the Illumina Infinium HumanMethylation450 BeadChip followed by quantile normalization. Since the data were from multiple datasets, batch effects were corrected using the ComBat function in the ChAMP Bioconductor package [Citation35,Citation36]. Multidimensional scaling plots of the 1000 most variable probes of the data were used to check for outliers. Sample sex was identified using the minfi package in which the median value of the β-values for probes that mapped uniquely for the X and Y chromosome, respectively, were determined [Citation37]. Differentially methylated CpG sites were identified using empirical Bayesian variance method in limma [Citation38]. The Bumphunter Bioconductor package was used to identify differentially methylated regions (DMRs) by running 1000 permutations of the data [Citation39]. We selected datasets (GSE44667, GSE46573, GSE52576, GSE54399, GSE57767 and GSE73375) that had used the Illumina Infinium HumanMethylation450 BeadChip array to assess sex differences in placental DNA methylation and differential methylation between PE and uncomplicated pregnancies (). In total, we had placental DNA methylation data for 70 preeclamptic and 62 uncomplicated pregnancies.
Gestational age prediction
We selected datasets for placental tissue samples that were publicly available and which contained individual gestational age information and were from healthy singleton pregnancies (GSE31781, GSE36829, GSE59274, GSE44667 and GSE74738) (). We also combined these datasets with our own dataset for 22 term placentas from uncomplicated pregnancies. Probes that were present in both the 27k and 450k array were kept, and probes that were annotated to the sex chromosomes were removed. Every sample in the training dataset contained β-values for 18,437 probes. Normalization was performed as described by Horvath [Citation5], using a modified version of the BMIQ [Citation34] method. This modified version of BMIQ rescales the Infinium II probes to the mean β-value of each probe in the largest dataset (GSE36829) prior to quantile normalization. Using the R package glmnet [Citation40], we regressed gestational age with the 18,437 probes. The elastic net regression or the α-parameter of glmnet was 0.5 and the minimum λ-value based on the training data was 0.6807. The elastic net model automatically selected 62 CpG sites, such that given the level of methylation of the 62 CpG sites, the gestational age of a placenta can be calculated.
Gestational age acceleration heritability
To determine the heritability of gestational age acceleration, which is defined as the difference between the chronological and predicted gestational age of a placenta we used Falconer’s formula (H2 = 2(cor(MZ)-cor(DZ))). Falconer’s formula was used to determine the broad sense heritability, which is the proportion of variance of gestational age acceleration as a result of genetic factors. The broad sense heritability was determined by using a dataset (GSE36642) that contained monozygotic (MZ) and dizygotic (DZ) twins of the same sex. First, the predicted gestational age of the twin placentas were determined as described above. The twin dataset was split into either MZ or DZ twins, and for each twin pair the gestational age acceleration was calculated. For each twin pair, each sibling was randomly selected as either twin 1 or twin 2 and the pearson correlation of gestational age acceleration was determined in both MZ and DZ twins. The correlations of both MZ and DZ twins were inputted into Falconer’s formula to determine the broad sense heritability.
Annotation of CpG sites
Annotation of all the CpG sites within the analysis of this study for the Illumina Infinium HumanMethylation27 and HumanMethylation450 BeadChip was performed using two annotation Bioconductor packages [Citation41,Citation42].
Results
Differential methylation in placentas from preeclamptic pregnancies
We searched Gene Expression Omnibus [Citation43] for DNA methylation datasets containing placental tissue that were measured on either the Illumina Infinium HumanMethylation27 BeadChip or Infinium HumanMethylation450 BeadChip. In total, we identified 387 placental tissue samples from 12 different datasets (). We selected only placentas assessed using the Illumina DNA methylation arrays, the most commonly used platform to quantify DNA methylation in the placenta. Here, we used publicly available placental DNA methylation data () to assess differential methylation between placentas from PE and uncomplicated pregnancies. We selected only datasets that used the Infinium HumanMethylation450 BeadChip for differential methylation analysis since most studies involving placental samples from women with PE had used this platform () and it contains the largest number of CpG sites available for analysis. When comparing 70 placentas from preeclamptic pregnancies and 62 placentas from term uncomplicated pregnancies we found that a total of 741 CpG sites (false discovery rate [FDR] <0.01) were differentially methylated (Supplementary Table 1). We then tested for DMRs and identified three DMRs in placentas from preeclamptic pregnancies, which overlapped the 5′ region of MARC2, FAM3B and TP53TG1.
Sex differences in DNA methylation
To identify whether sex differences also occur in the placental DNA methylome, we identified a total of 2898 differentially methylated CpG sites (FDR <0.01) between 35 male and 27 female placentas from uncomplicated singleton term pregnancies that had been analyzed using the Infinium HumanMethylation450 BeadChip (Supplementary Table 2). Of the 2898 CpG sites, 420 were located on autosomes, 2464 on the X chromosome and 14 on the Y chromosome. Although we are reporting Y chromosome CpG sites, we do not consider these CpG sites as differentially methylated between sexes. In addition, upon removing the Y chromosome from our analysis we did not observe a difference in the total number of differentially methylated X chromosome or autosomal CpG sites. We also identified a total of 396 DMRs between sexes (Supplementary Table 3). All of these were located on the X chromosome with 311 and 85 being hypermethylated in females and males, respectively. The 85 hypermethylated DMRs on the X chromosome in males did not overlap with any reported sex-biased genes [Citation44], with the exception of XIST, which is upregulated in females and is well known for its role in XCI in female mammalian somatic cells [Citation45]. The most statistically significant differentially methylated autosomal CpG site as defined by the empirical Bayesian variance method in limma between sexes was hypermethylated in females and was within exon 1 of TLE1, which is a marker of synovial sarcoma [Citation46]. Although TLE1 is expressed in the placenta, it has not been reported to be differentially expressed between fetal sexes in the placenta [Citation44,Citation47].
Gestational age calculator training dataset
Although a multitissue age predictor using DNA methylation data has been previously developed [Citation5], we set out to determine if DNA methylation can be used to predict the gestational age of the placenta. To develop our placental gestational age calculator, only placentas from healthy singleton pregnancies with individual gestational age information were included. Placental tissue samples from PE pregnancies were excluded from the training dataset to reduce potential confounding factors caused by the disease. In total, we used 170 placental tissue samples that had individual gestational age information to generate the gestational age calculator. The 170 placental tissue samples were taken from five publicly available datasets, along with our own generated data analyzing 22 term placentas (). Four of the six datasets were obtained using the Infinium HumanMethylation27 BeadChip and the other two on the Infinium HumanMethylation450 BeadChip, and the included datasets contained placental tissue samples that spanned 8–42 weeks gestation. We selected probes that were present in all six datasets and removed probes that were found on sex chromosomes, leaving a total of 18,437 probes (no missing data). We randomly assigned half of the 170 placental tissue samples to a training dataset, leaving the other 85 samples for validation.
Identifying & validating the gestational age calculator
Briefly, we first normalized the training data using the modified version of the BMIQ [Citation5,Citation34]. The mean β-value of each probe in the largest dataset (GSE3829) was used as the gold standard of the probes, similarly as previously described [Citation5], in the normalization step (Supplementary Table 4). A gold standard of the probes was used for normalization since the datasets were from two different microarray platforms and it rescaled the probes that were present in both microarray platforms. After normalization, we regressed the chronological gestational age against the 18,437 CpG sites using an elastic net penalized regression model [Citation40]. The model automatically selected 62 CpG sites (Supplementary Table 5) to predict the gestational age of a placenta. In the training dataset we found an extremely high correlation (r = 0.99, p < 2.2e-16) between the chronological and the predicted gestation age (A). In addition, the median absolute difference between the predicted and chronological gestational age in the training dataset was found to be 0.23 weeks. We then tested these 62 CpG sites in the validation dataset (B) and also found a high correlation between the chronological and predicted gestational age (r = 0.95, p < 2.2e-16). The median absolute difference in the validation dataset was 1.47 weeks and the root mean square error was 2.3. The heatmap (C) allows visualization of the CpG sites and shows changes in DNA methylation across gestation. Furthermore, the lack of vertical lines in the heatmap suggests that the CpG sites are robust against dataset effects. In order to further validate the 62 CpG sites, we predicted the gestational age of all remaining publicly available placental tissue samples from uncomplicated pregnancies that did not have individual gestational age information (Supplementary Table 6). Although these samples did not have individual gestational age information, we sought to determine if the predicted gestational age matched the labeled trimester of pregnancy for each sample. We found a concordance between the predicted gestational age and labeled trimester of pregnancy, which assured us that it is an accurate predictor of gestational age. Here in this study we refer to the predicted gestational age of each placenta as the DNA methylation gestational age (DNAm GA).
The 62 gestational clock CpG sites
The 62 gestational clock CpG sites can be characterized into two groups depending on the direction of their correlation with gestational age. Twenty-seven CpG sites were found to positively correlate and become hypermethylated with increasing gestational age whereas, the other 35 CpG sites negatively correlated and became hypomethylated with increasing gestational age.
Gestational age acceleration in placentas from preeclamptic pregnancies
Since differential DNA methylation occurs in placentas from PE compared with uncomplicated pregnancies [Citation17], we investigated if accurate prediction of gestation age can also be achieved in placentas from PE pregnancies. Two datasets (GSE44667 and GSE59274) contained individual gestational age information for placental tissue samples from PE pregnancies (26 early-onset PE and 18 late-onset PE). We then compared the chronological gestational age with the DNAm GA () and found that placentas from early-onset PE pregnancies (<34 weeks gestation) had a higher DNAm GA compared with their chronological gestational age (p = 3.44e-6, two-tailed, paired t-test). However, late-onset placentas from PE pregnancies (≥34 weeks gestation) did not show any significant difference between their chronological and DNAm GA (p = 0.38) indicating that late-onset PE does not affect placental aging. From here on, we refer to the difference between chronological and DNAm GA as gestational age acceleration, similar to what has been defined previously [Citation5]. One dataset (GSE36642) contained placentas from MZ and DZ twins with individual gestational age information, allowing the determination of gestational age acceleration heritability by calculating the broad-sense heritability using Falconer’s formula (H2 = 2(cor(MZ)-cor(DZ))). We conducted our analysis on gestational age acceleration heritability on twin samples of the same sex. The broad-sense heritability was used to determine the proportion of variance of gestational age acceleration as a result of genetic variation. Despite having a small sample size, we calculated the gestational age acceleration for each sample and determined the broad-sense heritability to be 57.2% in MZ and DZ twin pairs (Supplementary Figure 1).
Discussion
In this study, we investigated DNA methylation differences in the placenta across gestation and in different pregnancy outcomes. We found 34 genes that have been reported to be differentially expressed in placenta from PE compared with uncomplicated pregnancies [Citation30] to contain at least one differentially methylated CpG site (Supplementary Table 1). For example, there was a differentially methylated CpG site in the promoter of RAC1 (Supplementary Table 1), a member of the RAS superfamily [Citation48], which has been found to be upregulated in placenta from preeclamptic pregnancies [Citation30]. We also tested for DMRs in placentas from preeclamptic and uncomplicated pregnancies. We identified three DMRs, which overlapped the 5′ region of MARC2, FAM3B and TP53TG1. However, to our knowledge these genes have not been reported to be differentially expressed in placenta from women with PE.
The placenta has been implicated in a number of pregnancy complications, including preterm birth [Citation49]. Sex differences in pregnancy outcomes have also been reported, for example, women bearing a male fetus are at a 20% higher risk of preterm birth [Citation50–52]. The fetus and placenta are genetically identical [Citation53]. Therefore, it is reasonable to suggest that sex differences in outcomes may potentially be orchestrated by the placenta. Sex differences in placental gene expression have been previously reported [Citation44,Citation47] and in comparison to the placental sex-biased gene expression meta-analysis [Citation44], 20 genes (located on the X chromosome and upregulated in females) were found to contain at least one CpG site or DMR that was hypermethylated in females. However, this may be the result of XCI in females.
It is unclear why 85 X chromosome DMRs would be hypermethylated in males considering XCI occurs in females. Despite being hypermethylated in males, there are no reports of sex differences in expression of the genes in which these DMRs occur. Potentially they may regulate other sex-specific differentially expressed RNAs including ncRNAs or affect transcription factor binding. Further research is required to elucidate the precise mechanisms by which the 85 X chromosome hypermethylated DMRs in males act.
We therefore, found a lack of overlap with our differential DNA methylation analysis and two gene expression meta-analyses [Citation30,Citation44]. These findings were consistent with a previous study in which changes in gene expression and DNA methylation did not overlap from matched placental samples from PE and uncomplicated pregnancies [Citation21]. Furthermore, changes in DNA methylation within the promoters of genes do not always alter gene expression as reported for colon cancer [Citation54]. We also found little overlap between genes that have previously been reported to be sex biased in the placenta [Citation44] and our DNA methylation results. Interestingly, we did find 420 differentially methylated autosomal CpG sites and 85 X chromosome hypermethylated DMRs in males. However, due to the poor overlap with gene expression and the effect of XCI it is difficult to draw any conclusions on what the effect of the differences in DNA methylation that were observed had on gene expression.
The lack of overlap between DNA methylation and reported gene expression changes has several limitations. First, in comparison to the two gene expression meta-analyses, we analyzed far fewer samples and therefore may not have been statistically powered to detect small differences. Second, the Illumina DNA Methylation BeadChip arrays only assess approximately 2% of the CpG sites in the human genome and do not assess other methylated cytosine sites such as CHG and CHH. Therefore, we may not have captured the true landscape of DNA methylation in the placenta. Finally, the comparison between DNA methylation and gene expression was not in the same samples and therefore there may have been too much biological variability to detect any overlap. Despite these limitations, our findings suggest that CpG methylation in the placenta may not be a key regulator of gene expression and therefore may be more dependent on other epigenetic factors such as histone modifications, small RNA regulation or ncRNA changes. It has been reported that the placenta with the exception of the brain, has high levels of non-CpG methylation compared with other human tissues [Citation55], which may have a bigger influence on gene expression levels.
In this study, we identified 62 CpG sites, which together can be used to determine the gestational age of a placenta. Several limitations of our study for predicting gestational age do require discussion. First, the training dataset consisted of placentas from 8 to 42 weeks gestation with a bias of samples being from late third trimester. First and second trimester samples comprised only 11 and 9.5% of the training and validation data, respectively. This may have created some biases in the CpG sites chosen and may cause some inaccuracy in identifying the gestational age of placentas from first and second trimester. Second, in relation to gestational age acceleration heritability the twin dataset only contained 14 twin pairs. Therefore we may have not captured the true extent of gestational age heritability within this study. Future studies with large sample sizes of twin pairs are required to determine the true extent of the heritability of gestational age acceleration. In addition to gestational age prediction, we used first and second trimester placentas from terminated pregnancies. One limitation is that some of these placentas may have been from women destined to develop a pregnancy complication, which may have implications for our gestational age prediction. A possible approach to overcome this limitation is to use placental villi from chorionic villus sampling in ongoing pregnancies. Thereby, samples that were from complicated pregnancies could be excluded from the analysis. Unfortunately, to our knowledge there is no publicly available DNA methylation data on such samples that we could use to test our gestational age prediction.
Placentas from early-onset preeclamptic pregnancies were found to have a higher DNAm GA compared with their chronological gestational age. Using a twin dataset we were able to determine the broad-sense heritability of gestational age acceleration to be 57.2%. This finding suggests environmental factors also have an influence together with genetic factors on gestational age acceleration. Maternal lifestyle factors such as smoking [Citation56,Citation57] are known to alter DNA methylation levels in the placenta. Therefore, the maternal environment can affect the intrauterine environment, and thereby could influence gestational age acceleration in the placenta. Furthermore, other lifestyle factors such as BMI have been found to increase the epigenetic age in certain tissues such as the liver [Citation58]. It would therefore be important to investigate the effect of maternal lifestyle factors on gestational age acceleration as they may have implications for pregnancy success. Unfortunately these data are often not recorded for publicly available datasets. This limitation also applies to the exact nature of the twin placentas included in the publicly available data. For example, we do not know if the twin pregnancies had fused placentas or not. Although each individual twin is listed as having a separate placenta, it may be possible that some of the twin pregnancies had fused placentas and therefore the exact sampling sites could confound the data on heritability. Therefore, we suggest some caution in interpreting the heritability of gestational age acceleration analysis as we do not have full clinical details for the publicly available twin dataset (GSE36642).
Using our gestational age tool, we also provide an estimation of the gestational age of all remaining publicly available placenta samples from uncomplicated pregnancies for which gestational age information is not recorded as a resource to the scientific community (Supplementary Table 6). It has also been reported that the placental transcriptome is clearly distinct in PE compared with other pregnancy complications [Citation31]. It would therefore be of interest to determine if the placental gestational age acceleration observed in early-onset PE pregnancies also occurs in other pregnancy complications. Likewise, the gestational age acceleration observed in placentas from early- but not late-onset PE highlights potential differences in the etiology of the two diseases. Furthermore, gestational age acceleration may potentially reveal potential mechanisms in the development of early-onset PE and other pregnancy complications. First trimester chorionic villus samples could potentially be used to determine when accelerated placental aging first occurs. This may provide insight into the mechanisms and the association of placental aging and pregnancy complications such as early-onset PE. However, chorionic villus sampling is only used in some high-risk pregnancies but has a miscarriage risk, so tissue availability is limited.
Conclusion
In conclusion, we have identified 62 CpG sites that can be used to determine the DNAm GA of a placenta. Furthermore, we found evidence of placental aging in placentas from early-onset PE. Future studies are required to determine if gestational age acceleration is unique to early-onset PE or is common to other pregnancy complications. In addition, future research should also determine if gestational age acceleration or placental aging could be detected perhaps in maternal blood early in pregnancy in women who are destined to develop a pregnancy complication. Although, we found little overlap between DNA methylation and gene expression changes, further studies involving matched samples are required to confirm these findings.
Table 1. A description of the DNA methylation datasets containing placental tissue used in this study.
Aim
Preeclampsia is characterized by high-maternal blood pressure and proteinuria.
Differential placental gene expression is known to occur between preeclamptic and uncomplicated pregnancies.
Overall CpG methylation in the placenta has been shown to increase across gestation.
We sought to investigate DNA methylation changes in the placenta across gestation and pregnancy complications by using publicly available data.
Results
We identified a total of 741 differentially methylated CpG sites in the placenta between preeclampsia and uncomplicated pregnancies.
We developed an accurate tool using 62 CpG sites to predict the gestational age of a placenta.
Placentas from early-onset preeclampsia had a higher predicted gestational age compared with their chronological age.
Conclusion
Placentas from early-onset preeclampsia pregnancies are associated with placental aging.
Our gestational age prediction tool may offer important insight into the molecular mechanisms associated with pregnancy complications.
Author contributions
BT Mayne designed, conducted the study, analyzed and interpreted the data, and wrote the manuscript. SY Leemaqz analyzed the data, provided intellectual input into the manuscript. AK Smith was involved in data creation, provided critical discussion and intellectual input into the manuscript. J Breen, CT Roberts and T Bianco-Miotto were all involved in the study design, provided critical discussion and intellectual input into the manuscript. All authors read and approved the final manuscript.
Supplemental Information 1
Download PDF (40.3 KB)Supplemental Information 2
Download MS Excel (81.4 KB)Supplemental Information 3
Download MS Excel (286.8 KB)Supplemental Information 4
Download MS Excel (35.6 KB)Supplemental Information 5
Download MS Excel (558.7 KB)Supplemental Information 6
Download MS Excel (12.8 KB)Supplemental Information 7
Download MS Excel (13.9 KB)Acknowledgements
The authors would like to thank the generosity of all the individuals who were involved in the data creation of all datasets that were available for public analysis.
Supplementary data
To view the supplementary data that accompany this paper please visit the journal website at: https://www.tandfonline.com/doi/suppl/10.2217/3dp-2022-0019
Financial & competing interests disclosure
This project was funded in part by a National Health and Medical Research Council of Australia Project Grant (GNT1059120) awarded to CT Roberts and T Bianco-Miotto, and support from the NIH R01MD009064 awarded to AK Smith. BT Mayne is supported by an Australian Postgraduate Award. CT Roberts is supported by an National Health and Medical Research Council of Australia Senior Research Fellowship GNT1020749. The authors have no other relevant affiliations or financial involvement with any organization or entity with a financial interest in or financial conflict with the subject matter or materials discussed in the manuscript apart from those disclosed.
No writing assistance was utilized in the production of this manuscript.
Additional information
Funding
References
- Sharp AJ , StathakiE , MigliavaccaEet al. DNA methylation profiles of human active and inactive X chromosomes . Genome Res.21 ( 10 ), 1592 – 1600 ( 2011 ).
- Novak P , StampferMR , Munoz-RodriguezJLet al. Cell-type specific DNA methylation patterns define human breast cellular identity . PLoS ONE7 ( 12 ), e52299 ( 2012 ).
- Paulsen M , Ferguson-SmithAC . DNA methylation in genomic imprinting, development, and disease . J. Pathol.195 ( 1 ), 97 – 110 ( 2001 ).
- Robertson KD . DNA methylation and human disease . Nat. Rev. Genet.6 ( 8 ), 597 – 610 ( 2005 ).
- Horvath S . DNA methylation age of human tissues and cell types . Genome Biol.14 ( 10 ), R115 ( 2013 ).
- Simpkin AJ , HemaniG , SudermanMet al. Prenatal and early life influences on epigenetic age in children: a study of mother-offspring pairs from two cohort studies . Hum. Mol. Genet.25 ( 1 ), 191 – 201 ( 2016 ).
- Hon GC , HawkinsRD , CaballeroOLet al. Global DNA hypomethylation coupled to repressive chromatin domain formation and gene silencing in breast cancer . Genome Res.22 ( 2 ), 246 – 258 ( 2012 ).
- Berman BP , WeisenbergerDJ , AmanJFet al. Regions of focal DNA hypermethylation and long-range hypomethylation in colorectal cancer coincide with nuclear lamina-associated domains . Nat. Genet.44 ( 1 ), 40 – 46 ( 2012 ).
- Lister R , PelizzolaM , DowenRHet al. Human DNA methylomes at base resolution show widespread epigenomic differences . Nature462 ( 7271 ), 315 – 322 ( 2009 ).
- Schroeder DI , BlairJD , LottPet al. The human placenta methylome . Proc. Natl Acad. Sci. USA110 ( 15 ), 6037 – 6042 ( 2013 ).
- Schultz MD , HeY , WhitakerJWet al. Human body epigenome maps reveal noncanonical DNA methylation variation . Nature523 ( 7559 ), 212 – 216 ( 2015 ).
- Novakovic B , YuenRK , GordonLet al. Evidence for widespread changes in promoter methylation profile in human placenta in response to increasing gestational age and environmental/stochastic factors . BMC Genomics12529 ( 2011 ).
- Redman CW , SargentIL , StaffAC . IFPA Senior Award Lecture: making sense of pre-eclampsia – two placental causes of preeclampsia?Placenta35 ( Suppl. ) S20 – S25 ( 2014 ).
- Williams D , CraftN . Pre-eclampsia . BMJ345 , e4437 ( 2012 ).
- Monk C , SpicerJ , ChampagneFA . Linking prenatal maternal adversity to developmental outcomes in infants: the role of epigenetic pathways . Dev. Psychopathol.24 ( 4 ), 1361 – 1376 ( 2012 ).
- Novakovic B , SafferyR . The ever growing complexity of placental epigenetics – role in adverse pregnancy outcomes and fetal programming . Placenta33 ( 12 ), 959 – 970 ( 2012 ).
- Bianco-Miotto T , MayneB , BuckberryS , BreenJ , Rodriguez LopezC , RobertsC . Recent progress towards understanding the role of DNA methylation in human placental development . Reproduction152 ( 1 ), R23 – R30 ( 2016 ).
- Liu L , ZhangX , RongCet al. Distinct DNA methylomes of human placentas between pre-eclampsia and gestational diabetes mellitus . Cell. Physiol. Biochem.34 ( 6 ), 1877 – 1889 ( 2014 ).
- Anton L , BrownAG , BartolomeiMS , ElovitzMA . Differential methylation of genes associated with cell adhesion in preeclamptic placentas . PLoS ONE9 ( 6 ), e100148 ( 2014 ).
- Blair JD , YuenRK , LimBK , McfaddenDE , Von DadelszenP , RobinsonWP . Widespread DNA hypomethylation at gene enhancer regions in placentas associated with early-onset pre-eclampsia . Mol. Hum. Reprod.19 ( 10 ), 697 – 708 ( 2013 ).
- Chu T , BunceK , ShawPet al. Comprehensive analysis of preeclampsia-associated DNA methylation in the placenta . PLoS ONE9 ( 9 ), e107318 ( 2014 ).
- Finer S , MathewsC , LoweRet al. Maternal gestational diabetes is associated with genome-wide DNA methylation variation in placenta and cord blood of exposed offspring . Hum. Mol. Genet.24 ( 11 ), 3021 – 3029 ( 2015 ).
- Ruchat SM , HoudeAA , VoisinGet al. Gestational diabetes mellitus epigenetically affects genes predominantly involved in metabolic diseases . Epigenetics8 ( 9 ), 935 – 943 ( 2013 ).
- Hillman SL , FinerS , SmartMCet al. Novel DNA methylation profiles associated with key gene regulation and transcription pathways in blood and placenta of growth-restricted neonates . Epigenetics10 ( 1 ), 50 – 61 ( 2015 ).
- Jacob KJ , RobinsonWP , LefebvreL . Beckwith–Wiedemann and Silver–Russell syndromes: opposite developmental imbalances in imprinted regulators of placental function and embryonic growth . Clin. Genet.84 ( 4 ), 326 – 334 ( 2013 ).
- Fowden AL , SibleyC , ReikW , ConstanciaM . Imprinted genes, placental development and fetal growth . Horm. Res.65 ( Suppl. 3 ), 50 – 58 ( 2006 ).
- Yu L , ChenM , ZhaoDet al. The H19 gene imprinting in normal pregnancy and pre-eclampsia . Placenta30 ( 5 ), 443 – 447 ( 2009 ).
- Guo L , TsaiSQ , HardisonNEet al. Differentially expressed microRNAs and affected biological pathways revealed by modulated modularity clustering (MMC) analysis of human preeclamptic and IUGR placentas . Placenta34 ( 7 ), 599 – 605 ( 2013 ).
- Nishizawa H , Pryor-KoishiK , KatoT , KowaH , KurahashiH , UdagawaY . Microarray analysis of differentially expressed fetal genes in placental tissue derived from early and late onset severe pre-eclampsia . Placenta28 ( 5–6 ), 487 – 497 ( 2007 ).
- Van Uitert M , MoerlandPD , EnquobahrieDAet al. Meta-analysis of placental transcriptome data identifies a novel molecular pathway related to preeclampsia . PLoS ONE10 ( 7 ), e0132468 ( 2015 ).
- Sober S , ReimanM , KikasTet al. Extensive shift in placental transcriptome profile in preeclampsia and placental origin of adverse pregnancy outcomes . Sci. Rep.5 , 13336 ( 2015 ).
- Kaartokallio T , CerveraA , KyllonenA , LaivuoriK . Gene expression profiling of pre-eclamptic placentae by RNA sequencing . Sci. Rep.5 , 14107 ( 2015 ).
- Nishizawa H , OtaS , SuzukiMet al. Comparative gene expression profiling of placentas from patients with severe pre-eclampsia and unexplained fetal growth restriction . Reprod. Biol. Endocrinol.9 , 107 ( 2011 ).
- Teschendorff AE , MarabitaF , LechnerMet al. A beta-mixture quantile normalization method for correcting probe design bias in Illumina Infinium 450 k DNA methylation data . Bioinformatics29 ( 2 ), 189 – 196 ( 2013 ).
- Leek JT , JohnsonWE , ParkerHS , JaffeAE , StoreyJD . The SVA package for removing batch effects and other unwanted variation in high-throughput experiments . Bioinformatics28 ( 6 ), 882 – 883 ( 2012 ).
- Morris TJ , ButcherLM , FeberAet al. ChAMP: 450k Chip Analysis Methylation Pipeline . Bioinformatics30 ( 3 ), 428 – 430 ( 2014 ).
- Aryee MJ , JaffeAE , Corrada-BravoHet al. Minfi: a flexible and comprehensive Bioconductor package for the analysis of Infinium DNA methylation microarrays . Bioinformatics30 ( 10 ), 1363 – 1369 ( 2014 ).
- Ritchie ME , PhipsonB , WuDet al. limma powers differential expression analyses for RNA-sequencing and microarray studies . Nucleic Acids Res.43 ( 7 ), e47 ( 2015 ).
- Jaffe AE , MurakamiP , LeeHet al. Bump hunting to identify differentially methylated regions in epigenetic epidemiology studies . Int. J. Epidemiol.41 ( 1 ), 200 – 209 ( 2012 ).
- Friedman J , HastieT , TibshiraniR . Regularization paths for generalized linear models via coordinate descent . J. Stat. Softw.33 ( 1 ), 1 – 22 ( 2010 ).
- Davis S . IlluminaHumanMethylation27k.db: Illumina Illumina Human Methylation 27k annotation data (chip IlluminaHumanMethylation27k) . R package version 1.4.8 ( 2014 ).
- Triche T and Jr . IlluminaHumanMethylation450k.db: Illumina Human Methylation 450k annotation data . R package version 2.0.9 ( 2014 ).
- Barrett T , WilhiteSE , LedouxPet al. NCBI GEO: archive for functional genomics data sets – update . Nucleic Acids Res.41 , D991 – D995 ( 2013 ).
- Buckberry S , Bianco-MiottoT , BentSJ , DekkerGA , RobertsCT . Integrative transcriptome meta-analysis reveals widespread sex-biased gene expression at the human fetal–maternal interface . Mol. Hum. Reprod.20 ( 8 ), 810 – 819 ( 2014 ).
- Augui S , NoraEP , HeardE . Regulation of X-chromosome inactivation by the X-inactivation centre . Nat. Rev. Genet.12 ( 6 ), 429 – 442 ( 2011 ).
- Terry J , SaitoT , SubramanianSet al. TLE1 as a diagnostic immunohistochemical marker for synovial sarcoma emerging from gene expression profiling studies . Am. J. Surg. Pathol.31 ( 2 ), 240 – 246 ( 2007 ).
- Sood R , ZehnderJL , DruzinML , BrownPO . Gene expression patterns in human placenta . Proc. Natl Acad. Sci. USA103 ( 14 ), 5478 – 5483 ( 2006 ).
- Bai Y , XiangX , LiangC , ShiL . Regulating Rac in the nervous system: molecular function and disease implication of Rac GEFs and GAPs . Biomed. Res. Int.2015 , 632450 ( 2015 ).
- Roberts CT . IFPA award in placentology lecture: complicated interactions between genes and the environment in placentation, pregnancy outcome and long term health . Placenta31 ( Suppl. ), S47 – S53 ( 2010 ).
- Clifton VL . Review: sex and the human placenta: mediating differential strategies of fetal growth and survival . Placenta31 ( Suppl. ), S33 – S39 ( 2010 ).
- Vatten LJ , SkjaervenR . Offspring sex and pregnancy outcome by length of gestation . Early Hum. Dev.76 ( 1 ), 47 – 54 ( 2004 ).
- Verburg PE , TuckerG , ScheilW , ErwichJJ , DekkerGA , RobertsCT . Sexual dimorphism in adverse pregnancy outcomes – a Retrospective Australian Population Study 1981–2011 . PLoS ONE11 ( 7 ), e0158807 ( 2016 ).
- Gude NM , RobertsCT , KalionisB , KingRG . Growth and function of the normal human placenta . Thromb. Res.114 ( 5–6 ), 397 – 407 ( 2004 ).
- Moarii M , BoevaV , VertJ-P , ReyalF . Changes in correlation between promoter methylation and gene expression in cancer . BMC Genomics16 , 873 ( 2015 ).
- Guo JU , SuY , ShinJHet al. Distribution, recognition and regulation of non-CpG methylation in the adult mammalian brain . Nat. Neurosci.17 ( 2 ), 215 – 222 ( 2014 ).
- Suter M , MaJ , HarrisAet al. Maternal tobacco use modestly alters correlated epigenome-wide placental DNA methylation and gene expression . Epigenetics6 ( 11 ), 1284 – 1294 ( 2011 ).
- Maccani JZ , KoestlerDC , HousemanEA , MarsitCJ , KelseyKT . Placental DNA methylation alterations associated with maternal tobacco smoking at the RUNX3 gene are also associated with gestational age . Epigenomics5 ( 6 ), 619 – 630 ( 2013 ).
- Horvath S , ErhartW , BroschMet al. Obesity accelerates epigenetic aging of human liver . Proc. Natl Acad. Sci. USA111 ( 43 ), 15538 – 15543 ( 2014 ).