Abstract
A major challenge faced by epigenome-wide association studies (EWAS) is cell-type heterogeneity. As many EWAS have already demonstrated, adjusting for changes in cell-type composition can be critical when analyzing and interpreting findings from such studies. Because of their importance, a great number of different statistical algorithms, which adjust for cell-type composition, have been proposed. Some of the methods are ‘reference based’ in that they require a priori defined reference DNA methylation profiles of cell types that are present in the tissue of interest, while other algorithms are ‘reference free.’ At present, however, it is unclear how best to adjust for cell-type heterogeneity, as this may also largely depend on the type of tissue and phenotype being considered. Here, we provide a critical review of the major existing algorithms for correcting cell-type composition in the context of Illumina Infinium Methylation Beadarrays, with the aim of providing useful recommendations to the EWAS community.
Keywords::
Summary of the main advantages and disadvantages of the two major inference-paradigms for adjusting cell-type heterogeneity in EWAS.
DNAm: DNA methylation; EWAS: Epigenome-wide association study.
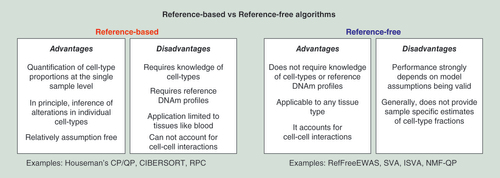
Recommended guideline for selecting an optimal algorithm for cell-type deconvolution in an EWAS. In the final column, within each box, recommended algorithms have been ordered in order of preference.
COMBAT: Combatting Batch Effects; CP: Constrained projection; DNAm: DNA methylation; EWAS: Epigenome-wide association studies; ISVA: Independent surrogate variable analysis; QP: Quadratic programming; RPC: Robust partial correlation; RUV: Removing unwanted variation; SVA: Surrogate variable analysis.
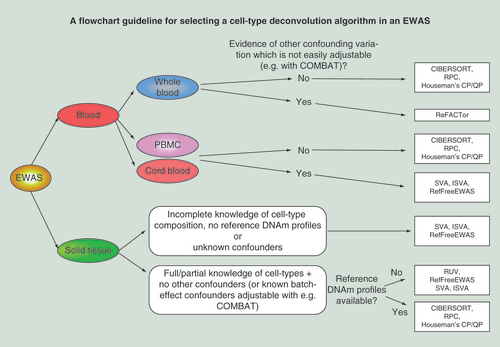
DNA methylation: the marker of choice for epigenome-wide association studies
Epigenetic modifications represent mitotically heritable changes which can affect gene activity and hence cellular phenotype [Citation1]. Epigenome-wide association studies (EWAS) seek to identify epigenetic alterations, which are associated with disease predisposition or disease itself. The number of such EWAS is growing at an unprecedented pace [Citation2], fueled in part by the realization that susceptibility to common diseases may be found also in alterations to the epigenome, in addition to the genome [Citation3,Citation4]. The epigenome is increasingly believed to play a major role in mediating the effects of environmental risk factors on disease [Citation3,Citation5].
In the context of EWAS, the most relevant epigenetic modification is DNA methylation (DNAm), which refers to the covalent addition of a methyl (–CH3) group at cytosines of CG dinucleotides (commonly referred to as ‘CpGs’). Its relevance to EWAS stems from the fact that DNAm is a biochemically stable mark, can be easily amplified from relatively small amounts of DNA and current technologies allow measurement of DNAm genome-wide in a high-throughput cost-effective manner [Citation6,Citation7]. Indeed, because of cost, Illumina Infinium beadchips are favored over whole-genome bisulfite sequencing (WGBS) as EWAS require DNAm measurements in hundreds, if not thousands, of independent samples. The popular Illumina 450k beadchip [Citation8], which measures DNAm at over 450,000 sites, has recently been superseded by the Illumina EPIC/850k beadarray [Citation9], which doubles the genomic coverage. Measuring epigenetic marks on this scale in hundreds to thousands of specimens, including clinical specimens, with a high degree of accuracy and reliability is not yet possible for other epigenetic marks such as histone modifications.
Besides these technical reasons, there are also several biological reasons why DNAm is currently the most relevant epigenetic modification to measure. It plays an essential role in development and tissue differentiation [Citation10]; DNAm in a differentiated cell of a given lineage is thought to play a critical role in irreversibly silencing genes that are not required for specification of that lineage [Citation11]. It further plays a key role in determining transcription factor binding and enhancer function during development [Citation11]. Hence, DNAm is highly tissue and cell-type specific [Citation11,Citation12]. DNAm constitutes a metastable modification, which is maintained during cell division due to the action of DNA methyltransferase enzymes. However, the fidelity of the DNAm copying machinery is significantly lower than that of its DNA counterpart, which may result in ‘epimutations’ every time a cell divides [Citation3]. The rate of such epimutations has been estimated to be as high as 10-5 per cytosine per cell division (cf. with a mutation rate of bases within CpG nucleotides of ∼10-7 per cell division [Citation13,Citation14]). Importantly, DNAm is also highly malleable, and has been shown to be influenced by many environmental exposures, including diet, levels of in utero nutrients and smoking [Citation15–19]. The DNAm landscape changes dramatically as a function of age [Citation20], in cancer [Citation5] and other complex diseases [Citation3]. Thus, DNAm represents not only an attractive disease biomarker, but also offers to improve our understanding of the interface between environmental risk factors and disease phenotypes [Citation3,Citation17,Citation21].
Cell-type heterogeneity in EWAS
A typical EWAS seeks to identify differentially methylated positions (DMPs) between a set of cases (e.g., individuals with a disease, or exposed to a disease risk factor, or predisposed to disease) and controls (usually, age-matched healthy or unexposed individuals). This task, however, is hampered by the complex nature of the tissues in which DNAm is measured. Easily accessible tissues such as blood, saliva/buccal epithelium and cervical smears represent a complex milieu of cell types. For instance, whole blood is composed of at least 7–8 main cell subtypes (neutrophils, eosinophils, basophils, CD14+ monocytes, CD4+ T cells, CD8+ T cells, CD19+ B cells and CD56+ natural killer cells). A major component of cervical smears is immune cell infiltrates [Citation22]. Because DNAm is highly cell-type specific [Citation11], variations in cell-type composition between phenotypes can therefore confound analyses. Often, this manifests itself as an inflated signal (i.e., a very high proportion of positive associations with most of these being false positives). The first clearest demonstration of this phenomenon was a study by Liu et al., which compared 354 rheumatoid arthritis (RA) cases with 337 controls in an EWAS performed in whole blood [Citation23]. Not adjusting for cell-type composition resulted in a large number of associations (caused by a shift in the granulocyte-to-lymphocyte ratio between cases and controls), which however disappeared after correction [Citation23]. Alternatively, variation in cell-type composition which is unrelated, or orthogonal, to the phenotype of interest can mask potential associations leading to deflated signals. For instance, if the EWAS sample size is not large, detection of smoking-associated DNAm changes in whole blood can be compromised by variation in blood-cell-type composition [Citation19]. Thus, adjusting for cell-type heterogeneity is critical as highlighted and emphasized subsequently by several authors [Citation24].
Correcting for cell-type heterogeneity: reference-based methods
Statistical methods aimed at adjusting for cell-type heterogeneity in the context of EWAS can be broadly classified into two types: reference-based and reference-free methods ( & ). Reference-based methods use an a priori defined set of DNAm reference profiles for the cell types of interest, in other words, those thought to be present in the tissue of interest. These reference profiles are defined over a selected set of CpGs, typically those which maximize the discriminative power of the different cell types. So far, these reference DNAm profiles have been generated for tissues such as whole blood (and peripheral blood mononuclear cells PBMC) [Citation25,Citation26], cord blood [Citation27–29], prefrontal cortex [Citation30] and breast [Citation31]. Given these reference DNAm profiles, the method proceeds by postulating that a sample’s DNAm profile is a weighted linear sum of these reference profiles. One then attempts to find the weights which best explain the observed sample profile, in other words, methods typically use a least-squares minimization procedure to accomplish this. Once the proportions of the underlying cell types in the sample have been estimated, these can then be incorporated as covariates in supervised models. This then allows identification of DMPs, where the differential methylation is not driven by underlying changes in cell-type composition.
So far, in EWAS, only one major reference-based algorithm, due to Houseman et al. [Citation26], has been considered. This particular algorithm infers proportions of cell types present in the reference DNAm database using a technique known as constrained projection (CP; in the literature, often also referred to as quadratic programming [QP]). Briefly, in CP/QP inference proceeds via least-squares minimization but subject to the constraint that weights cannot be negative and that the sum of weights must add to 1, or alternatively, that the sum adds to a number which is less than or equal to 1, the latter constraint allowing for the possibility that the reference database does not contain all relevant cell subtypes. Houseman’s CP algorithm has been extensively tested and demonstrated to work extremely well on whole blood [Citation23,Citation42–43], a tissue for which the composition is reasonably well known and for which accurate DNAm reference profiles can be generated [Citation25]. However, it is at present unclear whether the CP/QP technique is the best possible algorithmic framework among reference-based methods. For instance, in the context of gene expression, a nonconstrained reference-based approach called CIBERSORT [Citation32,Citation44], was found to outperform CP/QP, although the reduced performance of CP/QP in the gene expression context could be due to normalization issues which were not fully addressed. CIBERSORT uses an advanced form of Support Vector Regression to perform what is effectively a penalized multivariate regression, imposing non-negativity and normalization constraints only a posteriori, in other words, after estimating the regression weights. This gives the CIBERSORT algorithm more flexibility to search for a wider solution space, yet it may also be more susceptible to large sources of noise. A recent study compared Houseman’s CP algorithm with CIBERSORT and with another alternative based on robust partial correlations [Citation33], concluding that the CP algorithm is only optimal when noise levels are random (i.e., Gaussian) and very large. In fact, for realistic noise levels, robust partial correlations and CIBERSORT were found to outperform Houseman’s CP algorithm [Citation33], consistent with the observations made in the gene expression context [Citation32]. While these results indicate that CP/QP may not be the optimal framework for inference, reference-based algorithms remain underexplored in the context of EWAS, pointing toward the critical need to develop improved forms of existing algorithms.
An important ingredient of any reference-based method is the reference database itself. The quality of the reference DNAm profiles impacts on the quality of the subsequent inference, as nicely demonstrated in a recent study by Koestler et al. [Citation45]. Two related aspects of reference profiles that need particular attention are the number of features (i.e., CpGs/probes) and the resulting colinearity of the cell-type reference profiles. Highly colinear reference profiles (e.g., as for similar cell types) can cause well-known difficulties for statistical inference [Citation32]. For this reason, optimization of the reference database (i.e., selection and number of CpGs) through, say, optimization of the conditioning number of the reference DNAm matrix [Citation32], may result in a reference DNAm database that is more stable to random perturbation, for instance, to random removal of CpGs, as it may happen in practice when not all CpGs in the reference are available in the sample of interest. Other potential problems with constructing a reference DNAm database is the use of reference samples that are not representative of the cell types encountered in real EWAS. For instance, genetics and, in the case of primary cells, the age of the donor tissue can impact on DNAm [Citation20,Citation46–48]. However, in practice, this appears to be less of a limitation because supervised selection of DMPs between cell types generally results in CpGs that exhibit very large differences in DNAm, typically on the order of 70% if not higher, while age is only associated with relatively smaller shifts in DNAm (typically around 10–20% from young to old) [Citation21]. Indeed, large differences in DNAm, if inferred by comparing a number of samples representing two given cell types, are unlikely to be the result of confounding factors such as age, sex or genetics. Confirming this, typical R2 values that one obtains by applying reference-based methods like CP to simulated mixtures of purified blood cell subtypes, using completely independent reference DNAm profiles, are very high (R2 ∼ 0.9–1) [Citation33], suggesting that age, sex and genetic variation are not major confounders in this particular context.
Another possibility to improve the quality of a reference DNAm database is to use prior biological knowledge about which sites are more likely to be cell-type specific. For instance, it is well known that active enhancer regions are among the most cell-type-specific regions [Citation11,Citation12]. Using cell type-specific DNase Hypersensitive Sites information [Citation49] from the NIH Epigenomics Roadmap [Citation12] and ENCODE [Citation50] can, in principle, also help identify sites that are more likely to be bona fide cell type-specific DMPs. However, a recent study showed that, in practical terms, improvements are only rather modest [Citation33], mainly because supervised selection of DMPs, favoring CpGs that exhibit large differences in DNAm between relevant cell types, will identify true positive features, which are strongly enriched for enhancer and DNase Hypersensitive Site regions.
Correcting for cell-type heterogeneity: reference-free methods
An obvious disadvantage of a reference-based approach to cell-type composition estimation is that it requires not only knowledge of the underlying cell-types, but also the existence of an appropriate reference DNAm database (). Depending on the phenotype and tissue of interest, this may not present a major problem (e.g., whole blood EWAS); yet, in many scenarios, the exact composition of tissues is unknown or it might be challenging to obtain purified cell subtypes of these tissues (e.g., for cervical smears or buccal tissue). Even in the case of blood, different cell subsets exist (for example, CD8+ T-cell subsets) [Citation51], which are normally not accounted for in currently available reference DNAm databases [Citation25]. Another limitation of reference-based methods is that for other complex tissues like, for example, breast or skin, which contain a mixture of widely different cell types, including epithelial, adipose, fibroblasts and immune cell types, these cells are known to interact with each other, which can modify their (expression) profiles [Citation52], so that reference profiles obtained from cell types ex vivo may not be the representative of their profiles in vivo. While so far these intercellular interactions have only been shown to alter gene expression profiles [Citation52], concomitant DNAm alterations are likely to be present.
Thus, because of these inherent limitations of reference-based methods, algorithms that can adjust for cell-type composition in a reference-free manner have also attracted considerable attention. Reference-free algorithms specifically designed for EWAS (but which should work on other quantitative data types, e.g., gene expression) include EWASher [Citation53], RefFreeEWAS [Citation34] and more recently, ReFACTOR [Citation36]. In addition, there are reference-free approaches such as surrogate variable analysis (SVA) [Citation37,Citation54], independent surrogate variable analysis (ISVA) [Citation39], whose goal is to adjust the data for any type of confounder (be it cell-type composition or not) [Citation24,Citation55]. Another inference paradigm, which can be viewed as reference-free, is the removing unwanted variation (RUV) framework [Citation40], which has recently been adapted to Illumina DNAm data (RUVm) [Citation56]. Other reference-free approaches, which have been explored in the gene expression context, but which could be equally applied to DNAm data, are based on non-negative matrix factorization (NMF) [Citation57,Citation58]. Indeed, a more recent approach by Houseman combines NMF and QP/CP (a method we here call NMF–QP) [Citation41]. Generally speaking, most of these algorithms differ quite substantially in terms of their implementation, which can greatly impact on performance.
For instance, one key assumption of EWASher and ReFACTOR is that the top components of variation in the data are driven by cell-type composition. While this assumption is generally valid for EWAS conducted in whole blood (where relative fractions of granulocytes and lymphocytes vary substantially across even a healthy population of individuals), it is unclear whether this assumption would hold in other scenarios. For example, peripheral blood is devoid of granulocytes and is composed mainly of leukocytes. Thus, in this scenario, it is less clear if the top components of variation would all be associated with cell-type composition. Therefore, depending on the tissue and phenotype of interest, it could well be that the assumption underlying EWASher or ReFACTOR fails. As an extreme example, the EWASher study [Citation53] reported only a few differentially methylated CpGs between normal breast and breast cancer tissue in stark contrast to studies demonstrating that most of the breast cancer DNAm landscape is altered [Citation59] which is further supported by studies conducted in cell lines [Citation60]. Thus, methods like EWASher or ReFACTOR can remove genuine biological signal if top components of variation are associated with the phenotype of interest and not only driven by cell-type composition. This drawback can be avoided by using methods such as RefFreeEWAS, SVA or ISVA, which use the phenotype of interest from the outset in a supervised framework. In the case of SVA or ISVA, surrogate variables representing potential confounders are identified in the space of residual variation, in other words, in the data obtained after regressing out the effect due to the phenotype of interest. Thus, in SVA/ISVA, we no longer need to assume that the top components of variation in the data are due to changes in cell-type composition. Likewise, RefFreeEWAS first regresses out the effect of the phenotype of interest on the data and subsequently performs a singular value decomposition on an augmented matrix made up of the estimated regression and residual variation matrix [Citation34,Citation61]. As with SVA/ISVA, it, therefore, does not assume that the top components of data variation are associated with cell-type composition, although it does assume that the top components in the regression and residual variation space are, a subtle difference which sets it apart from SVA/ISVA. Comparing EWASher/ReFACTOR with SVA/ISVA/RefFreeEWAS, it is thus clear that the latter methods should be preferable, as the assumptions underlying their models are more realistic and more likely to hold, independently of tissue type and phenotype of interest. However, it is worth pointing out that the inference one draws from any method is only as good as the model itself, and approaches such as SVA/ISVA/RefFreeEWAS may also overfit. Indeed, if the underlying regression model linking phenotype of interest to the data is a poor one, then it is possible that residual variation may still be biological [Citation39]. With an approach such as SVA, this could then also lead to a loss of power [Citation39]. This problem can, in principle, be circumvented by judicious choice of the subspace in which to construct the surrogate variables, yet choosing this subspace is an unsolved problem if confounders are truly unknown [Citation39].
Another reference-free approach which was not specifically designed for cell-type composition, but which could be adapted, is RUVm [Citation56]. This builds on a previous version of the algorithm (RUV) designed for gene expression data and is based on the concept of using negative control probes to capture variation associated with confounding factors [Citation40]. RUVm uses in-built negative controls of the Illumina beadarray to model confounding variation and subsequently identifies a refined set of empirically determined control probes, which are then used as covariates in the final supervised regression to identify DMPs. As remarked by Jaffe and Irizarry [Citation24], this method could be easily adapted to the cell-type composition problem, by using as control probes, CpGs that discriminate relevant cell types. As such, this method would not be truly reference free, as it uses prior knowledge, of which CpGs are important cell type-specific markers. On the other hand, it would also not be an entirely reference-based method, since it does not need explicit reference DNAm profiles. Thus, such an approach could be categorized as ‘semireference free’, and algorithms based on this concept remain underexplored.
Correcting for cell-type heterogeneity: is there an optimal method?
We have summarized the main advantages and disadvantages of reference-free and reference-based methods (). In terms of actual performance, whether on simulated or real data, there is currently a lack of studies offering a comprehensive and objective comparison of these algorithms. So far, the most comprehensive analysis has been performed by McGregor et al. [Citation62], which compared mainly six algorithms (Houseman’s reference-based CP method, RefFreeEWAS, SVA, ISVA, EWASher and RUV). This study compared performance using extensively simulated data, as well as real EWAS, concluding that no single method performs best across all evaluation scenarios [Citation62]. Furthermore, the study concluded that SVA, although rarely the best in any single data set, was the most robust, in other word, the safest method. McGregor et al. are right in pointing out that robustness is, probably, also the most desirable feature of an algorithm, given that most methods exhibited substantial variation in performance. As explained earlier, SVA and ISVA are relatively free of underlying assumptions and are also able to adjust for confounders other than cell-type composition. Hence, this might explain why these methods were found to be more robust. Nevertheless, it is still premature to discard algorithms such as RefFreeEWAS or reference-based methods, since the evaluation of McGregor et al. did not consider many real data sets. Performance of a given method across different real data sets can be highly variable and often very different to what is observed on simulated data. Hence, a more objective comparison would require evaluation of all of these methods in at least five to six independent EWAS. To make this point clear, finding that ‘method-A’ outperforms ‘method-B’ in two EWAS is as significant as claiming that a coin is biased just because it yielded two heads when tossed twice. To conclusively demonstrate that ‘method-A’ outperforms ‘method-B’ would require at least five to six independent EWAS, since this would then be equivalent to obtaining five to six heads in a row, the probability of which is less than 0.05.
Another study comparing a number of different algorithms (Houseman’s reference-based CP, RefFreeEWAS, EWASher and ReFACTor) on simulated as well as real data is the one by Rahmani et al. [Citation36], which concluded that ReFACTor leads to improved modeling accuracy and power. Although ReFACTor has some merit, this study is problematic for a number of reasons. First, the comparison of ReFACTor to Houseman’s method in terms of modeling cell-type composition was biased. Using matched flow-cytometric estimates of underlying blood cell subtypes as gold standard, the authors compared R2 values obtained from ReFACTor with those from Houseman’s CP algorithm, yet the R2 values from ReFACTor were inflated because they were obtained by fitting a multivariate model which included up to ten linear components, without ever considering model complexity, a procedure which results in overfitting. Indeed, as recently pointed out by Houseman et al. [Citation41], it is extremely unlikely that any reference-free approach (without further biological input) could ever outperform a reference-based method in terms of modeling cell-type composition. Indeed, the reference-free NMF–QP approach of Houseman was not shown to model cell-type composition better than reference-based methods, and would require additional biological input (e.g., knowledge of which CpGs are cell type-specific DMPs) to offer comparable levels of modeling accuracy [Citation41]. A second major concern with the ReFACTor study is the use of inappropriate gold standards when evaluating methods on a real EWAS. Focusing only on one RA EWAS to define a gold-standard list of RA associated CpGs (which were identified using Houseman’s CP method) is inappropriate since these associations have yet to be replicated. It is therefore advisable to compare algorithms with other types of EWAS for which replicate studies have been performed. For instance, this is the case for smoking, for which there are at least five to six independent EWAS in whole blood, all leading to the same set of smoking-associated DMPs [Citation18]. This common set of smoking-associated DMPs can then be used to define a bona fide gold-standard true positive list. Thus, in summary, it is premature, based on existing comparative studies, to conclude that there is an algorithm which works optimally in all main scenarios. Moreover, current evaluations of cell-type deconvolution methods have been applied exclusively in the context of Illumina Infinium beadchips with as yet no comparative studies on WGBS data. To determine whether there is an optimal method will thus require much more extensive and objective comparisons, using also more comprehensive technologies such as WGBS. In the meantime, we provide a flowchart to help guide users to the best possible choice of algorithm to meet the needs of their specific EWAS ().
Future perspective
Looking into the future, it is clear that cell type heterogeneity will continue to present a major challenge to the analysis and interpretation of EWAS data. Although existing reference-based algorithms provide reasonably good approximations to cell type composition estimates in whole blood, these have only been assessed in relation to major blood cell subtypes. As already pointed out by a few authors [Citation51], there is an important need to accurately quantify and map the full extent of cell type heterogeneity within a known tissue such as blood, since otherwise inferred DMPs may still be caused by shifts in rare cell subtype proportions. However, for certain phenotypes such as age, it is fairly well accepted that specific DNAm alterations (e.g., those that map to repressed chromatin domains) [Citation20], or those making up Horvath’s clock [Citation63], are not driven by cell type composition [Citation64,Citation65]. Indeed, it would be hard to explain such tissue-independent age-associated DNAm shifts purely in terms of alterations to the proportion of underlying cell types, since these associations have even been observed in highly diverse and purified cell populations, including stem-cell populations [Citation20,Citation65–68]. On the other hand, this may not generalize to other phenotypes. For instance, in the context of smoking, a recent study concluded that differential DNAm of a site mapping to GPR15 (a well-known smoking associated EWAS hit [Citation18]) is caused by an expansion of a normal CD3+ T-cell subtype (marked by overexpression of GPR15) in the peripheral blood of smokers [Citation69,Citation70]. However, whether the increased proportion of this T-cell subtype in the blood of smokers is due to an expansion of a normal pre-existing rare T-cell subtype, or whether it is caused by de novo DNAm changes to a common T-cell subtype is still unclear, as the study was underpowered and could not conclusively assess the effect of smoking carcinogens on DNAm patterns [Citation70]. As the epigenomes, including DNA methylomes of all major and minor blood cell subtypes are generated as part of Blueprint [Citation71], this resource will undoubtedly help generate more complete reference DNAm databases, allowing more refined higher resolution analyses of blood EWAS, which may help resolve these outstanding issues. Yet an immediate statistical challenge in translating WGBS methylomes from the International Human Epigenome Consortium (IHEC) and Blueprint [Citation72] into reference DNAm profiles that could be used for cell type deconvolution in Illumina 450k/EPIC EWAS is that the coverage of WGBS data is also limited [Citation73]. For instance, the overlap between WGBS data for IHEC samples and Illumina 450k probes is typically only around 40% and can range from as low as 15% to as high as 70%. As pointed out by Libertini et al., the recommended IHEC coverage (30×) for WGBS means that up to 50% of DMPs could be lost, not allowing for high-resolution DMP analysis [Citation73]. How big a limitation this may present to cell type deconvolution algorithms, however, remains to be seen, since so far fairly accurate deconvolution is possible in specific tissues such as blood using reference DNAm profiles defined over only a relatively few DMPs (typically ∼100–500). If it does present a limitation, a potential solution will be to construct reference DNAm profiles, not at the level of DMPs, but at the level of differentially methylated regions, such as, for example, COMETS, which represent regions of differential co-methylation [Citation74,Citation75].
Generating more complete reference DNA methylomes for all major human cell types, as done by IHEC, will also allow direct application of reference-based methods to many other complex tissues like breast, brain, skin or cervix. For instance, a recent study successfully constructed a reference DNAm database for breast tissue, which included reference profiles for breast epithelial cells, adipose cells and all major immune cell subtypes, and used it in conjunction with CP/QP to infer fractions of these cell types in a breast cancer EWAS [Citation31]. This study demonstrated that the top component of variation correlates with variations in adiposity, underlining the importance of adjusting for this variation in breast tissue. As the number of available reference DNAm profiles grows, there will be a strong need to develop statistical methods which can optimize the construction of reference DNAm databases geared toward analysis of a given tissue type. This is particularly pertinent since colinearity of reference DNAm profiles is detrimental to the quality of inference [Citation32,Citation45], and colinearity would increase in line with the resolution and number of different cell types present in such reference DNAm databases. Given that cell types can be naturally arranged on a developmental tree representing a differentiation hierarchy with human embryonic stem cells at the top and terminally differentiated cells at the bottom, we anticipate that the most powerful cell type deconvolution algorithms may need to incorporate such a hierarchical tree-like structure in the inference. In line with this, and depending on the ultimate task and biological question, using an overly detailed reference DNAm database might be unnecessary and counterproductive [Citation32]. For example, if the aim is to adjust for immune cell contaminants to identify DNAm alterations which are specific to the epithelial compartment of a tissue, then it may suffice to have one reference DNAm profile to represent any immune cell type, which could be constructed by focusing on common differences between any type of immune cell and epithelial cells. The latter strategy was successfully adopted in a recent breast cancer EWAS [Citation31]. On the other hand, allowing for different types of immune cells can be critical in the context of epithelial tumors, where the type of immune-cell infiltrate has been shown to dictate risk of distant metastasis and clinical outcome [Citation44,Citation76].
Another outstanding question is the extent to which cell–cell interactions can affect and limit the applicability of reference-based methods. For instance, recent single-cell analyses, performed at the level of gene expression [Citation52], point toward the importance of cell–cell interactions in modifying specific gene expression programs of tumor, stromal and immune cells. Upcoming single-cell RNA-Seq and DNAm data may thus help provide a framework to more objectively compare reference-based with reference-free methods.
Single-cell DNAm analysis also offers a means of improving the resolution of reference-based methods. While cell-to-cell variation in DNAm within a phenotypically homogeneous cell population has been observed [Citation77], it is yet unclear whether this intercellular epigenetic variation is of functional or phenotypic importance. If important, such intercellular DNAm heterogeneity requires a revision of the notion of ‘cell type’. However, we anticipate that the functional or phenotypic importance of intercellular DNAm variability will manifest itself at the cell-population level, as has been proposed for the case of gene expression [Citation78]. This would then allow for a well-defined notion of ‘cell type’, and reference DNAm profiles for such cell types could be constructed fairly accurately by comparing bulk or pooled DNAm profiles of corresponding cell types and selecting DMPs that exhibit the largest differences in mean DNAm. DMPs exhibiting large (i.e., >80%) changes in DNAm between cell types are unlikely to represent loci that exhibit substantial cell-to-cell variation within a cell type.
Another interesting question which has not yet been addressed and which could serve to further assess the reliability of existing algorithms is a direct comparison between data types. While studies have compared expression (the ESTIMATE algorithm [Citation79]) and methylation (the MethylPurify algorithm [Citation80]) based estimates of tumor purity with those obtained using gold-standard SNP/copy-number assays, no study has yet evaluated the extent to which expression-based and DNAm-based cell type composition estimates agree, even in a common tissue such as blood. This is important to assess, since DNAm offers a more stable assay than RNA, yet is not directly functional, rendering a comparison between the two data types highly nontrivial and interesting. Such comparisons are possible using existing matched DNAm and RNA-Seq data sets [Citation65,Citation81]. In performing such comparisons, one should not forget to include statistical cell type deconvolution algorithms which have been developed mainly for gene expression (e.g., CIBERSORT [Citation32] or CellMix [Citation82,Citation83]), but which are, in principle, equally applicable to any other data type, including DNAm.
Integration of multiple data types, while not possible for most EWAS (which by definition only measure DNAm), presents an interesting methodological opportunity to improve the construction of reference DNAm databases, or, as a means of incorporating higher systems-level information as prior biological knowledge, which may be particularly useful for reference-free or semi-reference free approaches. For instance, cell type-specific DMPs which correlate with gene expression in-cis may represent a more biologically relevant set of features for constructing reference DNAm databases. Alternatively, previous studies have shown the value of integrating DNAm data with interaction networks to identify gene modules which mark specific cell types [Citation84]. This may provide another means of adjusting for cell type composition, either in a reference-based or semi-reference free framework.
Last but not least, another unmet yet key challenge is the identification of the specific cell types which drive the interesting DMPs. In principle, a DMP between phenotypes, which is not driven by cell type composition effects, could be the result of differential methylation in only one of the underlying cell types, or, at the other extreme, be present in all underlying cell types [Citation61]. Identifying which specific cell types carry the DNAm alteration is of fundamental importance, and statistical approaches to this problem are lacking. In the context of smoking, a recent experimental study has shown that specific well-validated smoking-associated DMPs may be present in all blood cell subtypes (e.g., cg05575921 in AHRR) or only in specific subsets, for instance, hypomethylation of two CpGs mapping to GFI1 and F2RL3 was found to be specific to granulocytes, while hypomethylation of another two CpGs mapping to CPOX and GPR15 were only found in peripheral blood mononuclear cells (T cells) from smokers [Citation69]. From a statistical-methods perspective, findings of this kind are extremely useful for developing and improving algorithms which could pinpoint the specific types of cells that are altered.
Table 1. Algorithm name, whether it is reference-free, reference-based or semireference free, what inference is possible with the algorithm, tissue types on which it has been successfully applied, whether algorithm adjusts for confounders other than cell-type composition, programming language in which it is available, website link and main reference.
Statistical algorithms aimed at correcting for cell type heterogeneity in epigenome-wide association studies (EWAS) can be broadly classified into reference-based and reference-free algorithms, with semireference-free emerging as a promising new inference paradigm.
For EWAS conducted in whole blood, peripheral blood mononuclear cells or cord blood, we recommend the use of reference-based methods like CIBERSORT or robust partial correlations. However, if there is evidence of additional confounding variation and absolute quantification of cell type proportions is not required, methods like surrogate variable analysis, independent surrogate variable analysis or RefFreeEWAS are a safer option.
For EWAS studies conducted in other tissues (e.g., buccal, cervical smears or other epithelial tissues), unless a high-quality DNA methylation reference database is available, we recommend the use of reference-free methods like surrogate variable analysis, independent surrogate variable analysis or RefFreeEWAS.
Financial & competing interests disclosure
This work was supported by a Royal Society Newton Advanced Fellowship (AET), NAF (project number: 522438, award number: 164914) and an NSFC (National Science Foundation of China) grant (grant number 31571359). The authors have no other relevant affiliations or financial involvement with any organization or entity with a financial interest in or financial conflict with the subject matter or materials discussed in the manuscript apart from those disclosed.
No writing assistance was utilized in the production of this manuscript.
Additional information
Funding
References
- Deaton AM , BirdA . CpG islands and the regulation of transcription . Genes Dev.25 ( 10 ), 1010 – 1022 ( 2011 ).
- Rakyan VK , DownTA , BaldingDJ , BeckS . Epigenome-wide association studies for common human diseases . Nat. Rev. Genet.12 ( 8 ), 529 – 541 ( 2011 ).
- Petronis A . Epigenetics as a unifying principle in the aetiology of complex traits and diseases . Nature465 ( 7299 ), 721 – 727 ( 2010 ).
- Zhernakova DV , DeelenP , VermaatMet al. Identification of context-dependent expression quantitative trait loci in whole blood . Nat. Genet.49 ( 1 ), 139 – 145 ( 2017 ).
- Feinberg AP , OhlssonR , HenikoffS . The epigenetic progenitor origin of human cancer . Nat. Rev. Genet.7 ( 1 ), 21 – 33 ( 2006 ).
- Beck S , RakyanVK . The methylome: approaches for global DNA methylation profiling . Trends Genet.24 ( 5 ), 231 – 237 ( 2008 ).
- Beck S . Taking the measure of the methylome . Nat. Biotechnol.28 ( 10 ), 1026 – 1028 ( 2010 ).
- Sandoval J , HeynH , MoranSet al. Validation of a DNA methylation microarray for 450,000 CpG sites in the human genome . Epigenetics6 ( 6 ), 692 – 702 ( 2011 ).
- Moran S , ArribasC , EstellerM . Validation of a DNA methylation microarray for 850,000 CpG sites of the human genome enriched in enhancer sequences . Epigenomics8 ( 3 ), 389 – 399 ( 2016 ).
- Reik W . Stability and flexibility of epigenetic gene regulation in mammalian development . Nature447 ( 7143 ), 425 – 432 ( 2007 ).
- Ziller MJ , GuHC , MullerFet al. Charting a dynamic DNA methylation landscape of the human genome . Nature500 ( 7463 ), 477 – 481 ( 2013 ).
- Roadmap Epigenomics CConsortium , KundajeA , MeulemanWet al.Integrative analysis of 111 reference human epigenomes . Nature518 ( 7539 ), 317 – 330 ( 2015 ).
- Campbell CD , ChongJX , MaligMet al. Estimating the human mutation rate using autozygosity in a founder population . Nat. Genet.44 ( 11 ), 1277 – 1281 ( 2012 ).
- Horsthemke B . Epimutations in human disease . Curr. Top. Microbiol. Immunol.310 , 45 – 59 ( 2006 ).
- Feil R , FragaMF . Epigenetics and the environment: emerging patterns and implications . Nat. Rev. Genet.13 ( 2 ), 97 – 109 ( 2011 ).
- Heijmans BT , TobiEW , SteinADet al. Persistent epigenetic differences associated with prenatal exposure to famine in humans . Proc. Natl Acad. Sci. USA105 ( 44 ), 17046 – 17049 ( 2008 ).
- Jirtle RL , SkinnerMK . Environmental epigenomics and disease susceptibility . Nat. Rev. Genet.8 ( 4 ), 253 – 262 ( 2007 ).
- Gao X , JiaM , ZhangY , BreitlingLP , BrennerH . DNA methylation changes of whole blood cells in response to active smoking exposure in adults: a systematic review of DNA methylation studies . Clin. Epigenetics7 , 113 ( 2015 ).
- Teschendorff AE , YangZ , WongAet al. Correlation of smoking-associated DNA methylation changes in buccal cells with DNA methylation changes in epithelial cancer . JAMA Oncol.1 ( 4 ), 476 – 474 ( 2015 ).
- Teschendorff AE , MenonU , Gentry-MaharajAet al. Age-dependent DNA methylation of genes that are suppressed in stem cells is a hallmark of cancer . Genome Res.20 ( 4 ), 440 – 446 ( 2010 ).
- Teschendorff AE , WestJ , BeckS . Age-associated epigenetic drift: implications, and a case of epigenetic thrift?Hum. Mol. Genet.22 ( R1 ), R7 – R15 ( 2013 ).
- Doufekas K , ZhengSC , GhazaliSet al. DNA methylation signatures in vaginal fluid samples for detection of cervical and endometrial cancer . Int. J. Gynecol. Cancer doi:10.1097/IGC.0000000000000739 ( 2016 ) ( Epub ahead of print ).
- Liu Y , AryeeMJ , PadyukovLet al. Epigenome-wide association data implicate DNA methylation as an intermediary of genetic risk in rheumatoid arthritis . Nat. Biotechnol.31 ( 2 ), 142 – 147 ( 2013 ).
- Jaffe AE , IrizarryRA . Accounting for cellular heterogeneity is critical in epigenome-wide association studies . Genome Biol.15 ( 2 ), R31 ( 2014 ).
- Reinius LE , AcevedoN , JoerinkMet al. Differential DNA methylation in purified human blood cells: implications for cell lineage and studies on disease susceptibility . PLoS ONE7 ( 7 ), e41361 ( 2012 ).
- Houseman EA , AccomandoWP , KoestlerDCet al. DNA methylation arrays as surrogate measures of cell mixture distribution . BMC Bioinformatics13 , 86 ( 2012 ).
- Gervin K , PageCM , AassHCet al. Cell type specific DNA methylation in cord blood: a 450K-reference data set and cell count-based validation of estimated cell type composition . Epigenetics11 ( 9 ), 690 – 698 ( 2016 ).
- Cardenas A , AllardC , DoyonMet al. Validation of a DNA methylation reference panel for the estimation of nucleated cells types in cord blood . Epigenetics11 ( 11 ), 773 – 779 ( 2016 ).
- Bakulski KM , FeinbergJI , AndrewsSVet al. DNA methylation of cord blood cell types: applications for mixed cell birth studies . Epigenetics11 ( 5 ), 354 – 362 ( 2016 ).
- Guintivano J , AryeeMJ , KaminskyZA . A cell epigenotype specific model for the correction of brain cellular heterogeneity bias and its application to age, brain region and major depression . Epigenetics8 ( 3 ), 290 – 302 ( 2013 ).
- Teschendorff AE , GaoY , JonesAet al. DNA methylation outliers in normal breast tissue identify field defects that are enriched in cancer . Nat. Commun.7 , 10478 ( 2016 ).
- Newman AM , LiuCL , GreenMRet al. Robust enumeration of cell subsets from tissue expression profiles . Nat. Methods12 ( 5 ), 453 – 457 ( 2015 ).
- Teschendorff AE . A comparison of reference-based algorithms for correcting cell type heterogeneity in epigenome-wide association studies . BMC Bioinformatics18 ( 1 ), 105 ( 2017 ).
- Houseman EA , MolitorJ , MarsitCJ . Reference-free cell mixture adjustments in analysis of DNA methylation data . Bioinformatics30 ( 10 ), 1431 – 1439 ( 2014 ).
- Zou J , LippertC , HeckermanD , AryeeM , ListgartenJ . Epigenome-wide association studies without the need for cell type composition . Nat. Methods11 ( 3 ), 309 – 311 ( 2014 ).
- Rahmani E , ZaitlenN , BaranYet al. Sparse PCA corrects for cell type heterogeneity in epigenome-wide association studies . Nat. Methods13 ( 5 ), 443 – 445 ( 2016 ).
- Leek JT , StoreyJD . Capturing heterogeneity in gene expression studies by surrogate variable analysis . PLoS Genet.3 ( 9 ), 1724 – 1735 ( 2007 ).
- Leek JT , JohnsonWE , ParkerHS , JaffeAE , StoreyJD . The sva package for removing batch effects and other unwanted variation in high-throughput experiments . Bioinformatics28 ( 6 ), 882 – 883 ( 2012 ).
- Teschendorff AE , ZhuangJ , WidschwendterM . Independent surrogate variable analysis to deconvolve confounding factors in large-scale microarray profiling studies . Bioinformatics27 ( 11 ), 1496 – 1505 ( 2011 ).
- Gagnon-Bartsch JA , SpeedTP . Using control genes to correct for unwanted variation in microarray data . Biostatistics13 ( 3 ), 539 – 552 ( 2012 ).
- Houseman EA , KileML , ChristianiDC , InceTA , KelseyKT , MarsitCJ . Reference-free deconvolution of DNA methylation data and mediation by cell composition effects . BMC Bioinformatics17 , 259 ( 2016 ).
- Langevin SM , HousemanEA , AccomandoWPet al. Leukocyte-adjusted epigenome-wide association studies of blood from solid tumor patients . Epigenetics9 ( 6 ), 884 – 895 ( 2014 ).
- Accomando WP , WienckeJK , HousemanEA , NelsonHH , KelseyKT . Quantitative reconstruction of leukocyte subsets using DNA methylation . Genome Biol.15 ( 3 ), R50 ( 2014 ).
- Gentles AJ , NewmanAM , LiuCLet al. The prognostic landscape of genes and infiltrating immune cells across human cancers . Nat. Med.21 ( 8 ), 938 – 945 ( 2015 ).
- Koestler DC , JonesMJ , UssetJet al. Improving cell mixture deconvolution by identifying optimal DNA methylation libraries (IDOL) . BMC Bioinformatics17 ( 1 ), 120 ( 2016 ).
- Van Dongen J , NivardMG , WillemsenGet al. Genetic and environmental influences interact with age and sex in shaping the human methylome . Nat. Commun.7 , 11115 ( 2016 ).
- Issa JP , AhujaN , ToyotaM , BronnerMP , BrentnallTA . Accelerated age-related CpG island methylation in ulcerative colitis . Cancer Res.61 ( 9 ), 3573 – 3577 ( 2001 ).
- Fraga MF , BallestarE , PazMFet al. Epigenetic differences arise during the lifetime of monozygotic twins . Proc. Natl Acad. Sci. USA102 ( 30 ), 10604 – 10609 ( 2005 ).
- Thurman RE , RynesE , HumbertRet al. The accessible chromatin landscape of the human genome . Nature489 ( 7414 ), 75 – 82 ( 2012 ).
- Gerstein MB , KundajeA , HariharanMet al. Architecture of the human regulatory network derived from ENCODE data . Nature489 ( 7414 ), 91 – 100 ( 2012 ).
- Horvath S , LevineAJ . HIV-1 infection accelerates age according to the epigenetic clock . J. Infect. Dis.212 ( 10 ), 1563 – 1573 ( 2015 ).
- Tirosh I , IzarB , PrakadanSMet al. Dissecting the multicellular ecosystem of metastatic melanoma by single-cell RNA-seq . Science352 ( 6282 ), 189 – 196 ( 2016 ).
- Zou J , LippertC , HeckermanD , AryeeM , ListgartenJ . Epigenome-wide association studies without the need for cell type composition . Nat. Methods11 ( 3 ), 309 – 311 ( 2014 ).
- Leek JT , StoreyJD . A general framework for multiple testing dependence . Proc. Natl Acad. Sci. USA105 ( 48 ), 18718 – 18723 ( 2008 ).
- Leek JT , ScharpfRB , BravoHCet al. Tackling the widespread and critical impact of batch effects in high-throughput data . Nat. Rev. Genet.11 ( 10 ), 733 – 739 ( 2010 ).
- Maksimovic J , Gagnon-BartschJA , SpeedTP , OshlackA . Removing unwanted variation in a differential methylation analysis of Illumina HumanMethylation450 array data . Nucleic Acids Res.43 ( 16 ), e106 ( 2015 ).
- Moffitt RA , MarayatiR , FlateELet al. Virtual microdissection identifies distinct tumor- and stroma-specific subtypes of pancreatic ductal adenocarcinoma . Nat. Genet.47 ( 10 ), 1168 – 1178 ( 2015 ).
- Repsilber D , KernS , TelaarAet al. Biomarker discovery in heterogeneous tissue samples – taking the in-silico deconfounding approach . BMC Bioinformatics11 , 27 ( 2010 ).
- Timp W , BravoHC , McdonaldOGet al. Large hypomethylated blocks as a universal defining epigenetic alteration in human solid tumors . Genome Med.6 ( 8 ), 61 ( 2014 ).
- Iorio F , KnijnenburgTA , VisDJet al. A landscape of pharmacogenomic interactions in cancer . Cell166 ( 3 ), 740 – 754 ( 2016 ).
- Houseman EA , KelseyKT , WienckeJK , MarsitCJ . Cell-composition effects in the analysis of DNA methylation array data: a mathematical perspective . BMC Bioinformatics16 , 95 ( 2015 ).
- Mcgregor K , BernatskyS , ColmegnaIet al. An evaluation of methods correcting for cell type heterogeneity in DNA methylation studies . Genome Biol.17 ( 1 ), 84 ( 2016 ).
- Horvath S . DNA methylation age of human tissues and cell types . Genome Biol.14 ( 10 ), R115 ( 2013 ).
- Yuan T , JiaoY , De JongS , OphoffRA , BeckS , TeschendorffAE . An integrative multi-scale analysis of the dynamic DNA methylation landscape in aging . PLoS Genet.11 ( 2 ), e1004996 ( 2015 ).
- Slieker RC , Van ItersonM , LuijkRet al. Age-related accrual of methylomic variability is linked to fundamental ageing mechanisms . Genome Biol.17 ( 1 ), 191 ( 2016 ).
- Rakyan VK , DownTA , MaslauSet al. Human aging-associated DNA hypermethylation occurs preferentially at bivalent chromatin domains . Genome Res.20 ( 4 ), 434 – 439 ( 2010 ).
- Reynolds LM , TaylorJR , DingJet al. Age-related variations in the methylome associated with gene expression in human monocytes and T cells . Nat. Commun.5 , 5366 ( 2014 ).
- Yang Z , WongA , KuhDet al. Correlation of an epigenetic mitotic clock with cancer risk . Genome Biol.17 ( 1 ), 205 ( 2016 ).
- Bauer M , FinkB , ThurmannL , EszlingerM , HerberthG , LehmannI . Tobacco smoking differently influences cell types of the innate and adaptive immune system-indications from CpG site methylation . Clin. Epigenetics7 , 83 ( 2015 ).
- Bauer M , LinselG , FinkBet al. A varying T cell subtype explains apparent tobacco smoking induced single CpG hypomethylation in whole blood . Clin. Epigenetics7 , 81 ( 2015 ).
- Adams D , AltucciL , AntonarakisSEet al. BLUEPRINT to decode the epigenetic signature written in blood . Nat. Biotechnol.30 ( 3 ), 224 – 226 ( 2012 ).
- Stunnenberg HG , International Human EpigenomeC , HirstM . The International Human Epigenome Consortium: a blueprint for scientific collaboration and discovery . Cell167 ( 7 ), 1897 ( 2016 ).
- Libertini E , HeathSC , HamoudiRAet al. Saturation analysis for whole-genome bisulfite sequencing data . Nat. Biotechnol. doi:10.1038/nbt.3524 ( 2016 ) ( Epub ahead of print ).
- Libertini E , HeathSC , HamoudiRAet al. Information recovery from low coverage whole-genome bisulfite sequencing . Nat. Commun.7 , 11306 ( 2016 ).
- Jaffe AE , MurakamiP , LeeHet al. Bump hunting to identify differentially methylated regions in epigenetic epidemiology studies . Int. J. Epidemiol.41 ( 1 ), 200 – 209 ( 2012 ).
- De Simone M , ArrigoniA , RossettiGet al. Transcriptional landscape of human tissue lymphocytes unveils uniqueness of tumor-infiltrating T regulatory cells . Immunity45 ( 5 ), 1135 – 1147 ( 2016 ).
- Farlik M , HalbritterF , MullerFet al. DNA methylation dynamics of human hematopoietic stem cell differentiation . Cell Stem Cell19 ( 6 ), 808 – 822 ( 2016 ).
- Macarthur BD , LemischkaIR . Statistical mechanics of pluripotency . Cell154 ( 3 ), 484 – 489 ( 2013 ).
- Yoshihara K , ShahmoradgoliM , MartinezEet al. Inferring tumour purity and stromal and immune cell admixture from expression data . Nat. Commun.4 , 2612 ( 2013 ).
- Zheng X , ZhaoQ , WuHJet al. MethylPurify: tumor purity deconvolution and differential methylation detection from single tumor DNA methylomes . Genome Biol.15 ( 8 ), 419 ( 2014 ).
- Cancer Genome Atlas Research Network , WeinsteinJN , CollissonEAet al.The Cancer Genome Atlas Pan-Cancer analysis project . Nat. Genet.45 ( 10 ), 1113 – 1120 ( 2013 ).
- Gaujoux R , SeoigheC . CellMix: a comprehensive toolbox for gene expression deconvolution . Bioinformatics29 ( 17 ), 2211 – 2212 ( 2013 ).
- Shen-Orr SS , GaujouxR . Computational deconvolution: extracting cell type-specific information from heterogeneous samples . Curr. Opin. Immunol.25 ( 5 ), 571 – 578 ( 2013 ).
- Jiao Y , WidschwendterM , TeschendorffAE . A systems-level integrative framework for genome-wide DNA methylation and gene expression data identifies differential gene expression modules under epigenetic control . Bioinformatics30 ( 16 ), 2360 – 2366 ( 2014 ).