Abstract
Aim: Despite numerous published prognostic methylation markers for renal cell carcinoma (RCC), none of these have yet changed patient management. Our aim is to systematically review and evaluate the literature on prognostic DNA methylation markers for RCC. Materials & methods: We conducted an exhaustive search of PubMed, EMBASE and MEDLINE up to April 2017 and identified 49 publications. Studies were reviewed according to the Preferred Reporting Items for Systematic Reviews and Meta-Analyses (PRISMA) statement, assessed for their reporting quality using the Reporting Recommendations for Tumor Marker Prognostic Studies (REMARK) criteria, and were graded to determine the level of evidence (LOE) for each biomarker. Results: We identified promoter methylation of BNC1, SCUBE3, GATA5, SFRP1, GREM1, RASSF1A, PCDH8, LAD1 and NEFH as promising prognostic markers. Extensive methodological heterogeneity across the included studies was observed, which hampers comparability and reproducibility of results, providing a possible explanation why these biomarkers do not reach the clinic. Conclusion: Potential prognostic methylation markers for RCC have been identified, but they require further validation in prospective studies to determine their true clinical value.
First draft submitted: 13 March 2017; Accepted for publication: 3 July 2017; Published online: 11 August 2017
Background
Renal cell carcinoma (RCC) is the most common malignancy of the kidney, and its incidence has been rising for the past few decades [Citation1]. Clear cell RCC (ccRCC) is the major histological subtype, accounting for 75–80% of all RCC cases [Citation2]. At time of diagnosis 20–30% of the patients present with distant metastases, and another 30% of all nonmetastatic ccRCC patients recur after (partial) nephrectomy and develop a lethal disease stage [Citation3]. The 5-year survival of distant metastasized ccRCC is as low as 12% [Citation4]. The currently used models for predicting patient outcome, such as the University of California Los Angeles (UCLA) Integrated Staging System (UISS) and the Stage Size Grade Necrosis (SSIGN) Risk Score [Citation5–8], are based on clinicopathological features (Tumor, Node, Metastasis [TNM] stage, tumor size, tumor grade, presence of necrosis and Eastern Cooperative Oncology Group [ECOG] performance status). Despite high predictive capacity of these models (C-statistics of 0.809 [Citation9] and 0.823 [Citation10], respectively), patients with similar clinicopathological features or risk scores can still have divergent outcomes [Citation11]. Therefore, addition of molecular markers to the current prognostic models could improve their prognostic value, which was demonstrated for ClearCode34 [Citation12].
It is becoming increasingly clear that epigenetic alterations, such as promoter methylation, play an important role in renal tumorigenesis by silencing tumor suppressor genes [Citation13]. This is illustrated by the relative high frequency of promoter methylation as compared to the number of somatic alterations in RCC [Citation14]. Furthermore, in contrast to somatic mutations in RCC, DNA methylation alterations are often shown to be associated with clinicopathological features and patient survival [Citation14,Citation15]. DNA methylation consists of the covalent addition of a methyl group to cytosines that precede a guanosine, the CpG dinucleotide, which are mainly clustered as CpG islands in the promoter region of genes [Citation13]. Promoter CpG island methylation represents a potential class of biomarkers for early detection, prognosis and predicting response to therapy [Citation16]. As promoter CpG island methylation markers reflect tumor biology, they might be able to identify RCC patients with more aggressive tumors who have high risk of metastatic disease and consequently poor prognosis. Identification of these high-risk patients could improve the management of RCC by directing these patients to adjuvant treatment trials, which are now ongoing [Citation17–19], and ultimately improve survival.
In recent years, a substantial amount of studies on the prognostic value of DNA methylation in RCC have been published. Here, we provide a systematic review of the literature on prognostic DNA methylation markers in RCC. We aimed to critically discuss the methodological quality of these biomarker studies and to select the most promising prognostic methylation markers for potential translation into clinic.
Materials & methods
This systematic review was performed according to the Preferred Reporting Items for Systematic Reviews and Meta-Analyses (PRISMA) statement [Citation20,Citation21], where applicable.
Eligibility criteria & study selection
Articles potentially eligible for this review were studies that reported on DNA methylation of one or more genes in RCC, which were assessed for their association with patient survival. The term DNA methylation in this review refers to CpG island methylation located in the promoter region of genes (i.e., around the transcription start site), but also to methylation at other locations in the genome.
The following inclusion criteria were applied: original article (not reviews, editorials, conference abstracts); English language; research was performed on RCC tissue samples or on bodily fluids derived from patients with RCC; and association of the methylation status of candidate genes with patient survival was investigated. Studies were excluded if they did not mention a specific methylation marker or performed clustering analysis based on (epi)genome-wide methylation data and only reported the association of a cluster with patient survival, without reporting methylation of specific genes. We did not restrict the inclusion of articles to specific study designs, to specific patient characteristics such as ethnicity, tumor subtype, disease stage or treatment information, or to the number of included patients.
Search strategy
We performed an electronic search of PubMed, EMBASE (via OvidSP) and MEDLINE (via OvidSP) up to April 2017 using the following search terms: renal cell carcinoma; kidney carcinoma; kidney neoplasms; kidney cancer; DNA methylation; methylation; prognosis; cancer prognosis; cancer survival. No additional limits were used. Furthermore, bibliographies of articles identified by the search were scanned to retrieve additional relevant publications.
Data collection & extraction
Data collection and extraction was performed using a standardized data registration form in which the following items were recorded: research group, year of publication, country, biomarker(s) studied, study design, source of patient material and population characteristics, tumor subtype studied, sample size, methods used to detect DNA methylation and to determine cut-off value, outcome measure(s) studied and statistical methods used, and reported association of the marker with patient outcome.
REMARK reporting & level of evidence assessment
The quality of the reporting of the selected articles was independently assessed by two authors (SC Joosten and IAG Deckers) using the criteria as formulated in the Reporting Recommendations for Tumor Marker Prognostic Studies (REMARK) guidelines [Citation22,Citation23].
The REMARK criteria consist of 20 items for good reporting of prognostic biomarker studies and are presented in Supplementary Table 1 (adapted from McShane et al. [Citation22]). Each item of the REMARK criteria can encompass several aspects, so a study was assigned one point if it stated all aspects of an item, 0.5 points if it mentioned some aspects and no points when an item was not reported on at all. The maximum score was 20 points. Based on the total score, studies were classified into three subgroups: studies with a score of 15 points or more (≥75% of the maximum score) were considered to have a high reporting quality according to REMARK; studies with an average reporting quality had a REMARK score between 5.5 and 14.5 points (25–75% of the maximum score); and ‘low reporting quality’ studies were studies with a REMARK score of 5 points or less (≤25% of the maximum score).
Scoring discrepancies between the two authors that led to changes in subgroup classification, were solved by consensus. This was the case in 6 out of 49 articles. If disagreement in scoring did not lead to differences in subgroup categorization, the score assigned by SC Joosten was considered leading.
Furthermore, studies were classified as category A (prospective randomized controlled trials), B (prospective trials not specifically designed to address biomarkers), C (prospective, observational studies) or D (retrospective, observational studies) and the level of evidence (LOE) for each biomarker was determined as proposed by Hayes et al. [Citation24] and Simon et al. [Citation25] (see Supplementary Table 2).
Results
Search results
An outline of the selection procedure is shown in . Searching the PubMed, EMBASE and MEDLINE databases initially yielded a total of 567 potentially relevant articles. After removal of duplicates, 369 articles remained and were screened for potential eligibility based on their title and abstract. This initial screen led to the exclusion of 221 articles. Of the remaining 148 articles, full text was retrieved. Furthermore, four articles were identified through screening of the reference lists and were also assessed based on full text. After reading the main text of these 152 articles, 103 articles were additionally discarded since they did not meet the predefined inclusion criteria (). This selection procedure resulted in 49 articles that were eventually included in this review.
Study characteristics
The characteristics of the included studies are summarized in Supplementary Table 3. The studies were conducted in 13 countries, of which 14 study populations were of Asian origin, 24 series were European, 9 study populations were American and two studies used both an Asian and an American study population. Studies were published between 2004 and 2017. The study sample size ranged from 16 to 993 patients, with a median of 113 patients (in one study the sample size was not provided). Twenty-six articles exclusively studied ccRCC, while one study only used papillary RCC (pRCC) samples and one study only chromophobe RCC tissue. The remaining 21 articles studied various subtypes of RCC. Of the 49 studies that reported on prognostic value of DNA methylation, 48 studies used tissue from the primary tumor and 1 study used cell-free DNA from blood [Citation26]. Only nine studies did not include matched adjacent tissue or normal renal tissue as control samples. In total, 14 different techniques were used to assess DNA methylation. Almost all articles studied methylation located in the promoter region of genes (46 studies), and from three articles it was not clear which genomic location was analyzed.
REMARK reporting assessment
The total REMARK scores ranged from 4.5 to 18 points, with an average of 11.7 points. Eight studies (16%) had a high REMARK score, 38 studies (78%) had an average REMARK score and 3 studies (6%) had a low REMARK score (Supplementary Table 3). In , the sum score per item of the REMARK criteria is depicted, which gives an overview of which items of the REMARK criteria are generally adequately or poorly reported among all the included studies.
REMARK: Reporting Recommendations for Tumor Marker Prognostic Studies.
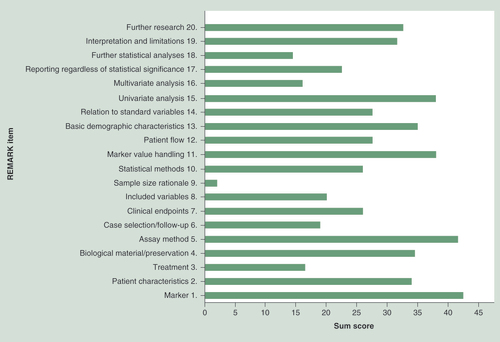
Overall, most studies adequately specified the examined marker and the objectives and hypothesis of their research. Studies that failed to do so, were mostly studies that used an (epi)genome wide approach to identify methylation markers, or studies in which identifying methylation markers was not the primary objective. The basic demographic characteristics of the included patients, the biological material that was used to study DNA methylation on, including the method of preservation and storage, assay methods, handling of marker values in the analyses (including method of cut point determination) and statistical methods were overall well reported, although methodology greatly varied among the different studies. All studies provided the results of univariate survival analyses, but results of survival analyses corrected for other potentially prognostic variables were often missing or not fully described. Strikingly, information on treatment that patients received lacked in almost all studies.
Whether case selection and follow-up was performed prospectively or retrospectively was also often not explicitly stated in the articles. We found only eight studies that clearly stated their study design. In order to categorize the studies as proposed by Hayes and Simon et al., we considered studies that reported a time period in the past during which tissue samples were collected or did not report any information on study design, to be retrospective. Consequently, 42 studies were considered as category D studies. There were seven (14%) category C studies, of which four studies retrospectively collected archived tissue from ccRCC cases identified in a prospective cohort study. We found no category A or B studies.
Study findings
& Supplementary Table 4 provide an overview of all the reported methylation markers and their association with RCC survival. For nine genes, that is SFRP1, BNC1, GREM1, RASSF1A, PCDH8, SCUBE3, GATA5, LAD1 and NEFH, promoter methylation was found to be associated with patient survival, and their prognostic value was independently validated in other studies ( & Supplementary Table 5).
Each dot represents one methylation marker. Large dots indicate methylation markers that have been independently validated in different studies, medium dots indicate that a methylation marker has been reported in one study but validated within this study in an additional patient cohort (internal validation) and small dots represent methylation markers that are reported by a single study in a single patient cohort.
Top left panel: Methylation markers with a statistically significant positive association with patient survival; Top right panel: Methylation markers with a nonsignificant positive association with patient survival; Bottom left panel: Methylation markers with a statistically significant negative association with patient survival; Bottom right panel: Methylation markers with a nonsignificant negative association with patient survival.
aMethylation marker panel: Three or more of the following genes methylated: p16, hMLH1, VHL, THBS-1, MINT1, MINT2, MINT12, MINT25 and MINT31.
bRisk score: Risk score based on a multivariate LASSO Cox regression model: Risk score = (0.0066 × PITX1) + (0.0034 × FOXE3) – (0.027 × TWF2) – (0.018 × EHBP1L1) – (0.03 × RIN1).
cMethylation marker panel: ≥16/23 CpG units methylated (encompassing seven genes, i.e., TRH, FAM150A, PRAC, SLC13A5, ZNF540, ZNF154 and ZNF671).
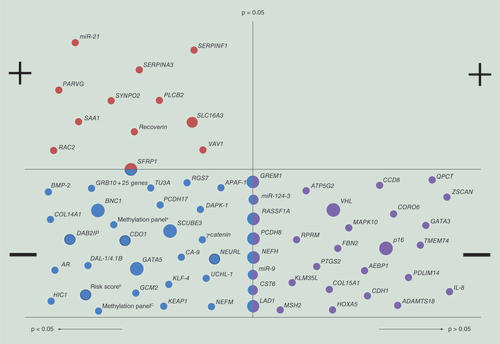
Forest plot of hazard ratios of patient survival associated with methylation of SFRP1 (A), BNC1 (B), GREM1 (C), SCUBE3 (D), GATA5 (E), RASSF1A (F), PCDH8 (G), LAD1 (H) and NEFH (I).
The x-axis shows the HR; the diamonds denote the HR and the horizontal lines represent 95% confidence intervals (CIs). The level of evidence for each marker is indicated in the lower right-hand corner.
aMorris 2010; bRicketts 2014, Infinium 27K cohort; cRicketts 2014, Infinium 450K cohort; dvan Vlodrop 2017, TCGA series; evan Vlodrop 2010, population-based series; fvan Vlodrop 2010, hospital-based series; gMorris, 2011; hPeters, 2014; iPeters 2012; jPeters, 2014; kvan Vlodrop 2017, population-based series; lvan Vlodrop 2017, hospital-based series.
95% CI: 95% confidence interval; DSS: Disease-specific survival; HR: Hazard ratio; KM: Kaplan–Meier curve; LOE: Level of evidence; Multi Cox: Multivariate Cox regression analysis; NA: Not available; OS: Overall survival; PFS: Progression-free survival; RFS: Recurrence-free survival; S/A Cox: Sex and age adjusted Cox regression analysis; Uni Cox: Univariate Cox regression analysis.
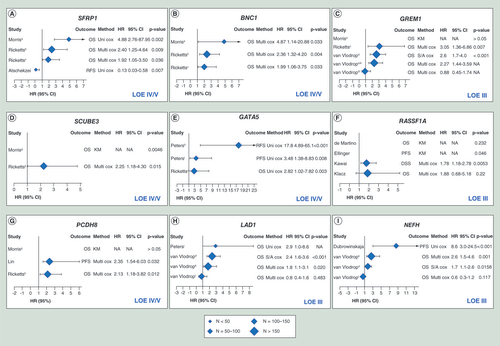
Promoter methylation of SFRP1 was associated with patient outcome in three different studies [Citation27–29]. Morris et al. [Citation27] performed a functional epigenome screen using high-density gene expression microarrays on 11 RCC cell lines. Of the identified genes, eight genes, including SFRP1, GREM1 and BNC1, were also frequently methylated in primary RCC samples. Promoter methylation of SFRP1 was associated with poorer overall survival (OS) (hazard ratio [HR]: 4.88; 95% confidence interval [CI]: 2.76–87.95; p = 0.002). These findings were confirmed by Ricketts et al. [Citation29] in two large cohorts derived from The Cancer Genome Atlas (TCGA) Kidney Renal Clear Cell Carcinoma (KIRC) project, in which they showed that promoter methylation of SFRP1 was an independent predictor of OS in both cohorts (HR: 2.40; 95% CI: 1.25–4.64 and HR: 1.92; 95% CI: 1.05–3.50). In contrast, a third study found that methylation of SFRP1 was associated with prolonged recurrence-free survival (RFS) (HR: 0.13; 95% CI: 0.03–0.58; p = 0.007) [Citation28] (Supplementary Table 5 & A). BNC1 was also identified as a gene that is frequently methylated in RCC and was associated with worse OS (HR: 4.87; 95% CI: 1.14–20.88; p = 0.033) [Citation27]. Promoter methylation of BNC1 was found to be an independent prognostic factor in the TCGA KIRC project [Citation29] (Supplementary Table 5 & B). Although GREM1 was frequently methylated in RCC, Morris and colleagues [Citation27] could not find an association with patient survival. In contrast, our group and Ricketts’ group [Citation29–31] identified promoter CpG island methylation of GREM1 as predictor of worse survival, even when corrected for other prognostic factors (HR: 3.05; 95% CI: 1.36–6.86 and HR: 2.27; 95% CI: 1.44–3.59, respectively) (Supplementary Table 5 & C). In a subsequent study of Morris’ group [Citation32], nine genes were identified to be frequently methylated in primary RCC tumor samples. Only methylation of SCUBE3 was found to be associated with increased risk of cancer death and relapse (p = 0.0046). The prognostic value of SCUBE3 was confirmed using data derived from the TCGA KIRC project [Citation29] (Supplementary Table 5 & D). In 2012, Peters et al. [Citation33] showed that promoter methylation of GATA5 is common in RCC and was associated with shorter progression-free survival (PFS), both in the overall RCC population (HR: 3.48; 95% CI: 1.38–8.83; p = 0.008) and in the ccRCC subgroup (HR: 4.59; 95% CI: 1.57–13.40; p = 0.005), which was confirmed in an independent patient cohort [Citation34]. Moreover, Ricketts and colleagues confirmed these results using data from the TCGA KIRC project [Citation29] (Supplementary Table 5 & E).
Besides for GREM1 and SFRP1, inconsistent results were also found for methylation of RASSF1A, PCDH8, LAD1 and NEFH and their association with patient outcome. Whereas Ellinger and colleagues [Citation35] and Kawai and colleagues [Citation36] were able to link promoter methylation of RASSF1A to shorter PFS in papillary RCC (p = 0.046) and shorter disease-specific survival (DSS) in ccRCC (multivariate HR: 1.78, 95% CI: 1.18–2.78, p = 0.0053), respectively, de Martino et al. [Citation26] could not find such an association when studying promoter methylation in cell-free DNA derived from blood samples from patients with various subtypes of RCC (p = 0.232). Recently, Klacz et al. [Citation37] found that promoter methylation of RASSF1A was associated with worse OS in patients with ccRCC in univariate analyses (HR: 3.00; 95% CI: 1.18–7.65; p = 0.02), however, this association was lost when correcting for tumor stage and grade in multivariate analyses (Supplementary Table 5 & F).
Methylation of PCDH8 was identified as an independent prognostic factor for PFS and OS by Lin et al. [Citation38] and Ricketts et al. [Citation29] (HR: 2.35; 95% CI: 1.54–6.03 and HR: 2.13; 95% CI: 1.18–3.82, respectively), but Morris’ group [Citation32] did not find any association with patient survival (Supplementary Table 5 & G).
In 2014, Peters et al. [Citation39] reported that methylation of LAD1 was associated with both shortened PFS and OS (HR: 6.4, 95% CI: 1.6–26.0 and HR: 2.9, 95% CI: 1.0–8.6, respectively) in RCC patients treated with antiangiogenic agents such as sunitinib, sorafenib, axitinib and bevacizumab. Recently, our group also demonstrated the prognostic value of methylation of LAD1 for patients with ccRCC [Citation31]. Promoter methylation of LAD1 was associated with poorer OS in a hospital-, population- and TCGA-based series (HR: 2.3; 95% CI: 1.2–4.3; HR: 2.3; 95% CI: 1.5–3.6 and HR: 2.4, 95% CI: 1.6–3.6, respectively), however, this association was lost in the hospital-based series when corrected for other prognostic variables (Supplementary Table 5 & H). These results indicate that methylation of LAD1 might be of potential clinical relevance for both prediction of prognosis as well as response to therapy. The same holds true for methylation of NEFH. Dubrowinskaja et al. [Citation40] found that methylation of NEFH not only predicted poorer PFS in patients with RCC (HR: 8.6, 95% CI: 3.0–24.5), but could also predict response to antiangiogenic therapy. The prognostic value of NEFH was confirmed by our group [Citation31] in the population- and TCGA-based series (multivariate HR: 2.6; 95% CI: 1.5–4.6 and sex/age adjusted HR: 1.7; 95% CI: 1.1–2.6, respectively), although again not in the hospital-based series (multivariate HR: 0.6; 95% CI: 0.3–1.2) (Supplementary Table 5 & I).
Furthermore, combination of methylation of GREM1, LAD1, NEFH and NEURL in a four-marker panel showed superior prognostic value as compared to the markers alone (Supplementary Table 4), indicating that the combination of several markers can improve predictive capacity. This was also shown by Wei et al. [Citation11] who developed a prognostic risk score based on a five-CpG-based-classifier encompassing methylation of PITX1, FOXE3, TWF2, RIN1 and EHBP1L1. Patients in the high-risk group had poorer OS as compared with patients in the low-risk group (HR: 4.27, 95% CI: 2.18–8.37), also when corrected for age, TNM stage, tumor grade and tumor necrosis (HR: 4.10; 95% CI: 2.05–8.19). These results were validated in three independent patient series, including in total up to almost 1000 patients, with comparable HRs of 1.80 (95% CI: 1.11–2.93, TCGA set), 3.36 (95% CI: 1.78–6.34, UTSW set) and 3.73 (95% CI: 2.28–6.09, MCHC set) (Supplementary Table 4). Furthermore, based on this five-CpG based classifier, patients within the same clinical stage could be successfully separated into subgroups with better and worse prognosis, providing prognostic information that complements the current staging system.
For several other genes statistically significant associations between methylation and patient survival were found, but were only reported in a single study [Citation27,Citation31,Citation39,Citation41–66], and for some other genes null associations were reported [Citation26–27,Citation32,Citation34–35,Citation39,Citation42,Citation67–70] ( & Supplementary Table 4).
Furthermore, it was demonstrated in several studies that promoter methylation of VHL [Citation26,Citation71–72] and p16 [Citation26,Citation73] has no impact on patient survival ( & Supplementary Table 4).
Discussion
In this systematic review, we performed an extensive search of the literature on prognostic methylation markers in RCC and we identified BNC1, SCUBE3, GATA5, SFRP1, GREM1, RASSF1A, PCDH8, LAD1 and NEFH as individual methylation markers that warrant further investigation of their prognostic value in patients with RCC. Promoter methylation of BNC1 [Citation27,Citation29] and SCUBE3 [Citation29,Citation32] was shown to be associated with poorer OS in two studies. Promoter CpG island methylation of GATA5 [Citation29,Citation33–34] was associated with poorer prognosis in three studies, although different end points were used. Promoter methylation of LAD1 [Citation31,Citation39] and NEFH [Citation31,Citation40] not only predicted patient survival, but also response to treatment with antiangiogenic agents. Conflicting results regarding the prognostic value of promoter methylation of SFRP1 [Citation27–29], PCDH8 [Citation29,Citation32,Citation38], GREM1 [Citation27,Citation29–30] and RASSF1A [Citation26,Citation35–37] were found. Several reasons for these inconsistent results can be adduced. The overarching issue is the observed methodological, clinical and statistical heterogeneity across the included studies.
The methodological heterogeneity is illustrated by the large variety in assay methods, with a total of 14 different techniques and also different thresholds used to asses DNA methylation. Moreover, also nonquantitative methods to detect DNA methylation were used, precluding the need for cut point determination. Besides choice of method and cut-off value, also the exact genomic location of the analyzed CpG dinucleotides is important [Citation74]. This was illustrated by van Vlodrop and colleagues [Citation30], who showed that GREM1 methylation varies within the promoter CpG island, with also methylation-region-specific effects on patient outcome.
In terms of the clinical heterogeneity, a lot of studies included various subtypes of RCC and often no subgroup analysis per tumor type was performed. This is important as the prognostic value of the studied methylation marker can vary according to the specific tumor subtype, which was demonstrated for methylation of RASSF1A [Citation26,Citation35–37]. Furthermore, only 30% of the studies specified whether patients had received systemic treatment. As this can influence disease outcome, it should be clearly stated which treatments patients received, and ideally, subgroup analyses of patients treated without systemic therapy (e.g., with assessing DFS) and of patients treated with the same systemic therapy should be performed [Citation23], to avoid confounding of the results. This is especially important in order to discriminate between the prognostic and predictive value of the investigated markers, as markers with predictive value have a different outcome related to a specific therapy. Moreover, it could be that methylation markers have combined prognostic and predictive value, as was demonstrated for methylation of NEFH [Citation31,Citation39] and LAD1 [Citation31,Citation40]. Additionally, most studies included in this review were retrospective and conducted in a small number of patients, with 21 out of 49 studies (43%) including <100 patients. Selecting patients based on the availability of tissue or patient data instead of on predefined in- and exclusion criteria, could lead to selection bias and thus to a study population that is not representative for the patient population [Citation23].
Another source of heterogeneity lies within statistical methodology. Besides great diversity in study end points used, we also observed lack of exact definition of these outcome measures in more than half of the studies. Absence of an uniform definition of clinical end points prevents results to be readily compared between studies [Citation23]. Effort to standardize time-to-event end points in RCC has already been made in the DATECAN project [Citation75], but needs to be implemented on a larger scale. Furthermore, the confounders assessed and corrected for differed from study to study. The prognostic value of a potential marker should always be adjusted for known prognostic variables, such as tumor stage, tumor size, Fuhrman grade and presence of necrosis, and preferably, evaluated in a multivariable prediction model to assess its incremental prognostic value compared to the current reference standard.
This multilevel heterogeneity influences the comparability and reproducibility of results, and hampers the validation of the biomarkers. The highest LOE found in this review was therefore only III (for GREM1, RASSF1A, LAD1 and NEFH). However, the fact that prognostic significance for these DNA methylation alterations is found in several studies despite the methodological, clinical and statistical heterogeneity, only further endorses the biological importance and the robustness of these markers. For SFRP1 [Citation27,Citation76], BNC1 [Citation27], GREM1, LAD1 and NEFH (unpublished data), SCUBE3 [Citation32] and RASSF1A [Citation77] tumor suppressor characteristics have been shown in RCC in vitro, which also further supports their value as possible prognostic markers.
The field of prognostic methylation marker research could greatly benefit from standardization of methylation analyses techniques, universal definition and use of clinical end points and collaboration between research groups to obtain larger patient cohorts. Moreover, despite the fact that criteria according to which prognostic biomarker studies have to report their results (i.e., the REMARK criteria) have already been installed, they have to be adopted progressively by the field. To this end, more and more journals include reporting according to REMARK as a prerequisite for publication of prognostic biomarker studies.
This systematic review has some limitations. First, we used the REMARK criteria to assess the quality of the reporting of the included articles. Because these criteria only say something about the reporting aspect of a study, they are not a measure of study quality as such, as reporting quality and study quality are not necessarily interchangeable. Furthermore, it should be noted here that the REMARK criteria tell us something about the quality of publications, but not about the potential quality of the biomarker per se. If a marker is described in a high-scoring study, but it cannot be validated by others, than implementation in the clinic will never be achieved. The question then rises whether the high-scoring studies indeed identified more methylation markers that were reproduced and validated. This does not appear to be the case since the average REMARK scores of the studies that reported on prognostic value of methylation of SFRP1, BNC1, GREM1, RASSF1A, PCDH8, SCUBE3, LAD1, NEFH and GATA5 is 11 (see also for average REMARK scores per marker). In this respect it should also be mentioned that assessment of reporting quality according to the REMARK criteria of studies that used a (epi)genome wide approach to identify methylation markers, or studies that used a large amount of candidate markers, was found to be troublesome as the REMARK criteria do not deal with the specific study design and statistical analyses that are required in these studies, and generally these studies therefore had lower REMARK scores. Furthermore, we selected markers to be ‘promising’, if their prognostic value was independently validated in other publications. It could be that in this way we missed methylation markers that are very promising, but are not yet reproduced by others. Finally, due to the extensive heterogeneity we were not able to perform meta-analyses to quantify the results, which makes interpretation of the prognostic value of each marker more difficult.
Conclusion & future perspective
In conclusion, BNC1, SCUBE3, GATA5, SFRP1, GREM1, RASSF1A, NEFH, LAD1 and PCDH8 have been identified as promising prognostic methylation markers for RCC in this systematic review, but they require further prospective validation and evaluation to assess their true biomarker potential before implementation in clinical practice can be considered.
This systematic review revealed some of the reasons why none of the so far published prognostic methylation markers for RCC are clinically successful, with extensive heterogeneity on several levels as overarching issue. These problems are not unique to the field of prognostic methylation marker research in RCC, but apply to the whole biomarker field in general. One step toward overcoming these problems is the establishment of the REMARK guidelines. However, we believe that in order to develop clinically successful prognostic methylation markers in the future, more rigorous changes are required. Standardization of methylation analyses techniques and marker values, and universal definition and use of clinical end points would be of great benefit. Also registration of planned (prognostic) biomarker studies in a database, accompanied by a prespecified protocol including a detailed description of the analysis methods, could help to reduce the number of overanalyzed studies that report specious findings in the future [Citation78]. Moreover, we think that more studies and research groups should focus on further validation of potential promising methylation markers, instead on further increasing the pool of methylation markers that have been reported in a single, underpowered study without any validation. In Box 1, we provide some recommendations for future studies on prognostic methylation markers. These recommendations are not exhaustive and should be used in addition to existing recommendations and guidelines such as REMARK. However, in our opinion, these recommendations represent the most important aspects specific for prognostic methylation marker studies.
If joint efforts are made to increase the quality of methylation biomarker research, we believe that methylation markers can become powerful tools in the management of several cancer types, including RCC.
Study design
– Study population
Include samples from one tumor subtype and TNM stage OR perform subgroup analysis for the different subtypes and stages.
Always include control samples (normal or normal adjacent tissue).
Specify whether patients received any (neo)adjuvant systemic treatment, if so, correct for these treatments in a multivariable analysis OR perform subgroup analysis per systemic agent.
Use a priori power analysis to calculate the minimum required sample size OR use alternative (post hoc) measures such as the minimal detectable hazard ratio (MDHR).
Use an independent study population to validate the results.
– Methylation analysis technique
If possible, use publicly available methylation data from The Cancer Genome Atlas to gain insight into the methylation pattern of the specific gene and to assess the best genomic location for designing the methylation marker assay.
When using primer-based techniques, be sure that the primers specifically amplify the region of interest and test this using tools such as BiSearch.
– Definition of end points
Use the end points and their definitions as they are established by the DATECAN Renal Cancer group.
– Statistical analysis
Correct for known prognostic variables such as tumor stage, tumor size, Fuhrman nuclear grade and the presence of necrosis, by performing multivariable analysis.
Evaluate the prognostic value of the methylation marker in a multivariable prediction model and assess its incremental prognostic value compared to the current reference standard (such as the Stage Size Grade Necrosis [SSIGN] score and the UCLA Integrated Staging System [UISS]).
Study reporting
Adhere to existing guidelines and checklists such as REMARK, CONSORT, STROBE, TRIPOD.
General recommendations
Check the literature for previous studies reporting the prognostic value of a specific methylation marker and establish its current level of evidence (LOE). Check whether your planned study will indeed contribute to the current LOE, if not, consider changing the study design to increase the LOE for that specific marker.
Systematic review of the literature on prognostic methylation markers for renal cell carcinoma
Forty-nine articles that studied the prognostic value of DNA methylation of one or more genes in renal cell carcinoma (RCC) were included.
In total, these studies reported over 70 potential prognostic methylation markers for RCC.
REMARK scoring
Scoring of the articles included in the systematic review according to the REMARK guidelines reveals incomplete reporting in almost all studies.
REMARK scoring also reveals substantial methodological heterogeneity among the studies, including characteristics of patient series, methylation detection techniques, determination of marker cut-off values, use of clinical end points and method of statistical analysis.
Promising prognostic methylation markers for RCC
Despite this extensive heterogeneity, BNC1, SCUBE3, GATA5, SFRP1, GREM1, RASSF1A, NEFH, LAD1 and PCDH8 are identified as promising prognostic methylation markers for RCC, since their prognostic value was independently validated in different studies.
Conclusion
BNC1, SCUBE3, GATA5, SFRP1, GREM1, RASSF1A, NEFH, LAD1 and PCDH8 are prognostic methylation markers for RCC that hold promise for the future, but they require further prospective validation.
Extensive methodological, clinical and statistical heterogeneity among studies on prognostic methylation markers for RCC, prevents translation of these markers to the clinic.
Rigorous changes in the (methylation) biomarker research field, such as standardization of methodology, is needed to bring more markers to the clinic.
Supplemental Table 1
Download MS Word (17.9 KB)Supplemental Table 2
Download MS Word (29.5 KB)Supplemental Table 3
Download MS Word (161 KB)Supplemental Table 4
Download MS Word (350.5 KB)Supplemental Table 5
Download MS Word (31.6 KB)Financial & competing interests disclosure
The authors have no relevant affiliations or financial involvement with any organization or entity with a financial interest in or financial conflict with the subject matter or materials discussed in the manuscript. This includes employment, consultancies, honoraria, stock ownership or options, expert testimony, grants or patents received or pending, or royalties.
No writing assistance was utilized in the production of this manuscript.
Supplementary data
To view the supplementary data that accompany this paper please visit the journal website at: www.tandfonline.com/doi/suppl/10.2217/3dp-2022-0019
References
- Ferlay J , SoerjomataramI , DikshitRet al. Cancer incidence and mortality worldwide: sources, methods and major patterns in GLOBOCAN 2012 . Int. J. Cancer136 ( 5 ), E359 – E386 ( 2015 ).
- Lopez-Beltran A , CarrascoJC , ChengLet al. 2009 update on the classification of renal epithelial tumors in adults . Int. J. Urol.16 ( 5 ), 432 – 443 ( 2009 ).
- Rydzanicz M , WrzesinskiT , BluyssenHA , WesolyJ . Genomics and epigenomics of clear cell renal cell carcinoma: recent developments and potential applications . Cancer Lett.341 ( 2 ), 111 – 126 ( 2013 ).
- American Cancer Society . Cancer Facts & Fetals 2015 ( 2015 ). www.cancer.org/research/cancerfactsstatistics/cancerfactsfetals2015/index
- Kattan MW , ReuterV , MotzerRJ , KatzJ , RussoP . A postoperative prognostic nomogram for renal cell carcinoma . J. Urol.166 ( 1 ), 63 – 67 ( 2001 ).
- Frank I , BluteML , ChevilleJCet al. An outcome prediction model for patients with clear cell renal cell carcinoma treated with radical nephrectomy based on tumor stage, size, grade and necrosis: the SSIGN score . J. Urol.168 ( 6 ), 2395 – 2400 ( 2002 ).
- Zisman A , PantuckAJ , DoreyFet al. Improved prognostication of renal cell carcinoma using an integrated staging system . J. Clin. Oncol.19 ( 6 ), 1649 – 1657 ( 2001 ).
- Zisman A , PantuckAJ , WiederJet al. Risk group assessment and clinical outcome algorithm to predict the natural history of patients with surgically resected renal cell carcinoma . J. Clin. Oncol.20 ( 23 ), 4559 – 4566 ( 2002 ).
- Patard JJ , KimHL , LamJSet al. Use of the University of California Los Angeles integrated staging system to predict survival in renal cell carcinoma: an international multicenter study . J. Clin. Oncol.22 ( 16 ), 3316 – 3322 ( 2004 ).
- Zigeuner R , HuttererG , ChromeckiTet al. External validation of the Mayo Clinic stage, size, grade, and necrosis (SSIGN) score for clear-cell renal cell carcinoma in a single European centre applying routine pathology . Eur. Urol.57 ( 1 ), 102 – 109 ( 2010 ).
- Wei JH , HaddadA , WuKJet al. A CpG-methylation-based assay to predict survival in clear cell renal cell carcinoma . Nat. Commun.6 , 8699 ( 2015 ).
- Brooks SA , BrannonAR , ParkerJSet al. ClearCode34: a prognostic risk predictor for localized clear cell renal cell carcinoma . Eur. Urol.66 ( 1 ), 77 – 84 ( 2014 ).
- Baylin SB . DNA methylation and gene silencing in cancer . Nat. Clin. Pract. Oncol.2 ( Suppl. 1 ), S4 – S11 ( 2005 ).
- Morris MR , MaherER . Epigenetics of renal cell carcinoma: the path towards new diagnostics and therapeutics . Genome Med.2 ( 9 ), 59 ( 2010 ).
- Morris MR , LatifF . The epigenetic landscape of renal cancer . Nat. Rev. Nephrol.13 ( 1 ), 47 – 60 ( 2017 ).
- Laird PW . The power and the promise of DNA methylation markers . Nat. Rev. Cancer3 ( 4 ), 253 – 266 ( 2003 ).
- Haas NB , ManolaJ , UzzoRGet al. Adjuvant sunitinib or sorafenib for high-risk, non-metastatic renal-cell carcinoma (ECOG-ACRIN E2805): a double-blind, placebo-controlled, randomised, Phase III trial . Lancet387 ( 10032 ), 2008 – 2016 ( 2016 ).
- Ravaud A , MotzerRJ , PandhaHSet al. Adjuvant sunitinib in high-risk renal-cell carcinoma after nephrectomy . N. Engl. J. Med.375 ( 23 ), 2246 – 2254 ( 2016 ).
- Patel DN , FiglinRA , KimHL . Adjuvant treatment for renal cell carcinoma: do we finally have a major breakthrough?Clin. Adv. Hematol. Oncol.14 ( 11 ), 907 – 914 ( 2016 ).
- Moher D , LiberatiA , TetzlaffJ , AltmanDG . Preferred reporting items for systematic reviews and meta-analyses: the PRISMA statement . PLoS Med.6 ( 7 ), e1000097 ( 2009 ).
- Liberati A , AltmanDG , TetzlaffJet al. The PRISMA statement for reporting systematic reviews and meta-analyses of studies that evaluate health care interventions: explanation and elaboration . PLoS Med.6 ( 7 ), e1000100 ( 2009 ).
- McShane LM , AltmanDG , SauerbreiWet al. Reporting recommendations for tumor marker prognostic studies (REMARK) . J. Natl Cancer Inst.97 ( 16 ), 1180 – 1184 ( 2005 ).
- Altman DG , McShaneLM , SauerbreiW , TaubeSE . Reporting recommendations for tumor marker prognostic studies (REMARK): explanation and elaboration . BMC Med.10 , 51 ( 2012 ).
- Hayes DF , BastRC , DeschCEet al. Tumor marker utility grading system: a framework to evaluate clinical utility of tumor markers . J. Natl Cancer Inst.88 ( 20 ), 1456 – 1466 ( 1996 ).
- Simon RM , PaikS , HayesDF . Use of archived specimens in evaluation of prognostic and predictive biomarkers . J. Natl. Cancer Inst.101 ( 21 ), 1446 – 1452 ( 2009 ).
- de Martino M , KlatteT , HaitelA , MarbergerM . Serum cell-free DNA in renal cell carcinoma: a diagnostic and prognostic marker . Cancer118 ( 1 ), 82 – 90 ( 2012 ).
- Morris MR , RickettsC , GentleDet al. Identification of candidate tumour suppressor genes frequently methylated in renal cell carcinoma . Oncogene29 ( 14 ), 2104 – 2117 ( 2010 ).
- Atschekzei F , HennenlotterJ , JanischSet al. SFRP1 CpG island methylation locus is associated with renal cell cancer susceptibility and disease recurrence . Epigenetics7 ( 5 ), 447 – 457 ( 2012 ).
- Ricketts CJ , HillVK , LinehanWM . Tumor-specific hypermethylation of epigenetic biomarkers, including SFRP1, predicts for poorer survival in patients from the TCGA kidney renal clear cell carcinoma (KIRC) project . PLoS ONE , 9 ( 1 ), e85621 ( 2014 ).
- van Vlodrop IJ , BaldewijnsMM , SmitsKMet al. Prognostic significance of Gremlin1 (GREM1) promoter CpG island hypermethylation in clear cell renal cell carcinoma . Am. J. Pathol.176 ( 2 ), 575 – 584 ( 2010 ).
- van Vlodrop IJH , JoostenSC , De MeyerTet al. A four-gene promoter methylation marker panel consisting of GREM1, NEURL, LAD1, and NEFH predicts survival of clear cell renal cell cancer patients . Clin. Cancer Res.23 ( 8 ), 2006 – 2018 ( 2017 ).
- Morris MR , RickettsCJ , GentleDet al. Genome-wide methylation analysis identifies epigenetically inactivated candidate tumour suppressor genes in renal cell carcinoma . Oncogene30 ( 12 ), 1390 – 1401 ( 2011 ).
- Peters I , EggersH , AtschekzeiFet al. GATA5 CpG island methylation in renal cell cancer: a potential biomarker for metastasis and disease progression . BJU Int.110 ( 2, Pt 2 ), E144 – E152 ( 2012 ).
- Peters I , GebauerK , DubrowinskajaNet al. GATA5 CpG island hypermethylation is an independent predictor for poor clinical outcome in renal cell carcinoma . Oncol. Rep.31 ( 4 ), 1523 – 1530 ( 2014 ).
- Ellinger J , HollD , NuhnPet al. DNA hypermethylation in papillary renal cell carcinoma . BJU Int.107 ( 4 ), 664 – 669 ( 2011 ).
- Kawai Y , SakanoS , SuehiroYet al. Methylation level of the RASSF1A promoter is an independent prognostic factor for clear-cell renal cell carcinoma . Ann. Oncol.21 ( 8 ), 1612 – 1617 ( 2010 ).
- Klacz J , WierzbickiPM , WronskaAet al. Decreased expression of RASSF1A tumor suppressor gene is associated with worse prognosis in clear cell renal cell carcinoma . Int. J. Oncol.48 ( 1 ), 55 – 66 ( 2016 ).
- Lin YL , WangYL , FuXL , MaJG . Aberrant methylation of PCDH8 is a potential prognostic biomarker for patients with clear cell renal cell carcinoma . Med. Sci. Monit.20 , 2380 – 2385 ( 2014 ).
- Peters I , DubrowinskajaN , AbbasMet al. DNA methylation biomarkers predict progression-free and overall survival of metastatic renal cell cancer (mRCC) treated with antiangiogenic therapies . PLoS ONE9 ( 3 ), e91440 ( 2014 ).
- Dubrowinskaja N , GebauerK , PetersIet al. Neurofilament heavy polypeptide CpG island methylation associates with prognosis of renal cell carcinoma and prediction of antivascular endothelial growth factor therapy response . Cancer Med.3 ( 2 ), 300 – 309 ( 2014 ).
- Yamada D , KikuchiS , WilliamsYNet al. Promoter hypermethylation of the potential tumor suppressor DAL-1/4.1B gene in renal clear cell carcinoma . Int. J. Cancer118 ( 4 ), 916 – 923 ( 2006 ).
- Ricketts CJ , MorrisMR , GentleDet al. Methylation profiling and evaluation of demethylating therapy in renal cell carcinoma . Clin. Epigenetics5 ( 1 ), 16 ( 2013 ).
- Li H , WangJ , XiaoWet al. Epigenetic alterations of Kruppel-like factor 4 and its tumor suppressor function in renal cell carcinoma . Carcinogenesis34 ( 10 ), 2262 – 2270 ( 2013 ).
- Kagara I , EnokidaH , KawakamiKet al. CpG hypermethylation of the UCHL1 gene promoter is associated with pathogenesis and poor prognosis in renal cell carcinoma . J. Urol.180 ( 1 ), 343 – 351 ( 2008 ).
- Hildebrandt MA , GuJ , LinJet al. Hsa-miR-9 methylation status is associated with cancer development and metastatic recurrence in patients with clear cell renal cell carcinoma . Oncogene29 ( 42 ), 5724 – 5728 ( 2010 ).
- Girgis AH , IakovlevVV , BeheshtiBet al. Multilevel whole-genome analysis reveals candidate biomarkers in clear cell renal cell carcinoma . Cancer Res.72 ( 20 ), 5273 – 5284 ( 2012 ).
- Gebauer K , PetersI , DubrowinskajaNet al. Hsa-mir-124–3 CpG island methylation is associated with advanced tumours and disease recurrence of patients with clear cell renal cell carcinoma . Br. J. Cancer108 ( 1 ), 131 – 138 ( 2013 ).
- Fisel P , KruckS , WinterSet al. DNA methylation of the SLC16A3 promoter regulates expression of the human lactate transporter MCT4 in renal cancer with consequences for clinical outcome . Clin. Cancer Res.19 ( 18 ), 5170 – 5181 ( 2013 ).
- Fisel P , StuhlerV , BedkeJet al. MCT4 surpasses the prognostic relevance of the ancillary protein CD147 in clear cell renal cell carcinoma . Oncotarget6 ( 31 ), 30615 – 30627 ( 2015 ).
- Eggers H , SteffensS , GrosshennigAet al. Prognostic and diagnostic relevance of hypermethylated in cancer 1 (HIC1) CpG island methylation in renal cell carcinoma . Int. J. Oncol.40 ( 5 ), 1650 – 1658 ( 2012 ).
- Deckers IA , SchoutenLJ , Van NesteLet al. Promoter methylation of CDO1 identifies clear-cell renal cell cancer patients with poor survival outcome . Clin. Cancer Res.21 ( 15 ), 3492 – 3500 ( 2015 ).
- Christoph F , WeikertS , KempkensteffenCet al. Promoter hypermethylation profile of kidney cancer with new proapoptotic p53 target genes and clinical implications . Clin. Cancer Res.12 ( 17 ), 5040 – 5046 ( 2006 ).
- Breault JE , ShiinaH , IgawaMet al. Methylation of the gamma-catenin gene is associated with poor prognosis of renal cell carcinoma . Clin. Cancer Res.11 ( 2, Pt 1 ), 557 – 564 ( 2005 ).
- Awakura Y , NakamuraE , ItoN , KamotoT , OgawaO . Methylation-associated silencing of TU3A in human cancers . Int. J. Oncol.33 ( 4 ), 893 – 899 ( 2008 ).
- Cancer Genome Atlas Research Network . Comprehensive molecular characterization of clear cell renal cell carcinoma . Nature499 ( 7456 ), 43 – 49 ( 2013 ).
- Golovastova MO , TsoyLV , BocharnikovaAVet al. The cancer-retina antigen recoverin as a potential biomarker for renal tumors . Tumour Biol.37 ( 7 ), 9899 – 9907 ( 2016 ).
- Lin YL , GuiSL , GuoH , MaJG , LiWP . Protocadherin17 promoter methylation is a potential predictive biomarker in clear cell renal cell carcinoma . Med. Sci. Monit.21 , 2870 – 2876 ( 2015 ).
- Mitsui Y , HirataH , ArichiNet al. Inactivation of bone morphogenetic protein 2 may predict clinical outcome and poor overall survival for renal cell carcinoma through epigenetic pathways . Oncotarget6 ( 11 ), 9577 – 9591 ( 2015 ).
- Zhao H , LeppertJT , PeehlDM . A protective role for androgen receptor in clear cell renal cell carcinoma based on mining TCGA data . PLoS ONE11 ( 1 ), e0146505 ( 2016 ).
- Chen G , WangY , WangL , XuW . Identifying prognostic biomarkers based on aberrant DNA methylation in kidney renal clear cell carcinoma . Oncotarget8 ( 3 ), 5268 – 5280 ( 2017 ).
- Fabrizio FP , CostantiniM , CopettiMet al. Keap1/Nrf2 pathway in kidney cancer: frequent methylation of KEAP1 gene promoter in clear renal cell carcinoma . Oncotarget8 ( 7 ), 11187 – 11198 ( 2017 ).
- Pompas-Veganzones N , SandonisV , Perez-LanzacAet al. Myopodin methylation is a prognostic biomarker and predicts antiangiogenic response in advanced kidney cancer . Tumour Biol.37 ( 10 ), 14301 – 14310 ( 2016 ).
- Wang ZR , WeiJH , ZhouJCet al. Validation of DAB2IP methylation and its relative significance in predicting outcome in renal cell carcinoma . Oncotarget7 ( 21 ), 31508 – 31519 ( 2016 ).
- Arai E , KanaiY , UshijimaSet al. Regional DNA hypermethylation and DNA methyltransferase (DNMT) 1 protein overexpression in both renal tumors and corresponding nontumorous renal tissues . Int. J. Cancer119 ( 2 ), 288 – 296 ( 2006 ).
- Tian Y , AraiE , GotohMet al. Prognostication of patients with clear cell renal cell carcinomas based on quantification of DNA methylation levels of CpG island methylator phenotype marker genes . BMC Cancer14 , 772 ( 2014 ).
- Urakami S , ShiinaH , EnokidaHet al. Wnt antagonist family genes as biomarkers for diagnosis, staging, and prognosis of renal cell carcinoma using tumor and serum DNA . Clin. Cancer Res.12 ( 23 ), 6989 – 6997 ( 2006 ).
- Xu B , ZhangL , LuoCet al. Hypermethylation of the 16q23.1 tumor suppressor gene ADAMTS18 in clear cell renal cell carcinoma . Int. J. Mol. Sci.16 ( 1 ), 1051 – 1065 ( 2015 ).
- Yoo KH , ParkYK , ChangSG . DNA hypomethylation of interleukin 8 in clear cell renal cell carcinoma . Oncol. Lett.5 ( 1 ), 39 – 42 ( 2013 ).
- Yoo KH , ParkYK , KimHS , JungWW , ChangSG . Epigenetic inactivation of HOXA5 and MSH2 gene in clear cell renal cell carcinoma . Pathol. Int.60 ( 10 ), 661 – 666 ( 2010 ).
- Yoo KH , ParkYK , KimHS , JungWW , ChangSG . Identification of MAPK10 as a novel epigenetic marker for chromophobe kidney cancer . Pathol. Int.61 ( 1 ), 52 – 54 ( 2011 ).
- Smits KM , SchoutenLJ , van DijkBAet al. Genetic and epigenetic alterations in the von hippel-lindau gene: the influence on renal cancer prognosis . Clin. Cancer Res.14 ( 3 ), 782 – 787 ( 2008 ).
- Young AC , CravenRA , CohenDet al. Analysis of VHL gene alterations and their relationship to clinical parameters in sporadic conventional renal cell carcinoma . Clin. Cancer Res.15 ( 24 ), 7582 – 7592 ( 2009 ).
- Vidaurreta M , MaestroML , Sanz-CaslaMTet al. Inactivation of p16 by CpG hypermethylation in renal cell carcinoma . Urol. Oncol.26 ( 3 ), 239 – 245 ( 2008 ).
- van Vlodrop IJ , NiessenHE , DerksSet al. Analysis of promoter CpG island hypermethylation in cancer: location, location, location! Clin. Cancer Res. 17 ( 13 ), 4225 – 4231 ( 2011 ).
- Kramar A , NegrierS , SylvesterRet al. Guidelines for the definition of time-to-event end points in renal cell cancer clinical trials: results of the DATECAN project . Ann. Oncol.26 ( 12 ), 2392 – 2398 ( 2015 ).
- Gumz ML , ZouH , KreinestPAet al. Secreted frizzled-related protein 1 loss contributes to tumor phenotype of clear cell renal cell carcinoma . Clin. Cancer Res.13 ( 16 ), 4740 – 4749 ( 2007 ).
- Dreijerink K , BragaE , KuzminIet al. The candidate tumor suppressor gene, RASSF1A, from human chromosome 3p21.3 is involved in kidney tumorigenesis . Proc. Natl Acad. Sci. USA98 ( 13 ), 7504 – 7509 ( 2001 ).
- McShane LM , AltmanDG , SauerbreiW . Identification of clinically useful cancer prognostic factors: what are we missing?J. Natl Cancer Inst.97 ( 14 ), 1023 – 1025 ( 2005 ).