Abstract
Aim: To identify differential methylation related to prescribed opioid use. Methods: This study examined whether blood DNA methylation, measured using Illumina arrays, differs by recent opioid medication use in four population-based cohorts. We meta-analyzed results (282 users; 10,560 nonusers) using inverse-variance weighting. Results: Differential methylation (false discovery rate <0.05) was observed at six CpGs annotated to the following genes: KIAA0226, CPLX2, TDRP, RNF38, TTC23 and GPR179. Integrative epigenomic analyses linked implicated loci to regulatory elements in blood and/or brain. Additionally, 74 CpGs were differentially methylated in males or females. Methylation at significant CpGs correlated with gene expression in blood and/or brain. Conclusion: This study identified DNA methylation related to opioid medication use in general populations. The results could inform the development of blood methylation biomarkers of opioid use.
Opioid use and dependence are major public health concerns. Opioid medications are prescribed to treat moderate-to-severe pain because they bind to opioid receptors to reduce pain intensity [Citation1]. Approximately 70% of drug overdose-related deaths in the USA involve opioids, including prescription opioids [Citation2]. Improved understanding of biological responses to prescription opioids could reduce these adverse consequences.
DNA methylation is an epigenetic mechanism that can influence gene expression without changing the underlying genetic sequence. Epigenome-wide association studies (EWAS) have shown that DNA methylation in blood can be altered by substance use such as cigarette smoking [Citation3] and alcohol consumption [Citation4]. The extensive methylation signals for these substances have led to the development of biomarkers of habitual use [Citation4,Citation5].
Some small to moderately sized studies (n ≤ 370) have reported methylation alterations related to opioid dependence, defined by the Diagnostic and Statistical Manual of Mental Disorders, fourth edition, criteria [Citation6–8], or to opioid use [Citation9,Citation10]. Candidate gene studies of the OPRM1 gene reported hypermethylation related to opioid dependence or use [Citation6,Citation7,Citation9–11]. An EWAS identified three CpG probes meeting genome-wide significance related to opioid dependence [Citation8]. To date, there have been no large-scale meta-analyses of genome-wide blood DNA methylation and opioid medication use.
Sex differences in pain and analgesia have been reported [Citation12,Citation13]. A recent large study found that men had a greater risk than women for opioid overdose after their initial prescription [Citation14]. In one study of opioid prescriptions to treat noncancer-related pain, men were more likely than women to escalate into higher-dose treatments and die of opioid-related causes [Citation15]. Two of the five candidate gene methylation studies focusing on the OPRM1 gene were limited to men (n < 120) [Citation6,Citation11], and the sole EWAS reporting three CpGs of genome-wide significance was conducted only in women (n = 220) [Citation8]. No studies have reported well-validated DNA methylation alterations related to opioid use using large-population studies or exploring whether associations between opioid use and DNA methylation differ by sex.
This study focused on the use of opioid medications and excluded illicit opioid use. Because any intake of opioid medications could provide an individual a chance to become a chronic user or develop a misuse disorder, generating an accurate biomarker to help identify individuals exposed to opioid medications is essential in opioid- and/or health effects-related research.
We conducted a coordinated, epigenome-wide association meta-analysis across five datasets (10,842 individuals comprising 282 opioid users and 10,560 nonusers), including four of European ancestry and one of African ancestry, from cohorts in the USA and Scotland to identify CpGs differentially methylated in relation to recent opioid medication use. Additionally, we explored whether opioid-related differential methylation differs by sex. To examine the functional implications of identified CpGs, we evaluated associations with nearby gene expression in blood (n = 3684) and brain (n = 515) and overlaps with histone marks and transcription factor (TF) binding motifs. This study of blood DNA methylation in general population studies could contribute to biomarker development and an improved understanding of the epigenomic mechanisms of response to opioid medications.
Materials & methods
Study population
This meta-analysis included 10,842 adults (≥30 years old) from five datasets, within the framework of the Cohorts for Heart and Aging Research in Genomic Epidemiology consortium. Participating studies included the Agricultural Lung Health Study (ALHS), Framingham Heart Study (FHS), Generation Scotland (GS Set1 and Set2), and Genetic Epidemiology Network of Arteriopathy (GENOA). Study participants were mainly of European ancestry (91% of the 10,842 adults). African-ancestry individuals were from GENOA. Each study was approved by its institutional review board. All study participants provided written informed consent.
Recent opioid medication use
The harmonized definition across studies categorized any recent use of opioid medications as exposed (users). In brief, ALHS participants, enrolled between 2009 and 2013, were asked at home visits to provide all prescription and nonprescription medications used in the last 7 days and field technicians recorded the medication names, which were coded using the Slone Drug Dictionary [Citation16], which links drug names to active ingredients and drug classes based on Pharmacologic-Therapeutic Classification from the American Hospital Formulary Service. Recent opioid use was classified based on any use of full opioid agonists in the past 7 days versus no use. In the FHS, participants were asked to bring all prescription and nonprescription medications taken regularly in the past month in their original containers to the study visit. Medication names were converted into Anatomical Therapeutic Chemical codes. Recent opioid use (users vs nonusers) was based on any use of full opioid agonists in the past month. In GS, participants reported prescription and nonprescription medications they regularly took. Any use of opioid medications was employed to define recent opioid use. In GENOA, participants were asked “During the last month, have you used any medication that was prescribed or recommended by a physician?”; if they responded ”yes”, they provided the names of the medications. The Medi-Span Therapeutic Classification was used to identify pharmacologic classes of prescriptions. Participants reporting any use of opioid medications were categorized as recent opioid users. In each study, participants who did not report any use of opioids were categorized as unexposed (nonusers).
DNA methylation
We measured genome-wide methylation in blood, collected at the time of the medication questionnaires, using Illumina methylation arrays. FHS used the Illumina HumanMethylation450 BeadChip (450K array; Illumina, Inc., CA, USA) and the other studies used the newer MethylationEPIC BeadChip (EPIC/850K array; Illumina, Inc.). Each study performed preprocessing and quality control filtering, including the removal of methylation values based on detection P, exclusion of poor-quality CpGs and samples, probe-type bias normalization and correction for batch effects. Additionally, we excluded CpGs previously reported as potentially problematic [Citation17], including “ch” probes, probes having an SNP in the extension base or color channel issues, and cross-reactive probes. Autosomal CpGs that passed these quality control steps were included in association analyses.
Differential methylation in relation to opioid use
Each study assessed associations of recent opioid medication use (opioid users vs nonusers) as the predictor with methylation, ranging between 0 (unmethylated) and 1 (methylated) as the response, using robust linear regression to account for potential heteroskedasticity and lessen the impacts of influential outliers on association results. To further reduce potential impacts of extreme outliers, we used winsorization (0.25% on both sides) [Citation18]. Each study adjusted for potential confounders, including age, sex, BMI, smoking (never/former/current as dummy variables), smoking pack-years, ancestry principal components based on genome-wide genetic variants and estimated cell-type proportions (monocyte, B cell, natural killer cell, CD8+ T cell, CD4+ T cell and granulocyte) [Citation19]. Additionally, the studies adjusted for analytic batch, study site and any study-specific selection factor or accounted for family relationships where appropriate. We meta-analyzed study-specific results using inverse-variance weighted fixed-effects models [Citation20,Citation21], including 778,492 CpGs analyzed in two or more datasets.
Additionally, we explored whether differential methylation related to opioid use differed by sex using studies having more than 15 opioid users in both sex groups, which limited the analysis to ALHS and FHS. Because large-sample statistics were used, we set a threshold of 15 in the exposed group within each analysis based on prior experience in consortium epigenomic meta-analyses. We analyzed the data separately in males and females using robust linear regression, adding a product term for opioid use × sex to a model with both main effects in all participants and with the same covariates as above. We meta-analyzed the results using inverse-variance weighting [Citation20,Citation21], including the 394,524 CpGs overlapping between EPIC/850K array (ALHS) and 450K array (FHS).
To account for multiple testing, we set a genome-wide significance threshold of a Benjamini–Hochberg false discovery rate (FDR) of 0.05 [Citation22]. Unless otherwise noted, CpGs of genome-wide significance refer to those with FDR < 0.05.
We also evaluated differentially methylated regions (DMRs) in relation to opioid use using the comb-p method [Citation23] implemented in the ENmix R package [Citation24]. EWAS meta-analysis results were used as the input. Comb-p combines irregularly spaced adjacent p-values to identify regions containing consecutive low p-values [Citation23]. Regional p-values were corrected for multiple testing using Šidák (default option) [Citation25]. We set a threshold of Šidák P < 0.05 for significant genome-wide DMRs. The DMR analysis complements individual CpG level analysis, as the method aggregates signals across multiple nearby CpGs and a DMR of statistical significance does not necessarily contain a CpG having genome-wide significance.
Functional & biological implications
Using the eFORGE integrative epigenomic approach [Citation26,Citation27], we explored whether our top 1000 CpGs (default option) were enriched in regulatory elements from the Roadmap Epigenomics Consortium [Citation28] across over 20 tissue types. To evaluate overlaps between the significant CpGs and TF binding motifs in the brain, we used eFORGE-TF [Citation26]. Additionally, we examined enrichment of TF motifs in implicated loci (±250 bp) using MEME Suite [Citation29].
To further evaluate the biological context of the methylation findings, we tested enrichment of pathways using robust rank aggregation [Citation30] and preranked gene set enrichment analysis [Citation31], which account for differing numbers of CpGs per gene on the methylation arrays. We used the methylRRA function in the methylGSA [Citation32] R package and reported pathways enriched at nominal significance (p < 0.05). Pathways were from the Kyoto Encyclopedia of Genes and Genomes gene sets via the org.Hs.eg.db [Citation33] R package.
Opioid-related CpGs & nearby gene expression: cis expression quantitative trait methylation analysis
To evaluate functional impacts of genome-wide significant CpGs, we assessed whether methylation at identified CpGs was associated with expression of nearby (±250 kb) genes: cis-expression quantitative trait methylation (eQTM). Linear regression was used to examine associations between methylation (predictor) and the expression of nearby genes (response). To identify cis-eQTMs in blood, we used paired DNA methylation (450K) and gene expression (Affymetrix Human Exon 1.0 ST GeneChip Platform) from FHS [Citation34] (n = 3684). For cis-eQTMs in the brain, we used paired DNA methylation (450K) and gene expression (Illumina HiSeq) from ROS/MAP [Citation35] (n = 515). We set a threshold of FDR < 0.05 for statistical significance.
Opioid-related CpGs & nearby genetic variants: cis methylation quantitative trait loci analysis
To examine whether genome-wide significant CpGs in all participants showed associations with nearby (±500 kb) genetic variants, we used the GoDMC database (N = 32,851 individuals) [Citation36] of methylation quantitative trait loci (meQTL).
Look-up of previously reported opioid-related methylation in our data
Because few data exist on genome-wide DNA methylation and opioid use, we attempted to validate findings from a study using saliva samples [Citation10] in our analysis of blood. We looked up CpGs reported (p < 0.0001) from a recent EWAS of prescription opioid use [Citation10]. We also examined whether CpGs previously related to opioid dependence [Citation8] were related to opioid use in our data. Additionally, we interrogated CpGs annotated to the OPRM1 gene in our data because candidate gene studies reported hypermethylation related to opioid addiction and use [Citation7,Citation10].
Additional analyses
We considered potential residual confounding by substances known for their impact on genome-wide blood DNA methylation. Although we had adjusted for smoking status (never/past/current) and lifetime intensity (pack-years), to evaluate possible residual confounding by smoking, we examined whether CpGs identified for opioid use (FDR < 0.05) in our data overlapped with CpGs associated with current smoking in a previous large EWAS meta-analysis [Citation3]. For alcohol use, we were not able to harmonize and adjust for alcohol consumption due to minimal information on alcohol use in the ALHS, one of the larger studies. However, we investigated whether CpGs identified (FDR < 0.05) in our meta-analysis overlapped with CpGs related to heavy alcohol consumption in a previous EWAS meta-analysis [Citation4]. Because we included one African-ancestry study (GENOA) in our meta-analysis of all participants, we conducted a leave-one-out meta-analysis to verify that our findings (FDR < 0.05) were not driven by this single study of non-European ancestry.
Results
This meta-analysis included 10,842 participants from five datasets (). On average, participants were 58 years old. About 3% (282/10,842) reported recent use (up to 30 days) of opioid medications, and this proportion did not differ by sex (males: 83/3,108; females: 117/3,348). We visualized meta-analysis results in Miami plots () [Citation37] and quantile-quantile plots (Supplementary Figure 1) with λ ranging between 1.1 and 1.3, suggesting minimal inflation.
Table 1. Characteristics of participating studies.
Each plot has two panels: upper for association results with positive regression coefficients and lower for association results with negative regression coefficients, with -log10 (p) on the Y axis and 22 chromosomes on the X axis. Horizontal lines depict p cutoffs for statistical significance after multiple-testing correction: Bonferroni and Benjamini–Hochberg false discovery rate. CpGs having uncorrected p > 0.05 were not displayed. (A) Epigenome-wide association study (EWAS) meta-analysis results in all participants. (B) EWAS meta-analysis results in males only. (C) EWAS meta-analysis results in females only.
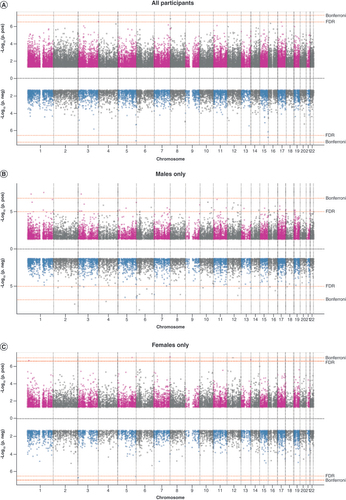
We identified six CpGs differentially methylated in relation to recent opioid medication use (FDR < 0.05; ). Of the six CpGs, four showed higher methylation in opioid users compared with nonusers. The CpGs annotated to six different genes: RUBCN (KIAA0226), CPLX2, TDRP, RNF38, TTC23 and GPR179. We did not observe significant heterogeneity across studies in the genome-wide significant findings (Supplementary Figure 2). Leave-one-out meta-analysis results confirmed that signals at the six CpGs were not driven by GENOA, a study of African ancestry (Supplementary Table 1).
Table 2. Six CpGs differentially methylated (false discovery rate < 0.05) in relation to opioid medication use (Nexposed = 282; Nunexposed = 10,560).
In sex-stratified meta-analyses, we found 74 CpGs related to opioid use (FDR <0.05): 68 CpGs in males (top 30 CpGs in ; all 68 CpGs in Supplementary Table 2) and six CpGs in females (); one additional CpG, cg07295964 (CPLX2), significant in females but not males, was also identified in the meta-analysis of all participants. The directions of associations were skewed toward positive in both sexes. Although none of the sex-specific findings showed genome-wide significant interactions between opioid use and sex, 51% showed at least nominal evidence of interaction (Pinteraction < 0.05; Supplementary Table 2 & & ), much more than expected by chance (Penrichment < 2.2 × 10-16).
Table 3. Top 30 differentially methylated CpGs (false discovery rate < 0.05) in relation to opioid medication use in males (Nexposed = 83; Nunexposed = 3025), sorted by chromosomal location.
Table 4. Seven CpGs differentially methylated (false discovery rate <0.05) in relation to opioid medication use in females (Nexposed = 117; Nunexposed = 3231), sorted by chromosomal location.
In regional analyses of all participants, 20 regions were differentially methylated in relation to opioid use (Šidák P < 0.05; Supplementary Table 3). One DMR (Chr15:99789621-99790022) included a significant genome-wide CpG (cg13401703). Sex-stratified analyses identified 24 DMRs for males and 15 for females (Supplementary Table 4). One additional region, Chr6:31650734-31651291 (LY6G5C), identified in females but not males showed genome-wide significance in analysis of all participants. Just four DMRs identified in sex-stratified analyses contained a significant genome-wide CpG (Supplementary Table 5). displays the top ten DMRs in all participants and by sex.
Table 5. Top ten differentially methylated regions related to opioid medication use (Šidák p < 0.05), sorted by chromosomal location.
Integrative epigenomic analyses using eFORGE identified enrichment for active transcriptional histone mark H3K36me3, which recruits DNA repair machinery upon damage to maintain genomic stability [Citation38], in several tissue types, including blood and brain (Supplementary Figure 3), supporting potential functional implications. In TF binding motif analyses using eFORGE-TF, we found an overlap with the motif V_ZID_01, corresponding to the transcription factor ZBTB6, in brain (p = 0.00002). An additional TF motif analysis using Analysis of Motif Enrichment [Citation39] identified the motif ZBT18_HUMAN.H11MO.0.C near all six significant CpGs but only 22 of 100 random nonsignificant CpGs (p = 0.01).
Pathway analysis identified enrichment for 19 gene sets, including the chemokine signaling pathway, a mechanism related to pain modulation. Most pathways were related to metabolism and inflammation (Supplementary Table 6).
By linking the significant CpGs (FDR < 0.05 in either the all-participants or sex-specific analyses) to transcriptome data, we identified CpGs related to the expression of nearby (±250 kb) genes: cis-eQTMs. In blood (FHS, n = 3684) [Citation34], three CpGs showed genome-wide significant associations with gene expression (FDR < 0.05). At nominal significance (p < 0.05), 29 CpGs showed associations (Supplementary Table 7). In brain (ROS/MAP, n = 515), four of 45 CpGs having one or more nearby transcripts were related to nearby gene expression at FDR < 0.05, and 11 (24%) showed nominally significant (p < 0.05) associations with expression (Supplementary Table 8). Six CpGs were related to the expression of nearby genes in both blood and brain.
In the GoDMC database [Citation36] of paired genetic and Illumina450K methylation data in 32,851 individuals, of the six CpGs significant in all participants, cg13401703 (TTC23) was associated with two nearby (both within 145 kb) genetic variants (meQTLs): chr15:99642518 and chr15:99932797.
Given the paucity of data on epigenome-wide blood DNA methylation and opioid use, we looked up findings from a recent EWAS [Citation8] (N = 220) of opioid dependence. Of the three CpGs meeting genome-wide significance in the previous study, two were marginally related to opioid use in our data (P = 0.07 for cg21381136; P = 0.04 for cg17426237). Of the remaining 499 CpGs reported at a threshold of p < 0.0001 and available in the data, 69 (14%) were related to opioid use at p < 0.05 (Penrichment = 4.09 × 10-14; Supplementary Table 9). Because several candidate gene studies reported higher methylation at the OPRM1 gene with opioid dependence and use [Citation6,Citation7,Citation9–11], we interrogated 61 CpGs annotated to the gene available in our data after CpG filtering. Of these, four were at least nominally (p < 0.05) associated with opioid use in our meta-analysis of all participants. Another three met p < 0.05 in sex-stratified meta-analyses. Finding these seven CpGs is slightly more than would be expected by chance (Penrichment = 0.032; Supplementary Table 10).
Although the meta-analysis results were adjusted for smoking, we assessed possible residual confounding by smoking by examining whether the opioid-related CpGs, in either overall or sex-stratified analyses, were associated with current smoking in a previous large Illumina450K EWAS meta-analysis [Citation3]. Of the 78 CpGs (450K) available for look-up, only 11 were associated with current smoking (Supplementary Table 11). We also addressed potential residual confounding by alcohol consumption, which we did not adjust for in the meta-analyses due to limited alcohol intake data in ALHS, by looking up the significant CpGs in a previous large 450K EWAS meta-analysis of heavy alcohol use [Citation4]. Of the 78 CpGs available for look-up, only nine CpGs were related to heavy alcohol consumption (Supplementary Table 11). Just four CpGs were associated with both smoking and alcohol consumption in these look-ups. None of the four genome-wide significant 450K CpGs in analyses of all participants were related to smoking or alcohol consumption.
Discussion
To our knowledge, this is the first large-scale, epigenome-wide association meta-analysis of blood DNA methylation and recent opioid medication use in general population studies. We identified several CpGs significantly differentially methylated in relation to opioid use in all participants. Additionally, we found CpGs showing differential methylation specific to men or women. Our findings were novel, meaning CpGs and genes identified in this study were not previously reported in studies of DNA methylation and opioids. Notably, our findings included CpGs related to the expression of nearby genes (cis-eQTM) in blood and brain. Integrative epigenomic results linked our methylation findings to enrichment of an active histone mark and an overlap with a transcription factor motif, suggesting functional implications. Pathway analysis results highlighted the chemokine signaling pathway, an emerging therapeutic option for pain management [Citation40,Citation41]. In addition to identifying novel methylation signals in relation to opioid use, our data provide the first validation of some previous opioid-related methylation findings in the general population. Extending knowledge from previous studies that focused on opioid dependence, this comprehensive investigation of blood DNA methylation and opioid medication use in the general population will help elucidate molecular mechanisms and population-level impacts of these commonly prescribed medications.
Notably, the opioid-related CpGs identified in the analyses of all participants implicate genes linked to brain development or brain disorders. Three of the six genes identified, KIAA0226, CPLX2 and GPR179, contain one or more CpGs associated with fetal brain age (n = 179 samples), suggesting a role in fetal brain development (Supplementary Table 12) [Citation42]. A genetic mutation in KIAA0226 (RUBCN) was related to the developmental disorder ataxia [Citation43,Citation44]. Genetic variants in the CPLX2 gene have been related to cognitive function in schizophrenia [Citation45]. Genetic variants in TDRP have been associated with attention deficit hyperactivity disorder [Citation46]. Another of the differentially methylated genes, RNF38, has been shown in animal studies to be regulated by the serotonin system in the brain [Citation47,Citation48], which modulates behaviors and psychological functions. Finally, the gene TTC23, which was highlighted in our differential methylation analysis and in our analysis linking methylation to nearby gene expression, was linked to schizophrenia in a recent EWAS [Citation49].
Integrative epigenomic analyses highlighted enrichment of an active transcriptional histone mark, H3K36me3, in both blood and brain. This suggests that the blood findings could help inform epigenomic mechanisms in brain. Additionally, the TF ZBTB18 of the motif ZBT18_HUMAN.H11MO.0.C, common across all six significant CpGs in the overall analysis, has been shown in mice to play a pivotal role in normal brain development through the time-sensitive repression of several proneurogenic genes [Citation50].
We also performed sex-specific analyses because some previous reports suggested differential effects of opioids by sex and some methylation studies analyzed only one sex. We found CpGs showing significant associations in males or females, suggesting some sex differences in the effects of opioids on methylation. We acknowledge that none of the identified CpGs showed genome-wide significant interactions. However, much larger sample sizes are needed to fully examine interactions, and sex-specific methylation findings may shed light on sex differences in opioid use and health effects.
Data on blood DNA methylation and opioid use are sparse. Several studies have examined DNA methylation in relation to opioid dependence. These studies differed from ours because they compared individuals with opioid dependence with individuals exposed to opioids but not dependent [Citation8], analyzed data with small-to-moderate sample sizes [Citation9] or were restricted to either women [Citation8] or men [Citation11]. Most used candidate gene approaches [Citation6,Citation7,Citation9–11], except for one EWAS [Citation8] of opioid dependence in 220 European-ancestry women. Two candidate gene studies (both n ≤ 123) examined methylation of the OPRM1 gene in relation to opioid use: long-term (for at least 1 year prior to sample selection) opium use using blood samples [Citation11] and short-term prescription opioid use using saliva samples [Citation10]. Compared with studies of individuals with opioid dependence in clinical settings, our study of opioid medication use in the general population and molecular impacts has value for deeper understanding of the population-level impacts of opioid medication use.
When studying the biological effects of opioid use, the primary tissue of interest is brain. However, data on DNA methylation in brain are understandably very limited. When developing biomarkers, blood, an easily accessible tissue, is useful. To help interpret the blood methylation at implicated CpGs, we evaluated correlations between methylation in blood and brain [Citation51]. Of four CpGs differentially methylated in relation to opioid use in all participants and available in the blood and brain methylation correlation tool [Citation51] (450K), methylation in blood at cg13401703 (TTC23) was highly correlated with methylation at four brain regions (Pearson correlation: 0.63 to 0.71; Supplementary Figure 4). The observed high correlation suggests that these findings in blood may indicate methylation alterations in the brain.
This study has some limitations. Although the authors attempted to harmonize the definition of recent opioid medication use, it differed by study, and the exact time course or dosage of ingestion was unknown. This is because this meta-analysis combined data from population-based studies in which inventories of recently used medications were available. In ALHS, we asked about medications in the past 7 days, the shortest period of any of the studies. The reported recent use in our participating studies is likely a mix of short- and long-term use. Longer use would be predicted to have greater impacts on DNA methylation, and the study may have missed these or underestimated them. However, in ALHS, we did not visit participants with abdominal or chest surgery in the past 3 months, which will have reduced short-term postoperative prescriptions. There were no data to investigate whether the reasons for taking opioids differ between sexes, which might contribute to sex-specific findings. The study participants were predominantly older individuals. Indications for time-limited prescription opioid use are likely to change over the life course. The participating studies are population-based studies of free-living people. Blood was collected during visits specifically conducted for the study – not during visits to the hospital. Population-based studies of older people will include people with various age-related conditions. However, we find that if participants are feeling acutely ill on a given day or so chronically disabled that participation is difficult, they will not agree to the study visit. It is standard in population-based studies not to exclude people based on chronic disease or age. However, it is standard to carefully adjust for age in all analyses. We were not able to replicate these meta-analysis findings in an independent population, as the analysis included as many population-based studies as we were able to identify with the available data. Instead, we provide evidence of validation for some previously reported methylation signals related to opioid dependence or use in our data.
This study has several strengths. To our knowledge, this is the largest study of genome-wide DNA methylation and opioid medication use. We carried out sex-specific analyses and included studies of African ancestry and European ancestry. We identified several DMRs. By linking our methylation findings to transcriptome in blood and brain, we found CpGs with potential functional impacts. Integrative epigenomic results link our data to regulatory elements, including histone marks and TF motifs, in blood and/or brain. Because this meta-analysis included individuals participating in population-based studies not enriched for opioid dependence, the results are particularly useful for the development of biomarkers of opioid medication use.
Publication bias is an issue for meta-analyses involving published studies. However, this is a de novo meta-analysis where none of the individual studies had previously published on this association. Therefore, publication bias is not an issue in this study.
In population-based studies, it is expected there will be a low proportion of opioid users and thus that most of the participants are in the unexposed group. The power of the study comes primarily from the number of exposed, but the imbalance with the expected large proportion of unexposed in these studies is not a source of bias.
To better understand health effects of an exposure, objective measures of exposure are useful. Some epidemiologic studies may have asked participants to self-report use of opioid medications but likely have limited information on duration or dosage. Where available, linkage to prescription registries can be used but lack information on what is actually taken. Furthermore, participants may be reluctant to acknowledge the use of opioids, leading to underreporting. Given that many studies have EWAS data but limited data on medication use, a well-validated biomarker of opioid use would increase the usefulness of existing studies to elucidate the full range of the health effects of this exposure. In clinical settings, objective biomarkers could improve the identification of individuals using these medications and help clinicians manage opioid prescriptions and alternative treatment options for chronic pain.
Conclusion
In summary, this large-scale epigenome-wide association study of blood DNA methylation and recent opioid medication use extends the literature by greatly increasing the sample size and including general-population studies with recent genome-wide DNA methylation arrays in two ancestries. This epigenome-wide meta-analysis identifies loci that have not previously been linked to opioid use. The findings could serve as a resource that can be leveraged for the future development of epigenomic biomarkers of opioid use and to extend our understanding of the effects of these drugs.
This is the first epigenome-wide association meta-analysis of opioid medication use and blood DNA methylation.
The study identified several CpGs differentially methylated in relation to opioid medication use in all participants.
There were many differential methylation signals specific to males or females.
Using integrative epigenomic tools, we found the differential methylation signals overlap with regulatory elements in blood and/or brain.
Methylation levels at significant CpGs were correlated with the expression of nearby genes in blood and/or brain, furthering functional relevance.
Author contributions
M Lee and SJ London conceived and designed the study. M Lee, R Joehanes, DL McCartney and M Kho completed study-specific analyses. M Lee, R Joehanes, A Hüls, S Sikdar and A Burkholder performed follow-up analyses. AB Wyss, C Liu, RM Walker, SLR Kardia, TS Wingo, J Ma, A Campbell, AP Wingo, T Huan, A Keshawarz, DA Bennett, JA Smith, KL Evans, D Levy and SJ London contributed to study design and/or supervised data analysis in each cohort. M Lee wrote the first draft of the manuscript. All authors contributed to interpretation of the results and/or critical revision of the manuscript. All authors approved the final version.
Ethical conduct of research
Each study was approved by its institutional review board. All study participants provided written informed consent.
Supplemental application 1
Download PDF (1.9 MB)Acknowledgments
We thank Frank Day of NIEHS and Jianping Jin of Westat, Inc. (Durham, NC, USA) for providing expert assistance.
Supplementary data
To view the supplementary data that accompany this paper please visit the journal website at: www.tandfonline.com/doi/suppl/10.2217/epi-2022-0353
Financial & competing interests disclosure
This work was supported in part by the Intramural Research Program of the National Institutes of Health, National Institute of Environmental Health Science (Z01-ES043012). Infrastructure for the CHARGE Consortium is supported in part by the National Heart, Lung, and Blood Institute grant HL105756. The Agricultural Lung Health Study is supported by the Intramural Research Program of the National Institutes of Health, National Institute of Environmental Health Sciences (Z01-ES102385, Z01-ES049030, Z01-ES043012 and for ABW, contract no. HHSN273201600003I) and the National Cancer Institute (Z01-CP010119). This work was supported in part by American Recovery and Reinvestment Act (ARRA) funds through NIEHS contract N01-ES-55546. The Framingham Heart Study is funded by National Institutes of Health contract N01-HC-25195 and HHSN268201500001I. The laboratory work for this investigation was funded by the Division of Intramural Research, National Heart, Lung, and Blood Institute, National Institutes of Health. The analytical component of this project was funded by the Division of Intramural Research, National Heart, Lung, and Blood Institute, and the Center for Information Technology, National Institutes of Health, Bethesda, MD. Disclaimer: The views expressed in this manuscript are those of the authors and do not necessarily represent the views of the National Heart, Lung, and Blood Institute; the National Institutes of Health; or the U.S. Department of Health and Human Services. Generation Scotland received core support from the Chief Scientist Office of the Scottish Government Health Directorates (CZD/16/6) and the Scottish Funding Council (HR03006) and is currently supported by the Wellcome Trust (216767/Z/19/Z). Genotyping of the GS:SFHS samples was carried out by the Genetics Core Laboratory at the Edinburgh Clinical Research Facility, University of Edinburgh, Scotland, and was funded by the Medical Research Council UK and the Wellcome Trust (Wellcome Trust Strategic Award “STratifying Resilience and Depression Longitudinally” [STRADL] reference 104036/Z/14/Z). The DNA methylation profiling and analysis were supported by Wellcome Investigator Award 220857/Z/20/Z and grant 104036/Z/14/Z (PI: AM McIntosh) and through funding from NARSAD (ref: 27404; awardee: DM Howard) and the Royal College of Physicians of Edinburgh (Sim Fellowship; awardee: HC Whalley). Support for the Genetic Epidemiology Network of Arteriopathy was provided by the National Heart, Lung and Blood Institute (U01 HL054457, RC1 HL100185, R01 HL087660, R01 HL119443 and R01 HL133221). ROS/MAP is supported by P30AG10161, P30AG72975, R01AG15819, R01AG17917, U01AG46152, U01AG61356. ROS/MAP resources can be requested at https://www.radc.rush.edu. A Hüls is supported by the HERCULES Center (NIEHS P30ES019776). The authors declare that they have no relevant conflicts of interest.
No funded writing assistance has been used in the creation of this manuscript.
Data sharing statement
Complete meta-analysis results can be found at Zenodo 10.5281/zenodo.7545108.
Additional information
Funding
References
- Kieffer BL , EvansCJ. Opioid receptors: from binding sites to visible molecules in vivo. Neuropharmacology56(Suppl. 1), S205–S212 (2009).
- Mattson CL , TanzLJ , QuinnK , KariisaM , PatelP , DavisNL. Trends and geographic patterns in drug and synthetic opioid overdose deaths – United States, 2013–2019. MMWR Morb. Mortal. Wkly Rep.70(6), 202–207 (2021).
- Joehanes R , JustAC , MarioniREet al. Epigenetic signatures of cigarette smoking. Circ. Cardiovasc. Genet.9(5), 436–447 (2016).
- Liu C , MarioniRE , HedmanAKet al. A DNA methylation biomarker of alcohol consumption. Mol. Psychiatry23(2), 422–433 (2018).
- Andersen AM , RyanPT , GibbonsFX , SimonsRL , LongJD , PhilibertRA. A droplet digital PCR assay for smoking predicts all-cause mortality. J. Insur. Med.47(4), 220–229 (2018).
- Chorbov VM , TodorovAA , LynskeyMT , CiceroTJ. Elevated levels of DNA methylation at the OPRM1 promoter in blood and sperm from male opioid addicts. J. Opioid Manage.7(4), 258 (2011).
- Doehring A , OertelBG , SittlR , LotschJ. Chronic opioid use is associated with increased DNA methylation correlating with increased clinical pain. Pain154(1), 15–23 (2013).
- Montalvo-Ortiz JL , ChengZ , KranzlerHR , ZhangH , GelernterJ. Genomewide study of epigenetic biomarkers of opioid dependence in European-American Women. Sci. Rep.9(1), 4660 (2019).
- Xu J , WangT , SuZet al. Opioid exposure is associated with aberrant DNA methylation of OPRM1 promoter region in a Chinese Han population. Biochem. Genet.56(5), 451–458 (2018).
- Sandoval-Sierra JV , SalgadoGarcia FI , BrooksJH , DerefinkoKJ , MozhuiK. Effect of short-term prescription opioids on DNA methylation of the OPRM1 promoter. Clin. Epigenetics12(1), 76 (2020).
- Ebrahimi G , AsadikaramG , AkbariHet al. Elevated levels of DNA methylation at the OPRM1 promoter region in men with opioid use disorder. Am. J. Drug Alcohol Abuse44(2), 193–199 (2018).
- Craft RM . Sex differences in analgesic, reinforcing, discriminative, and motoric effects of opioids. Exp. Clin. Psychopharmacol.16(5), 376–385 (2008).
- Mazure CM , FiellinDA. Women and opioids: something different is happening here. Lancet392(10141), 9–11 (2018).
- Weiner SG , ElIbrahimi S , HendricksMAet al. Factors associated with opioid overdose after an initial opioid prescription. JAMA Netw. Open5(1), e2145691 (2022).
- Kaplovitch E , GomesT , CamachoX , DhallaIA , MamdaniMM , JuurlinkDN. Sex differences in dose escalation and overdose death during chronic opioid therapy: a population-based cohort study. PLOS ONE10(8), e0134550 (2015).
- Kelley K , KelleyT , KaufmanD , MitchellA. The Slone Drug Dictionary: a research driven pharmacoepidemiology tool. Pharmacoepidemiol. Drug Saf.12(Suppl. 1), S168–S169 (2003).
- Zhou W , LairdPW , ShenH. Comprehensive characterization, annotation and innovative use of Infinium DNA methylation BeadChip probes. Nucleic Acids Res.45(4), e22 (2017).
- Davidov O , JelsemaCM , PeddadaS. Testing for inequality constraints in singular models by trimming or winsorizing the variance matrix. J. Am. Stat. Assoc.113(522), 906–918 (2018).
- Houseman EA , AccomandoWP , KoestlerDCet al. DNA methylation arrays as surrogate measures of cell mixture distribution. BMC Bioinformatics13, 86 (2012).
- Rice K , HigginsJPT , LumleyT. A re-evaluation of fixed effect(s) meta-analysis. J. R. Stat. Soc. Ser. A Stat. Soc.181(1), 205–227 (2018).
- Willer CJ , LiY , AbecasisGR. METAL: fast and efficient meta-analysis of genomewide association scans. Bioinformatics26(17), 2190–2191 (2010).
- Benjamini Y , HochbergY. Controlling the false discovery rate – a practical and powerful approach to multiple testing. J. R. Stat. Soc. B57(1), 289–300 (1995).
- Pedersen BS , SchwartzDA , YangIV , KechrisKJ. Comb-p: software for combining, analyzing, grouping and correcting spatially correlated p-values. Bioinformatics28(22), 2986–2988 (2012).
- Xu Z , XieC , TaylorJA , NiuL. ipDMR: identification of differentially methylated regions with interval p-values. Bioinformatics37(5), 711–713 (2021).
- Sidak Z . Rectangular confidence regions for the means of multivariate normal distributions. J. Am. Stat. Assoc.62(318), 8 (1967).
- Breeze CE , ReynoldsAP , Van DongenJet al. eFORGE v2.0: updated analysis of cell type-specific signal in epigenomic data. Bioinformatics35(22), 4767–4769 (2019).
- Breeze CE . Cell type-specific signal analysis in EWAS. bioRxivdoi:10.1101/2021.05.21.4452092021.2005.2021.445209 (2021) ( Epub ahead of print).
- Roadmap Epigenomics Consortium , KundajeA , MeulemanWet al. Integrative analysis of 111 reference human epigenomes. Nature518(7539), 317–330 (2015).
- Bailey TL , JohnsonJ , GrantCE , NobleWS. The MEME Suite. Nucleic Acids Res.43(W1), W39–W49 (2015).
- Kolde R , LaurS , AdlerP , ViloJ. Robust rank aggregation for gene list integration and meta-analysis. Bioinformatics28(4), 573–580 (2012).
- Subramanian A , TamayoP , MoothaVKet al. Gene set enrichment analysis: a knowledge-based approach for interpreting genome-wide expression profiles. Proc. Natl Acad. Sci. USA102(43), 15545–15550 (2005).
- Ren X , KuanPF. methylGSA: a bioconductor package and shiny app for DNA methylation data length bias adjustment in gene set testing. Bioinformatics35(11), 1958–1959 (2019).
- Carlson M . org.Hs.eg.db: genome wide annotation for human. R package version 3.8.2. (2019). https://bioconductor.org/packages/release/data/annotation/html/org.Hs.eg.db.html
- Huan T , JoehanesR , SongCet al. Genome-wide identification of DNA methylation QTLs in whole blood highlights pathways for cardiovascular disease. Nat. Commun.10(1), 4267 (2019).
- Huls A , RobinsC , ConneelyKNet al. Brain DNA methylation patterns in CLDN5 associated with cognitive decline. Biol. Psychiatry91(4), 389–398 (2022).
- Min JL , HemaniG , HannonEet al. Genomic and phenotypic insights from an atlas of genetic effects on DNA methylation. Nat. Genet.53(9), 1311–1321 (2021).
- Winkler TW , KutalikZ , GorskiM , LottazC , KronenbergF , HeidIM. EasyStrata: evaluation and visualization of stratified genome-wide association meta-analysis data. Bioinformatics31(2), 259–261 (2015).
- Sun Z , ZhangY , JiaJet al. H3K36me3, message from chromatin to DNA damage repair. Cell Biosc.10, 9 (2020).
- McLeay RC , BaileyTL. Motif enrichment analysis: a unified framework and an evaluation on ChIP data. BMC Bioinformatics11, 165 (2010).
- Melik Parsadaniantz S , RivatC , RosteneW , Reaux-LeGoazigo A. Opioid and chemokine receptor crosstalk: a promising target for pain therapy?Nat. Rev. Neurosci.16(2), 69–78 (2015).
- Montague K , MalcangioM. The therapeutic potential of targeting chemokine signalling in the treatment of chronic pain. J. Neurochem.141(4), 520–531 (2017).
- Spiers H , HannonE , SchalkwykLCet al. Methylomic trajectories across human fetal brain development. Genome Res.25(3), 338–352 (2015).
- Assoum M , SalihMA , DrouotNet al. Rundataxin, a novel protein with RUN and diacylglycerol binding domains, is mutant in a new recessive ataxia. Brain133(Pt. 8), 2439–2447 (2010).
- Seidahmed MZ , HamadMH , AlbakheetAet al. Ancient founder mutation in RUBCN: a second unrelated family confirms Salih ataxia (SCAR15). BMC Neurol.20(1), 207 (2020).
- Begemann M , GrubeS , PapiolSet al. Modification of cognitive performance in schizophrenia by complexin 2 gene polymorphisms. Arch. Gen. Psychiatry67(9), 879–888 (2010).
- Harich B , KleinM , OckeloenCWet al. From man to fly – convergent evidence links FBXO25 to ADHD and comorbid psychiatric phenotypes. J. Child Psychol. Psychiatry61(5), 545–555 (2020).
- Cham KL , SogaT , ParharIS. Expression of RING finger protein 38 in serotonergic neurons in the brain of Nile tilapia, Oreochromis niloticus. Front. Neuroanat.12, 109 (2018).
- Moriya S , KhelNB , ParharIS. Cloning and serotonergic regulation of RING finger protein38 (rnf38) in the brain of medaka (Oryzias latipes). Neuroscience294, 109–115 (2015).
- Li M , LiY , QinHet al. Genome-wide DNA methylation analysis of peripheral blood cells derived from patients with first-episode schizophrenia in the Chinese Han population. Mol. Psychiatry26(8), 4475–4485 (2021).
- Xiang C , BaubetV , PalSet al. RP58/ZNF238 directly modulates proneurogenic gene levels and is required for neuronal differentiation and brain expansion. Cell Death Differ.19(4), 692–702 (2012).
- Hannon E , LunnonK , SchalkwykL , MillJ. Interindividual methylomic variation across blood, cortex, and cerebellum: implications for epigenetic studies of neurological and neuropsychiatric phenotypes. Epigenetics10(11), 1024–1032 (2015).