Abstract
Aim: Whole-genome methylation sequencing carries both DNA methylation and structural variant information (single nucleotide variant [SNV]; copy number variant [CNV]); however, limited data is available on the reliability of obtaining this information simultaneously from low-input DNA using various library preparation and sequencing protocols. Methods: A HapMap NA12878 sample was sequenced with three protocols (EM-sequencing, QIA-sequencing and Swift-sequencing) and their performance was compared on CpG methylation measurement and SNV and CNV detection. Results: At low DNA input (10–25 ng), EM-sequencing was superior in almost all metrics except CNV detection where all protocols were similar. EM-sequencing captured the highest number of CpGs and true SNVs. Conclusion: EM-sequencing is suitable to detect methylation, SNVs and CNVs from single sequencing with low-input DNA.
DNA methylation plays an important role in human physiology and disease; this phenomenon is one of the most active research areas in epigenomics, particularly for understanding regulatory mechanisms of gene expression and in the development of biomarkers of disease. Measurement of DNA methylation has historically required bisulfite treatment, which converts unmethylated cytosine to uracil and subsequently to thymine during PCR amplification. Whole-genome bisulfite sequencing is the most comprehensive and commonly used approach for such measurement in the genome-scale; however, this chemically harsh treatment often leads to analyte loss, mitigated by high DNA input. A new method, enzymatic methyl-sequencing (EM-seq), is reported to overcome this limitation (input as low as 100 picograms) [Citation1] and is becoming a preferred alternative [Citation2–4]. This method uses TET2 and T4-BGT to protect 5 mC and 5 hmC while unmodified cytosines are then converted to uracils after APOBEC3A treatment. In addition to methylation, whole-genome methylation sequencing (WGMS) data can also be used for single nucleotide variation (SNV) or copy number variation (CNV) detection. Theoretically, WGMS could allow investigators to obtain multiple types of genomic information from a single sequencing run at high sample efficiency and lower cost. However, limited data are available for these additional benefits from different library protocols and analytical tools, particularly at low DNA input.
To address these questions, libraries with low input amounts (10–25 ng) of HapMap NA12878 DNA were prepared using four library preparations: QIAseq methyl-sequencing (QIAseq; Qiagen, MD, USA), Swift Accel-NGS-Methyl-Seq (Swift-seq; Integrated DNA Technologies, IA, USA), NEBNext Enzymatic Methyl-Seq (EM-seq; New England Biolabs Inc., MA, USA) and nontreated NEBNext Ultra II whole-genome sequencing (WGS; New England Biolabs Inc.). Various quality control matrices, DNA methylation estimates and the accuracy of SNV and CNV detection were compared.
Materials & methods
Sample, library preparation & sequencing
HapMap NA12878 (obtained from the NIGMS Human Genetic Cell Repository at the Coriell Institute for Medical Research) was used for all sequencing modalities, including bisulfite treatment-based QIAseq and Swift-seq, enzymatically treated EM-seq and nontreated WGS (). WGS was used mainly as a more comparable reference for SNP and CNV detection (in addition to public SNV and CNV call standards), as it was generated from the same sequencing facility at the same time as the methylation sequencing data. All sequencing had DNA input of 25 ng except that an additional replicate was sequenced at an input of 10 ng for EM-seq to test the impact of further reduced DNA amount. Another replicate for EM-seq at 25 ng was also generated at a higher sequencing depth.
Table 1. Mapping and CpG capture quality control matrices for different sequencing libraries.
Swift-seq was prepared using a Swift Biosciences (MI, USA) Accel-NGS Methyl-Seq DNA Library Kit. DNA (25 ng) was sheared to a size of 300 bp using a Covaris LE-220 (Covaris, LLC., MA, USA). After shearing, DNA was bisulfite-converted using the EZ DNA Methylation Gold Kit (Zymo Research, CA, USA) according to the manufacturer’s instructions. Bisulfite-converted DNA was prepared into paired-end libraries according to the manufacturer’s guidelines. For QIAseq, DNA was bisulfite-converted using an EpiTect Fast Bisulfite Conversion Kit (Qiagen) following the manufacturer’s guidelines. Converted DNA was then prepared into paired-end libraries using a QIAseq Methyl DNA Library Kit according to the manufacturer’s directions. EM-seq was prepared following the NEBNext Enzymatic Methyl-seq Kit manufacturer’s guidelines (New England BioLabs Inc.). An input amount of 10 or 25 ng of DNA for the whole genome was sheared to a size of 300 bp using a Covaris LE-220 (Covaris). Sheared DNA was prepared using NEBNext Ultra II library prep following the manufacturer’s instructions. The concentrations and sizes of all prepared libraries were determined using a Qubit fluorometry (Invitrogen, CA, USA), Fragment Analyzer or Agilent 2100 Bioanalyzer (Agilent, CA, USA). Prepared libraries were sequenced at an average coverage of ∼30x following Illumina’s standard protocol using a Illumina NovaSeq™ 6000 and S4 flow cell (Illumina, CA, USA). The flow cells were sequenced as 150 × 2 paired-end reads using NovaSeq S4 sequencing kits and NovaSeq Control Software v1.8.0 (Illumina). Base calling was performed using Illumina’s RTA version 3.4.4 (Illumina).
Sequence data preprocessing & analysis
Both the internal streamlined analysis and annotation pipeline for reduced representation bisulfite sequencing (SAAP-BS) [Citation5] and popular Bismark [Citation6] pipelines were used to process the raw methylation sequencing data to assess the variability from different pipelines. Briefly, for SAAP-BS, raw sequences were checked for quality by FastQC, were trimmed by TrimGalore to remove adaptor sequences and reads with less than 15 bp after trimming were discarded. Trimmed FastQs were then aligned against the reference genome hg38 using BSMAP [Citation7]. Samtools [Citation8] was used to get Mpileup and custom Perl scripts to determine CpG methylation and bisulfite conversion ratios after removing duplicate reads. Similar preprocessing was applied for the Bismark pipeline where cleaned reads were mapped to hg38 using Bowtie [Citation9], duplicate reads were removed afterward and Bismark scripts were used to extract DNA methylation data. In both pipelines, only uniquely mapped reads were retained and duplicate reads were those mapped to the exact same start and end positions in the same orientation (only one read pair was kept in this case). For SAAP-BS, the samtools rmdup command was used and for Bismark, deduplicate_bismark from the Bismark pipeline was used.
The WGS was processed by Genome_GPS v5.0.3 (formerly named TREAT [Citation10]). FastQ files were aligned to the hg38 reference genome using bwa-mem (VN:V0.7.10) [Citation11] using default options. Realignment was performed using GATK [Citation12] (VN:3.4–46). Variant calling was performed using the GATK (VN: 3.4–46) haplotype caller, and variants were filtered using a variant recalibration variant quality score recalibrator for both single nucleotide variants and insertions and deletions. Only SNVs were used for further comparison in this study.
SNP & CNV detection
Three methylation sequencing-specific callers, BisSNP (v0.82.2) [Citation13], BSSNPer (v1.1) [Citation14] and Biscuit (https://github.com/huishenlab/biscuit) were used to make SNV calls from methylation sequencing data with their default settings.
CNVs were called using CNVpytor (v1.2.1) [Citation15] at bin sizes of 1K and 5K. At bin size 1K, only read depth information was used so, it essentially was the same algorithm as its previous version CNVnator [Citation16]. To take an advantage of CNVpytor, SNP data called from each sample itself was incorporated as well as Genome in a Bottle (GIAB) SNP set (see later) at 5k bin size (the smallest recommended bin size). Default CNV calling parameters were used. Postfiltering was performed for the raw calling results with the following criteria: regions containing more than half of nonuniquely mapped reads, regions with e-val1 (adjusted p-value) >0.001, regions containing more than 50% undefined Ns in the reference genome and regions close to gaps in the reference genome (<100K).
Reference SNV & CNV sets for performance comparisons
High-confidence genomic variants (SNVs, small indel, version 3.3.2) of NA12878 were downloaded from GIAB project (https://ftp-trace.ncbi.nlm.nih.gov/ReferenceSamples/giab/release/NA12878_HG001/latest/GRCh38). Only SNVs were extracted as a truth set to compare variant data from methylation sequencing as these callers do not call indels from methylation sequencing data. After filtering, the variant set had 2,863,508 SNVs.
For CNVs, four CNV sets were used as ‘truth’ for comparisons, including the gold standard set from the 1000 Genomes Project, which was derived from WGS of this same sample using a combination of callers [Citation17]. This set has 2271 CNV calls (referred to as ‘gold’); CNV calls from that same sample (∼60X from Illumina HiSeq) using CNVnator alone by Haraksing et al. (4,295 CNVs) [Citation18] (“1k.cnvnator”); CNV set for NA12878, which was sequenced by Illumina and made by Lumpy with paired-end and split-read algorithm [Citation19] (‘lumpy’). This set had 3131 structural variants (deletion, inversion, tandemup or unknown categories) but only ’deletion’ and ’tandemup’ were kept for comparison; and CNVs were called from the internal WGS data using CNVpytor (‘i.cnvpytor’). This CNV set was expected to be the most comparable with methylation sequencing data as the data were generated in the same environment and with the same caller using the same parameters and filtering criteria. All public CNV sets were in hg19 coordinates but were lifted over to hg38.
Performance comparisons with benchmarking SNVs & CNVs
The performance matrices for SNPs were calculated using Haplotype Comparison Tools (Happy, https://github.com/Illumina/hap.py#happy). True-positive or recall (TP) is a variant or genotype that matches between truth and query sets. False-positive (FP) is a variant that has mismatching with or not present in a truth set. False-negative (FN) is variant present in the truth but missing in the query set. Precision is obtained using the formula TP/(TP+FP) and F1 score is from 2*Precision*Recall/(Precision+Recall).
Due to variable sequence depths of different libraries and more comprehensive GIAB truth set derived from very high depth of sequencing, performance measurements were also calculated at different depths of coverage (0X, 1X, 5X and 10X) in the query sample at the positions of the truth set (i.e., 0X for all positions in the truth set and 1X, 5X and 10X for the genomic positions in the truth set where the query sample had the minimum of that coverage). Similar benchmarking measurements were obtained for CNVs using Truvari (v3.4.0) [Citation20] against the reference sets. Sequence comparison was turned off and if query and reference region had at least one base overlap it was considered a match.
Results
Read quality, alignment efficiency & CpG coverage
Sequencing reads for each library ranged from 600 million to 1.2 billion (pair ends). The mapping efficiency for EM-seq ranged from 82.2 to 89.2% for three replicates but was lower for QIAseq and Swift-seq at 67.7 and 73.6%, respectively (SAAP-BS pipeline). While over 50 million CpGs were detected at 5X for EM-seq, about 30 million and 48 million were detected for QIAseq and Swift-seq. The estimated conversion rates were all >0.99 for EM-seq and QIAseq while the rate was lower for Swift-seq at 0.98 (). As a reference, the regular WGS had a mapping rate of 0.99. The Bismark pipeline generally had lower mapping rates, higher duplicate rates and lower numbers of detected CpGs compared with SAAP-BS. The estimated conversion rates were also all >0.99 except for Swift-seq, which was estimated at 0.95.
CpG coverage & methylation estimate among different libraries
Among three library protocols, EM-seq captured the highest number of CpGs (A). In contrast, QIAseq captured the smallest number of CpGs after duplication removal (the deduplication rate had a very large impact as there was 29.7% CpG reduction compared with nonduplicate removed data). When the data was processed by Bismark, only 1.1 million CpGs (at 5X or above) remained after duplicate removal, and about a third of CpGs from the SAAP-BS pipeline, an indication of pipeline differences in how duplicate reads are defined and removed. The performance of Swift-seq sits between EM-seq and QIAseq but was closer to EM-seq regardless of input DNA at 10 or 25 ng. It was the only library where the Bismark pipeline detected more CpGs than the SAAP-BS pipeline.
(A) Number of CpGs captured at 5X from SAAP-BS and Bismark pipelines. Except for Swift-seq, all others have a higher number of CpGs at 5X from SAAP-BS pipeline. This is quite dramatic for QIAseq and is mostly related to mapping rate and duplication read identification and removal. (B) CpG methylation correlations among libraries by SAAP-BS. The highest correlation was between Swift-seq and EM-seq 25 ng across protocols while the lowest was between QIAseq and EM-seq 10 ng, which have the highest read duplication rates. (C). Pairwise correlations among libraries and processing pipelines. (D) Correlation heatmap among libraries and processing pipelines. Pipelines make little difference.
Bis: Bismark; Bsm: SAAP-BS with BSMAP; EM: NEBNext Enzymatic Methyl-Seq; QIAseq: QIAseq methyl-sequencing; SwiftSeq: Swift Accel-NGS-Methyl-Seq; SAAP-BS: Streamlined Analysis and Annotation Pipeline for Bisulfite Sequencing.
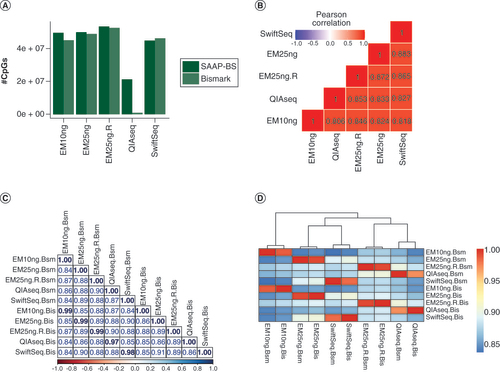
For commonly detected CpGs (at 5X coverage) among different libraries, their methylation estimate consistency was compared. For data within pipeline (SAAP-BS or Bismark), the highest correlation was between EM-seq 25 ng and Swift-seq 25 ng (correlation coefficient: 0.88) and then the two replicates of EM-seq 25 ng (0.87). EM-seq 10 ng had the lowest correlation with other libraries ranging from 0.81 to 0.85, suggesting too low input could compromise data quality slightly and library protocols played a lesser role in DNA methylation estimates (B; SAAP-BS data shown). Processing pipelines of Bismark and SAAP-BS made little difference in DNA measurements as the data from the same library but processed by the two pipelines were clustered together (correlation >0.97; C & D). It was also noted that libraries from the same protocol did not necessarily have the highest correlation. For example, QIAseq clustered with one of EM-seq libraries and Swift-seq clustered with another (D). For the common CpGs covered at 30X or above, all the correlations increased to ≥0.93, confirming that methylation estimate accuracy increases with depth of coverage (Supplementary Figure 1).
SNV detection accuracy varies among libraries & variant callers
Generally, there was much better performance for EM-seq with input either at 10 or 25 ng compared with other libraries (A) in SNV detection. QIAseq and Swift-seq performed poorly in terms of recall using BisSNP and BSSNPer (<25%) while Biscuit provided better results for these two (25–80%). Bismark-processed data had lower recalls than SAAP-BS-processed data for all callers, although the differences were mostly small. Biscuit had the best recall in all libraries, particularly for QIAseq and Swift-seq, where BisSNP and BSSNPer performed poorly. BisSNP had the highest precision in most cases. Overall, Biscuit provided a better balance between recall and precision and could work superiorly in different library preparation protocols.
(A) GIAB SNP set as truth set. (B) Internal whole-genome sequencing variant set as truth set. In both cases, EM-seq leads to the best recall, precision and F1 score. QIAseq performs poorly for this purpose while it can be largely rescued using SAAP-BS pipeline and Biscuit caller. For genomic positions with 5 or 10X coverage, all callers make very accurate calls (high precision) although some variants may be missed.
EM: NEBNext Enzymatic Methyl-Seq; GIAB: Genome in a bottle; lib.aln.caller: Library preparation, aligner and caller combination; QIAseq: QIAseq methyl-sequencing; SwiftSeq: Swift Accel-NGS-Methyl-Seq; SAAP-BS: Streamlined Analysis and Annotation Pipeline for Bisulfite Sequencing; 1,5,10X: Coverage at 1, 5, 10 sequence reads.
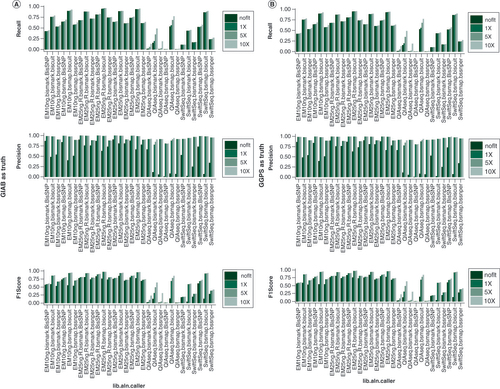
The SNP set from the internal WGS sample was also used to better gauge the variant information the investigator could get from a WGMS run alone without additional WGS. The performances was quite similar to GIAB data (B). With EM-seq, SAAP-BS and Biscuit, over 90% of variants called from the WGS could be correctly called from the methylation sequencing, although the rate was a bit lower for Bismark-processed data (about 80%). Bisulfite-based sequencing had much lower SNP detection rates (<25%) using BisSNP and BSSNPer while it could be significantly improved to 75 to 80% with Biscuit. For genomic locations with 5X or above coverage, all library, processing pipeline and caller combinations had high precision (generally >95%).
CNV detection performance from WGMS is similar to WGS
At 1K bin size (read depth information only), all libraries (including WGS) had sensitivity of less than 20% when truth sets of gold, 1k.cnvnator and Lumpy were used; however, when the internally generated WGS CNV set with the same caller, parameters and filtering criteria was used, the sensitivity increased to about 70%, although the precision and F1 score remained low (A). At a bin size of 5K (B), fewer CNVs were called in all libraries, which led to even lower recalls with all three public reference sets but increased recalls with the internal WGS reference set (75% or above).
(A) Bin size of 1K without SNV usage. For comparisons with public CNV truth sets, performances of methylation sequencing libraries were similar to WGS although they all had low recall and F1 scores. Methylation sequencing gets about 75% of CNVs detected from WGS. (B) Bin size of 5K without SNVs. (C) Bin size of 5K with GIAB SNVs. (D) Bin size of 5K with SNPs called from each library itself. Improved performance can be seen with incorporating SNVs using CNVpytor, particularly for SNV set for GIAB, which has more complete and higher quality SNPs (versus SNVs from each library, which is more variable depending on libraries). Gold: Gold reference CNV set from 1000 genome project. 1K.cnvnator: CNV calls from 1000 genome project sample using CNVnator alone by Haraksing et al. [Citation17]. Lumpy: CNV calls using Lumpy along with orthogonal validations. i.cnvpytor: CNV calls from an internal WGS sample using CNVpytor, the new version of CNVnator with python implementation and extended functionality such as incorporation of SNVs to increase CNV call accuracy.
EM: NEBNext Enzymatic Methyl-Seq; QIAseq: QIAseq methyl-sequencing; SwiftSeq: Swift Accel-NGS-Methyl-Seq.
![Figure 3. CNV call accuracies (recall, precision and F1 score) with four reference sets from different methylation libraries at bin sizes of 1k and 5k. (A) Bin size of 1K without SNV usage. For comparisons with public CNV truth sets, performances of methylation sequencing libraries were similar to WGS although they all had low recall and F1 scores. Methylation sequencing gets about 75% of CNVs detected from WGS. (B) Bin size of 5K without SNVs. (C) Bin size of 5K with GIAB SNVs. (D) Bin size of 5K with SNPs called from each library itself. Improved performance can be seen with incorporating SNVs using CNVpytor, particularly for SNV set for GIAB, which has more complete and higher quality SNPs (versus SNVs from each library, which is more variable depending on libraries). Gold: Gold reference CNV set from 1000 genome project. 1K.cnvnator: CNV calls from 1000 genome project sample using CNVnator alone by Haraksing et al. [Citation17]. Lumpy: CNV calls using Lumpy along with orthogonal validations. i.cnvpytor: CNV calls from an internal WGS sample using CNVpytor, the new version of CNVnator with python implementation and extended functionality such as incorporation of SNVs to increase CNV call accuracy.EM: NEBNext Enzymatic Methyl-Seq; QIAseq: QIAseq methyl-sequencing; SwiftSeq: Swift Accel-NGS-Methyl-Seq.](/cms/asset/1f19e5e4-79d8-40f3-968c-df9946c440f0/iepi_a_12324449_f0003.jpg)
Among three public CNV reference truth sets, the used CNV call sets had the highest recall, precision and F1 score with 1k.cnvnator but the worst with the Lumpy set. This was not surprising considering Lumpy uses a very different algorithm (using split and pair-end read information) and often calls many short CNVs. Between Lumpy and gold CNV sets, about half of Lumpy CNVs (54.7%) overlapped with gold CNVs while only 23.3% of its CNVs were part of the 1k.snvnator set. Regardless, compared with WGS, not many differences were seen for the CNV calls from methylation sequencing protocols and the poor performance with the public truth sets was mostly related to the known challenges in CNV detection from different algorithms, parameter settings and filtering criteria.
Taking advantage of the SNV incorporation feature in CNVpytor, it was assessed the utilization of SNV would improve the CNV call performance. Adding GIAB SNPs to CNV detection led to an improvement in performance to all reference sets, particularly for the internal WGS set (C). With the possibility of SNV calling from methylation sequencing data itself, it was also assessed whether using SNVs called from each library would improve CNV detection. The results appeared mixed. While it indeed increased recalls compared with all three public reference sets, there was reduced precision and F1 score with all but the Lumpy reference set for EM-Seq samples (D).
Discussion
Accurate measurement of DNA methylation modification is critical to multiple scientific and clinical disciplines. While bisulfite-based sequencing or microarray have been used for years, new methods and technologies are needed to work with degraded or low-input DNA, which is commonly the case when measuring methylation in clinical samples such as blood plasma or formalin-fixed, paraffin-embedded samples. Additionally, WGMS is essentially the same as the WGS in terms of read coverage in the genome so that CNVs can be detected. While cytosine modification in the methylation sequencing data complexes SNV calling, special tools have been developed to handle this challenge. Significant efficiency could be gained if investigators could measure methylation, CNVs and SNVs from a single sequencing step. With the wider availability of EM-seq, the literature now includes emerging data quality and DNA methylation measurement accuracy comparisons between different DNA library preparation methods [Citation2–4]. However, data are lacking for quality and accuracy in calling SNVs and CNVs.
Using the common NA12778 sample, we compared EM-seq, QIAseq and Swift-seq at low input of 25 ng or lower for methylation, SNVs and CNVs. The data consistently showed that EM-seq is the best to achieve all three purposes at once. EM-seq has the highest mapping efficiency, lowest rate of duplication reads and highest number of captured CpGs. EM-seq also leads to the highest SNV calling accuracy and up to 96% SNVs can be reliably called compared with GIAB or WGS. For CNVs, we did not observe significant differences among different library preparations; all were comparable to WGS.
The data also show clear differences among different aligner and variant caller combinations for SNVs. SAAP-BS (with BSMAP) along with Biscuit appears to obtain the highest recall without compromising precision at genomic locations with sufficient coverage. BisSNP or BSSNPer generally does not work well for traditional bisulfite sequencing and Biscuit would be preferred in this case.
Performance comparisons for CNV calls were quite challenging. First, there is no true gold standard for CNVs in NA12878 samples. For the three public standards we downloaded, the ’gold’ is the call set using multiple callers while ’1k.CNVnator’ and ’Lumpy’ are by a single caller but from very different algorithms. From called CNV number perspective, ’gold’ has 2271, 1k.CNVnator has 4295 and Lumpy has 3131 CNVs. However, the common CNVs among the three are only 305 and about 20% 1k.CNVnator CNVs overlap with gold CNVs and 54% of Lumpy CNVs overlap with gold CNVs. Second, the public 1K.CNAnator set has 4295 CNVs, which is substantially higher than what we obtained after filtering. The associated paper did not provide information about the settings and filtering. We expect it was the call set without filtering. The dramatic number difference would significantly affect recall or performance. Third, none of the public truth sets uses SNP information calling CNVs, so the only fair comparison is our internal WGS set where similar calling settings, filtering and incorporation of SNP were applied. With this in mind, we did not observe much difference among different sequencing libraries and suspect that library preparation methods may not have much impact on calling CNVs.
Although the data presented here were from a single sample where ’gold’ standards of SNVs and CNVs were readily available to benchmark, we also sequenced other samples, including another HapMap sample (NA12891) and formalin-fixed paraffin-embedded brain and lung samples by the three protocols and cell-free DNA from plasma by EM-seq only. These results also showed EM-seq had a consistently higher mapping rate and conversion rate and captured more CpG sites at 5X while the duplicate rate was lower compared with the Swift-seq and QIAseq. As observed in the NA12878 presented here, QIAseq generally had a higher duplication rate, yet the conversion was more complete than Swift-seq (Supplementary Figure 2).
The methylation data quality matrixes were in line with several literature reports (although none of them evaluated SNVs or CNVs). Using fresh frozen tissue with DNA input ranging from 10 to 300 ng, Morrison and colleagues [Citation3] compared four library protocols or kits (EM-seq, Swift, postbisulfite adaptor tagging [PBAT] and Roche Kapa) and found that EM-seq had consistently higher mapping rate, library complexity (lower duplication), genome coverage uniformity and methylation correlation coefficients within replicates and with the Swift protocol. With DNA input from 1 to 10 ng extracted from cerebrospinal fluid, Han and colleagues [Citation2] compared EM-seq and PBAT and also found that EM-seq had a higher mapping rate (85 vs 43%), lower duplicate rates (25 vs 35–52%), more CpGs covered at 5X (18.8–22.5M vs 3.2–8.5M) and higher methylation correlation within replicates.
The main purpose of this work was to compare different library and sequencing protocols for DNA methylation measurement and structural variant detection as free add-on information; thus, the tools we used are not comprehensive but common ones. CNVpytor fits methylation sequencing better, as it is read depth-based and does not need split-read or read-pair information like others that may be aligner-specific (e.g., Lumpy needs bwa alignment). From the limited SNV calling tools, we have observed similar properties for the variant callers with a previous comprehensive tool comparison work where the authors found that Bis-SNP was more conservative (higher precision), Biscuit was more sensitive and other tools performed in the middle [Citation21].
Conclusion
In summary, when dealing with low-input DNA for DNA methylation sequencing, EM-seq appears the best choice for data quality, genome coverage and SNV calls, which would enable multiple genomic feature characterizations from a single sequencing experiment. Methylation preprocessing pipelines generally make small differences; however, they differ in handling reads and have different alignment efficiencies. Variant callers can make a noticeable difference, particularly for QIAseq and Swift-seq samples. CNV calling remains challenging in the field, although calls from WGMS are equally as good as traditional WGS.
Whole-genome methylation sequencing (WGMS) carries both DNA methylation and structural variant information; however, reliability for the latter is not well described and evaluated.
Traditional bisulfite treatment methods lead to DNA damage and generally require higher amounts of DNA for methylation sequencing.
Enzymatic methyl sequencing (EM-seq) uses TET2 and T4-BGT enzymes to protect 5 mC and 5 hmC while unmodified cytosines are converted to uracils after APOBEC3A treatment. It is reported to overcome these limitations.
The new EM-seq along with two other bisulfite treatments (QIAseq and Swift-seq) were compared on their performance on DNA methylation measurement and structural variant detections.
EM-seq demonstrated better performance in alignment rates, duplication rates, genome coverage evenness and CpG coverage.
EM-seq generated higher accuracy for SNV detections while CNV detections were similar among libraries.
Different processing pipelines had little impact on DNA methylation measurement; however, they differed in how duplicates were removed, which affected variant calling and CpG coverage.
Overall, EM-seq is better suited to detect methylation, SNVs and CNVs from single sequencing with low-input DNA.
Ethical conduct of research
WR Taylor and JB Kisiel are inventors of Mayo Clinic intellectual property that is licensed to Exact Sciences from which they may receive royalties, paid to Mayo Clinic. No authors have any conflict of interest with the library protocol or kit vendors.
Supplemental Document
Download PDF (322.1 KB)Supplementary data
To view the supplementary data that accompany this paper please visit the journal website at: www.tandfonline.com/doi/suppl/10.2217/epi-2022-0453
Financial & competing interests disclosure
This work was funded by the Mayo Clinic Center for Individualized Medicine, NIH R37CA214679 (to JB Kisiel) and a sponsored research agreement with Exact Sciences (Madison, WI) and Mayo Clinic. The authors have no other relevant affiliations or financial involvement with any organization or entity with a financial interest in or financial conflict with the subject matter or materials discussed in the manuscript apart from those disclosed.
No writing assistance was utilized in the production of this manuscript.
Additional information
Funding
References
- Vaisvila R , PonnaluriVKC , SunZet al. Enzymatic methyl sequencing detects DNA methylation at single-base resolution from picograms of DNA. Genome Res. doi:10.1101/gr.266551.120 (2021).
- Han Y , ZheleznyakovaGY , Marincevic-ZunigaYet al. Comparison of EM-seq and PBAT methylome library methods for low-input DNA. Epigenetics doi:10.1080/15592294.2021.19974061–10 (2021).
- Morrison J , KoemanJM , JohnsonBKet al. Evaluation of whole-genome DNA methylation sequencing library preparation protocols. Epigenetics Chromatin14(1), 28 (2021).
- Feng S , ZhongZ , WangM , JacobsenSE. Efficient and accurate determination of genome-wide DNA methylation patterns in Arabidopsis thaliana with enzymatic methyl sequencing. Epigenetics Chromatin13(1), 42 (2020).
- Sun Z , BahetiS , MiddhaSet al. SAAP-RRBS: streamlined analysis and annotation pipeline for reduced representation bisulfite sequencing. Bioinformatics28(16), 2180–2181 (2012).
- Krueger F , AndrewsSR. Bismark: a flexible aligner and methylation caller for bisulfite-seq applications. Bioinformatics27(11), 1571–1572 (2011).
- Xi Y , LiW. BSMAP: whole genome bisulfite sequence MAPping program. BMC Bioinformatics10, 232 (2009).
- Li H , HandsakerB , WysokerAet al. The sequence alignment/map format and SAMtools. Bioinformatics25(16), 2078–2079 (2009).
- Langmead B , SalzbergSL. Fast gapped-read alignment with Bowtie 2. Nat. Methods9(4), 357–359 (2012).
- Asmann YW , MiddhaS , HossainAet al. TREAT: a bioinformatics tool for variant annotations and visualizations in targeted and exome sequencing data. Bioinformatics28(2), 277–278 (2012).
- Li H , DurbinR. Fast and accurate long-read alignment with Burrows-Wheeler transform. Bioinformatics26(5), 589–595 (2010).
- Mckenna A , HannaM , BanksEet al. The Genome Analysis Toolkit: a MapReduce framework for analyzing next-generation DNA sequencing data. Genome Res.20(9), 1297–1303 (2010).
- Liu Y , SiegmundKD , LairdPW , BermanBP. Bis-SNP: combined DNA methylation and SNP calling for bisulfite-seq data. Genome Biol.13(7), R61 (2012).
- Gao S , ZouD , MaoLet al. BS-SNPer: SNP calling in bisulfite-seq data. Bioinformatics31(24), 4006–4008 (2015).
- Suvakov M , PandaA , DieshC , HolmesI , AbyzovA. CNVpytor: a tool for copy number variation detection and analysis from read depth and allele imbalance in whole-genome sequencing. Gigascience10(11), 1–9 (2021).
- Abyzov A , UrbanAE , SnyderM , GersteinM. CNVnator: an approach to discover, genotype, and characterize typical and atypical CNVs from family and population genome sequencing. Genome Res.21(6), 974–984 (2011).
- Sudmant PH , RauschT , GardnerEJet al. An integrated map of structural variation in 2,504 human genomes. Nature526(7571), 75–81 (2015).
- Haraksingh RR , AbyzovA , UrbanAE. Comprehensive performance comparison of high-resolution array platforms for genome-wide copy number variation (CNV) analysis in humans. BMC Genomics18(1), 321 (2017).
- Layer RM , ChiangC , QuinlanAR , HallIM. LUMPY: a probabilistic framework for structural variant discovery. Genome Biol.15(6), R84 (2014).
- English AC , MenonVK , GibbsRA , MetcalfGA , SedlazeckFJ. Truvari: refined structural variant comparison preserves allelic diversity. Genome Biol.23(1), 271 (2022).
- Lindner M , GawehnsF , TeMolder S , VisserME , Van OersK , LaineVN. Performance of methods to detect genetic variants from bisulphite sequencing data in a non-model species. Mol. Ecol. Resour.22(2), 834–846 (2022).