Abstract
Aim: Wild-type transthyretin amyloid cardiomyopathy (ATTRwt-CM) is frequently misdiagnosed, and delayed diagnosis is associated with substantial morbidity and mortality. At three large academic medical centers, combinations of phenotypic features were implemented in electronic health record (EHR) systems to identify patients with heart failure at risk for ATTRwt-CM. Methods: Phenotypes/phenotype combinations were selected based on strength of correlation with ATTRwt-CM versus non-amyloid heart failure; different clinical decision support and reporting approaches and data sources were evaluated on Cerner and Epic EHR platforms. Results: Multiple approaches/sources showed potential usefulness for incorporating predictive analytics into the EHR to identify at-risk patients. Conclusion: These preliminary findings may guide other medical centers in building and implementing similar systems to improve recognition of ATTRwt-CM in patients with heart failure.
Transthyretin amyloid cardiomyopathy (ATTR-CM) is a life-threatening disease associated with an increased risk of heart failure and other cardiac and noncardiac abnormalities; gradual worsening in physical function and health-related quality of life; and elevated rates of hospitalization, morbidity and mortality [Citation1–5]. The disease is most frequently acquired through age-related aggregation of wild-type transthyretin (TTR) protein, but it may also be inherited as a TTR gene variant [Citation6,Citation7]. The diagnosis of wild-type ATTR-CM (ATTRwt-CM) poses a considerable challenge for patients, clinicians and healthcare organizations, with frequent misdiagnosis and delays in its recognition [Citation8,Citation9]. In a large, prospective, observational study of patients with ATTRwt-CM, the median diagnostic delay was 39 months, and 42% of patients waited more than 4 years after the presentation of cardiac symptoms for a diagnosis [Citation5]. Delayed ATTR-CM diagnosis can lead to unnecessary consultations, testing and treatment prior to diagnosis, as well as more advanced disease at diagnosis [Citation10,Citation11].
Recognition of the potential underdiagnosis of ATTR-CM and substantial disease burden, along with the current availability of disease-modifying, TTR-stabilizing therapy, has highlighted the need for improved screening to facilitate early diagnosis [Citation2,Citation9,Citation12,Citation13]. Recent reports of diagnostic algorithms and expert recommendations have increased awareness of the constructive use of diagnostic “red flags” to prompt suspicion of ATTRwt-CM and noninvasive imaging techniques to effectively screen at-risk patients [Citation9,Citation14–17]. Current consensus recommendations indicate that a noninvasive diagnosis can be reached in select patients with suspected disease based on characteristic echocardiography/cardiac magnetic resonance findings, Grade 2/3 myocardial uptake of radiotracer on bone scintigraphy and exclusion of clonal dyscrasia on appropriate serum and urine tests [Citation18]. However, additional work is required to shorten the diagnostic odyssey of patients with this now treatable but debilitating disease.
In patients with ATTRwt-CM, clinical manifestations, such as arrhythmia, conduction system disease and carpal tunnel syndrome, may develop several years before the onset of heart failure [Citation7,Citation19,Citation20], providing clues for ATTR-CM that can aid earlier diagnosis and treatment [Citation9]. However, clinical decision support (CDS) systems or reporting tools incorporating these clinical manifestations have not yet been developed or implemented to enhance the screening of patients at risk for ATTRwt-CM. As an initial step in addressing this problem, researchers developed and validated a Random Forest-based machine-learning (ML) risk-prediction model for ATTRwt-CM using administrative medical claims data, creating a systematic framework to raise suspicion of potential ATTRwt-CM in patients with heart failure [Citation21]. This model was shown to identify ATTRwt-CM in patients with heart failure with high sensitivity, specificity and accuracy in a large, nationally representative database (87%, 87% and 87%, respectively [IQVIA, NC, USA]). As summarized in the following report, the authors advanced this foundational research by implementing adapted algorithms at the point of care. The authors describe how the adapted versions were implemented in EHRs through a series of pilot studies, with the goal of establishing a resource that enables the systematic screening of patients with heart failure who are at risk for this form of amyloid disease.
Methods
The original ATTR-CM Random Forest prediction model
A Random Forest ML classifier was created to determine the clinical features associated with ATTRwt-CM versus non-amyloid heart failure [Citation21]. The model was derived using International Classification of Diseases 9 and 10 (ICD-9, ICD-10) diagnosis codes in US medical claims data sourced from IQVIA and validated in IQVIA nationally representative medical claims–based and OPTUM® EHR data–based cohorts. The cohorts included patients 50 years of age or older with diagnosis codes for ATTRwt-CM and heart failure (cases) and patients matched for age, sex, duration of medical history and number of healthcare visits with codes for heart failure but not for amyloidosis or amyloid-related conditions (controls). All ICD-9 and ICD-10 codes found in ≥2% of patients with ATTRwt-CM in the IQVIA medical claims data were used as potential predictive features for this Random Forest model; one or more related ICD codes were combined into distinct phenotypes using a phenotype grouping system.
The original Random Forest model performed well in identifying ATTRwt-CM in the heart failure populations, with sensitivity, specificity and accuracy of 87%, 87% and 87% in IQVIA medical claims data and 90%, 79% and 84% in the OPTUM EHR data [Citation21]. The algorithm also subsequently demonstrated consistent performance when tested in similarly defined cases and controls using retrospective external real-world EHR datasets from two large academic medical centers [Citation22].
Adapted ML model design & implementation in pilot studies
Pilot study aim & sites
From the previously developed Random Forest model, the authors created and piloted adaptations within EHR-based CDS and reporting systems with the aim of establishing pragmatic and replicable approaches for medical center staff to use in identifying patients at high risk for ATTRwt-CM. As described below, the authors scaled down the original Random Forest model by selecting high-performing phenotypes and phenotype combinations and tested the adapted versions in three distinct pilot studies. The programs were conducted at academic medical institutions with specialized multidisciplinary amyloidosis centers and large patient databases located in the eastern, midwestern and western US: MedStar Health (Baltimore/Washington, DC, metropolitan area); Washington University (WashU) School of Medicine and affiliated hospitals/clinics (MO, USA); and University of Utah Health (UUH; UT, USA).
Patient criteria & phenotypes/phenotype combinations
In these pilot studies, the authors captured and compared the number of patients with heart failure who were identified as being at risk for ATTRwt-CM based on patient criteria and selected phenotypes/phenotype combinations within different functional EHR designs and different data sources in the EHR. The EHR-based implementation approaches were developed based on technical and clinical considerations. The two most widely used EHR platforms in the USA, Cerner® (MO, USA) and Epic (WI, USA), were used for these pilot studies (Cerner at Medstar, Epic at UUH and WashU).
A series of instructional guides were developed to support the EHR-based CDS and reporting approaches, incorporating patient inclusion/exclusion criteria and high-performing phenotypes and phenotype combinations (). In all three pilot studies, patient criteria were the following: age ≥50 years; presence of diagnostic codes for heart failure (ICD-10 codes I50; active or resolved); and absence of diagnosis codes for end-stage renal disease (N18.6) or amyloidosis (E85).
Table 1. Patient criteria and ATTRwt-CM–associated phenotypes/phenotype combinations implemented in the EHR-based pilot programs.
To select high-performing phenotypes and phenotype combinations, the authors compared the strength of association of the individual phenotypes (from the original Random Forest model [Citation21]) with ATTRwt-CM versus non-amyloid heart failure using logistic regression analyses. For the adapted models employed in the MedStar and WashU programs, nine cardiac and noncardiac phenotypes were selected with odds ratios (ORs) in the highest tertile for prediction of ATTRwt-CM and grouped into 20 phenotype combinations of up to five phenotypes each, based on ORs and performance characteristics. For the UUH program’s adapted model, 11 top predictive phenotypes were selected and grouped into 21 phenotype combinations. A set cover algorithm was used to reduce patient overlap between the combinations, with filtered combinations covering ≥5% of the ATTR-CM population and having an OR >5.0 for identifying patients with ATTRwt-CM versus non-amyloid heart failure. The clinical combinations were developed as a set and were not designed to risk-stratify patients by likelihood of an ATTRwt-CM diagnosis.
CDS/reporting tools & data sources
The CDS approaches explored on the Cerner and Epic platforms were Discern Alert and Best Practice Advisory (BPA), respectively (). CDS alerts for patients who satisfied the specified patient and phenotype criteria were run “silently” in the background to allow assessment of the system’s feasibility and provide information about the triggers. On the Cerner and Epic platforms, EHR reports were run using Discern Analytics 2.0 (DA2) and Reporting Workbench™ (RWB), respectively, in the MedStar Health and WashU pilot studies. Also, based on the available instructional guides, these reporting tools generated and displayed a list of patients who matched the specified criteria and may therefore be at risk for ATTRwt-CM.
The warehouse reports were based on queries run in the analytical systems at each institution (Cerner: Cerner Command Language; Epic: Clarity™ and SlicerDicer) and were used to generate a list of patients who satisfied the phenotype criteria and were at potential risk. No instructional guides were available to assist with development of the reports, but each pilot study generated its own process based on the logic of the existing instructional guides to improve understanding of the findings obtained with the other tools.
On the Cerner platform, clinical condition data displayed on the patient problem list is stored in two separate tables (problem and diagnosis) (), but only one (diagnosis) was employed in the Medstar pilot study. The Medstar Cerner diagnoses data source included billing transactions. On the Epic platform at WashU, three different EHR diagnosis data sources (problem list, encounter diagnosis and medical history) and one non-EHR diagnosis data source (billing transactions) were evaluated separately for functional quality, although these data sources were not available for all CDS and reporting tools. An iterative process was followed for development and testing, so that the authors could better understand the technical, operational and clinical steps needed to integrate the CDS and reporting tools into each EHR system.
Results
All the CDS and reporting tools on the Cerner and Epic platforms were successfully implemented to provide a framework supporting the identification of patients at risk for ATTRwt-CM. Patients included in the at-risk heart failure cohorts and patients identified by the adapted algorithms platform are summarized in by pilot program, platform, configuration and data source. A quantitative comparative analysis of the outputs across programs and configurations was not conducted to detect differences due to variability among the datasets. Each tool (and data source) yielded different counts of at-risk patients in part due to differences in data documentation completeness and data retrieval time frames. Because the pilot studies were conducted at specialized amyloidosis centers, differences in patient referral patterns may also have contributed to differences in data completeness. These constraints, which may have limited the number of at-risk patients identified using some tools, warrant consideration as additional institutions seek to implement ATTR-CM phenotype algorithms into live Cerner or Epic environments in the future.
The most commonly found phenotype combinations associated with ATTRwt-CM in the heart failure cohorts across the three pilot studies are shown in [Citation23,Citation24].
Afib: Atrial fibrillation; ATTR-CM: Transthyretin amyloid cardiomyopathy; CKD: Chronic kidney disease; HF: Heart failure; OA: Osteoarthritis; pts: Patients.
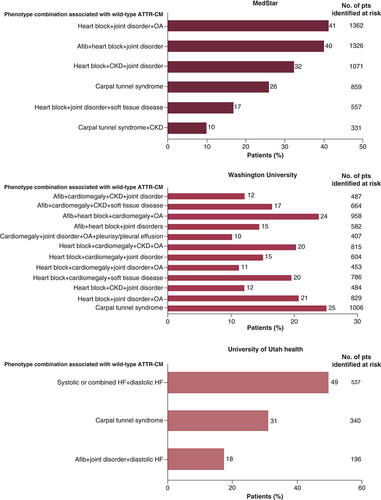
Discussion
Strengths & limitations
The strengths and limitations of the original Random Forest model have been previously summarized [Citation21]. Like the original model, the current adapted algorithm was developed to predict patients at risk for ATTRwt-CM using ICD codes readily available in the EHR. These codes represent the international language of medical diagnoses and are easy to use in the EHR setting. However, conditions documented in patient charts by means other than ICD code (e.g., textual description of echocardiographic findings) were not captured in the algorithm.
On the Cerner platform, the Discern Alert tool was used to identify patients in real time. However, because the tool triggers only during an interaction with a patient’s chart and stops evaluation after finding a single phenotype combination match, further analysis was often needed to obtain the complete clinical profile. In the authors’ experience, the DA2 report was the most comprehensive of the Cerner tools, as it allowed for the longest search interval. The disadvantages of this tool included common performance and timeout issues and its capacity to run only one phenotype combination at a time, which required a greater manual workload to reconcile duplicates.
On the Epic platform, based on their design, BPAs had the advantage of identifying at-risk patients in real time, but these only included patients with an active encounter at the time of the alert. In addition, some diagnosis data sources (e.g., billing transactions) within the system were not available for use as criteria in the BPA logic as it was built in the instructional guides.
The RWB is an operational report for identifying patients in shorter time windows (e.g., 2 weeks or a month), serving as a useful option for clinical care but having limited value in other, broader applications. Because the RWB report was implemented within the EHR, it was run for only a single amyloid specialist provider for the specified period; it could also only be implemented using the problem list data source.
The authors found the Epic Clarity report to be very comprehensive, allowing for the longest search period and most diagnosis data sources. Because of the substantial amount of data gathered, the Clarity reports may require a considerable amount of time to run, but the system did not demonstrate anticipated performance issues with any report configuration.
Finally, SlicerDicer is also only intended to process simple queries and is not capable of scaling complex combinations. Following the authors’ approach, SlicerDicer allowed only one phenotype combination to be implemented at a time, which resulted in a greater manual workload to avoid duplication. An additional consideration is that SlicerDicer presents data at the population level, not the patient level.
Ad hoc & recommended modifications
In the pilot studies, some modifications to the instructional guides were recommended to improve usability and achieve other important learnings that may help guide other organizations in the future. At Medstar Health, for the Cerner platform CDS and reports, the authors updated the instructions to use diagnoses instead of problems because diagnoses were more comprehensive (e.g., they may have included billing diagnoses). Because patient lists “timed out” or took several minutes to run, the authors scheduled reports to run automatically or to use run-time prompts to add additional filters, which reduced the time necessary for staff to receive and review results. The authors also modified build-step sequencing to reduce overall configuration time. Finally, when using Cerner, they observed that the CDS alert fired only for the first qualifying phenotype combination; as a result, training materials for future users should note the need for organizations to review patients’ complete charts and list combinations.
In the Epic platform, because conditions were recorded in multiple sources and in different ways across these sources, the authors recommend that future users evaluate existing diagnosis and problem documentation practices before selecting the sources. For example, because clinicians in healthcare institutions generally do not maintain a complete and accurate problem list, the inclusion of visit diagnoses in the phenotyping process may be advisable. Future users may also choose to examine the feasibility of using billing diagnoses, as these data sources appear to yield the most accurate results. In keeping with its design, the RWB tool timed out when run over long periods and when many patients/groups were included; however, the authors were able to handle this challenge by narrowing the search criteria, such as by querying for one physician, one location or a shorter time interval.
When following their build approach on the Epic platform, the authors noted that the BPA tool did not dynamically display the combination triggering notification, requiring all relevant diagnoses to be listed in the notification window and/or clear language used in the notification. However, future users may overcome these technical obstacles by using a services-based approach such as the HL7® International CDS Hooks specification [Citation25], which enables the generation of dynamic CDS messages. The use of CDS Hooks can allow for increasing numbers of patient problems and diagnoses without requiring a higher level of effort from healthcare organization staff, as the algorithms used to process the diagnoses are encoded by the CDS Hooks service provider rather than configured by the local healthcare system.
The authors acknowledge that other approaches could have been implemented in these pilot studies to achieve the aim of identifying patients at risk of ATTRwt-CM. For example, in the future, Cerner users may consider using the programmable population health management platform HealtheIntent, whereas Epic users may consider additional tools such as registries and programs that enable Clarity-based insights to be incorporated back into the EHR and leveraged within the RWB platform. Moreover, as mentioned, researchers may also choose to follow standards-based approaches such as CDS Hooks for future initiatives.
Conclusion
In these pilot studies, the authors found that predictive phenotype combinations associated with ATTRwt-CM can be successfully implemented using EHR-based tools to identify patients with heart failure at risk for the disease. While encouraged by the numbers of patients with suspected disease “flagged” with these comprehensive screening approaches (although the patient lists were not verified), additional work is needed to help develop a multistep approach and enhance implementation resources that can be used to identify patients with heart failure who are most in need of ATTRwt-CM screening in clinical practice and healthcare systems.
Future perspective
ATTRwt-CM is very difficult to identify in patients with heart failure in part due to the number of other, more common possible etiologies of their disease. However, advances in ML technology and greater availability of patient data are enabling computational solutions to this diagnostic challenge. We adapted and implemented a Random Forest model for identifying patients in the EHR who met prespecified criteria associated with ATTRwt-CM. Although successful, further refinement and investigation are required to achieve more workable approaches that will result in broader adoption. For example, we suggest that further risk stratification (e.g., inclusion of patients >60 years of age) or other modifications be considered to ensure that the estimated workload can be managed with allocated staffing resources. In future research, we also plan to assess performance metrics of the phenotype combinations used in our pilot studies by calculating the frequency of confirmatory diagnoses observed in a sample of patients identified as being at risk. Over time, as the tools’ clinical usefulness improves and they are adopted more widely, their use may lead to important changes in routine evaluation procedures followed for patients with suspected cardiac amyloid disease, such as less reliance on echo-/electrocardiographic findings and invasive tissue biopsy, which could help to shorten and simplify patients’ diagnostic odyssey. Finally, importantly, while the current research focused specifically on ATTRwt-CM, we believe our approaches will likely prove scalable to other difficult-to-diagnose, rare diseases.
Wild-type transthyretin amyloid cardiomyopathy (ATTRwt-CM) is a progressive, life-threatening disease and a frequently underrecognized cause of heart failure in older patients; it is often misdiagnosed or diagnosed late in the disease course, as patients’ heart failure is attributed to more common causes such as hypertension or renal disease.
Several cardiac and noncardiac clinical manifestations are known to be “red flags” for ATTRwt-CM, developing several years prior to heart failure onset in many patients. However, to date, no clinical decision support (CDS) or reporting tools have been developed incorporating these clinical clues to improve screening and identification of patients with the disease.
As reported here, in pilot studies conducted at three large academic medical institutions, the classifier of a previously developed and validated Random Forest machine learning risk-prediction model for ATTRwt-CM was judiciously adapted and implemented into multiple electronic health record CDS and reporting systems.
After selected patient criteria and phenotypes/phenotype combinations were implemented using different data sources and different electronic health record–based CDS and reporting tools on Cerner and Epic platforms, the numbers of patients with heart failure identified as at risk for ATTRwt-CM were compared.
All approaches showed potential usefulness in identifying patients at risk for ATTRwt-CM, but a quantitative comparison of their yield was not conducted due to variability among datasets, related to differences in data documentation completeness, data collection time frames and patient referral patterns.
The phenotypes/phenotype combinations most often associated with ATTRwt-CM in patients with heart failure in the three pilot studies included carpal tunnel syndrome; heart block plus joint disorders plus osteoarthritis; heart block plus chronic kidney disease plus joint disorders; atrial fibrillation plus heart block plus joint disorders; and atrial fibrillation plus heart block plus cardiomegaly plus osteoarthritis.
Based on the pilot program experiences, each approach had strengths and limitations that will require consideration with future use, including the potential need for ad hoc modifications to overcome obstacles encountered at the time of implementation.
Additional work is under way to refine the approaches piloted at these medical centers and to further examine their usefulness and applicability in clinical practice and healthcare system settings.
Author contributions
All authors participated sufficiently in the work to take responsibility for the manuscript content; made substantial contributions to the conception or design of the work or to data acquisition, analysis or interpretation; drafted the work or revised it critically for important intellectual content; approved the submitted version; and agreed to be accountable for all aspects of the work.
Ethical conduct of research
Use of the datasets from the respective health system electronic health records was approved by the institutional review boards at MedStar Health, Washington University School of Medicine and University of Utah Health. Informed consent was waived under each institution’s institutional review board regulations. The use of patient data was performed in accordance with the Declaration of Helsinki.
Financial & competing interests disclosure
This study was sponsored by Pfizer. Medical writing support was provided by D McGuire of Engage Scientific Solutions and funded by Pfizer. C Willis, A H Watanabe and J Biskupiak have received research grants from Pfizer. J Hughes has no disclosures to report. K Nolen, J O’Meara, A Schepart and M Bruno are full-time employees of Pfizer and own Pfizer stock and/or stock options. K Kawamoto reports receiving sponsored research grants, writing assistance, consulting fees and honoraria from Pfizer. N Shara has received advisory board honoraria from Pfizer. T Kannampallil has received consulting fees from Pfizer and compensation from Elsevier for serving on editorial boards. The authors have no other relevant affiliations or financial involvement with any organization or entity with a financial interest in or financial conflict with the subject matter or materials discussed in the manuscript apart from those disclosed.
No writing assistance was utilized in the production of this manuscript.
References
- Rapezzi C , QuartaCC, RivaLet al. Transthyretin-related amyloidoses and the heart: a clinical overview. Nat. Rev. Cardiol.7(7), 398–408 (2010).
- Ruberg FL , GroganM, HannaM, KellyJW, MaurerMS. Transthyretin amyloid cardiomyopathy: JACC state-of-the-art review. J. Am. Coll. Cardiol., 73(22), 2872–2891 (2019).
- Castaño A , DrachmanBM, JudgeD, MaurerMS. Natural history and therapy of TTR-cardiac amyloidosis: emerging disease-modifying therapies from organ transplantation to stabilizer and silencer drugs. Heart Fail. Rev., 20(2), 163–178 (2015).
- Ruberg FL , MaurerMS, JudgeDPet al. Prospective evaluation of the morbidity and mortality of wild-type and V122I mutant transthyretin amyloid cardiomyopathy: the Transthyretin Amyloidosis Cardiac Study (TRACS). Am. Heart J., 164(2), 222–228.e221 (2012).
- Lane T , FontanaM, Martinez-NaharroAet al. Natural history, quality of life, and outcome in cardiac transthyretin amyloidosis. Circulation, 140(1), 16–26 (2019).
- Ruberg FL , BerkJL. Transthyretin (TTR) cardiac amyloidosis. Circulation, 126(10), 1286–1300 (2012).
- Koike H , OkumuraT, MuroharaT, KatsunoM. Multidisciplinary approaches for transthyretin amyloidosis. Cardiol. Ther., 10(2), 289–311 (2021).
- Gertz M , AdamsD, AndoYet al. Avoiding misdiagnosis: expert consensus recommendations for the suspicion and diagnosis of transthyretin amyloidosis for the general practitioner. BMC Fam. Pract., 21(1), 198 (2020).
- Witteles RM , BokhariS, DamyTet al. Screening for transthyretin amyloid cardiomyopathy in everyday practice. JACC Heart Fail., 7(8), 709–716 (2019).
- Ladefoged B , DybroA, PovlsenJA, VaseH, ClemmensenTS, PoulsenSH. Diagnostic delay in wild type transthyretin cardiac amyloidosis – a clinical challenge. Int. J. Cardiol., 304, 138–143 (2020).
- Bishop E , BrownEE, FajardoJ, BarouchLA, JudgeDP, HalushkaMK. Seven factors predict a delayed diagnosis of cardiac amyloidosis. Amyloid, 25(3), 174–179 (2018).
- Adams D , Gonzalez-DuarteA, O’RiordanWDet al. Patisiran, an RNAi therapeutic, for hereditary transthyretin amyloidosis. N. Engl. J. Med., 379(1), 11–21 (2018).
- Maurer MS , SchwartzJH, GundapaneniBet al. Tafamidis treatment for patients with transthyretin amyloid cardiomyopathy. N. Engl. J. Med., 379(11), 1007–1016 (2018).
- Dorbala S , AndoY, BokhariSet al. ASNC/AHA/ASE/EANM/HFSA/ISA/SCMR/SNMMI expert consensus recommendations for multimodality imaging in cardiac amyloidosis: part 1 of 2 – evidence base and standardized methods of imaging. J. Card. Fail., 25(11), e1–e39 (2019).
- Dorbala S , AndoY, BokhariSet al. ASNC/AHA/ASE/EANM/HFSA/ISA/SCMR/SNMMI expert consensus recommendations for multimodality imaging in cardiac amyloidosis: part 2 of 2 – diagnostic criteria and appropriate utilization. J. Nucl. Cardiol., 27(2), 659–673 (2020).
- Gillmore JD , MaurerMS, FalkRHet al. Nonbiopsy diagnosis of cardiac transthyretin amyloidosis. Circulation, 133(24), 2404–2412 (2016).
- American Society of Nuclear Cardiology . ASNC practice points: 99mTechnetium-pyrophosphate imaging for transthyretin cardiac amyloidosis (2019). https://www.asnc.org/content.asp?contentid=290
- Garcia-Pavia P , RapezziC, AdlerYet al. Diagnosis and treatment of cardiac amyloidosis: a position statement of the ESC Working Group on Myocardial and Pericardial Diseases. Eur. Heart J., 42(16), 1554–1568 (2021).
- Fosbøl EL , RørthR, LeichtBPet al. Association of carpal tunnel syndrome with amyloidosis, heart failure, and adverse cardiovascular outcomes. J. Am. Coll. Cardiol., 74(1), 15–23 (2019).
- Rapezzi C , MerliniG, QuartaCCet al. Systemic cardiac amyloidoses: disease profiles and clinical courses of the 3 main types. Circulation, 120(13), 1203–1212 (2009).
- Huda A , CastañoA, NiyogiAet al. A machine learning model for identifying patients at risk for wild-type transthyretin amyloid cardiomyopathy. Nat. Commun., 12(1), 2725 (2021).
- Heitner S , ElmanMR, MasriAet al. Performance evaluation of a machine learning model for systematic identification of wild-type transthyretin amyloid cardiomyopathy at two academic medical centers. J. Card. Fail., 26(Suppl. 10), S38 (2020).
- Nolen K , BrunoM, MitchellJet al. Applying phenotypes to operationalize high-yield clinical features derived from a heuristic artificial intelligence model for a rare disease in the EHR. Annual meeting of the American Medical Informatics Association. Virtual, 18–20 May 2020.
- Willis C , KawamotoK, WatanabeAet al. Screening of patients at risk for wild type ATTR-CM using a computational machine learning algorithm. American College of Cardiology 70th Annual Scientific Session & Expo. Virtual, 15–17 May 2021.
- HL7 & Boston Children’s Hospital . HL7® International CDS Hooks (2019). https://cds-hooks.hl7.org/