Abstract
A central phase in the evaluation of a biomarker is the assessment of its clinical validity. In most cases, clinical validity will be expressed in terms of the marker's diagnostic accuracy: the degree to which it can be used to identify diseased patients or, more generally, patients with the target condition. Diagnostic accuracy is evaluated in diagnostic accuracy studies, in which the biomarker values are compared to the outcome of the clinical reference standard in the same patients. There are several ways in which the results of diagnostic accuracy studies can be summarized, reported, and interpreted. We present an overview and a critical commentary of the available measures. We classify them as error-based measures, information-based measures, and measures of the strength of the association.The diagnostic accuracy is not a fixed property of a marker. All accuracy measures may vary between studies, not just through chance, but also with changes in the definition of the target condition, the spectrum of disease, the setting, and the amount of prior testing. We discuss the relativity of the claim that likelihood ratios are a superior way of expressing the accuracy of biomarkers, and defend the use of the sometimes downgraded statistics sensitivity and specificity.
Introduction
A biomarker should not just have analytical validity; it should also be clinically useful. This can be evaluated by assessing whether the marker measures anything clinical meaningful and, ultimately, by seeing whether use of the biomarker in clinical practice leads to a gain in health outcome, or to a more efficient use of health care resources.
If the biomarker is proposed for diagnostic purposes, one can evaluated its diagnostic accuracy. The diagnostic accuracy of a marker is its ability of a marker to distinguish between patients with and patients without disease or, more generally, between those with and without the target condition [Citation1].
There are several ways in which the results of studies to evaluate the diagnostic accuracy of a test can be summarized and reported. This paper presents an overview and a critical commentary of the available measures. It tries to do so from the perspective of decision making, in which accuracy studies must provide the evidence to guide decisions, decisions about approval for marketing, about purchasing, decisions whether or not to include a test in practice guidelines, or decisions about test ordering and interpretation in individual patients.
The first part of this paper presents the design of a diagnostic accuracy study and summarizes existing measures for reporting accuracy studies. The second offers a critical analysis of these measures. We defend the use of the sometimes downgraded sensitivity and specificity, and discuss the relativity of the claim that likelihood ratios are a superior way of expressing diagnostic accuracy. In subsequent sections we discuss how diagnostic test accuracy should be looked at in an appraisal of claims about health benefits of biomarkers. In a final section we also discuss the quantification of the performance of biomarkers used for other purposes. The presentation is based on a more general analysis of diagnostic accuracy statistics for medical tests [Citation2].
Diagnostic accuracy studies
In studies of diagnostic accuracy, the outcomes from one or more tests are compared with outcomes of the reference standard in the same study participants. The clinical reference standard is the best available method to establish the presence of the target condition in patients. The target condition can be a target disease, a disease stage, or some other condition that qualifies patients for a particular form of management. The reference standard can be a single test, a series of tests, a panel based decision, or some other procedure [Citation3]. For simplicity, we will assume that the results of the biomarker can be classified as positive, pointing to the presence of disease, or negative. We also assume that the target condition is either present or absent, and that the clinical reference standard is able to identify it in all patients.
A shows the basic structure of a typical diagnostic accuracy study. B offers an example of a (hypothetical) diagnostic accuracy study of kallikrein-related peptidase 2 (KLK2), to help identifying patients with prostate cancer in screening. KLK2 is a secreted serine protease from the same gene family as prostate-specific antigen. In the study in B, needle biopsy was used as the reference standard.
Figure 1. A schematic representation of a diagnostic accuracy study (A: left) and an example of a group to be screened by a marker (KLK2) (B: right).
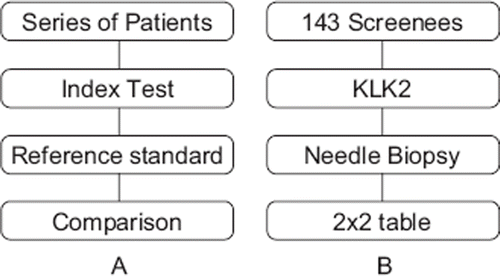
There are several potential threats to the internal and external validity of a study of diagnostic accuracy. Poor internal validity will produce bias, or systematic error, because the estimates do not correspond to what one would have obtained using optimal methods. Poor external validity limits the generalisability of the findings. In that case, the results of the study, even if unbiased, do not correspond to the data needs of the decision-maker.
The ideal study examines a consecutive series of patients, enrolling all consenting patients suspected of the target condition within a specific period. All of these patients then undergo the index test and then all undergo the reference test. Alternative designs are possible, some of which can be quite difficult to unravel. Some studies first select patients known to have the target condition, and then contrast the results of these patients with those from a control group. If the control group consists of healthy individuals only, diagnostic accuracy of the test will be overestimated. Studies of diagnostic tests with a suboptimal design are known to produce biased estimates. Unfortunately, studies of diagnostic accuracy suffer from poor reporting [Citation4]. The STARD initiative has been set up to improve the completeness and transparency of reporting [Citation3].
summarizes the results of the study of the accuracy of KLK2 in screening men for prostate cancer. These results were actually inspired by a similar study, performed and reported by Martin and colleagues in the Journal of Urology [Citation5]. The table reports four numbers, one in each cell, corresponding to the true and false positives, and the true and false negatives. shows a number of measures that can be calculated from the data obtained, grouped in three categories. In the following subsection we discuss these three groups of measures.
Error-based measures
Accuracy refers to the quality of the diagnostic classification by the test under evaluation: its ability to correctly identify patients with cancer as such. One of the measures of diagnostic accuracy is the overall fraction correct, sometimes also referred to as simple ‘accuracy’. In the example in , 45% of the study patients were correctly classified by the KLK2 test.
The overall fraction correct is usually not a very helpful measure. With most conditions, there is a substantial difference between a false positive and a false negative test result. In the KLK2 example, a false negative test result would mean that a screening participant with prostate cancer is sent home with false reassurance. A false positive test result most likely means that a man without prostate cancer is referred for further testing. Needle biopsy is uncomfortable and carries a risk, but that risk cannot be compared to missing a case of prostate cancer. Total accuracy does not offer us that distinction between the false positive and the false negatives.
Two of the more frequently used measures of diagnostic accuracy take the differential nature of misclassification errors into account. The diagnostic, or clinical, sensitivity of the test is the proportion of the diseased correctly classified as such. In the example of , the sensitivity of the test is estimated as 98%. Its counterpart is the diagnostic specificity: the proportion of the patients who do not have the target condition correctly classified as such. In the example of , the specificity of the test is estimated as 21%. Sensitivity and specificity go hand in hand. If the threshold for positive results were set at a lower concentration , the number of true positives would increase, but so would the number of false positives.
Table I. Diagnostic accuracy study results.
Table II. Measures for reporting and interpreting results of diagnostic accuracy studies.
Youden's J index is an alternative single measure of error-based accuracy [Citation6]. It can be defined in many ways, one of them being the true positive fraction minus the false positive fraction [Citation7]. If the positivity rate is the same in patients with prostate cancer and patients without prostate cancer, the test is useless and the Youden index is zero. With a perfect test, all prostate cancer patients are positive and there are no false positives, so the Youden index is 1. Youden's index has the advantage of being a single measure, but it also looses the distinction between the false positives and the false negatives. So do other error-based measures, such as the area under the ROC curve.
Information based measures
Sensitivity and specificity describe how well the test is able to identify patients with the target disease. They do not tell, however, what to make of a single test result. There are a number of alternative measures that express the information value in specific test results.
The positive predictive value of a test is the proportion of patients with a positive test result that actually have the target condition. Its counterpart, the negative predictive value, stands for the proportion of patients with a negative test result that do not have the target condition. For the study results summarized in , the positive predictive value is estimated at 36% and the negative predictive value as 95%. If the KLK2 test is used to rule out prostate cancer, the negative predictive value tells us the proportion of patients sent home after a negative KLK2 result who did not have prostate cancer. The positive predictive value tells us the prevalence of prostate cancer in those referred for needle biopsy after a positive KLK2 result.
Positive and negative predictive values have been criticized because they vary with prevalence. In screening, for example, the prevalence of prostate cancer will differ from that in a urology outpatient department, and so will the positive and negative predictive values. These predictive values can be interpreted as risks: the risk of disease in marker positives versus the risk in marker negatives. In clinical trials, the inverse of the risk difference is used to calculate the number needed to treat, and some have suggested that the inverse of the difference in predictive values can be interpreted as the number needed to test. That cannot be true, as, unlike the choice of treatment, the test result is not something that can be assigned. The number needed to test can indeed be calculated, but based on the downstream consequences of testing, as the inverse of the expected value of complete information [Citation4].
Predictive values are essentially group based measures. Large differences may exist in the strength of pre-test suspicion of prostate cancer in a clinical setting, based on the patient's presentation, his risk factors, and findings from history, and physical examination. The positive predictive value ignores all of that: it just tells us the proportion of cancer patients within those with a positive test result. A different set of information-based measures has been proposed as an alternative: diagnostic likelihood ratios. The likelihood ratio (LR) of a particular test result is the proportion of subjects with the target condition who have that test result relative to the proportion without the target condition who have the same test result. Unlike sensitivity and specificity, LRs can also be obtained for tests that can have multiple, or even continuous, test results, without the need for dichotomization [Citation2]. If such a test is evaluated in an accuracy study, a set of LRs, one for each test result, or a LR function, will be reported.
Diagnostic LRs can be used with subjective pre-test probability expressions and Bayes’Theorem. The pre-test odds of the target condition being present – the pre-test probability relative to one minus that probability – must be multiplied with the LR of the obtained test result to produce the post-test odds the post-test probability relative to one minus that probability. LRs above unity increase the probability of disease, whereas LRs lower than one decrease that probability. If the pre-test probability of prostate cancer is 0.01, for example, the pre-test odds will be 1 to 99. After a positive KRK test 2, and using the data in , the post-test odds would be 1.24 to 99, and the post-test probability must be 0.012 (1.24/(1.24+99)).
McGee has suggested a very rough simplification for the interpretation of LRs [Citation9]. For him, clinicians must remember only 3 LRs – 2, 5, and 10 – and the first 3 multiples of 15: 15, 30 and 45. For probabilities between 10% and 90%, a LR of 2 increases the probability with approximately 15%, an LR of 5 with 30%, and a LR of 10 with 45%. For likelihood ratios less than 1, the rule works in the opposite direction.
Association based measures
Diagnostic accuracy studies can also be used to estimate the strength of the association between the index test results and the outcomes of the reference standard. Better tests will show stronger associations with the clinical reference standard. Some of the familiar epidemiology statistics are called upon to express the strength of associations.
One such statistic is the odds ratio, in this context also known as the diagnostic odds ratio [Citation10]. The odds ratio expresses the odds of positivity in the diseased relative to the odds of positivity in the non-diseased. A property of the odds ratio is that this also equals the odds of disease in those with a positive test result relative to the odds of disease in those with a negative test result. Unlike the odds ratios in most other areas of epidemiology, diagnostic odds ratios tend to be quite high. In the example in , the diagnostic odds ratio of the KLK2 is estimated at 11.2.
The odds ratio is a single measure, in contrast to many other measures, which come in pairs. Odds ratios can be used to make rapid comparisons of tests used for the same target condition. Yet the absence of the differential nature of the information and of the errors limit their use for decision-making.
The error odds ratio is another odds ratio: It expresses the odds of correct classification in the diseased relative to the odds of correct classification in the non-diseased [Citation10]. That may be informative to some, but tells us little about the quality of the test, as both classification odds may be quite high, or both quite low, and that distinction is lost in calculating the ratio.
One could also calculate relative risks: the risk of disease is the marker positives versus the risk of disease in marker negatives. This has been called the positive predictive ratio [Citation9]. Although quality tests will have more extreme relative risks, it is unclear how such relative risks should be used in any form of decision making.
Even more association-based measures have been used and proposed for diagnostic accuracy studies, such as kappa statistics, and adjusted kappa statistics [Citation10]. Kappa statistics express chance-corrected agreement, but that agreement is highly dependent on the prevalence in a particular study. Similar objections apply against Pearson's correlation coefficient, and other measures of agreement.
In short, measures of the strength of the association between test and reference standard, borrowed from epidemiology, are rarely helpful for interpreting accuracy studies to support decision making. The diagnostic and test evaluation literature borrows probably too heavily from the general epidemiological literature, which may be seen as a sign of its immaturity.
Accuracy: A variable test property
Sensitivity and specificity tell us something about the conditional classification quality of the test, but they are not intrinsic test characteristics. The values that sensitivity and specificity take are conditional on the target condition, on how that target condition is defined, and on the clinical reference standard. Sensitivity and specificity do not only differ based on the target condition and the reference standard used. They are also likely to vary with clinical setting. KLK2 may behave differently in inpatients compared to outpatients. Test accuracy will also vary with the level of pre-testing. Sensitivity and specificity should be looked upon as group averages. They vary across groups, but they will also vary within subgroups [Citation13,Citation14].
Most demonstrations of variability in accuracy have focused on sensitivity and specificity. Yet there is no evidence that other types of measures are unaffected, on the contrary. If the true positive and false positive fractions vary, so will the likelihood ratios, and this should lead to some caution in unconditional application of Bayes’ theorem to individual patients.
Researchers should spend more time on exploring and documenting that variability, because it could be useful for practice [Citation15]. Finding conditions that are more likely to generate false positives, for example, could be extremely useful. The methodology for doing such analyses is well available [Citation16]. Unfortunately, we do not find such explorations on a regular basis in the literature. One reason could be the fact that many – if not most – diagnostic accuracy studies have limited sample size. In a survey of papers on tests used for reasons other than population screening, Bachman and colleagues showed that the median sample size was 119, and the median number with the target condition was only 49 [Citation17]. These numbers are small for reliable estimates of diagnostic accuracy, and way too small for explorations of sources of heterogeneity in accuracy. Meta-analysis may help in obtaining more precise estimates, but they cannot act as a substitute for exploring sources of variability [Citation10].
In principle, Bayes’ theorem should only be used for a single patient on two conditions. The first is that the clinician's pretest probability is an adequate and substantiated expression of the strength of suspicion in that patient. The second condition is that the likelihood ratio expresses how much more likely a positive result, say, is in that patient, if diseased, compared to when that patient were not diseased. Applying a published, group-based likelihood ratio to a specific patient's test result to generate that patient's post-test probability is a leap of faith. Such a leap can only sensibly be made if it based on a solid understanding of that test, and the modifying effects of any condition, such as age, sex and co-morbidity, that may affect the rates of false positives or false negatives.
Sensitivity and specificity or likelihood ratios?
The concepts of sensitivity and specificity are usually attributed to Yerushalmy's work in the 1940s, but they are older than that [Citation19]. The notions became more prominent in medical science after the 1959 Science paper by Ledley and Lusted, although the terms themselves do not appear as such in that paper [Citation20]. Ledley and Lusted discussed the use of conditional probabilities and Bayes’ theorem, and stated that these conditional probabilities can be grounded in medical knowledge, unlike the probabilities of disease in single patients.
A series of authors have challenged the prominence of these error-based notions and lamented their widespread use. We can arrange the criticism in two lines of thinking, each arriving at a plea for information-based accuracy measures.
One form of criticism stresses that, for practical reasons, predictive values are what matters most, expressed as probabilities, calculated with the help of diagnostic probability functions [Citation21–23]. These functions not only include the test result, but also all other available information with diagnostic value, such as data from history, from the physical examination, and all other test results. Using such functions, one can also evaluate the added value of new medical tests.
The second form of criticism discards error-based measures in favor of a more widespread use of diagnostic likelihood ratios. The proponents of this view can be found in many sectors of the evidence-based medicine movement [Citation24–26]. The first users’ guide to the medical literature, for example, published by the EBM group, used the availability of likelihood ratios as an essential element in the critical appraisal of reports of diagnostic accuracy studies [Citation26].
Some of the arguments in favour of likelihood ratios can be regarded as a sign of proselyte EBM enthusiasm, and are not always grounded on the truth. An example: “While predictive values relate test characteristics to populations, likelihood ratios can be applied to a specific patient.” [Citation26]. As discussed before, the likelihood ratios are just as well calculated on the basis of groups, they are subject to variability and bias. Applying them in individual patients is an act of judgment. Another quote from the same paper: “Moreover, likelihood ratios, unlike traditional indices of validity, incorporate all four cells of a 2×2 table, and thus is more informative than any of the other measures alone.” [Citation26]. This is not a fair reflection of the truth; most statistics discussed so far, including the sensitivity-specificity pair (when considered together), rely on all four cells of the 2×2 table.
The argument that likelihood ratios are more intuitive can also be challenged [Citation27]. Most likely none of the measures presented here is very intuitive, as few of us humans are trained to think in terms of probabilities. Likelihood ratios are, in isolation, not very helpful for comparing tests or making decisions about tests. It has been suggested, for example, that test for which the LR of one of its result is 20 or more, or 10 or more, are very useful [Citation26,Citation28]. Imagine then a study of a KLK2 with a positive LR of 37. Then learn that this test was positive in only 3 of the 2311 patients: one of the 2193 without prostate cancer – a specificity almost 100% – but positive in just 2 of the 118 prostate cancer patients – a sensitivity of 2%. Despite the high LR, a test that is positive in barely 1 in every 1000 is unlikely to be useful.
Concluding remarks
There seems to be some confusion about the statistics to report after a diagnostic accuracy study, and what to look for when interpreting the results. Some authors seem to be LR believers, while others believe in the logistic-regression based diagnostic function. Still others seem to be confused, and report every statistic mentioned in this paper. A paper on a study to evaluate ST-segment elevation in predicting acute occlusion in patients with acute coronary syndrome reported no less than a bewildering number of 11 accuracy measures [Citation11].
We would like to propose that measures have to be selected based on the type of clinical study question that is to be answered. If the study question regards the evaluation of the quality of one or more tests, the error-based measures are probably better suited to summarize the results. In the KLK2 example, the quality of the assay to rule out prostate cancer can be judged by an appraisal of its sensitivity, as this indicates the proportion of prostate cancer patients that would be missed by the test.
Early evaluations of a new assay can evaluate the sensitivity for various subtypes of the target condition, or the specificity in different classes of non-diseased, such as these with specific co-morbidities or conditions that mimic the target condition, such as other infections when evaluating test for infectious diseases. Sensitivities and specificities can also come into play when considering the use of tests in guidelines and clinical flowcharts.
Many questions about tests are comparative in nature [Citation29]. Is KLK2 better than free PSA? Is a qualitative point-of-care test as good as a quantitative test? Can the number of negative biopsies after KLK2 testing be reduced by inserting another triage test in the workup, such as cPSA? For replacement questions, relative true and false positive fractions can be calculated, and hypotheses of superiority or equivalence can be statistically tested [Citation16].
Studies aimed at helping clinicians in interpreting test results, on the other hand, should consider reporting primarily in terms of information-based measures, possibly using likelihood ratios or diagnostic functions. For practical purposes, one should consider the transformation of these functions into simple decision rules or ‘fast and frugal heuristics’ [Citation30].
In the end, one important question has not yet been addressed in this paper: why accuracy? Some authors have argued that not only sensitivity and specificity are overrated, but that the whole accuracy paradigm is woefully inadequate for adequately expressing the benefits and harms of testing [Citation31,Citation32]. It is based on a definition of true disease, on definitive evidence, that was formerly found only on post-mortem examination and it does not show the effects of testing on patient outcome [Citation33].
Does that mean we then have to abandon the accuracy paradigm, and move to evaluations of clinical utility altogether? Probably not. To be useful, we only need a redefinition of what the clinical reference standard is supposed to detect. The pathological gold standard of disease has to be traded in for the notion of a target condition. The clinical reference standard should not be asked to distinguish the diseased from the non-diseased, but to identify those that are better off with a particular form of treatment or, more generally, management, versus those that are not. Such information could come from subgroup analysis in randomized clinical trials, or other evidence [Citation34]. In terms of health outcomes, evaluating how well the target condition can be detected is an intermediate outcome measure. With claims of superior sensitivity for a new test, when a test identifies more cases, it is not unlikely that randomized clinical trials or other forms of research are needed to show that these additional cases benefit as much from treatment, or other interventions, as the cases identified by the older tests [Citation35]. After all, diagnosis is but a stepping stone to treatment, to changes in outcome, and to benefits in individual patients.
Redefining the reference standard may not be enough, especially when the test is used for purposes other than diagnosis. We should keep in mind that diagnostic accuracy is but one way of looking at clinical validity, a far wider concept. The process of clinically validating biomarkers, or medical tests, will usually involve the exploration of significant relations between marker results on the one hand, and results from other tests on the other hand, as well as clinical characteristics. Based on prior clinical knowledge, one should be able to predict the likely magnitude of associations between the marker and items from the patients’ history, clinical examination, imaging, laboratory tests, function tests, or disease severity scores. The process of marker validation is completed if the predicted pattern of associations is empirically conformed.
Declaration of interest: The authors report no conflicts of interest. The authors alone are responsible for the content and writing of the paper.
References
- Knottnerus JA, van WC, Muris JW. Evaluation of diagnostic procedures. BMJ 2002;324(7335):477–80.
- Bossuyt PM. Interpreting diagnostic test accuracy studies. Semin Hematol 2008;45(3):189–95.
- Bossuyt PM, Reitsma JB, Bruns DE, Gatsonis CA, Glasziou PP, Irwig LM, . Towards complete and accurate reporting of studies of diagnostic accuracy: the STARD initiative. Standards for Reporting of Diagnostic Accuracy. Clin Chem 2003;49(1):1–6.
- Smidt N, Rutjes AW, van der Windt DA, Ostelo RW, Reitsma JB, Bossuyt PM, . Quality of reporting of diagnostic accuracy studies. Radiology 2005;235(2):347–53.
- Martin BJ, Cheli CD, Sterling K, Ward M, Pollard S, Lifsey D, . Prostate specific antigen isoforms and human glandular kallikrein 2–which offers the best screening performance in a predominantly black population? J Urol 2006;175(1): 104–7.
- Youden WJ. Index for rating diagnostic tests. Cancer. 1950; 3(1):32–5.
- Hilden J, Glasziou P. Regret graphs, diagnostic uncertainty and Youden’s Index. Stat Med 1996;15(10):969–86.
- Hunink MG, Glasziou P, Siegel JE, Weeks JC, Pliskin JS, Elstein AS, . Decision Making in Health and Medicine: Integrating Evidence and Values: Cambridge University Press;2001.
- McGee S. Simplifying likelihood ratios. J Gen Intern Med 2002;17(8):646–9.
- Glas AS, Lijmer JG, Prins MH, Bonsel GJ, Bossuyt PM. The diagnostic odds ratio: a single indicator of test performance. J Clin Epidemiol 2003;56(11):1129–35.
- Rostoff P, Piwowarska W, Gackowski A, Konduracka E, El MN, Latacz P, . Electrocardiographic prediction of acute left main coronary artery occlusion. Am J Emerg Med. 2007;25(7):852–5.
- Kraemer HC.Evaluating medical tests: objective and quantitative guidelines:Sage Publications 1992.
- Moons KG, van Es GA, Deckers JW, Habbema JD, Grobbee DE. Limitations of sensitivity, specificity, likelihood ratio, and bayes’ theorem in assessing diagnostic probabilities: a clinical example. Epidemiology 1997;8(1):12–7.
- Diamond GA. Reverend Bayes’ silent majority. An alternative factor affecting sensitivity and specificity of exercise electrocardiography. Am J Cardiol 1986;57(13):1175–80.
- Irwig L, Bossuyt P, Glasziou P, Gatsonis C, Lijmer J. Designing studies to ensure that estimates of test accuracy are transferable. BMJ 2002;324(7338):669–71.
- Pepe MS.The Statistical Evaluation of Medical Tests for Classification and Prediction: Oxford University Press; 2003.
- Bachmann LM, Puhan MA, ter RG, Bossuyt PM. Sample sizes of studies on diagnostic accuracy: literature survey. BMJ 2006;332(7550):1127–9.
- Leeflang MM, Deeks JJ, Gatsonis C, Bossuyt PM. Systematic reviews of diagnostic test accuracy. Ann Intern Med 2008;149(12):889–97.
- Lilienfeld DE. Abe and Yak: the interactions of Abraham M. Lilienfeld and Jacob Yerushalmy in the development of modern epidemiology (1945–1973). Epidemiology 2007;18(4): 507–14.
- Ledley R, Lusted L. Reasoning foundations of medical diagnosis. Science 1959;130:9–21.
- Miettinen OS, Henschke CI, Yankelevitz DF. Evaluation of diagnostic imaging tests: diagnostic probability estimation. J Clin Epidemiol 1998;51(12):1293–8.
- Moons KG, Harrell FE. Sensitivity and specificity should be de-emphasized in diagnostic accuracy studies. Acad Radiol 2003;10(6):670–2.
- Guggenmoos-Holzmann I, van Houwelingen HC. The (in)validity of sensitivity and specificity. Stat Med 2000;19(13): 1783–92.
- Perera R, Heneghan C. Making sense of diagnostic tests likelihood ratios. Evid Based Med 2006;11(5):130–1.
- Jaeschke R, Guyatt G, Sackett DL. Users’ guides to the medical literature. III. How to use an article about a diagnostic test. A. Are the results of the study valid? Evidence-Based Medicine Working Group. JAMA 1994;271(5): 389–91.
- Chien PF, Khan KS. Evaluation of a clinical test. II: Assessment of validity. BJOG. 2001;108(6):568–72.
- Puhan MA, Steurer J, Bachmann LM, ter RG. A randomized trial of ways to describe test accuracy: the effect on physicians’ post-test probability estimates. Ann Intern Med 2005;143(3):184–9.
- Fischer JE, Bachmann LM, Jaeschke R. A readers’ guide to the interpretation of diagnostic test properties: clinical example of sepsis. Intensive Care Med 2003;29(7):1043–51.
- Bossuyt PM, Irwig L, Craig J, Glasziou P. Comparative accuracy: assessing new tests against existing diagnostic pathways. BMJ 2006;332(7549):1089–92.
- Hutchinson JM, Gigerenzer G. Simple heuristics and rules of thumb: where psychologists and behavioural biologists might meet. Behav Processes 2005;69(2):97–124.
- Feinstein AR. Misguided efforts and future challenges for research on “diagnostic tests”. J Epidemiol Community Health 2002;56(5):330–2.
- Mrus JM. Getting beyond diagnostic accuracy: moving toward approaches that can be used in practice. Clin Infect Dis 2004;38(10):1391–3.
- Fryback DG, Thornbury JR. The efficacy of diagnostic imaging. Med Decis Making 1991;11(2):88–94.
- Bossuyt PM, Lijmer JG, Mol BW. Randomised comparisons of medical tests: sometimes invalid, not always efficient. Lancet 2000;356(9244):1844–7.
- Lord SJ, Irwig L, Simes RJ. When is measuring sensitivity and specificity sufficient to evaluate a diagnostic test, and when do we need randomized trials? Ann Intern Med 2006;144(11):850–5.