Abstract
Background: Programme evaluation of medical education should be multi-dimensional. While structural and organisational aspects of teaching are frequently assessed, programme evaluation tools are rarely matched to specific learning objectives.
Aims: This study used one medical school's catalogue of specific learning objectives to implement and critically appraise a novel programme evaluation tool based on comparative student self-assessments.
Method: Medical students enrolled in the clinical phase of the undergraduate curriculum in Göttingen were invited to self-rate their knowledge, skills and attitudes before and after each course. A newly developed formula controlling for student performance levels when entering a course was used to compute a percentage gain in knowledge, skills and attitudes. Data derived from a prospective, longitudinal intervention study on the development of electrocardiogram interpretation skills including 636 students from four consecutive cohorts were used to provide validity evidence of the new approach.
Results: The novel tool appeared superior to plain mean differences and effect sizes in detecting outstanding teaching as well as shortcomings of the curriculum. In addition, it adequately reflected objectively measured performance levels and was responsive to curriculum change.
Conclusions: Comparative student self-assessment is a valid tool to appraise undergraduate medical curricula at the level of specific learning objectives.
Introduction
Programme evaluation in higher education is defined as ‘a systematic process that judges the worth of an educational programme’ (Maudsley Citation2001). In the context of medical education, there is substantial ambiguity as to what should be regarded as the principal ‘worth’ of medical training. For the Dean, the medical profession and society, becoming a ‘good’ doctor (Hurwitz & Vass Citation2002) is the ultimate goal of medical education. Valid programme evaluation instruments ideally should assess the extent to which this goal is met during medical education. As a consequence, evaluation data might be used to identify shortcomings of a curriculum in order to guide programme modification.
The first decade of the twenty-first century has witnessed the advent of outcome-based medical education which is characterised by an emphasis on learning outcomes and their assessment (Shumway & Harden Citation2003). In this context, a clear definition of learning objectives is as crucial as aligning teaching and assessment methods to these objectives (Kern et al. Citation1998), and educational institutions are required to document their outcomes (LCME Citation2003). Interestingly, programme evaluation appears to lag behind current developments in curriculum research in that many medical schools (at least in our country) still use traditional evaluation forms focusing on student satisfaction with courses and organisational/structural aspects of teaching. Since they are not aligned to specific learning objectives, such global ratings yield little information on the extent to which specific objectives have been met during a particular course. In addition, they have been shown to be severely biased by numerous confounders (Naftulin et al. Citation1973; Marsh Citation1982; Divoky Citation1995; Jackson et al. Citation1999).
In order to obtain valid data on the true impact of teaching, evaluation tools should focus on student learning outcome (Gibson et al. Citation2008). The gold standard for evaluating student performance levels is a summative assessment which is usually placed at the end of a course or academic year. However, due to differing performance levels of students entering a course, final examination results cannot reflect the actual gain of knowledge, skills and attitudes that has occurred during a course. In order to detect specific achievements and shortcomings of particular courses, a comparison of performance levels of individual students before and after course participation is necessary. The regular implementation of a pre- and post-test design in medical education is costly and further complicated by the fact that learning objectives pertaining to professionalism and communication are genuinely hard to assess (Epstein Citation2007). While considerable advances have been made towards developing a more integrated assessment system which will allow more valid inferences to be drawn on teaching and learning effectiveness (Holmboe et al. Citation2010), the aim of this study was to develop, implement and critically appraise an outcome-based programme evaluation tool which uses student comparative self-assessments (CSA) and addresses all domains of medical education (knowledge, skills and attitudes).
The research questions thus addressed were:
How do the results obtained with the CSA tool compare to established methods to measure pre-post differences? These methods included a visualisation of mean values and standard errors, calculation of plain differences as well as effect sizes expressed as Cohen's d (Cohen Citation1992) with values above 0.8 representing large effects.
Does the CSA tool produce stable and reproducible results?
Are matched pair-wise comparisons of student self-assessments necessary?
Do student self-assessments adequately reflect objectively measured performance levels?
Is the CSA tool responsive to curricular change?
Methods
The 6-year undergraduate medical curriculum at our institution comprises 2 pre-clinical and 3 clinical years, followed by a practice year. The clinical part of the curriculum has a modular structure: there are 21 modules lasting two to 7 weeks each; the sequence of modules is identical for all students. The CSA tool was implemented for all the 21 modules in the clinical curriculum. However, in order to address the research questions listed above, objective student performance measurements before and after participation in a module were needed. These were derived from a larger study including four consecutive cohorts of medical students (winter term 2008/09 until summer term 2010) who were enrolled in the 6-week interdisciplinary cardio-respiratory module which occurs at the beginning of the second clinical year. The results of that study were partially published in 2010 (Raupach et al. Citation2010).
Development of the new programme evaluation tool
An evaluation committee consisting of experienced faculty as well as experts on medical education discussed methods to assess potential increases in skills, knowledge and attitudes during the 21 modules. In order to circumvent the logistic challenge of implementing pre- and post-tests covering all educational domains in all modules, a decision was made to compare student self-ratings of knowledge, skills and attitudes before and after each module. Identical questionnaires were made available to students 3 days prior to and after the first and last day of a module, respectively. For each module, 15 questions covering the principal teaching content were used. Items were matched to the Göttingen Medical School's Catalogue of Specific Learning Objectives (2008), and members of the evaluation committee cross-checked each item for alignment to the catalogue. For example, students were asked to rate the statement ‘I can interpret an electrocardiogram.’ on a scale from 1 (fully agree) to 6 (completely disagree). Data collection periods were standardised using an automated online survey system (EvaSys®, Electric Paper, Lüneburg, Germany). For ethical and privacy reasons, students could neither be forced to use the CSA tool nor to reveal their identity. As a consequence, participation was voluntary and anonymous, thus precluding matched pair-wise comparisons of individual self-assessments.
The gain in knowledge, skills and attitudes that occurred during a module was defined as the difference in mean ratings (pre/post) within a student cohort enrolled in the module. In order to adjust for students’ differing initial performance levels, item-specific gain (%) was computed according to the following formula (Formula 1):where μpre is the mean initial self-assessment and μpost the mean self-assessment after the course.
According to this formula, the large net increase in self-assessment from 5.0 to 3.0 would produce the same gain (50%) as the much smaller net increase from 2.0 to 1.5. Thus, the formula takes into account the difficulty of further increasing skills, knowledge and attitudes in advanced students. Starting in winter 2008/09, all modules were evaluated using CSA (315 specific learning objectives). In addition to using sample items from different modules to illustrate the tool's capability to reflect gains in all three educational domains, evaluation data obtained from four consecutive student cohorts enrolled in the cardio-respiratory module were used to address research questions 1 and 2 (‘How do the results obtained with the CSA tool compare to established methods to measure pre-post differences?’ and ‘Does the CSA tool produce stable and reproducible results?’). Computed gains for five specific learning objectives were compared to effect sizes (Cohen Citation1992), and cross-cohort comparisons of both measures were made to assess the stability of results obtained with the new programme evaluation tool.
Pair-wise versus aggregated differences in self-assessments
Data derived from a large longitudinal study including a total of 636 students were used to address research questions 3, 4 and 5. As part of that study (Raupach et al. Citation2010), students were invited to take a written test on electrocardiogram (ECG) interpretation skills both on the first day and during the last week of the module. At both time-points, student self-ratings of their performance level were obtained before the test was taken. Research question 3 (‘Are matched pair-wise comparisons of student self-assessments necessary?’) was addressed by comparing the skills gain using mean ratings (Formula 1) with the mean of individual skills gains computed as follows (Formula 2):
In addition to comparing individual and CSA gain values, we investigated the impact of varying response rates on CSA gain calculated from mean ratings: the participation rate in the ECG study was almost 100% in all four cohorts. By including the statement ‘I can interpret an electrocardiogram’ in both the CSA tool (varying response rate) and the study questionnaire (∼100% participation), we were able to compare skills gains derived from the entire cohort with skills gains derived from the subset of students who voluntarily provided self-assessments before and after the module.
Objective performance measurements
On the first day of the cardio-respiratory module, students enrolled in the ECG study were asked to produce a written interpretation of three ECG tracings. During the last week of the module, the same students took a second examination including five different tracings. Only unambiguous ECGs with medically important findings (i.e. myocardial infarction, atrial fibrillation and ventricular hypertrophy) were used for these assessments. Details of the marking process are described elsewhere (Raupach et al. Citation2010). In brief, two independent raters completed a standardised checklist. Following a first exploration of results, the marking scheme was adjusted to emphasising the most important findings, thus producing a maximum of 10 points per tracing (i.e. 30 points in the first and 50 points in the second examination). All assessments were identical in both study cohorts. In order to avoid contamination, all test materials were collected after each assessment and model answers not provided. In an attempt to address research question 4 (‘Do student self-assessments adequately reflect objectively measured performance levels?’) and, thus, to establish criterion validity of the self-assessment approach, individual performance levels at the beginning and the end of the module were compared to individual self-ratings. In addition, mean percent scores for student cohorts were calculated for both the entry and the exit examination, and the effect size of the pre-post change was compared to the skills gain as computed using Formula 1.
The four student cohorts differed with respect to the intensity of teaching and assessment: in both winter terms, exit examinations were summative and yielded a considerable amount of credit points for students. In both summer terms, exit examinations were formative in nature. Extensive teaching (lectures and peer-led small-group discussions) was offered to all students in the first two cohorts (winter 08/09 and summer 2009), while students in the latter two cohorts (winter 09/10 and summer 2010) were offered three introductory lectures and then asked to self-study a 40-page guide to ECG interpretation. These differences in assessment and instructional format were expected to be reflected in both the objective performance measurement and the CSA tool (research question 5: ‘Is the CSA tool responsive to curricular change?’).
Data acquisition, statistical analysis and ethics approval
Students completing the post-evaluation of a module were asked to indicate whether they had participated in the pre-evaluation of that same module. Data analysis was restricted to students who participated in both evaluations. Data analysis was performed with SPSS® 14.0 (Illinois, USA). Aggregated group data are given as mean ± standard error of the mean (SEM). Correlation coefficients are given as Pearson's r. Differences in mean values between the four cohorts were analysed using the Kruskal–Wallis–H Test. Effect sizes were calculated as Cohen's d with values above 0.8 indicating large effects (Cohen Citation1992). Significance levels were set to 5%. At our institution, studies requiring students to provide anonymous ratings are exempt from Institutional Review Board (IRB) approval. Data derived from the larger ECG study are reported according to IRB approvals no. 23/2/09, 18/8/09 and 1/3/10.
Results
Response rates and descriptive analysis of evaluation results
Response rates per module varied between 36.7% and 75.4%. Sample items, including mean values and their SEM as well as the proportion of students who had chosen each of the six options from ‘fully agree’ to ‘completely disagree’, are visualised in . Nine of the 315 learning objectives out of 21 modules are displayed to illustrate that, in each educational domain (cognitive/skills/affective), gain values between 10% and 80% were obtained. As expected, similar differences in mean values produced either large or medium-sized gains, depending on prior levels of performance. The statement ‘I know which agents can be used to treat urinary tract infections’ yielded a negligible gain (). Several items with small gains were discussed with students and teachers as well as programme administrators. We consistently observed that teaching performance regarding these items was inadequate. In the case of the objective no. 6, faculty organising the module had in fact overseen it while planning the module. As a result, there had been no teaching on urinary tract infections.
Figure 1. Illustration of evaluation data presentation to faculty. One sample learning goal with high, moderate or low learning outcome is displayed for each of the three learning domains (knowledge, skills and attitudes). Questions were translated from their original German into English. Error bars indicate standard errors of the mean. CSA, comparative self-assessment.
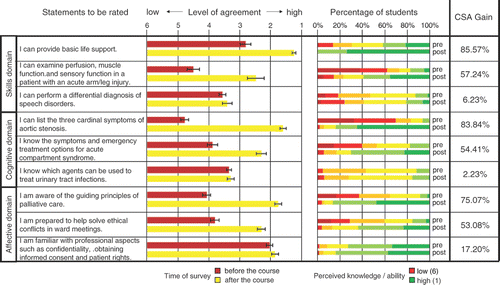
Comparison of different methods measuring performance increase
In order to address research questions 1 and 2 (‘How do the results obtained with the CSA tool compare to established methods to measure pre-post differences?’ and ‘Does the CSA tool produce stable and reproducible results?’), five distinct learning objectives assigned to the cardio-respiratory module were evaluated in four consecutive student cohorts. Teaching and assessment of these items remained unchanged during the study period to facilitate a critical appraisal of the reproducibility of CSA gain values. As these data were derived from the voluntary evaluation, response rates need to be taken into account. These were 58.0%, 62.0%, 59.7% and 57.0% in the four cohorts, respectively.
Three major findings are presented in : first, particularly large (dark green line) and small (dark red line) differences in mean values were adequately reflected in both the effect size and the CSA gain (Formula 1). Second, all methods produced different rankings of learning objectives: while – on the basis of plain differences or the effect size – the learning objective represented by the light green line could be considered as producing only a moderate effect, accounting for the relatively high performance level at module entry revealed a favourable CSA gain. Likewise, despite similar mean values and SEMs at the end of the module (), the two learning objectives represented by the light and dark green lines could be clearly distinguished in the gain plot (). Finally, the new programme evaluation tool appeared to yield reproducible results across student cohorts. The effect size for the learning objective represented by the dark green line showed considerable variation between the cohorts. This was unlikely to be caused by differing response rates as these were very similar (around 60%) in all four cohorts. Rather, the relatively low effect size observed in the fourth cohort was due to a higher variance of mean self-assessment values at module entry (compare ). In contrast to effect sizes, the CSA gain produced stable results as should be expected when considering the original data shown in .
Figure 2. Comparison of different methods measuring differences in student self-ratings before and after attending the cardio-respiratory module. Each colour represents one specific learning objective: dark green – pharmacotherapy for heart failure; light green – therapy for acute myocardial infarction; yellow – heart murmurs; orange – signs of heart failure on physical examination; red – therapeutic options for peripheral vascular disease. (A) mean values of student ratings before (dotted lines) and after (solid lines) the module. Error bars indicate standard errors of the mean. (B) absolute differences in mean values for each learning objective across the four cohorts. (C) effect sizes of pre-post changes in student self-assessments. (D) CSA gain computed from Formula 1 (see text).
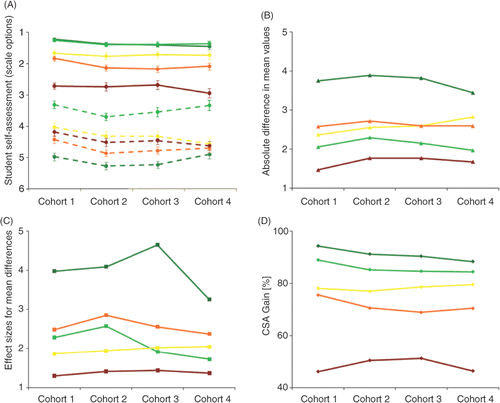
Impact of comparison type (matched vs. unmatched) and response rate on computed gain
In order to address research question 3 (‘Are matched pair-wise comparisons of student self-assessments necessary?’), individual (pair-wise) gain values were calculated using Formula 2 and averaged across each student cohort. The values thus obtained were compared to the CSA gain derived from aggregated cohort data (Formula 1). illustrates the correlation between the two measures (r = 0.992, p = 0.008), both of which were derived from the results of voluntary module evaluation with varying response rates.
Figure 3. Impact of comparison type (matched vs. unmatched) and response rate on computed gain for the learning objective ‘ECG interpretation’. Each data point represents a student cohort. (A) correlation between gains computed from pair-wise comparisons and gains computed from group mean comparisons (r = 0.992, p = 0.008). (B) correlation between gains calculated for all students and those calculated for the subgroup of students voluntarily using the new programme evaluation tool (r = 0.981, p = 0.019).
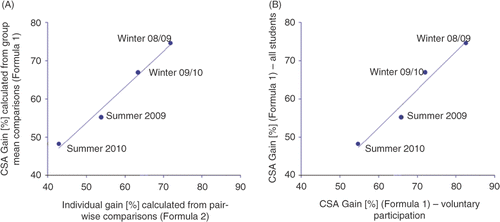
The impact of varying response rates on CSA gain was assessed by comparing data derived from the ECG Study (∼100% participation) with data obtained as part of the voluntary module evaluation. The correlation between gain values computed for all students and those computed for students who voluntarily used the CSA tool are displayed in (r = 0.981, p = 0.019).
Relation to objective performance measurements
Research questions 4 (‘Do student self-assessments adequately reflect objectively measured performance levels?’) and 5 (‘Is the CSA tool responsive to curricular change?’) were addressed by comparing student self-ratings at the beginning and the end of the module with their respective performance levels in the entry and exit examinations. illustrate that, at both time-points, higher self-perceived competence to interpret an ECG is associated with significantly higher exam performance (p < 0.001, Kruskal–Wallis–H Test). As scores in the entry examination were similar in all four cohorts (i.e. no adjustment for prior performance level was necessary), effect sizes could be used to compare the actual increase in performance to the CSA gain. There was a significant correlation between the two measures (r = 0.980, p = 0.02, ). As expected, the summative assessments used in both winter terms led to a substantially greater increase in performance, and this was also reflected in the gain computed from student self-assessments.
Figure 4. Relation of calculated gain values to objective performance measurements. (A) student performance level in the ECG entry examination by self-perceived competence to interpret an ECG (n = 636). (B) student performance level in the ECG exit examination by self-perceived competence to interpret an ECG (n = 636). (A/B) p values derived from Kruskal–Wallis–H Test. (C) correlation between pre-post performance difference effect sizes and gain as computed from student self-ratings (r = 0.980, p = 0.020). CSA, comparative self-assessment.
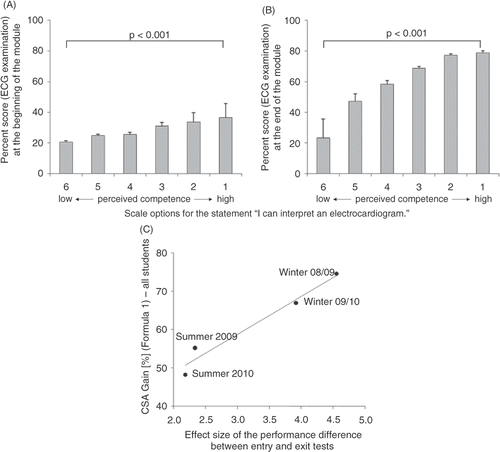
Discussion
In this study, comparative student self-assessments were used to measure the extent to which specific learning objectives had been achieved during a teaching module. With regard to the research questions raised, the above results indicate that CSA gain compares favourably with more traditional measures of pre- and post-differences. In contrast to the effect size, it is robust against variances in mean values at module entry. Unlike the plain difference between pre- and post-values, CSA gain accounts for student performance levels when entering a module. Second, cross-cohort comparisons of learning objectives that were taught and assessed identically in all cohorts revealed that the new method produces more stable results than effect sizes. Third, gain calculations from matched pair-wise comparisons yielded the same results as calculations using group mean values, indicating that student identification is not necessary. Fourth, with regard to the practical skill ‘ECG interpretation’, there was good agreement between CSA gain and objective measures of performance. Finally and most importantly, CSA gain appeared to be responsive to changes in the curriculum in that learning objectives for which there was no teaching produced no gain, whereas interventions impacting on student performance in objective examinations were adequately reflected in the data produced by the new programme evaluation tool. Taken together, our results suggest that CSA gain is a reproducible and valid tool to measure student performance gain on the level of specific learning objectives.
Advantages of the novel method
To our knowledge, this is the first study to report a curriculum-wide implementation of an evaluation tool that is matched to specific learning objectives from all three major domains of medical education. The information gathered from CSA gain may add to the value of existing multi-dimensional evaluation programmes (McOwen et al. Citation2009; Holmboe et al. Citation2010). In addition to being easy to implement and resource-saving, this ‘outcome-based evaluation tool’ (Harden Citation2007) can contribute to faculty development by reminding faculty of the learning objectives to be met by their students. In turn, it will help programme coordinators judge whether specific learning objectives have been met or not. By taking into account students’ self-assessed performance levels before entering a specific course, CSA gain might also prove useful for the appraisal of spiral curricula in which students repeatedly face similar learning objectives at different levels of expertise (Harden & Stamper Citation1999).
Limitations and suggestions for future research
In medical education research, there is a long history of comparing student self-ratings before and after educational interventions (Bray-Hall et al. Citation2010). In fact, Thompson et al. recently reported the detection of a student learning curve based on repeated self-assessments (Thompson & Rogers Citation2008). However, the validity of self-assessments has been challenged (Eva & Regehr Citation2005; Davis et al. Citation2006) due to the high inter- and intra-individual variabilities of this measure (Ward et al. Citation2002). At the same time, research in medical education indicates that the ability to self-assess is relatively stable over time (Fitzgerald et al. Citation2003). In agreement with our findings of good correlations between CSA gain and ECG examination score difference effect sizes, student self-ratings of clinical behaviours have been shown to adequately reflect performance in an objective assessment involving standardised patients (Frank et al. Citation2005). However, more research is needed on the validity of the new approach in the context of learning objectives pertaining to factual knowledge and professionalism.
One surprising finding of this study was that pair-wise comparisons of student data yielded the same results as aggregated group data. Absolute CSA gain appears to increase with decreasing response rates (), but its capacity to detect curricular change remains untouched. Thus, although a 100% response rate (Gerrity & Mahaffy Citation1998) might not be necessary to produce reliable data, the question of a possible lower cut-off for response rates to be judged acceptable warrants further discussion.
At present, CSA gain data are used to critically appraise teaching and learning of specific learning objectives within modules at our institution. Cross-module or even cross-institution comparison is an intriguing possibility. In accordance with recent attempts to reward outstanding teaching successes (Humanities Citation2008), one might envision resource allocation within medical schools being partially guided by results obtained with the new tool.
Conclusion
By comparing student self-assessments regarding specific learning objectives before and after participation in medical school courses, reproducible and valid programme evaluation data can be obtained. Thus, CSA gain may add to the value of existing evaluation methods. A particular strength of this approach is its alignment to the specific learning objectives of a given medical school. Future research should address problems associated with low response rates as well as the validity of the approach in different domains of medical education.
Acknowledgements
The authors thank everyone involved in the development of the new programme evaluation tool. In particular, the input of Wolfgang Himmel, Werner Albig, Peter-Ulrich Haders, Thomas Kleinöder, Monja Tullius and Jakob Schumacher is greatly valued.
Declaration of interest: The authors report no conflicts of interest. The authors alone are responsible for the content and writing of the paper.
References
- Bray-Hall S, Schmidt K, Aagaard E. Toward safe hospital discharge: A transitions in care curriculum for medical students. J Gen Intern Med 2010; 25: 878–881
- Cohen J. A power primer. Psychol Bull 1992; 112: 155–159
- Davis DA, Mazmanian PE, Fordis M, Van Harrison R, Thorpe KE, Perrier L. Accuracy of physician self-assessment compared with observed measures of competence: A systematic review. JAMA 2006; 296: 1094–1102
- Divoky J. Eliciting teaching evaluation information interactively. J Educ Business 1995; 70: 317–332
- Epstein RM. Assessment in medical education. N Engl J Med 2007; 356: 387–396
- Eva KW, Regehr G. Self-assessment in the health professions: A reformulation and research agenda. Acad Med 2005; 80: S46–S54
- Fitzgerald JT, White CB, Gruppen LD. A longitudinal study of self-assessment accuracy. Med Educ 2003; 37: 645–649
- Frank E, McLendon L, Denniston M, Fitzmaurice D, Hertzberg V, Elon L. Medical students’ self-reported typical counseling practices are similar to those assessed with standardized patients. MedGenMed 2005; 7: 2
- Gerrity MS, Mahaffy J. Evaluating change in medical school curricula: How did we know where we were going?. Acad Med 1998; 73: S55–S59
- Gibson KA, Boyle P, Black DA, Cunningham M, Grimm MC, McNeil HP. Enhancing evaluation in an undergraduate medical education program. Acad Med 2008; 83: 787–793
- Harden RM. Learning outcomes as a tool to assess progression. Med Teach 2007; 29: 678–682
- Harden RM, Stamper N. What is a spiral curriculum?. Med Teach 1999; 21: 141–143
- Holmboe ES, Sherbino J, Long DM, Swing SR, Frank JR. The role of assessment in competency-based medical education. Med Teach 2010; 32: 676–682
- Hurwitz B, Vass A. What's a good doctor, and how can you make one?. BMJ 2002; 325: 667–668
- Jackson DL, Teal CR, Raines SJ, Nansel TR, Force RC, Burdsal CA. The dimensions of students’ perceptions of teaching effectiveness. Educ Psychol Measure 1999; 59: 580–596
- Kern DE, Thomas PA, Howard DM, Bass EB. Curriculum development for medical education – A six-step approach. The John Hopkins University Press, Baltimore and London 1998
- Liaison Committee on Medical Education (LMCE). Accreditation Guidelines for New and Developing Medical Schools. Available from: http://www.lcme.org/newschoolguide.pdf [Accessed 14 June 2011]
- Marsh HW. SEEQ: A reliable, valid, and useful instrument for collecting students’ evaluations of university teaching. Br J Psychol 1982; 52: 77–95
- Maudsley G. What issues are raised by evaluating problem-based undergraduate medical curricula? Making healthy connections across the literature. J Eval Clin Pract 2001; 7: 311–324
- McOwen KS, Bellini LM, Morrison G, Shea JA. The development and implementation of a health-system-wide evaluation system for education activities: Build it and they will come. Acad Med 2009; 84: 1352–1359
- Naftulin DH, Ware JE, Jr, Donnelly FA. The Doctor Fox Lecture: A paradigm of educational seduction. J Med Educ 1973; 48: 630–635
- Raupach T, Hanneforth N, Anders S, Pukrop T, Th JtCO, Harendza S. Impact of teaching and assessment format on electrocardiogram interpretation skills. Med Educ 2010; 44: 731–740
- Shumway JM, Harden RM. AMEE Guide no. 25: The assessment of learning outcomes for the competent and reflective physician. Med Teach 2003; 25: 569–584
- The German Council of Science and Humanities (Wissenschaftsrat). 2008. Empfehlungen zur Qualitaetsverbesserung von Lehre und Studium (Drs. 8639–08). Berlin.
- The Göttingen Medical School's Catalogue of Specific Learning Objectives. 2008. Göttingen Medical School.
- Thompson BM, Rogers JC. Exploring the learning curve in medical education: Using self-assessment as a measure of learning. Acad Med 2008; 83: S86–S88
- Ward M, Gruppen L, Regehr G. Measuring self-assessment: Current state of the art. Adv Health Sci Educ Theory Pract 2002; 7: 63–80