Abstract
During their course, medical students have to become proficient in a variety of competencies. For each of these competencies, educational design can use cognitive load theory to consider three dimensions: task fidelity: from literature (lowest) through simulated patients (medium) to real patients (highest); task complexity: the number of information elements in a learning task; and instructional support: from worked examples (highest) through completion tasks (medium) to autonomous task performance (lowest). One should integrate any competency into a medical curriculum such that training in that competency facilitates the students’ journey that starts from high instructional support on low-complexity low-fidelity learning tasks all the way to high-complexity tasks in high-fidelity environments carried out autonomously. This article presents twelve tips on using cognitive load theory or, more specifically, a set of four tips for each of task fidelity, task complexity, and instructional support, to achieve that aim.
Introduction
Medical students have to develop a number of competencies during their training, such as conducting a patient interview, selecting appropriate diagnostic procedures, and formulating a management plan. A variety of concepts and principles of curriculum design can help teachers when making decisions on how to structure their courses in order to support student learning. Using a set of concepts and principles from cognitive load theory (Young et al. Citation2014; Leppink et al. Citation2015), this twelve tips article attempts to bridge theory and practice in curriculum development. In essence, cognitive load theory deals with the potential problem that the processing demands evoked by learning tasks as complex as those in medical training may exceed the processing capacity of the learner’s cognitive system (i.e. they cause overload) or that the learner’s cognitive resources are allocated in a suboptimal manner. This is a challenge for both teachers and students, as meaningful learning in medicine often requires substantial cognitive processing using a cognitive system that is inherently limited (namely, the human brain). In cognitive load theory, learning and instruction are structured in such a way that we take into account the mental effort being used in the working memory when dealing with a particular task.
Task fidelity, task complexity, and instructional support as core issues
When using cognitive load theory as the principal model, curriculum planners ought to consider three issues: task fidelity, task complexity, and instructional support (Leppink et al. Citation2015). These three issues can be conceived as three dimensions of a cube, as depicted in .
Figure 1. A holistic model for how to integrate a competency into a medical curriculum (Leppink & Van den Heuvel Citation2015) applied to learning how to make a (particular type of) diagnosis: (1) simple textual diagnoses with and then without instructional support; (2) more complex textual diagnoses with and then without instructional support; (3) simple diagnoses in a simulated environment with and without instructional support; (4) more complex diagnoses in a simulated environment with and without instructional support; (5) simple diagnoses in a real patient environment; and (6) more complex diagnoses in a real patient environment.
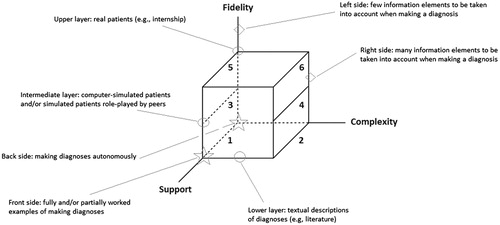
The first issue, task fidelity, includes initial learning from textbooks and other literature, and advanced learning with simulated patients and eventually real patients. The second issue, task complexity, pertains to the difficulty of a learning task or activity. For example, a task becomes more complex when it has a shorter duration, it is unfamiliar to the learner, or includes a large amount of new information. Finally, the third or instructional support issue includes the use of technology, and the selection of learning and teaching resources. It extends all the way from fully worked examples to autonomous task performance.
Using competencies to individualize learning
When designing, implementing, assessing and evaluating a medical program, there is value in using an organizing framework of competencies. The benefits of a clearly defined framework include the opportunity to standardize learning outcomes, while allowing flexibility and the opportunity to progress as learners achieve competency milestones (Irby et al. Citation2010). Applying the cube model allows for individualizing the learning experience, and enables students to move through different competencies at different paces. It also allows for curriculum design to tweak the instructional format to the individual student’s level of expertise. An example might help to illustrate this further. In learning about the management options for diabetes, the most useful technique for novice learners might include an overview of the various strategies and worked examples with explanation on clinical decision-making rather than asking students to solve a clinical problem and search for solutions by themselves. As expertise increases, one might present cases in a smaller group and encourage learners to present their suggested treatment plan without any kind of template. It might be helpful to consider that competencies can be conceptualized as paralleled cubes in the model as is visualized in .
Figure 2. Medical competencies and topics as paralleled cubes (Leppink & Van den Heuvel Citation2015) in a coherent program.
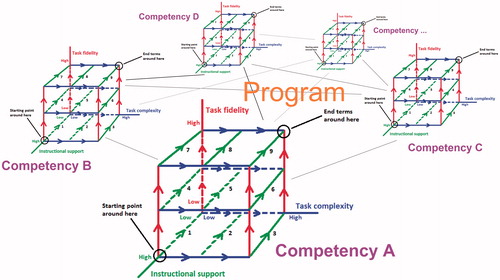
That is, instructional support, task complexity and task fidelity require continuous attention. Besides, competency development is typically not a process of developing one after another; students receive training in a variety of competencies at the same time. Medical programs across the globe use progress testing as a means to assess students’ development throughout the curriculum of some of these competencies (e.g. medical knowledge, basic science knowledge) and stimulate that development (Schuwirth & Van der Vleuten 2012).
Twelve tips
Whichever competency we are considering – patient care, communication skills, professionalism, intercultural communication, or other – we have to work from a high-support low-complexity low-fidelity starting point to clearly defined end terms presenting an ability to function under low-support high-complexity high-fidelity circumstances. Therefore, we discuss ways to integrate learning tasks and activities that facilitate an individual student’s journey through a medical curriculum and start from high instructional support on low-complexity low-fidelity learning tasks all the way to high-complexity tasks in high-fidelity environments carried out autonomously. We do this in the form of a set of four tips for each of task fidelity, task complexity and instructional support.
Task fidelity
Learning how to make a particular type of diagnosis can be practiced first by reviewing textual descriptions, subsequently through practice with computer-simulated patients and/or patients role-played by peers, next through simulated patients played by more professional actors, and finally with real patients in an internship (Van Merriënboer & Sweller Citation2010).
Tip 1
Determine the number of fidelity levels
Whether all of the aforementioned levels of fidelity – literature, each of the types of simulated patients, real patients – are needed may depend on students’ prior knowledge, the competency under consideration, and choices with regard to competency end terms. Besides, logistic factors may put some constraints on for instance how many and which types of simulated patients are feasible. However, having at least one type of simulation appears necessary for a friendly transition from literature to real patients.
Tip 2
Gradually increase fidelity
Starting in a high-fidelity environment too early may overload students in two ways, especially when accompanied with a lack of support. Firstly, high-fidelity environments may comprise so many elements students are still unfamiliar with that their working memories are simply overloaded. Lower-fidelity environments typically allow for more control with regard to having learners focus on specific elements and practice with these elements first. Secondly, emotions evoked in higher-fidelity environments may certainly at first contribute to redundant working memory load and as such inhibit learning if these emotions stimulate bias (Kahneman Citation2011) or result in having students focus on the events that caused these emotions rather than on the process of coming to a diagnosis itself (Fraser, et al. Citation2012,Citation2014). It is therefore important that fidelity is increased gradually. For example, when learning about psychiatric diagnoses such as depression, curriculum design can deal with increasing fidelity by introducing students to paper-based cases first (low fidelity), before exposing them to scenarios with a simulated patient (medium fidelity) or an immersive experience on the psychiatric ward talking to real patients (high fidelity). In the case of surgical procedures, high-fidelity tasks such as laparoscopic colectomy for early learners could overload learners and pose patient safety risks, and lower fidelity tasks are likely more appropriate for early surgical learners (Young & Sewell 2015). This includes part-task trainers whereby students perform basic tasks in isolation, such as camera manipulation, hand-eye coordination, tissue dissection and hand-transferring skills.
Tip 3
Stimulate an ongoing dialogue between trainer and student
Different students have different levels of experience, learn at different paces, and deal with emotions in somewhat different manners. Consequently, some students may be ready for a higher level of fidelity sooner than other students will. Making appropriate decisions with regard to which level of fidelity is appropriate for a given student requires an ongoing dialogue between trainer and trainee. This implies amongst others the need for repeated evaluation (or assessment) moments throughout a trajectory rather than having a single evaluation session at the end of a trajectory.
Tip 4
Moving to higher fidelity requires appropriate instructional support
Tips 1–3 should not be interpreted as a statement that exposing inexperienced students to higher-fidelity contexts is necessarily a bad thing. However, we must be aware of students’ zone of proximal development (Vygotsky Citation1978) or the distance between what a given student can perform autonomously and what this student could learn with the help from an expert or in collaboration with more capable peers. To avoid overload, starting at or moving to a higher level of fidelity should be accompanied by sufficient instructional support.
Task complexity
Independent of fidelity levels, some diagnoses are more complex than others in that there are more factors to take into consideration, more sources of information to integrate and/or more “unknowns” in the case. Likewise, an integrated physical examination is of a higher level of complexity than doing a series of movements in the right sequence.
Tip 5
Determine the number of complexity levels
As for fidelity levels, choices with regard to the extent of variation in complexity depend on students’ prior knowledge, the competency under consideration, choices with regard to competency end terms, and logistic factors. Failing to differentiate in complexity, we may under-load some students (Young & Stanton Citation2002) and overload others (Kalyuga & Hanham Citation2011).
Tip 6
Gradually increase complexity
The more a student advances, the more complexity – in terms of for instance factors to consider in a diagnosis or in terms of the elaborateness of a sequence of moves – this student can handle. To neither under-load nor overload the student, we should strive for a gradual increase in task complexity (Van Merriënboer & Sweller Citation2010; Leppink & Van den Heuvel Citation2015). An example of this gradual increase could be from problem-based learning whereby paper cases progress from simple to more complex by making the presenting symptoms atypical and the diagnosis less clear. Likewise, in the case of mastering clinical skills, consider a resident in acute care whereby increasingly complex patients (e.g. higher acuity, more comorbidities) are treated with more autonomy. The gradual increase in complexity stems from the number of interacting elements (time-pressure, unfamiliarity, large amounts of information) and needs to be carefully anticipated and regulated by the supervisor.
Tip 7
Stimulate self-regulation
Different students deal with complexity in somewhat different manners. Consequently, some students may be ready for a higher level of complexity sooner than other students will. Determining which level of complexity is appropriate for which student may be facilitated to some extent through self-regulated learning. Self-regulated learning thrives on two key processes: monitoring and control (Nelson & Narens Citation1990; Zimmerman & Schunk Citation2001). The term monitoring refers to the thoughts learners have about their cognition, whereas the term control is used to refer how these meta-cognitive thoughts trigger responses on the part of the learner in terms of adapting behavior. Choosing subsequent learning activities or indicating what level of complexity one could feel comfortable with are examples of the latter.
Tip 8
Moving to higher complexity requires appropriate instructional support
As for fidelity, we must keep students’ zone of proximal development in mind. To avoid overload, starting at or moving to a higher level of fidelity should be accompanied by sufficient instructional support.
Instructional support
The use of worked examples, completion tasks, non-specific goals (e.g. “find as many etiological explanations for these symptoms as you can”), and presentations that avoid learners having to split their attention between multiple sources or having to process information through a suboptimal modality (e.g. having to process information verbally that actually ought to be presented verbally) constitute well-known tools to reduce redundant cognitive load among novice learners (Sweller et al. Citation1998; Van Merriënboer & Sweller Citation2010). These tools can be applied at any level of fidelity and at any level of complexity within a given fidelity level. For instance, examples of a process of coming to a particular type of diagnosis in a textbook and examples of such a process in a simulated or real-patient environment are different ways to put the worked example tool to practice, and one can apply this tool independent of the relative complexity of cases.
Tip 9
Determine the number of support levels
As for fidelity and complexity, choices with regard to the extent of variation in support depend on students’ prior knowledge, the competency under consideration, choices with regard to competency end terms, and logistic factors. It is of crucial importance to consider which tools are needed to what extent for which complexity and which fidelity levels to avoid increases in redundant cognitive load. That is, tools aimed at reducing redundant cognitive load among novices tend to contribute to redundant cognitive load among learners who are able to find their way without that guidance (Kalyuga et al. Citation2001, Citation2003). The distinction between worked examples and autonomous performance is important for that reason, and completion tasks may make the transition towards the latter more gradual. For instance, during a surgery, one could have a trainee observe a surgical operation and perform only a specific part of it (Van Merriënboer & Sweller Citation2010).
Tip 10
Gradually decrease support
From the robust finding that the need for instructional support on a type of learning task of a given complexity decreases as learners advance (Kalyuga et al. Citation2001, Citation2003), it follows that one should start with relatively high support and gradually fade that support for that type of learning task with time. Consider the clinical skill of taking a blood pressure. When learning how to do this for the first time, provide students with step-by-step instructions and feedback, then only provide them with feedback, and finally provide no feedback at all. This principle of gradually fading support is to be repeated at subsequent complexity and fidelity levels.
Tip 11
Enable practice at different levels of support
One way to support monitoring and control on the part of the learner (i.e. self-regulated learning) is through well-timed formative assessments, and this process may involve considering alternatives to commonly encountered assessment methods. For example, hypothesis-driven physical exams (Yudkowski et al. 2009) may be slightly more demanding during study but may also be more beneficial to learning and closer to professional practice than typical objective structured clinical examinations (OSCEs) (Lafleur et al. Citation2015). When organized carefully, formative assessments can serve multiple purposes. Firstly, they may stimulate self-regulated learning. Secondly, they may provide teachers with information on how to adjust learning materials or strategies employed – such as: fading support more slowly or more quickly or a slower or faster increase in complexity or fidelity – in a particular course or training. Finally, formative assessments can serve as anchors with regard to students’ advancement on the journey towards reaching end terms. Formative tests can pertain but are not limited to autonomous task performance; self-explanation of worked examples or for instance performing only a specific part of a surgery (Van Merriënboer & Sweller, Citation2010) can provide excellent moments for formative assessment.
Tip 12
Keep track of cognitive load
Apart from qualitative and quantitative indicators of performance in formative assessments, teachers and curriculum developers should consider keeping track of cognitive load experienced by their students along the way (Leppink & Van den Heuvel Citation2015; Leppink et al. Citation2015). The question of how to measure cognitive load has however proven difficult for researchers. Some recent studies have provided psychometric measures of different types of cognitive load (Leppink et al. Citation2013, Citation2014). Further, the Paas Cognitive Load Scale is a single-item measure whereby learners are asked to rate the perceived intensity of their mental effort at a given task or activity along a 9-point scale (1 = very, very-low mental effort; 9 = very, very-high mental effort) (Paas Citation1992). Another frequently used instrument is the NASA Task Load index, which uses a visual analogue scale to indicate the perceived level on six subscales: mental demand; physical demand, temporal demand; performance; effort; and frustration (Hart & Staveland Citation1988). When timed carefully, these measures can help teachers and curriculum developers to understand to what extent specific choices made on the dimensions of the cube may stimulate or hamper learning on the part of individual learners. This may result in specific adjustments in terms of personalized learning trajectories, the design of specific coursework or training or perhaps adjustments in the end terms or assessment thereof. Simultaneously, questions on cognitive load may help students to reflect on their learning experiences and stimulate self-regulated learning.
Conclusions
One can use cognitive load theory to integrate any competency into a medical curriculum such that training in that competency facilitates the students’ journey that starts from high instructional support on low-complexity low-fidelity learning tasks all the way to high-complexity tasks in high-fidelity environments carried out autonomously. The three sets of four tips presented in this article on how to achieve this aim can be summarized in the following three bullet points:
Task fidelity: to avoid cognitive and/or emotional overload, we move up from literature through (different types of) simulated patients to real patients; starting at or moving to a higher level of fidelity should be accompanied by sufficient instructional support.
Task complexity: to avoid cognitive overload, we should gradually increase learning task complexity and provide sufficient instructional support when moving to more complex tasks.
Instructional support: for a given fidelity and complexity, instructional support should be faded gradually from worked examples through completion tasks to tasks performed autonomously.
Notes on contributors
JIMMIE LEPPINK, MSc, MSc, LLM, PhD, is currently a postdoctoral researcher, consultant for quantitative methodology and analysis, and data manager for the School of Health Professions Education, Maastricht University, the Netherlands. His research focuses on self-regulated learning, computerized adaptive testing, cognitive load theory and measurement, and multilevel analysis of educational data.
ROBBERT DUVIVIER, MD, PhD, is currently a Senior Lecturer in medicine for the School of Medicine and Public Health, University of Newcastle, Australia. His research interests focus on skills training in the medical domain.
Declaration of interest: The authors report no conflicts of interest. The authors alone are responsible for the content and writing of the article.
This work was funded by the Netherlands Organisation for Scientific Research (NWO) Programme Council for Educational Research (PROO) Scaffolding self-regulation: effects on the acquisition of domain-specific skills and self-regulated learning skills (grant number: 411-12-015).
References
- Fraser K, Huffman J, Ma I, Sobczak M, McIlwrick J, Wright B, McLaughlin K. 2014. The emotional and cognitive impact of unexpected simulated patient death: A randomized controlled trial. Chest 145:958–963
- Fraser K, Ma I, Teteris E, Baxter H, Wright B, McLaughlin K. 2012. Emotion, cognitive load and learning outcomes of simulation training. Med Educ 46:1055–1062
- Hart SG, Staveland LE. 1988. Development of NASA-TLX (Task Load Index): results of empirical and theoretical research. In: Hancock PA Meshtaki N, editors. Human mental workload. Amsterdam: North-Holland. pp 139–183
- Irby DM, Cooke M, O’Brien BC. 2010. Calls for reform of medical education by the Carnegie foundation for the advancement of teaching: 1910 and 2010. Acad Med 85:220–227
- Kahneman D. 2011. Thinking, fast and slow. New York: Farrar, Straus and Giroux
- Kalyuga S, Ayres P, Chandler P, Sweller J. 2003. The expertise reversal effect. Educ Psychol 38:23–31
- Kalyuga S, Chandler P, Tuovinen J, Sweller J. 2001. When problem-solving is superior to studying worked examples. J Educ Psychol 93:579–588
- Kalyuga S, Hanham J. 2011. Instructing in generalized knowledge structures to develop flexible problem solving skills. CHB 27:63–68
- Lafleur A, Côté L, Leppink J. 2015. Influences of OSCE design on students’ diagnostic reasoning. Med Educ 49:203–214
- Leppink J, Paas F, Van der Vleuten CPM, Van Gog T, Van Merriënboer JJG. 2013. Development of an instrument for measuring different types of cognitive load. Behav Res Methods 45:1058–1072
- Leppink J, Paas F, Van Gog T, Van der Vleuten CPM, Van Merriënboer JJ. G. 2014. Effects of pairs of problems and examples on task performance and different types of cognitive load. Learning and Instruction 30:32–42
- Leppink J, Van den Heuvel A. 2015. The evolution of cognitive load theory and its application to medical education. Perspect Med Educ 4:119–127
- Leppink J, Van Gog T, Paas F, Sweller J. 2015. Cognitive load theory: Researching and planning teaching to maximize learningIn: Cleland J. Durning SJeditors. Researching Medical education, currently in press, http://www.bookdepository.com/Researching-Medical-Education-Jennifer-Cleland/9781118839201
- Nelson T, Narens L. 1990. Metamemory: A theoretical framework and new findings. In: Bower G, editor. The psychology of learning and motivation. New York: Academic Press. pp 125–173
- Paas F. 1992. Training strategies for attaining transfer of problem-solving skills in statistics: A cognitive load approach. J Educ Psychol 84:429–434
- Schuwirth L, Van der Vleuten CPM. 2011. Programmatic assessment: From assessment of learning to assessment for learning. Med Teach 33:478–485
- Sweller J, Van Merriënboer JJG, Paas F. 1998. Cognitive architecture and instructional design. Educ Psychol Rev 10:251–296
- Van Merriënboer JJG, Sweller J. 2010. Cognitive load theory in health professional education: Design principles and strategies. Med Educ 44:85–93
- Vygotsky LS. 1978. Mind in society: Development of higher psychological processes. Cambridge, MA: Harvard University Press
- Young JQ, Sewell JL. Applying cognitive load theory to medical education: Construct and measurement challenges. Perspect Med Educ 4:107–109
- Young JQ, Van Merriënboer JJG, Durning S, Ten Cate O. 2014. Cognitive load theory: Implications for medical education. AMEE Guide No. 86. Med Teach 36:371–384
- Young MS, Stanton NA. 2002. Attention and automation: New perspectives on mental underload and performance. Theor Issues Ergon Sci 3:178–94
- Yudkowsky R, Otaki J, Lowenstein T, Riddle J, Nishigori H, Bordage G. 2009. A hypothesis-driven physical examination learning and assessment procedure for medical students: Initial validity evidence. Med Edu 43:729–740
- Zimmerman B, Schunk D. 2001. Self-regulated learning and academic achievement: Theoretical perspectives. 2nd ed. Mahwah: Erlbaum