Abstract
Background. Accurate target volume segmentation is crucial for success in image-guided radiotherapy. However, variability in anatomical segmentation is one of the most significant contributors to uncertainty in radiotherapy treatment planning. This is especially true for lung cancer where target volumes are subject to varying magnitudes of respiratory motion.
Material and methods. This study aims to analyze multiple observer target volume segmentations and subsequent intensity-modulated radiotherapy (IMRT) treatment plans defined by those segmentations against a reference standard for lung cancer patients imaged with four-dimensional computed tomography (4D-CT). Target volume segmentations of 10 patients were performed manually by six physicians, allowing for the calculation of ground truth estimate segmentations via the simultaneous truth and performance level estimation (STAPLE) algorithm. Segmentation variability was assessed in terms of distance- and volume-based metrics. Treatment plans defined by these segmentations were then subject to dosimetric evaluation consisting of both physical and radiobiological analysis of optimized 3D dose distributions.
Results. Significant differences were noticed amongst observers in comparison to STAPLE segmentations and this variability directly extended into the treatment planning stages in the context of all dosimetric parameters used in this study. Mean primary tumor control probability (TCP) ranged from (22.6 ± 11.9)% to (33.7 ± 0.6)%, with standard deviation ranging from 0.5% to 11.9%. However, mean normal tissue complication probabilities (NTCP) based on treatment plans for each physician-derived target volume well as the NTCP derived from STAPLE-based treatment plans demonstrated no discernible trends and variability appeared to be patient-specific. This type of variability demonstrated the large-scale impact that target volume segmentation uncertainty can play in IMRT treatment planning.
Conclusions. Significant target volume segmentation and dosimetric variability exists in IMRT treatment planning amongst experts in the presence of a reference standard for 4D-CT-based lung cancer radiotherapy. Future work is needed to mitigate this uncertainty and ensure highly accurate and effective radiotherapy for lung cancer patients.
Accurate target volume definition is of paramount importance in radiation treatment planning for non-small cell lung cancer (NSCLC). Thoracic tumors pose a challenge for this as they are characteristically subject to varying magnitudes of motion due to respiration [Citation1]. Lung tumors have been shown to move up to 5 cm with free breathing [Citation2] and the magnitude of this motion is variable and unpredictable [Citation3]. For image-guided radiotherapy (IGRT), three-dimensional computed tomography (3D-CT) imaging lacks accurate information on tumor motion, providing images with significant artifacts that occur due to changes in tumor shape, volume, and position due to respiratory motion [Citation4]. Manual GTV segmentation variability has previously been demonstrated for lung cancer in 3D image acquisition [Citation5]. Ancillary measures, such as co-registration with functional/metabolic imaging and/or automatic segmentation strategies, have been shown potential to reduce inter- and intra-segmentation variability [Citation6–8].
Due to this uncertainty, intensity-modulated radiation therapy (IMRT) plans based on conventional 3D-CT images are subject to increased target volume margin sizes to account for largely misinterpreted tumor locations [Citation3]. Using arbitrarily large volumes does not address the inherent variability of tumor motion on an inter-patient basis and variation in tumor geometry on both an inter- and intra-patient basis and limits local control during radiotherapy. 4D-CT imaging allows for the acquisition of information about changes in tumor shape, volume, and location associated with respiratory motion in CT image datasets. The basic method for target volume segmentation in 4D-CT consists of delineating the gross tumor volume (GTV) on each respiratory phase based CT dataset (typically 8–12 phases) with a subsequent internal gross tumor volume (IGTV) created by combining multiple GTV segmentation into one enveloped volume [Citation9]. The GTV to clinical target volume (CTV) margin expansion of typical 3D-CT-based radiotherapy (8 mm for both adenocarcinoma and squamous cell carcinoma at LRCP) remains the same. However, it is applied to the IGTV to create the internal target volume (ITV) that accounts for both microscopic disease and internal motion. Although methods currently exist to expedite the segmentation of the IGTV in 4D-CT datasets [Citation7,Citation8,Citation10], the most reliable method for 4D-CT target volume segmentation is still time-consuming manual segmentation of the GTV for all respiratory phases and subsequent margin expansions for the ITV and planning target volume (PTV) [Citation9]. For treatment planning purposes, the PTV requires a margin expansion of 5–10 mm upon the ITV to account for setup uncertainty.
Geometric uncertainties due to target volume segmentation have recently been explored in the joint context of 4D-CT imaging and lung cancer [Citation11]. These studies have shown improvements over conventional 3D-CT for mitigating uncertainty. Quantifying variability and accuracy at the treatment planning stage is equally important in measuring the dosimetric variability due to segmentation-related geometric uncertainties. If the variability of anatomical segmentation exceeds the margin sizes applied to target volumes, the potential for geographic miss of the tumor volume and/or irradiation of normal tissues and organs at risk (OARs) can be expected to increase. Conversely, if current clinical margins are overly conservative in addressing geometric uncertainties, increased normal tissue irradiation can mitigate the effects of targeted radiotherapy and impact patient outcomes negatively due to radiation-induced lung injury (RILI) and/or secondary malignancies. As very little literature exists on the dosimetric impact of target volume segmentation variability in 4D-CT-based NSCLC radiotherapy, this work aims to examine the impact of tumor volume segmentation accuracy and variability for IMRT treatment planning for NSCLC in terms of segmentation accuracy and quality when compared to an estimate of the ground truth.
Material and methods
Image acquisition and reconstruction
4D-CT imaging was performed on 10 patients with NSCLC, with patient demographics and disease information presented in Supplementary Table I (to be found online at http://informahealthcare.com/doi/abs/10.3109/0284186X.2014.970666). Local Research Ethics Board (REB) approval was obtained and all data was anonymized prior to segmentation. A Philips 16-slice Brilliance Big Bore CT scanner (Philips Medical Systems, Cleveland, OH, USA) was used. The Real-Time Position Management (RPM) respiratory gating system (Varian Medical Systems, Palo Alto, CA, USA) was used as a respiratory surrogate. The RPM system uses an infrared camera that follows reflective markers placed on the patient's chest or abdomen. For all 10 patients, a long spiral CT scan with pitch < 0.1 was performed to cover the entire thorax. Pulmonary signal data was collected from the RPM system simultaneously with CT data. CT data was then reconstructed at 10 different respiratory phases (i). Respiratory phases were tagged as percentage of full inspiration, indicating temporal steps from one full inspiration phase to another (i = 0, 10,..., 90%). This form of image reconstruction allows for visualization of tumor volume displacement at 10 equidistant points in time throughout the respiratory cycle.
Manual segmentation
The GTV was segmented on each of the 10 respiratory phases for 10 patients by six radiation oncologists at the London Regional Cancer Program (LRCP) with clinical experience ranging from 1 to 25 years. Manual segmentation was performed based on radiological reports and difficulty was ranked from 1 (least difficult) to 5 (most difficult) for each case by each observer (Supplementary Table I to be found online at http://informahealthcare.com/doi/abs/10.3109/0284186X.2014.970666). Target volume segmentation was performed using default visualization parameters for lung tumor segmentation by all physicians in accordance with recommendations by Giraud et al. [Citation5] (−600/1600 HU for lung window, + 20/400 HU for the mediastinal window). GTV segmentation and creation of the IGTV envelope, defined as the union of GTV segmentation from all respiratory phases was performed using MiMVista v.5.2 (MiM Software, Cleveland, OH, USA). Nodal volumes were also present for eight out of 10 patients and were segmented by physicians similarly to the GTV to allow for construction of a Nodal ITV, Nodal PTV and a Total PTV [Primary + Node(s)] at the treatment planning stage. Experts were blinded to one another's segmentations and were provided with a representative axial 2D-CT image indicating location of the primary GTV and specific node(s). Manual GTV segmentations from all respiratory phases were fused to create an IGTV upon which clinical margin expansions were applied. OARs were segmented by a single observer in accordance with the 1993 ICRU Report #62 (http://www.icru.org/home/reports/prescribing-recording-and-reporting-photon-beam-therapy-report-62) to allow for a standardized interpretation amongst observers.
Reference segmentations
Manual segmentations of the six participating physicians were incorporated into multi-expert ground truth estimates via the simultaneous truth and performance level estimation (STAPLE) algorithm to create reference standard segmentations in this study [Citation12]. STAPLE calculations were made possible with software provided by Harvard's Computational Radiology Laboratory (CRL) and a user interface built in C# (Microsoft. Redmond, Washington). This method allows for comparison of both target volume segmentations and corresponding treatment plan dose distributions to a gold standard with equal bias assumed towards each observer. Reference target volumes were constructed with the same clinical margins applied as manual segmentations.
IMRT treatment planning
Segmentations were transferred to the Pinnacle™ (Philips Radiation Oncology Systems; Milpitas, USA) treatment planning system (TPS) 9.1 Beta for IMRT treatment planning and dose distribution calculation. Treatment planning was fully automated using in-house scripts written in the Pinnacle™ scripting language. To eliminate any planning bias, no plans were subject to any manual fine-tuning post optimization. The collapsed cone convolution algorithm was selected in Pinnacle software to compute the 3D dose matrix with 4 mm grid spacing. Treatment planning DVH objectives were chosen to comply with RTOG criteria (Trial 0617) [Citation13] (http://www.rtog.org/clinicaltrials/protocoltable/studydetails.aspx?study = 0617) with a fixed 5-beam arrangement at 6 and 10 MV energies. Only a single energy was implemented in automated planning for individual patients across all observers and the STAPLE plan. Gantry angles were determined on a patient-specific basis in efforts to achieve plans compliant with RTOG 0617. The target prescription dose was 60 Gy in 30 fractions normalized to 100% at the target's centroid. Target coverage was assessed using D95 [the dose to 95% of the PTV volumes (PTV-Primary, PTV-Nodes, and PTV-Total)] during treatment planning to ensure adequate target coverage. While RTOG 0617 calls for assessment of target volume coverage in terms of the PTV-Total, this constraint was applied individually to both PTV-Primary and PTV-Nodes as well to ensure all targets were sufficiently covered during dose calculation and optimization.
Segmentation comparison
Manually derived, physician segmentations were compared to the STAPLE segmentations for Primary and Nodal PTVs for all 10 patients. All segmentations were reconstructed using a novel global optimization framework in C++ for 3D shape reconstruction proposed by Lempitsky and Boykov which ensured sets of closed, minimal surfaces were generated for comparison [Citation14]. This software also allowed for volume-based segmentation comparisons, given by volume overlap error (VOE). Distance-based measurements, given by the root mean square (RMS) symmetric surface distance, were calculated using in-house software built in MATLAB (MathWorks, Natick, MA, USA). The VOE was chosen in accordance with studies performed by Heimann et al. [Citation15] and Fotina et al. [Citation16] who observed both the popularity of the metrics and its superiority compared to other volume-based metrics in the context of segmentation analysis, respectively. As stated by Heimann et al., the RMS symmetric surface distance metric is one of the most important in evaluating segmentation accuracy [Citation15]. The extent of variability for segmentation comparison is demonstrated by standard deviations (SD) and the coefficients of variance (COV) for each metric across the observer group, where the COV is given in percent and defined as the SD divided by the mean, multiplied by one hundred.
Treatment plan comparison
Treatment plans were optimized for all observer-based target volume segmentations and STAPLE segmentations for primary and nodal (if present) targets. Observer-based treatment plan dose distributions were then analyzed in the context of the STAPLE segmentation, i.e. observer-based dose distributions overlaid on STAPLE segmentations. This was done to assess variable dosimetry inherent to each physician's target volume segmentation against optimal dosimetry calculations based on those of the GT estimate. Several measures were used to assess the quality of treatment plan dose distributions. Metrics representative of both physical and radiobiological dosimetric characteristics were used to quantitatively evaluate the variability of target volume segmentation for NSCLC radiotherapy.
In assessing the physical characteristics of optimized dose distributions, dose homogeneity/ heterogeneity calculations throughout the target volume were performed. Homogeneity index (HI) was evaluated as the difference between the maximum and minimum dose to the target volume (D2% and D98%, respectively) divided by the prescription dose [Citation17]. Uniformity index (UI) was also used and defined as the ratio of D5% to D95% [Citation18]. A value of zero for HI and unity for UI indicates optimal dose homogeneity.
Radiobiological parameters for assessment in this study included the primary tumor control probability (TCP) and the normal tissue complication probability (NTCP) for the healthy lung (defined as lung minus CTV-primary per RTOG 0617). For TCP calculations, a D50 of 70 Gy, a γ of 2.0 were assumed and an (α/β) of 10. TCP calculation for this study did not take into account dose inhomogeneties within the target sub-volume. Parameters for TCP calculations were chosen in accordance with the study performed by van Baardwijk et al. [Citation19]. For NTCP calculations, the Lyman-Kutcher-Burman (LKB) model was used where the estimated volume parameter was n = 0.41 as per the study by Tucker et al. who showed it better predicted RILI risk compared to the MLD (n = 1) [Citation20]. If the Deff [or equivalent uniform dose (EUD)] is distributed uniformly throughout the entire volume, it will yield an equivalent NTCP as the actual non-uniform dose distribution. For NTCP calculations, additional parameters of m = 0.31, and a threshold dose of 43.20 Gy (TD50 = 43.20 Gy) were used, again in accordance with the study by Tucker et al. [Citation20]. Radiobiology calculations were performed using in-house software built in C++ (Microsoft, Redmond, WA, USA) with a wrapper built in C# (Microsoft).
As previously stated, GTVs were manually segmented on 4D-CT data sets by six different radiation oncologists. However, PTV segmentations (IGTV + 1.5cm) were compared and planned upon to provide insights into accuracy of treatment planning target volumes and subsequent dosimetric variability. Appropriate margin expansions were applied to IGTVs to construct PTVs as treatment planning in the clinical setting routinely assigns 95% of prescription does to the PTV during treatment plan optimization. In doing this, dosimetric characteristics based on multiple target volume segmentations are more appropriately analyzed in the context of the dose to the PTV. As such, different segmentations and were compared to STAPLE-derived segmentations with the precisely the same margins to avoid any systematic errors in segmentation analysis and treatment plan comparison.
Results
Segmentation variability
All manual segmentation results were compared to their respective STAPLE GT estimate segmentations in evaluating segmentation accuracy and quality. shows mean observer VOE for primary and nodal IGTV volumes and their respective SDs. For primary IGTVs, mean observer VOE ranged from (9.0 ± 0.6)% to (35.6 ± 7.5)%, with SD ranging from 0.6% to 13.2%. Nodal IGTV demonstrated mean observer VOE ranging from (16.4 ± 1.5)% to (29.1 ± 4.1)% with SD ranging from 1.5% to 13.1%. shows mean observer symmetric RMS distances for primary and nodal IGTV segmentations compared to STAPLE segmentations with respective SD for all patients. For primary IGTVs, mean observer RMS symmetric distances ranged from (3.4 ± 0.4)mm to (7.8 ± 1.8)mm, with SD ranging from 0.2 mm to 1.2 mm. Nodal IGTVs demonstrated mean observer RMS symmetric distances ranging from (4.1 ± 0.8)mm to (6.5 ± 0.7)mm with SD ranging from 0.2 mm to 3.2mm.
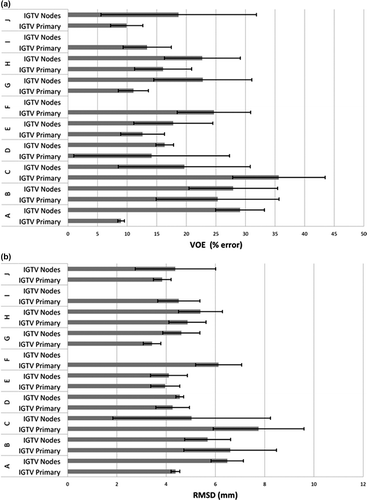
and show maximum and minimum measures as well as coefficients of variation for both VOE and RMS symmetric distances, respectively. Looking at the COV across both VOE and RMS symmetric distances, nodal IGTV segmentation was typically subject to larger errors and variance, however, the variability was still quite high for both target volumes across the observer group and fluctuating COVs indicates the presence of outlier segmentations for each patient.
Table I. Maximum, minimum and COV measures for VOE.
Table II. Maximum, minimum and COV measures for symmetric RMS distances.
Dosimetric variability
demonstrates the subsequent effect of variable target volume segmentation on treatment planning for a moderately variable case (Patient F).
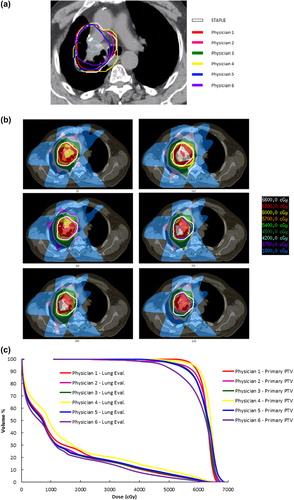
The D95 for primary and nodal PTVs is shown in . Primary IGTV segmentations across the observer segmentation groups yielded only four cases in which clinically acceptable target volume coverage of the PTV was achieved (D95 = 60 Gy) (Patients A, E, G, J) (). Nodal IGTV segmentations across this group yielded only one case in which mean observer segmentations provided for clinically acceptable target coverage (Patient J).
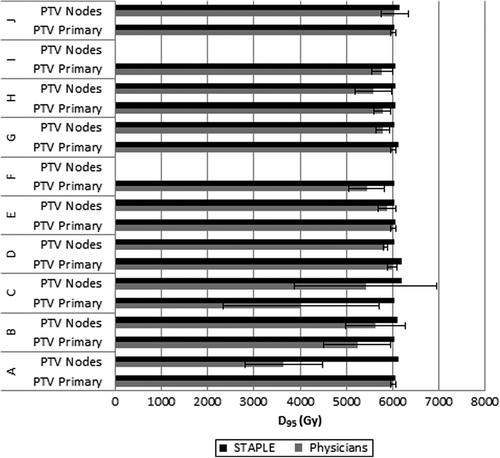
Supplementary Figure 1(a) (to be found online at http://informahealthcare.com/doi/abs/10.3109/0284186X.2014.970666) shows mean uniformity indices (UI) for primary and nodal IGTVs compared to STAPLE segmentation-based UI. For primary IGTVs, mean observer UI ranged from 1.1 ± 0.10 to 1.9 ± 0.9, with SD ranging from 0.01 to 0.9. Nodal IGTV demonstrated mean observer UI ranging from 1.1 ± 0.1 to 1.9 ± 0.8 with SD ranging from 0.1 to 0.8. Dose uniformity was consistently worse amongst observers compared to the STAPLE-derived target volume dose distributions. Nodal IGTV segmentation typically provided for slightly increased UI and associated variance, indicative of higher segmentation uncertainty being propagated into the treatment planning stage. Supplementary Figure 1(b) to be found online at http://informahealthcare.com/doi/abs/10.3109/0284186X.2014.970666 shows mean homogeneity indices (HI) for primary and nodal IGTVs compared to STAPLE segmentation-based HI. For primary IGTVs, mean observer HI was routinely higher than it was for the STAPLE-based target, ranging from (11.4 ± 2.4)% to (61.1 ± 26.5)%, with SD ranging from 2.1% to 26.5%. Nodal IGTV demonstrated similarly higher mean observer HI ranging from (13.4 ± 9.2)% to (75.2 ± 4.7)% with SD ranging from 2.0% to 27.6%. Dose homogeneity reflects a similar trend demonstrated in UI measurements with greater sensitivity and magnitude of deviation. Nodal IGTV segmentations provided for increased variability and uncertainty in optimized dose distributions compared to Primary IGTVs. However, the magnitude of variation varied between physicians on an inter-patient basis, most noticeably for Patients B (extreme variance) and E (minimal variance). Variability was largely inconsistent for both metrics. However, trends demonstrated for segmentation-based analysis were reflected in subsequent dosimetric analysis for corresponding patients indicating that target volume segmentation variability influences dosimetric uncertainty and fluctuates on both an inter-physician and an inter-patient basis.
shows mean TCP based on treatment plans for each physician's primary IGTV segmentation as well as the TCP derived from STAPLE-based treatment plans. Mean observer-based TCPs were again subject to a wide range of variability and were typically lower than that of the STAPLE-based TCP for both scenarios. For primary IGTVs, mean observer TCPs ranged from (22.6 ± 11.9)% to (33.7 ± 0.6)%, with SD ranging from 0.5% to 11.9%. In certain cases, single observer segmentation was close to or exceeded the TCP of the STAPLE- derived target volume. For these cases, this effect was due to the fact that the observer segmentation was smaller in volume than the STAPLE volume and was largely contained within it. However, the mean TCP derived from the observer group never exceeded that of the STAPLE target segmentation itself.
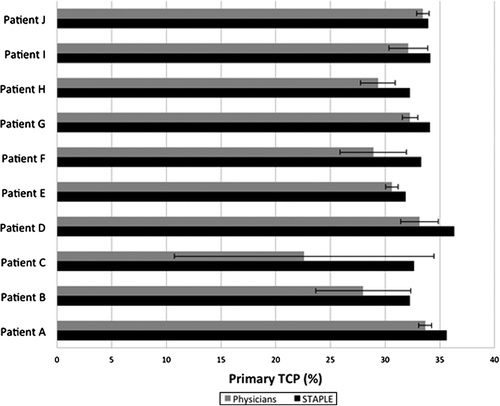
All treatment plans were able to meet RTOG 0617 standards for all critical structures (spinal cord, esophagus, heart, healthy lung). OAR analysis focused on the total lung volume as it is directly related to target volume segmentation variability unlike the other critical structures present during treatment planning. Total lung was assessed based on the dose to the total lung minus CTVSTAPLE with the total lung minus CTVObserver overlaid for each physician's respective treatment plan dose distributions per RTOG 0617 lung-eval criteria. Supplementary Table II (to be found online at http://informahealthcare.com/doi/abs/10.3109/0284186X.2014.970666) shows mean total lung dosimetric measures across the physician group (± SD)compared to those derived from the STAPLE-based dose distribution. Very little variation is present on an intra-patient basis, with inter-patient fluctuation occurring most likely as a result of varying IGTV size. shows mean NTCP based on treatment plans for each physician-derived target volume well as the NTCP derived from STAPLE-based treatment plans. No discernible trends are present and variability appears to be patient specific.
Discussion
Multiple studies on tumor volume segmentation uncertainty and variability have been performed previously. Studies performed in the context of both 3D- and 4D-CT reported the effects of target volume segmentation for lung tumors with results demonstrating different ranges of uncertainty and/or variability. The most commonly studied influences on segmentation-related geometric uncertainties are the effects of inter-/intra-observer variability [Citation21], the relationship between patients breathing patterns and image artifact presence [Citation22], and tumor artifacts inherent to the 4D-CT acquisition modality and/or reconstruction technique [Citation23]. While numerous suggestions have been made in efforts to identify, mitigate and/or rectify uncertainty in the presence of these sources of error, very little information exists regarding the dosimetric and/or radiobiological impact of tumor volume segmentation-related geometric uncertainty. Studies by Spoelstra et al. [Citation24] and Le Maitre et al. [Citation25] analyzed the effects of tumor volume delineation on subsequent radiotherapy planning for lung cancer. Spoelstra et al. demonstrated significant inter-clinician variability in lung tumor delineation leading to confounding variability in clinical outcomes and emphasized a need for standardization of target volume segmentation. They concluded that uncertainty in anatomical delineation was the largest source of systematic error in image-guided lung cancer radiotherapy. This idea was extrapolated upon and reinforced by Jameson et al. who made similar conclusions in their review of segmentation analysis strategies in radiation oncology [Citation26]. Le Maitre et al. examined the dosimetric effect of PET-based functional volume auto-segmentation variability and demonstrated a reduction in dosimetric errors using more advanced segmentation strategies [Citation25]. However, this study focused on functional volume derived from static PET-CT images and lacked the influence of respiratory motion on target volume segmentation, which does not reflect current clinical practice. More recently, Jameson et al. conducted a study in which they showed a strong correlation between geometric uncertainties and TCP in the context of lung tumor segmentation [Citation27]. Our study attempts to build on these works in reporting on the effects of GTV/IGTV segmentation accuracy and variability on IMRT treatment planning for lung tumors in the context of 4D-CT imaging and respiratory motion. The results of this study suggest that a wide range of variability within 4D-CT-derived tumor volume segmentations extends into the treatment planning stages of IMRT and affects both physical and radiobiological characteristics of the calculated dose distributions. The largest variability resulted from comparison of primary and nodal target volume dose distributions as well as inter-patient comparison. The dosimetric uncertainty that arises due to variable primary and nodal IGTV segmentations is most likely attributed the vastly different geometry between these structures. Nodal targets were routinely smaller than primary target volumes and accordingly, geometric differences of the same magnitude have different relative effects on both segmentation accuracy and dosimetric variability. Nodal volumes can be quite difficult to identify within 3D image volumes without contrast enhancement as they typically reside in areas of low contrast and defining normal versus abnormal lymph nodes can be a challenge without functional and/or metabolic imaging, such as positron emission tomography (PET)-CT [Citation28] and dynamic contrast-enhanced (DCE) magnetic resonance imaging (MRI) [Citation29]. This issue is challenging to address in the context of segmentation variability, as it is subject to increased inter-/intra-observer variance as well. Previous studies indicated that PET-CT could be valuable in helping to more accurately identify abnormal nodes in lung cancer patients [Citation6,Citation28]. Subsequently, advanced imaging strategies incorporating functional information and/or metabolic information could possibly incur advantages in reducing dosimetric variability at the treatment planning stage for nodal target volumes. Inter-patient dosimetric uncertainty is considerably more difficult to mitigate.
Lung tumor geometry is inherently patient specific and highly variable factors contribute to varying levels of segmentation difficulty for each incoming case viewed by physicians in clinic. For this study, a wide range of patients were selected to quantify the dosimetric impact of target volume segmentation accuracy and variability on treatment planning for lung cancer patients. However, this presents as a limitation in this study. Analysis showed a correlation between dosimetric variability and patient-specific characteristics such as tumor volume, location, and segmentation difficulty as scored by observers. Therefore, further sub-classification based on patient-specific characteristics prior to analysis would be beneficial for future studies focused on quantifying segmentation-related variability in the treatment planning context. Another limitation in this study is the small number of patients and observers. While only 10 patients and six observers were utilized, this provided for approximately 600 manually defined primary and nodal IGTV segmentations, yielding a considerable amount of data for this and future studies. It is important to note that considerably more information can be acquired and studied in the context of 4D-CT compared to other conventional image acquisition techniques with a relatively low number of patients and observers when needed. Such observations may prove valuable in future work with sub- classified groups where limited patient data is available. Additionally, the inclusion of surgical specimens and/or tumor pathology would help to verify the accuracy of the STAPLE algorithm. Currently, STAPLE only gives us a ground truth estimate based solely on image information. Further verification of the algorithm in the context of lung tumors analogous to the work of Gordon et al. [Citation30] would validate studies such as ours working towards the reduction of segmentation-related geometric uncertainties.
One of the most common solutions presented for mitigating geometric uncertainties in target volume segmentation is automatic and/or semi-automatic segmentation. These strategies rely on the notion that by reducing the amount of manual target volume segmentation, inter- and intra-observer variance can be greatly reduced and the consistency and integrity of segmentation is increased without sacrificing accuracy. However, auto-segmentation strategies pose considerable difficulty in both development and implementation. Segmentation must be at least as accurate as manual strategies while also being computationally efficient. In cases where auto-segmentation is used for IGRT, automatic techniques must be validated and incorporated into the routine clinical framework. Automated segmentation techniques have been proposed to utilize different approaches [Citation8,Citation31]. These techniques generally provide a starting point for target delineation in the clinical setting, subject to review and edit by physicians. The possibility of amalgamating ground truth estimation techniques, such as STAPLE [Citation14], that are based on multiple manual observer segmentations with auto-segmentation techniques utilizing a priori information could complement existing strategies in reducing inter- and intra-observer variability. This type of strategy could be augmented by some form of colleague peer-review [Citation32] but would entail an exceptionally complex clinical segmentation framework for efficient utilization. Another possible solution to address this problem could be the initiation of multi-institution, multi-observer studies focused on determining clinical margins to compensate for uncertainties based on target volume definition. These margins could be determined for a variety of treatment techniques with varying degrees of conformity and different dose prescriptions, i.e. stereotactic body radiation therapy/stereotactic ablative radiation therapy (SBRT/SABR) and 3D-conformal radiation therapy (3D-CRT). Much like the margins that exist to account for microscopic disease, inter-fraction motion and/or setup error, clinical margins could be developed to account for geometric uncertainties arising in the process of target volume segmentation for all available treatment techniques. However, this would also necessitate large-scale segmentation studies consisting of multiple observers across multiple institutions as well as extensive validation to be fully accepted at the clinical level. Additionally, the inclusion of supplementary information such as that provided by hybrid and/or functional/metabolic imaging would require different sets of guidelines depending on the diagnostic information that is available and the disease pathology. While there is no immediate solution to this problem, there are a number of different tools and research possibilities to address it as diagnostic imaging and radiotherapy techniques evolve.
In conclusion, a considerable amount of variability currently exists in target volume segmentation for lung tumors in the context of 4D-CT imaging. Based on primary and nodal lung tumor target segmentations amongst multiple experts in the presence of a reference standard, this variability extends into the treatment planning stages for IMRT. Although the use of 4D-CT allows for more accurate target volume segmentation by accounting for respiratory motion, further improvements need to be made to provide for more consistent target volume segmentation.
Supplementary material available online
Supplementary Figure 1, Table I and II to be found online at http://informahealthcare.com/doi/abs/10.3109/0284186X.2014.970666
ionc_a_970666_sm9170.pdf
Download PDF (442.2 KB)Acknowledgements
The authors would like to thank Jeff Kempe for his help in software analysis and implementation. This work was funded by the Ontario Institute for Cancer Research (OICR) through funding provided by the government of Ontario and by the Canadian Institute of Health Research (CIHR), CIHR Strategic Training Initiative in Cancer Research.
Declaration of interest: The authors report no conflicts of interest. The authors alone are responsible for the content and writing of the paper.
References
- Plathow C, Ley S, Fink C, Puderbach M, Hosch W, Schmähl A, et al. Analysis of intrathoracic tumor mobility during whole breathing cycle by dynamic MRI. Int J Radiat Oncol 2004;59:952–9.
- Chen QS, Weinhous MS, Deibel FC, Ciezki JP, Macklis RM. Fluoroscopic study of tumor motion due to breathing: Facilitating precise radiation therapy for lung cancer patients. Med Phys 2001;28:1850–6.
- Keall PJ, Mageras GS, Balter JM, Emery RS, Forster KM, Jiang SB, et al. The management of respiratory motion in radiation oncology report of AAPM Task Group 76a). Med Phys 2006;33:3874–900.
- Ezhil M, Vedam S, Balter P, Choi B, Mirkovic D, Starkschall G. et al. Determination of patient-specific internal gross tumor volumes for lung cancer using four-dimensional computed tomography. Radiat Oncol 2009;4:1–14.
- Giraud P, Elles S, Helfre S, De Rycke Y, Servois V, Carette MF, et al. Conformal radiotherapy for lung cancer: Different delineation of the gross tumor volume (GTV) by radiologists and radiation oncologists. Radiother Oncol 2002;62:27–36.
- Geets X, Sterpin E, Wanet M, Di Perri D, Lee J. Metabolic imaging in non-small-cell lung cancer radiotherapy. Cancer/Radiothérapie Epub 2014 Aug 29.
- Kaus MR, Netsch T, Kabus S, Pekar V, McNutt T, Fischer B. Estimation of organ motion from 4D CT for 4D radiation therapy planning of lung cancer. Medical Image Computing and Computer-Assisted Intervention–MICCAI 2004. Springer: Berlin, Heidelberg; 2004. pp 1017–24.
- Gaede S, Olsthoorn J, Louie AV, Palma D, Yu E, Yaremko B, et al. An evaluation of an automated 4D-CT contour propagation tool to define an internal gross tumour volume for lung cancer radiotherapy. Radiother Oncol 2011;101:322–8.
- Ge H, Cai J, Kelsey CR, Yin FF. Quantification and minimization of uncertainties of internal target volume for stereotactic body radiation therapy of lung cancer. Int J Radiat Oncol 2013;85:438–43.
- Muirhead R, McNee SG, Featherstone C, Moore K, Muscat S. Use of maximum intensity projections (MIPs) for target outlining in 4DCT radiotherapy planning. J Thorac Oncol 2008;3:1433–8.
- Speight R, Sykes J, Lindsay R, Franks K, Thwaites D. The evaluation of a deformable image registration segmentation technique for semi-automating internal target volume (ITV) production from 4DCT images of lung stereotactic body radiotherapy (SBRT) patients. Radiother Oncol 2011;98:277–83.
- Warfield SK, Zou KH, Wells WM. Simultaneous truth and performance level estimation (STAPLE): An algorithm for the validation of image segmentation. IEEE Trans Med Imaging 2004;23:903–21.
- Bradley JD, Paulus R, Komaki R, Masters GA, Forster K, Schild SE. A randomized phase III comparison of standard-dose (60 Gy) versus high-dose (74 Gy) conformal chemoradiotherapy with or without cetuximab for stage III non-small cell lung cancer: Results on radiation dose in RTOG 0617. J Clin Oncol 2013;31:7501.
- Lempitsky V, Boykov Y. Global optimization for shape fitting. CVPR 2007;7:1–8.
- Heimann T, Van Ginneken B, Styner MA, Arzhaeva Y, Aurich V, Bauer C, et al. Comparison and evaluation of methods for liver segmentation from CT datasets. IEEE Trans Med Imaging 2009;28:1251–65.
- Fotina I, Lütgendorf-Caucig C, Stock M, Pötter R, Georg D. Critical discussion of evaluation parameters for inter- observer variability in target definition for radiation therapy. Strahlenther Onkol 2012;188:160–7.
- Whitelaw GL, Blasiak-Wal I, Cooke K, Usher C, Macdougall ND, Plowman PN. A dosimetric comparison between two intensity-modulated radiotherapy techniques: Tomotherapy vs dynamic linear accelerator. Br J Radiol 2008;81:333–40.
- Iori M, Cattaneo GM, Cagni E, Fiorino C, Borasi G, Riccardo C, et al. Dose-volume and biological-model based comparison between helical tomotherapy and (inverse-planned) IMAT for prostate tumours. Radiother Oncol 2008;88:34–45.
- van Baardwijk A, Wanders S, Boersma L, Borger J, Öllers M, Dingemans AMC, et al. Radiation dose prescription for non-small-cell lung cancer according to normal tissue dose constraints: An in silico clinical trial. Int J Radiat Oncol 2008;71:1103–10.
- Tucker SL, Mohan R, Liengsawangwong R, Martel MK, Liao Z. Predicting pneumonitis risk: A dosimetric alternative to mean lung dose. Int J Radiat Oncol 2013;85:522–7.
- Louie AV, Rodrigues G, Olsthoorn J, Palma D, Yu E, Yaremko B, et al. Inter-observer and intra-observer reliability for lung cancer target volume delineation in the 4D-CT era. Radiother Oncol 2010;95:166–71.
- Yamamoto T, Langner U, Loo Jr. BW, Shen J, Keall PJ, et al. Retrospective analysis of artifacts in four-dimensional CT images of 50 abdominal and thoracic radiotherapy patients. Int J Radiat Oncol 2008;72:1250–8.
- Ehrhardt J, Werner R, Säring D, Frenzel T, Lu W, Low D, et al. An optical flow based method for improved reconstruction of 4D CT data sets acquired during free breathing. Med Phys 2007;34:711–21.
- Spoelstra, FO, Senan S, Le Péchoux C, Ishikura S, Casas F, Ball D, et al. Variations in target volume definition for postoperative radiotherapy in stage III non-small-cell lung cancer: Analysis of an international contouring study. Int J Radiat Oncol 2010;76:1106–13.
- Le Maitre A, Hatt M, Pradier O, Cheze-le Rest C, Visvikis D. Impact of the accuracy of automatic tumour functional volume delineation on radiotherapy treatment planning. Phys Med Biol 2012;57:5381.
- Jameson MG, Holloway LC, Vial PJ, Vinod SK, Metcalfe PE. A review of methods of analysis in contouring studies for radiation oncology. J Med Imaging Radiat Oncol 2010; 54:401–10.
- Jameson MG, Kumar S, Vinod SK, Metcalfe PE, Holloway LC. Correlation of contouring variation with modeled outcome for conformal non-small cell lung cancer radiotherapy. Radiother Oncol Epub 2014 May 19.
- Kubota K, Murakami K, Inoue T, Itoh H, Saga T, Shiomi S, et al. Additional value of FDG-PET to contrast enhanced-computed tomography (CT) for the diagnosis of mediastinal lymph node metastasis in non-small cell lung cancer: A Japanese multicenter clinical study. Ann Nucl Med 2011;25:777–86.
- Khoo VS, Joon DL. New developments in MRI for target volume delineation in radiotherapy. Br J Radiol 2006;79:S2–15.
- Gordon S, Lotenberg S, Long R, Antani S, Jeronimo J, Greenspan H. Evaluation of uterine cervix segmentations using ground truth from multiple experts. Comput Med Imag Graph 2009;33:205–16.
- Gu Y, Kumar V, Hall LO, Goldgof DB, Li CY, Korn R, et al. Automated delineation of lung tumors from CT images using a single click ensemble segmentation approach. Pattern Recogn 2013;46:692–702.
- Rooney K, Hanna G, Harney J, Eakin R, Young VAL, Dunn M, et al. The impact of peer review on the radiotherapy treatment planning process in the treatment of lung cancer: Radiotherapy Peer Review. Lung Cancer 2014;83(Suppl 1):S58–9.