ABSTRACT
Background. A murine breast cancer xenograft model was employed to evaluate inter- and intra-variability of various parameters derived from dynamic positron emission tomography with [18F]-fluorodeoxyglucose as tracer (FDG-PET).
Material and methods. Seventeen female athymic nude foxn1/nu mice with bilaterally implanted triple-negative basal-like ductal carcinoma (MAS98.12) breast cancer xenografts underwent a dynamic PET scan over an hour after injection of approximately 10 MBq FDG. Inter-animal data were obtained from the entire animal cohort, while intra-animal data were from four mice receiving an additional scan after one or two days. Standardised uptake values (SUVmax, SUVmean and SUVmedian) were estimated for all tumours at different time points. Tumour uptake was analysed with a kinetic two-compartment model for estimation of pharmacokinetic parameters. The coefficient of variation (CV) was calculated for all PET-derived metrics.
Results. The CVs for SUVmean and SUVmedian were typically 10–20% for the tumours, depending on the time post-injection and group (intra vs. inter). The CV for SUVmax was mostly higher. The variability in the pharmacokinetic parameters ranged from 23 to almost 150%.
Conclusions. SUVmean and SUVmedian show less variability than SUVmax. The pharmacokinetic tumour metrics again display much greater variability than the SUV-based metrics. However, it is generally not known which of these metrics that best represents cancer aggressiveness and their use may still depend on the research questions addressed.
Positron emission tomography (PET) using 2-deoxy-2-[18F]-fluoro-D-glucose (FDG) as tracer may serve several purposes in oncology. First, it provides a means for detection of malignant lesions within the body, and may aid disease staging and subsequent treatment selection [Citation1]. Second, FDG-PET images allow for tumour delineation in radiotherapy [Citation2]. Third, FDG-PET may be used to monitor and evaluate treatment efficacy during and after therapy [Citation3]. Thus, FDG-PET may serve as a ‘compass for safe navigation’ in the era of personalised medicine [Citation4]. However, when using quantitative measures in the evaluation of treatment efficacy pre- and post-therapy, the measured change reflects a combination of the actual (biological) change and the inherent variability of the measurement. Therefore, a careful evaluation of FDG-PET as a test monitor is needed for both clinical and pre-clinical applications.
The tissue accumulation of the glucose analogue FDG reflects a combination of perfusion, transporter receptor (GLUT1-4) density and hexokinase activity [Citation5]. As many solid tumours employ glycolysis as their main energy source (Warburg effect), they are distinguishable from the background in conventional PET scans performed typically one hour after FDG injection. FDG uptake can be quantified in terms of the standardised uptake value (SUV), which is the amount of tracer accumulated in a region of interest relative to the injected specific activity per body weight [Citation6]. However, the SUV represents both the free (non-metabolised) and bound (metabolised) FDG, where only the latter reflects the Warburg effect. Furthermore, tissue accumulation is a continuous process, and conventional PET only provides a snapshot of this accumulation. Using dynamic PET, tissue accumulation can be monitored in both time and space, allowing for not only estimation of SUVs at specific time points post-injection, but also full pharmacokinetic modelling [Citation7]. The derived model parameters have been shown to reflect the underlying tissue processes leading to FDG uptake.
PET may be used for experimental testing of new therapies and assessment of treatment response [Citation7–9]. We have used dynamic FDG-PET and pharmacokinetic analysis for assessing response to irradiation in prostate cancer xenografts [Citation10], to imatinib therapy of gastrointestinal stromal xenografts [Citation11] and to anti-angiogenic therapy of breast cancer xenografts [Citation12]. In conjunction with such studies, it is timely to address the reproducibility of the parameters derived from dynamic PET used to assess therapy response. Of particular interest in this respect are the pharmacokinetic parameters, which derive from a statistical model analysis requiring an arterial input function [Citation7]. In the current work, we investigate the reproducibility of parameters derived from dynamic PET of breast cancer xenografts and discuss the implications for experimental response monitoring.
Material and methods
Xenograft tumour model
Seventeen female athymic nude Foxn1nu mice with triple-negative basal-like ductal carcinoma (MAS98. 12) breast cancer xenografts [Citation13] form the basis for this study. All experimental protocols were approved by the National Animal Research Authority and conducted in accordance with the guidelines of the Federation of European Laboratory Animal Science Association (FELASA).
Xenograft fragments of approximately 1 mm3 obtained from previous passage were implanted bilaterally in the mammary fat pads of 7–8-week-old mice (weight 23–25 g). Before implantation of xenografts or PET imaging, animals were anaesthetised by subcutaneous injection at dosage 75 μl/10 g body weight with a combination of 3.8 mg/ml xylazine (Narcoxyl Vet, Roche, Basel, Switzerland), 2.4 mg/ml tiletamine and 2.4 mg/ml zolazepam (Zoletil Vet, Virbac Laboratories, Carros, France), and 0.1 mg/ml butorphanol (Torbugesic, Fort Dodge Laboratories, IA, USA), diluted 1:5 in sterile water.
Dynamic FDG-PET imaging
PET imaging commenced once tumours reached a diameter of 8–10 mm in the longest axis, approximately five weeks after implantation. Following overnight fasting, animals were anaesthetised and catheterised in the tail vein, and groups of three mice were placed with tumours centred in the gantry of a Siemens microPET Focus 120 (Erlangen, Germany) pre-clinical scanner. Ten min transmission scans using a 68Ge point source were conducted for scatter and attenuation correction. Sixty min PET acquisitions were then started in list mode prior to intravenous (i.v.) injection of approximately 10 MBq of FDG (GE Healthcare AS, Oslo, Norway) diluted in 0.9% NaCl. During scanning, animals were covered with an insulating material and kept warm by a heating lamp. Body temperature was maintained at about 35°C as measured by rectal probe in a representative mouse. After completed imaging, anaesthetised animals were sacrificed by cervical dislocation.
Images were reconstructed using OSEM3D-MAP (two OSEM iterations, 18 MAP iterations, β = 0.5, matrix size = 128 × 128 × 95) [Citation14] with resulting voxel size of 0.87 × 0.87 × 0.80 mm3. Reconstructed time frames ranged from 10 s for early time points to 10 min for late time points.
All 17 animals each received one scan. Additionally, four animals underwent a repeat scan one or two days after the first and form the basis for the intra-reproducibility study. Mice were untreated and no major tumour progression or physiological changes were assumed to occur during the experiment.
Image analysis
Images were analysed with bespoke software in IDL 8.3 (ITT Visual Information Solutions, Boulder, CO, USA). Tumours were manually delineated in final frame images.
For normalisation of dPET-data, full-body VOIs were segmented and the total injected activity determined for all animals. This method was chosen as the extracted activity was thought to more accurately represent the actual injected activity than the nominal injected activity of 10 MBq, as all three mice were consecutively injected with a single syringe of FDG, making measurement of residual activity uninformative for individual injected activities. We did indeed find less variability in the data using this approach (data not shown). Pseudo-SUVs (hereafter SUVs) were calculated as the tissue activity concentration in MBq/ml divided by the integrated injected activity (MBq) normalised to full-body volume (ml). Maximum, mean and median SUVs over all contained voxels were determined for all tumours, and three corresponding SUV time activity curves (TACs) were generated for each individual VOI.
Individual arterial input functions (AIFs) were obtained by contouring of the maximal voxels of the aorta in the initial frame of injection for each animal (9–13 voxels). For a given animal, the time at which the AIF reached a maximum was determined and used to shift corresponding tumour TACs along the time axis to a common origin. Animals with AIFs which peaked in the first frame were excluded from the analysis. As one set of animals was scanned for 45 min only, all TACs were interpolated to a uniform time axis with a temporal resolution of 10 s and range 0–45 min.
A total of 38 tumours could be identified in the image material using the final frame of the dynamic acquisition. Two animals were excluded from the analysis due to inadequate AIFs, and one borderline occult tumour was removed. This gave a data set of 34 tumours. Intra-animal reproducibility was evaluated over test-retest scans for a set of seven.
The injected activity estimated from full-body segmentations ranged from 6.5 to 14.6 MBq (mean, 10.8 MBq).
Kinetic modelling
Tumour TACs (max, mean and median) were fitted to a two-tissue compartment model using individual AIFs, as described by Røe et al. [Citation10,Citation15]. In brief, the model assumes that tracer taken up from the blood pool may be present in cells in a free (non-metabolised) or a bound (metabolised) state or compartment. The four rate constants K1, k2, k3 and k4 describe the exchange of FDG between the two compartments; K1 gives a measure of capillary permeability and perfusion rate, k2 describes the efflux of FDG to the blood stream, k3 gives a measure of metabolic phosphorylation of available FDG, whilst k4 represents dephosphorylation of metabolised tracer. In our analysis, FDG is assumed to be irreversibly metabolised and k4 was set to zero.
Variability was examined for K1, k2 and k3, as well as two additional kinetic parameters; vB and MRFDG. vB estimates the fraction of vascular volume in tissues, whilst MRFDG gives a measure of the metabolic rate of FDG, given by MRFDG = (K1k3)/(k2 + k3).
Patlak analysis was performed using SUVmedian AIFs for all tumour TACs by linear regression on data in the equilibrium region from 7.5 min to the end of acquisition. The slope of the regression line, K, gives another measure of metabolic rate of FDG whilst the intercept V0 reflects volume of distribution.
Statistics
The variability of SUVs and pharmacokinetic parameters was examined in terms of the coefficient of variation (CV) expressed as a percentage, given by the standard deviation (SD) divided by the mean. The CV for intra-animal repeatability was calculated as divided by the grand mean of test and retest values, where d is the difference between test and retest values, and n is the number of mice in the intra group. Goodness of fit for the tracer kinetic curves resulting from kinetic modelling was evaluated with the squared Pearson's correlation coefficient (r2) between the original and the fitted data.
Results
shows coronal slices of dPET images obtained for an individual representative animal on Day 1 and 2 at three acquisition times; 2 min, 5 min and 45 min. The tumours, as delineated in the final PET frame, are also shown. As seen, the tumour uptake patterns appear quite similar, both temporally and spatially. At 2 min p.i., little uptake is present in either image, while at 45 min p.i. an active tumour rim is apparent in both tumours at Day 1 and 2.

shows TACs for all the tumours, extracted for the maximum, mean and median uptake in the tissue. An initially rapid uptake is succeeded by a slower accumulation of FDG after about 5–10 minutes. All tumours displayed overall a relatively modest FDG uptake, with a population-based mean SUVmax of 1.9 at 45 min p.i. (). Furthermore, the corresponding SUVmean and SUVmedian in the tumours were similar to each other but almost 60% lower than SUVmax. The uptake curves in reflect rather similar uptake patterns from animal to animal, although SUVmax for the tumours shows greater variability at late time points.

Table I. Overview of PET-data from tumours with average values, standard deviations (SD) and inter and intra coefficients of variation (CV). Pharmacokinetic parameters (non-SUVs) are given as × 102.
From the individual SUVmax, SUVmean and SUVmedian data displayed in , CVs were generated for the inter- and intra-animal groups. The tumours were treated as independent. We also performed the analysis treating tumours from the same animal as a single lesion, but this yielded virtually no change in the numbers (<± 1%). The right column of shows CVs plotted against time p.i. For SUVmean and SUVmedian intra-animal variability is relatively stable 10–45 min p.i. at a level of about 9%. However, SUVmax displays increasing variability after 10 min. The inter-animal CV is mostly greater than the CV for the intra-animal group, especially at late time points. These findings are summarised in for selected time points.
Shown in are the individual AIFs (SUVmedian) obtained for all animals. Panel B shows the range of measured arterial activity over the first 2 min post-injection (p.i.). CVs were calculated for the inter and intra groups, and are shown plotted against time p.i. in Panels C and D of . The inter-animal variability is high with CVs of approximately 35% immediately after injection. Test-retest variability is somewhat higher at 50%. After the initial peak, the intra- and inter-animal CVs drop to 10–25%, with intra-animal variability slightly lower than inter-animal variability.
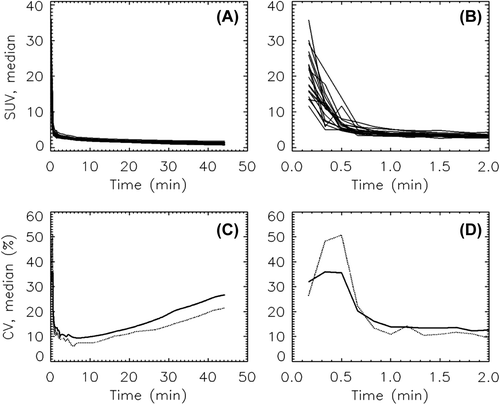
The tumour TACs were fitted to the two- compartment model using individual AIFs, and estimates for pharmacokinetic parameters K1, k2, k3, MRFDG and vB were obtained. A high agreement between experimental and fitted uptake values was found for SUVmean and SUVmedian (r2 = 0.97), but was lower for SUVmax (r2 = 0.66). Population-based mean values and inter- and intra-animal variability for kinetic parameters derived from SUVmax, SUVmean and SUVmedian are given in . As seen, the relative variability for the pharmacokinetic parameters was much higher than for SUV-derived metrics. While CVs for SUV were mostly limited to the range 9–22%, CVs for the pharmacokinetic parameters ranged from 24% to as high as 143%.
Patlak plots (not shown) were constructed for all tumour TACs. Agreement between experimental data and values fitted in the linear region (7.5–45 min) was high (r2 = 0.98). Estimates for the parameters K and V0 were extracted and evaluated for inter- and intra-variability, see . The variability in K is very similar to that found for MRFDG, with CV approximately 35% for the intra group and CV approximately 45% for the inter group. V0 displayed somewhat lower variability with CV approximately 20% for all metrics.
Discussion
In this study, we evaluated the variability of SUV-derived metrics and of parameters from pharmacokinetic analysis of dynamic 18F-FDG-PET of breast cancer xenografts in athymic mice. It was found that: 1) SUVmax shows greater variability compared to SUVmean and SUVmedian; 2) intra-animal variability was lower than inter-animal variability; 3) variability is dependent on the time post-injection; and 4) pharmacokinetic parameters show much greater variability compared with SUV-based metrics.
SUVs obtained late in the acquisition phase (45 min p.i.) were found to have test-retest CVs of 9% for SUVmean/median and 16% for SUVmax. This level of variability is comparable with previous pre-clinical work on a murine melanoma xenograft model in which SUVmean and SUVmax of test-retest scans acquired 6 h apart yielded CVs of around 15% and 18%, respectively [Citation16]. Furthermore, the large difference in variability found between SUVmax and SUVmean/median is in line with previous clinical findings [Citation17]. Nahmias and Wahl studied variability in SUV-based metrics (max and mean) for various cancers in patients [Citation17]. For breast cancer lesions, the variability of test-retest SUVmean ranged from 2% to 4% for the hottest lesions through 10% for intermediate lesions and up to almost 18% for the occult lesions. Considering the rather low uptake and small lesion sizes of the xenografts included in our study, the reproducibility is rather good as compared with clinical data. This is likely due to the superior resolution of animal scanners relative to clinical scanners, reducing partial volume effects. Furthermore, the intra- and inter-animal variability was rather similar in the current work. This is not unexpected, given the highly standardised conditions implemented in the animal model, anticipating a between subject variability which is greater than, but close to the level of within subject variability.
It is not known which SUV-metric is most representative of tumour aggressiveness or best predicts prognosis. Several studies show that a higher FDG uptake as measured by SUVmax typically 1 hr p.i. is associated with a poorer prognosis [Citation18]. Some studies have also compared the prognostic power of different metrics; Borst et al. found that SUVmax better predicted treatment response in inoperable NSCLC patients than SUVmean [Citation19]. Higgins et al., however, found better prognostic value for disease-free survival in SUVmean than SUVmax for head and neck cancer patients [Citation20]. However, tumour hot spots, which may influence prognosis, will not be detected by SUVmean. Furthermore, none of the aforementioned metrics will reflect intra-tumour heterogeneity. The recently published PERCIST guidelines suggest the use of SUVmax or SUVpeak for evaluation of treatment response in solid cancers. In that work, a 30% relative decrease (and 0.8 units absolute decrease) in SUVmax/peak is taken to indicate a significant treatment effect (partial response) [Citation8]. For our data (late acquisition phase; 45 min p.i.), this level of confidence is reached with a relative change of 17% in SUVmean/median and 28% in SUVmax (95% relative test-retest variability). However, in the meta-analysis on test-retest variability in SUV-derived metrics, de Langen et al. [Citation21] found less variability in SUVmean than SUVmax, suggesting that treatment response may be quantified in terms of a 20% relative reduction combined with 1.2 units absolute reduction in SUVmean.
We found that the tumour TACs overall follow similar patterns, with relatively low FDG-uptake which stabilises quite quickly, typically after 5–10 min. The variability of the SUV-based metrics, as measured by the CV, varies over time post-injection. The high variability in the initial phase is in part due to inherent variability and noise in the early uptake of individual tumours, but also a result of the CV being a mean normalised quantity. There is a slight increasing trend in variability with time between tumours, which is likely a reflection of the varying metabolic activity of the different lesions.
The SUV obtained at a given time point p.i. represents the amount of FDG accumulated in the tissue in question up to that point. It is as such a time dependent measure, an aspect which gives rise to variability, and standardisation of time point is thus of importance when comparing SUVs between studies. Through pharmacokinetic modelling, the observed FDG accumulation is explained in terms of vascular perfusion and permeability, GLUT receptor density and glycolytic activity of the tissue. The resulting rate constants are time-invariant, providing physiological descriptors which could be readily comparable between studies. We have, however, found that these pharmacokinetic parameters are highly variable, with poorer reproducibility than SUV-based metrics.
The late SUV (45 min p.i.) is commonly used as a surrogate for metabolic activity. Yet, pharmacokinetic parameters such as MRFDG and k3 are expected to more closely relate to the true metabolism, and are as such attractive as prognostic markers. For instance, Okazumi et al. [Citation22] found a significant decrease in k3 of liver tumours after chemotherapy, and suggest the use of this parameter as a quantitative index for tumour viability. Also, Wahl et al. [Citation23] found a significant correlation between outcome of therapy and early decrease in k3 in breast cancer patients. However, Minn et al. [Citation24] found substantial variability in pharmacokinetic rate constants in lung cancers, with levels of variability slightly lower than in our study, and advocate caution for their use in monitoring of individual patients. One might expect MRFDG to be a more robust metabolic parameter than k3, as large relative noise in k3 propagated from poor signal into the free compartment (low K1) is partially compensated for in MRFDG. Indeed, a substantially lower variability in MRFDG compared to k3 was found in the current work. However, the variability in MRFDG is still roughly three times higher than SUV at 45 min p.i. Both the full pharmacokinetic analysis and the Patlak approach yielded roughly the same level of variability in the metabolic parameters MRFDG and K. As the Patlak approach employs linear regression with only two parameters, whilst the full pharmacokinetic analysis uses non-linear regression with five parameters, it could be expected that Patlak analysis is more robust than pharmacokinetic modelling. This was however not reflected in our findings.
The potential of dynamic PET for evaluating tumour vasculature [Citation12,Citation25] and the associated predictive significance has not been commonly addressed. PET parameters of relevance are in this case K1, V0 and SUV at 2 and 5 min p.i., all reflecting the early tissue distribution phase. Partridge et al. used longitudinal FDG-PET/CT, and found a correlation between reduction in K1 and pathologic response status for patients with breast cancer undergoing neoadjuvant chemotherapy [Citation26]. For complete pathologic responders (pCR), a median reduction of 83% in K1 was found, against a median reduction of 38% for patients not classified as pCR. Previously, we studied the effects of anti-angiogenic therapy in breast cancer xenografts, and could show that K1 was significantly altered 24 h after therapy [Citation12]. Thus, K1 may play a role in response assessment following chemotherapy. Comparing K1 with SUV at 2 min p.i. and V0, the present work indicates that the variability in these metrics is comparable at around 20%. The only exception was K1 obtained for maximum tumour uptake, for which the variability was roughly twice as high. Furthermore, there was a 25–30% reduction in all K1 metrics and a 30–35% reduction in k2 metrics between our test-retest data. As no major tumour progression is assumed to occur in the interim between scans, this is most likely due to the small population size sampled, and may be ascribed to randomness.
The use of an image-derived input function (IDIF) offers an elegant, non-invasive alternative to more laborious blood sampling procedures, particularly in small animal imaging where blood supply is limited. There are however drawbacks to the method, including sensitivity to partial volume effects, movement of the animal during the acquisition and limited temporal resolution. Imprecise representation of the AIF is potentially a great source of error for the estimated parameters. The AIFs obtained across animals in the current work were similar in shape, but, e.g. the SUV in the peak region ranged from 11 to 36. The AIF is thus expected to be a major contributor to the variability observed in pharmacokinetic parameters in this study. A pooled AIF (average of all individual AIFs) was therefore tested in the pharmacokinetic modelling for individual tumours, but this did not reduce variability (data not shown). It may well be that the temporal resolution of the initial frames of PET acquisition was slightly low, giving both smearing and erroneous sampling of the AIF peak. Smearing will lead to a reduction in the AIF peak and thus to overestimation of K1. Furthermore, erroneous sampling will for some miss the peak altogether, again leading to an overestimation of K1. These errors will be further magnified for estimation of parameters associated with the bound compartment (k3, MRFDG), since these depends on input from the free compartment. One could improve accuracy by reconstructing shorter time frames for the first minute of acquisition, but this comes at a cost of decreased signal relative to noise. Our method of IDIF extraction should however be validated against blood sampling. De Geus-Oei et al. found a high correlation (r = 0.98) between IDIFs obtained from the ascending aorta in FDG-PET images and arterial blood samples for a cohort of 76 cancer patients [Citation27]. The temporal sampling rate was 30 s and 15 s for PET and blood sampling, respectively. The plasma peak was underestimated in the IDIFs as compared with the blood samples, which was likely due to the relatively few initial frames acquired, giving rise to an increased averaging effect. Validation of IDIF by arterial sampling in mice is however more challenging because of the small volume which may be withdrawn. Alf et al. suggested the use of an arteriovenous shunt for time-resolved validation [Citation28]. They found a trend towards overestimation of the pharmacokinetic parameters K1 – k3 and MRFDG and greater variability in these when using an IDIF as compared with the shunt input function. In particular, K1 and k2 could be estimated with higher precision using the shunt IF due to the improved temporal resolution in the interval immediately following injection. The authors do recognise the limitations of the shunt IF for high throughput and longitudinal studies and deem the IDIF a satisfactory alternative for such experiments.
To conclude, whether the level of variability in a potential predictive parameter is acceptable depends on the magnitude of the treatment effect. If there is a very small effect of the treatment relative to the variability of the parameter, increasing sample size is not a fruitful way to identify changes in the tumour. It is not certain how much variability is inherently present in the tumour physiology, and as such it may be preferable to use SUVs over pharmacokinetic parameters if the treatment effect is small. Even though SUVs may not provide any major insight into tumour physiology, such parameters display less variability than kinetic parameters.
Acknowledgements
Financial support received from MLSUiO – Molecular Life Science, University of Oslo, and from the K.G. Jebsen Center for Breast Cancer Research, Oslo, Norway is gratefully acknowledged. The authors report no conflicts of interest. The authors alone are responsible for the content and writing of the paper.
Declaration of interest: The authors report no conflicts of interest. The authors alone are responsible for the content and writing of the paper.
References
- Gambhir SS, Czernin J, Schwimmer J, Silverman DH, Coleman RE, Phelps ME. A tabulated summary of the FDG PET literature. J Nucl Med 2001;42(5 Suppl):1S–93S.
- Troost EG, Schinagl DA, Bussink J, Oyen WJ, Kaanders JH. Clinical evidence on PET-CT for radiation therapy planning in head and neck tumours. Radiother Oncol 2010;96:328–34.
- Weber WA. Use of PET for monitoring cancer therapy and for predicting outcome. J Nucl Med 2005;46:983–95.
- de Geus-Oei LF, Vriens D, Arens AI, Hutchings M, Oyen WJ. FDG-PET/CT based response-adapted treatment. Cancer Imaging 2012;12:324–35.
- Pauwels E, Ribeiro M, Stoot J, McCready V, Bourguignon M, Maziere B. FDG accumulation and tumor biology. Nucl Med Biol 1998;25:317–22.
- Strauss LG, Conti PS. The applications of PET in clinical oncology. J Nucl Med 1991;32:623–48; discussion 49–50.
- Castell F, Cook G. Quantitative techniques in 18FDG PET scanning in oncology. Br J Cancer 2008;98:1597–601.
- Wahl RL, Jacene H, Kasamon Y, Lodge MA. From RECIST to PERCIST: Evolving considerations for PET response criteria in solid tumors. J Nucl Med 2009;50(Suppl 1): 122S–50S.
- Fuss M. Strategies of assessing and quantifying radiation treatment metabolic tumor response using F18 FDG positron emission tomography (PET). Acta Oncol 2010;49:948–55.
- Røe K, Aleksandersen TB, Kristian A, Nilsen LB, Seierstad T, Qu H, et al. Preclinical dynamic 18F-FDG PET-tumor characterization and radiotherapy response assessment by kinetic compartment analysis. Acta Oncol 2010;49:914–21.
- Revheim M-E, Kristian A, Malinen E, Bruland ØS, Berner J-M, Holm R, et al. Intermittent and continuous imatinib in a human GIST xenograft model carrying KIT exon 17 resistance mutation D816H. Acta Oncol 2013;52:776–82.
- Kristian A, Revheim ME, Qu H, Mælandsmo GM, Engebråten O, Seierstad T, et al. Dynamic 18F-FDG-PET for monitoring treatment effect following anti-angiogenic therapy in triple-negative breast cancer xenografts. Acta Oncol 2013;52:1566–72.
- Bergamaschi A, Hjortland GO, Triulzi T, Sørlie T, Johnsen H, Ree AH, et al. Molecular profiling and characterization of luminal-like and basal-like in vivo breast cancer xenograft models. Mol Oncol 2009;3:469–82.
- Qi J, Leahy RM. Resolution and noise properties of MAP reconstruction for fully 3-D PET. Med Imaging IEEE Trans 2000;19:493–506.
- Rusten E, Rødal J, Revheim ME, Skretting A, Bruland ØS, Malinen E. Quantitative dynamic 18FDG-PET and tracer kinetic analysis of soft tissue sarcomas. Acta Oncol 2013; 52:1160–7.
- Dandekar M, Tseng JR, Gambhir SS. Reproducibility of 18F-FDG microPET studies in mouse tumor xenografts. J Nucl Med 2007;48:602–7.
- Nahmias C, Wahl LM. Reproducibility of standardized uptake value measurements determined by 18F-FDG PET in malignant tumors. J Nucl Med 2008;49:1804–8.
- Baba S, Isoda T, Maruoka Y, Kitamura Y, Sasaki M, Yoshida T, et al. Diagnostic and prognostic value of pretreatment SUV in 18F-FDG/PET in breast cancer: Comparison with apparent diffusion coefficient from diffusion-weighted MR imaging. J Nucl Med 2014;55:736–42.
- Borst GR, Belderbos JSA, Boellaard R, Comans EFI, Jaeger KD, Lammertsma AA, et al. Standardised FDG uptake: A prognostic factor for inoperable non-small cell lung cancer. Eur J Cancer 2005;41:1533–41.
- Higgins KA, Hoang JK, Roach MC, Chino J, Yoo DS, Turkington TG, et al. Analysis of pretreatment FDG-PET SUV parameters in head-and-neck cancer: Tumor SUVmean has superior prognostic value. Int J Radiat Oncol Biol Phys 2012;82:548–53.
- de Langen AJ, Vincent A, Velasquez LM, van Tinteren H, Boellaard R, Shankar LK, et al. Repeatability of 18F-FDG uptake measurements in tumors: A metaanalysis. J Nucl Med 2012;53:701–8.
- Okazumi S, Isono K, Enomoto K, Kikuchi T, Ozaki M, Yamamoto H, et al. Evaluation of liver tumors using fluorine-18-fluorodeoxyglucose PET: Characterization of tumor and assessment of effect of treatment. J Nucl Med 1992; 33:333–9.
- Wahl RL, Zasadny K, Helvie M, Hutchins G, Weber B, Cody R. Metabolic monitoring of breast cancer chemohormonotherapy using positron emission tomography: Initial evaluation. J Clin Oncol 1993;11:2101–11.
- Minn H, Zasadny KR, Quint LE, Walh RL. Lung cancer: Quantitative measurements for evaluating 2-IF-1 8J-Fluoro-2deoxy-Dglucose uptake at PET. Radiology 1995;1: 167–73.
- Malinen E, Rodal J, Knudtsen IS, Sovik A, Skogmo HK. Spatiotemporal analysis of tumor uptake patterns in dynamic (18)FDG-PET and dynamic contrast enhanced CT. Acta Oncol 2011;50:873–82.
- Partridge SC, Vanantwerp RK, Doot RK, Chai X, Kurland BF, Eby PR, et al. Association between serial dynamic contrast-enhanced MRI and dynamic 18F-FDG PET measures in patients undergoing neoadjuvant chemotherapy for locally advanced breast cancer. J Magn Reson Imaging 2010;32: 1124–31.
- de Geus-Oei L-F, Visser EP, Krabbe PF, van Hoorn BA, Koenders EB, Willemsen AT, et al. Comparison of image-derived and arterial input functions for estimating the rate of glucose metabolism in therapy-monitoring 18F-FDG PET studies. J Nucl Med 2006;47:945–9.
- Alf M, Martić-Kehl M, Schibli R, Krämer S. FDG kinetic modeling in small rodent brain PET: Optimization of data acquisition and analysis. EJNMMI Res 2013;3:1–14.