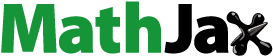
Abstract
Over the last decade, nanotherapeutics gained increasingly important role in drug delivery because of their frequently beneficial pharmacokinetics (PK) and lower toxicity when compared to classical systemic drug delivery. In view of therapeutic payload delivery, convective transport is crucial for systemic distribution via circulatory system, but the target domain is tissue outside vessels where transport is governed by diffusion. Here, we have computationally investigated the understudied interplay of physical transports to characterize PK of payload of nanotherapeutics. The analysis of human vasculature tree showed that convective transport is still 5 times more efficient than diffusion suggesting that circulating and payload releasing drug vectors can contribute mostly to systemic delivery. By comparing payload delivery using systemic circulation and drug vectors to microenvironment, internalized vectors were the most efficient and showed Area under the Curve almost 100 higher than in systemic delivery. The newly introduced zone of influence parameter indicated that vectors, especially internalized, lead to the largest tissue fraction covered with therapeutically significant payload concentration. The internalization to microenvironment minimizes effects of plasma domain on payload extravasation from nanotherapeutics. The computed results showed that classical PK, which mostly relies on concentration profiles in plasma, sometimes might be inadequate or not sufficient in explaining therapeutic efficacy of nanotherapeutics. These results provide a deeper look into PK of drug vectors and can help in the design of better drug delivery strategies.
Introduction
To date, several dozens of drug delivery systems are present in clinics or clinical trials which rely on liposomes, micelles or proteins as drug carriers (Norvaisas & Ziemys, Citation2014). Drug vectors showed improvements in enhanced pharmacokinetics (PK), reduced toxicity or improved therapeutics compared to conventional drugs (Wacker, Citation2014). Because of their size and different surface properties, vectors generate enhanced permeability and retention (EPR) effects in tumors, leading to drug concentrations up to 10 times higher than those in plasma or normal tissues (Maeda, Citation2001). Because of EPR, and other effects such as mononuclear phagocyte system (Geissmann et al., Citation2010), PK of vectors may be very different from PK of small molecule drugs. Therefore, drug molecules carried by a vector adopt the carrier's PK profile (Simovic & Prestidge, Citation2007).
Although nanotherapeutics can be advantageous over free chemotherapy, benefits of drug vectors and pharmacodynamics (PD) can vary from patient to patient based on differences in the tumor and organ microenvironment (Maeda et al., Citation2000; Davis, Citation2008); sometimes having only modest effects (Gordon et al., Citation2001; O'Brien et al., Citation2004; Jain & Stylianopoulos, Citation2010). Although the systemic PK of drugs has been thought to be a major determinant of its utility in clinics, clinical evidences indicate that systemic PK may not be indicative of its therapeutic efficacy and does not reflect intra- and inter-tumor heterogeneity (Müller et al., Citation2004; Wolf & Presant, Citation2004). Moreover, an excellent association between tumor-based PK and PD was shown to 5-FU (Presant et al., Citation1994). Our recent in vivo and computational study has revealed that phenotypic differences of tumor microenvironment can negate enhanced PK properties of nanotherapeutics (Yokoi et al., Citation2014). There, capillary collagen of type IV can be a biophysical marker determining the extravasation of doxorubicin (DOX) by using its liposomal formulation (PLD). It was shown that the 3LL tumors having more collagen than the 4T1 tumors prevent DOX extravasation, in opposite to the 4T1 tumors.
The limited understanding of tumor microenvironment and tumor-based PK/PD relationships can be contributing to shortfalls of nanotherapeutics, which even provokes questioning the future of nanotherapeutics (Park, Citation2013). While systemic PK/PD relationships are heavily scrutinized, tumor-based PK/PD remains an underexplored territory hiding answers to improve drug delivery. The reason this is becoming such a significant issue is the advancement of NP drug delivery systems, which have the capability to reduce the systemic circulation effects of drug compounds and the concentration effects of these particles in the tumor microenvironment, where nanotherapeutics comes to rest.
It is therefore very important to have an adequate knowledge of the mechanisms which determine the transport and kinetics of drugs from systemic circulation to tumor sites (McVie, Citation1984). Because nanotherapeutics alters biodistribution of therapeutic payload, the most efficient drug delivery can be expected if the major payload release takes place at the location of interest, i.e. inside tumor tissue. The drug release from a vector can start immediately after an injection or once vectors meet certain conditions, e.g. get trapped into lysosomes with different pH. If payload starts leaking while vectors are circulating within blood stream, then the release of drug could be subjected to a systemic circulation and, consequently, would have the PK of free drug. Therefore, nanotherapeutics and leaked drug would obey the circulation transport, unless a vector gets physically trapped on vessel wall or is internalized deeper into microenvironment. In the latter case, diffusion becomes the remaining transport mechanism in distributing payload locally among cells.
In this study, we have focused on drug payload transport and PK in tumor microenvironment by using our developed computational model. We investigate how circulation and diffusion transports could affect the delivery of payload to microenvironment from vectors at their different endpoints in circulation. This study quantifies the PK differences at systemic and microenvironment levels and emphasizes critical properties of vectors for optimal drug delivery and its interpretation.
Methods
Drug transport model
We applied a recently developed hierarchical multiscale diffusion modeling technique (Kojic et al., Citation2011a,Citationb; Ziemys et al., Citation2011; Kojic et al., Citation2014), which has an advantage over other approaches because it accounts for characteristics of the diffusion medium microstructure and physico-chemical properties of the diffusing molecules, including the size of the molecules or particles. Also, the model includes motion of particles within fluid which is based on a finite element (FE) solid–fluid interaction solution with large displacements of the moving solids. For simplicity, we neglect drug vector (particle) degradation and consider that drug vector releases its payload as long as the concentration within the vector is larger than in the surrounding.
To evaluate diffusion and convective transport effects in microenvironment, we have adopted a simplification of general 3D unsymmetrical conditions and developed a 2D axisymmetric FE model of capillary and its surrounding, to simulate drug release from a vector, accounting for flow and diffusion effects (). We considered a spherical particle as a vector with 0.25 µm radius and loaded with 1 M concentration of drug. Three cases were simulated: (a) the systemic example, when payload is dissolved in plasma and no drug vector is present, (b) the vector is adhering to capillary wall and (c) the vector is internalized. Each model contains an equivalent mass of payload: 1 M concentration in vectors and 0.60 M in plasma before simulation starts. The following payload diffusivities were used to in the model, unless stated differently: 10−7 cm2/s in fluid (plasma), 10−9 cm2/s in vector and 10−10 cm2/s in solid (tissue). Vectors were immobile during simulations. In all the cases, the entering concentration in fluid is zero. Area under the curve (AUC) was calculated using mean concentration in a specific compartment (plasma or microenvironment) of interest in our model.
Finite element implementation
Here are summarized the fundamentals of the computational model. Considering blood (fluid) and drug vector (solid) as continuous media, the basic equations consist of the balance of linear momentum,
(1)
(1)
and incompressibility condition
(2)
(2)
where σij are stresses,
are volumetric forces and vj are velocity components of a material point; as indicated, repeated index j implies summation j = 1,2,3 for derivatives with respect to coordinates xj. Constitutive laws for elastic solid include Young's modulus E and Poisson's ratio ϑ (equal 0.5 for incompressible material), and viscosity coefficient μ for fluid. We will consider that the drug vector does not deform and hence take for E a large number. Diffusion is governed by Fick's law,
(3)
(3)
so that the mass balance equation, which includes convection, can be written as
(4)
(4)
where qi is mass flux, c is concentration, Dij are diffusion coefficients and qV is a source term.
By a common implementation of the principle of virtual power and Galerkin procedure (Kojic et al., Citation2008), the above balance and continuity equations (1), (2) and (4) can be transformed into FE incremental-iterative algebraic equations of the following form:
(a) for fluid
(6)
(6)
(b) for solids
(7)
(7)
(c) for diffusion
(8)
(8)
Here, ΔVf and ΔVs represent increments of nodal velocity vectors of fluid Vf and solid Vs within time step Δt, ΔPf and ΔPs are increment of the element pressure in fluid and solid, respectively, and Δ C is increment of nodal concentration C; Fext and Fint are external and internal nodal forces, respectively; Qext is external mass flux; and the upper right index “i” denotes equilibrium iteration number, while the index “n” stands for start of a time step. The expressions for the element matrices and vectors are given in the Supplementary Material. In case of diffusion through porous solid, there are no convective terms in Equation (8). The above system of equations corresponds to one FE. This system is further assembled and solved for the nodal quantities for time steps used to calculate evolution of the physical fields of velocity, pressure and concentration; with iterations performed for each time step until convergence is reached (Kojic et al., Citation1998, 2009).
Vascular tree analysis
Human vascular tree was analyzed based on human pulmonary artery tree data (Supplementary Table S1) (Singhal et al., Citation1973). We have characterized each vessel segment by the residence time tR, and the characteristic diffusion time tD,l:
(9)
(9)
(10)
(10)
where v is the blood flow velocity a given vessel segment, L is the vessel length of the segment, R is the radius of vessels in a segment, D is the diffusion coefficient of payload outside of a drug vector. tR characterizes the time of blood elementary volume spending within a given vasculature segment of that vessel size, while tD = tD,l + tD,w – the time payload has to diffuse the longest path (the middle of vessel) through vessel lumen and wall. tD,l was evaluated using D = 10−6 cm2/s. tD,w was evaluated using physiological wall thickness of vessels (Supplementary Figure S2) with diffusivity D = 10−8 cm2/s, because D was found up to 100 times in tissues than fluid (Nugent & Jain, Citation1984).
Results
Considering the overall drug transport, a part corresponding to vector delivery after an injection exclusively depends on flow (convective transport), while another part related to the payload delivery is mostly associated with diffusion (diffusive transport) through microenvironment and is driven by concentration gradient: from concentrated state inside vectors to the surrounding. We analyze the interplay between convective and diffusion transports controlling payload delivery, starting from circulating vector after injection and continuing by diffusion through vessel wall and microenvironment. In our analysis, human pulmonary arterial tree serves as a general model of human vasculature and systemic delivery, which can be of interest for transport within a tumor capillary network.
Systemic circulation
Human vascular tree can be segmented based on a vessel diameter, which has a characteristic flow rate. As estimated for human pulmonary arterial tree, the tree has 17 segments and the flow velocity decreases by decreasing a physiological diameter of human vessels d: the flow velocity dropped from 11 cm/s in aorta (d = 30 mm) down to 0.5 mm/s in capillaries (d = 10 μm) (Singhal et al., Citation1973) (Supplementary Table S1). Consequently, nanotherapeutics is supposed to circulate with similar velocities. In view of payload transport, the characterization of segments in human pulmonary vessel tree was accomplished by calculated the residence time tR and the characteristic diffusion time tD. The ratio tR/tD differentiates the vessels dominated by convective or diffusion transport: in vessels with tR/tD < < 1 dominates convective flow, and in vessels with tR/tD > > 1 – diffusion prevails. The latter can evaluate if payload can reach the microenvironment of a local vessel by passive diffusion from circulating vector.
depicts the calculated tR/tD ratios for the human pulmonary tree. When tR was calculated by using the total length of the segment, the tR/tD ratio shows that diffusion becomes increasingly important in vessels with the diameter of 0.6 mm and smaller. It also suggests that large flow velocity and relatively small fraction of vessels with d > 0.6 mm in the vascular tree eliminate those vessels in the perspective of systemic drug delivery. However, if tR was the calculated by using a characteristic length of each segments, the tR/tD ratio never approaches 1 even for smallest capillaries, suggesting that microenvironment has little chance to be affected directly by the free flowing and locally present drug vector that leaks its payload. Based on physiological data, the convective transport is still 5 times more efficient than diffusion locally in the smallest capillaries of human pulmonary tree with characteristic diameter of 10 µm and length of 130 µm.
Figure 2. Dependence of tR/tD (residence time versus diffusion time) on vessel diameter in the human pulmonary artery tree. Significance of diffusion with respect to convective transport increases with a decrease of vessel diameter; dashed line marks the ratio, where convective and diffusion transport equalize, tR/tD = 1.
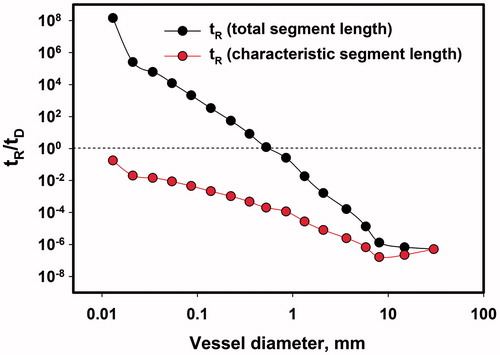
Concentration kinetics
The analysis of vascular tree showed that the release of drug from circulating vectors is not efficient to deliver payload directly to the tissue, because flow overpowers diffusion even in capillaries, where flow velocity is weakest. Most nanotherapeutics deliver payload by adhering of drug vector to a vessel wall or by being internalized into the wall or perivascular space (Ferrari, Citation2010). Upon immobilization of a vector on vessel wall the residence time tR becomes very large, tR∞, because a vector particle can stay attached to the wall until it is degraded. Therefore, the ratio tR∞/tD becomes large and payload release relies on diffusion only. Since the smallest capillaries are dominating in the payload exchange with tissues, we have studied in our model the payload release in a capillary and surrounding microenvironment.
Three examples were compared in our capillary model: (A) systemic circulation, (B) the vector is adhering to capillary wall and (C) the vector is internalized (). In order to compare systemic delivery with the other two cases, we have taken the mass of drug within the drug vector and equally distributed over the plasma domain as shown in . This initial concentration of the model (A) changes due to plasma flow through the domain and due to extravasation through capillary walls (initial concentration in the microenvironment is zero). In other two models, the assumption was that the initial concentration outside the drug vector was equal to zero. The calculated results revealed that the highest concentration in plasma was found in case of systemic delivery and an adhering vector, but in few hundreds of seconds the highest concentration in plasma is calculated for the internalized vector that leaks its payload out (). On the other hand, concentration in microenvironment was the highest when using drug vectors, especially when a vector is internalized: the concentration was by two orders of magnitude higher than in other cases. The systemic delivery among all cases showed the least payload delivered to microenvironment. Naturally, AUC in microenvironment was found to be the largest for an internalized vector, while the systemic delivery produced the largest AUC in plasma ().
Zone of influence in microenvironment
We have investigated in details the concentration fields in microenvironment produced by different endpoints of vectors, and have introduced the concept of zone of influence (ZOI). ZOI represents the tissue domain in microenvironment affected by the concentration, which is above an arbitrary no-effect level, i.e. having a therapeutic effect. For visually better comparison of ZOI, we have used sub-physiological plasma flow velocity of 10 µm and the 0.0001 M concentration as the no-effect value. illustrates ZOI evolutions for adhering and internalized drug vectors. The computed results show that adhered particle delivers the payload by diffusion to microenvironment, but a substantial part of payload is removed by plasma flow in capillary at the beginning of the release (). In opposite, the internalized drug vector develops ZOI gradually that increases in size with time as seen in . shows that ZOI increases all the time in case of internalized vector, while an adhered vector has therapeutic potential only in the initial phase of release until payload is cleared by plasma flow (). For the sake of better presentation, these examples used plasma flow velocity 10 times lower than physiological leading to proportionally larger ZOI within analysis window; the calculated ZOI in both cases could be lower in physiological conditions; that is especially true for the example of an adhering vector.
Figure 5. Characteristic evolution of ZOI in microenvironment for the adhered (top row; A–C) and internalized (bottom row; D–F) drug vectors at the beginning (A and D), middle (B and E) and end of payload release (C and F). ZOI was evaluated by assuming the arbitrary concentration 0.0001 M, below which there is no therapeutic effect (the “no effect” concentration); the concentration scale depicted in (A) has units of 10−3 M.
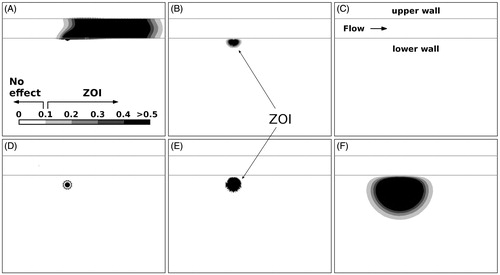
Figure 6. Evolution of ZOI in microenvironment for different drug delivery modes: systemic, adhered vector and internalized vector.
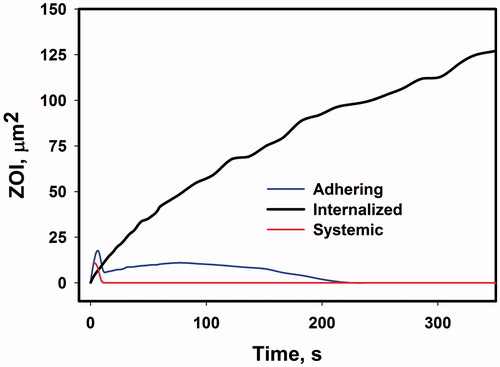
The coupling of flow and diffusion in microenvironment was further investigated by exploring AUC and ZOI at different payload diffusivities in tissue and plasma flow velocities by using same model as presented in . The computed results show that the payload diffusivity in tissue does not modulate AUC in fluid in case of internalized or adhering vectors (). However, AUC in fluid is 5 times lower for the internalized drug vector than adhering. On the other hand, AUC in tissue was exclusively affected by payload diffusion in microenvironment, with almost no sensitivity to the velocity of plasma flow. ZOI revealed the dependence on diffusivity similar to AUC in tissue domain, suggesting that diffusivity is crucial determining the payload penetration, and that once a vector is internalized, the plasma domain has little effect on concentration gradients inside microenvironment. However, if the payload penetration is coming from the plasma domain, the flow velocity is important for the efficacy of payload extravasation.
Figure 7. AUC and ZOI in microenvironment and plasma in terms of payload diffusivity in tissue D and plasma flow velocity v for a drug vector adhering to vessel wall (left column) and internalized into vessel microenvironment (right column). The insets with number indicate AUC (top row – plasma; middle row – microenvironment) in M·s, and ZOI (bottom row) in μm2.
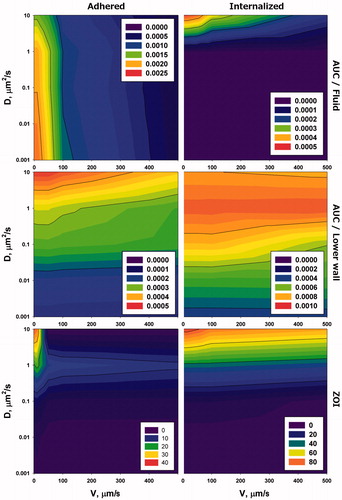
Discussion
The analysis of convective and diffusion transports has provided an interesting insight into drug delivery. First, the residence time tR calculated by using the total length of a segment in human pulmonary tree shows that vessels smaller than 0.6 mm in diameters can contribute to systemic delivery. If tR is calculated for a characteristic length of vessel of a specific diameter, the parameters tR and tD quantified that a circulating vector does not have much efficiency to deliver its payload locally, because tR is too small to ensure that payload diffuses through vessel lumen and wall to perivascular space. For example, 10 µm capillary with the characteristic length of 130 µm has a physiological velocity that is at least 5 times too high to make diffusion efficient for circulating vectors (assuming they are leaky to drugs). These results show clearly that only the domain of the smallest capillaries can be efficient in drug delivery to microenvironment. tR and tD also help to understand better why nanotherapeutics is not efficient from systemic drug delivery. Based on this analysis, circulating drug vectors that leak payload should have little different outcome from systemic delivery. Circulating nanotherapeutic particulates can eventually be immobilized on vessels and most frequently – on capillaries, which compose 95% of vascular tree length. The capillary segment of the vascular tree is mostly relevant to the human pulmonary tree, however, our analysis provides a physics-based insight into what aspects of physiology might be important to drug delivery and nanotherapeutics.
Once drug vectors are immobilized by capillaries, the results showed major differences with respect to systemic drug delivery, and revealed an important interplay between convective and diffusion transport. As tR becomes very large – an immobilized vector does not flow anymore, and payload delivery becomes diffusion controlled. Therefore, the condition whether vectors circulate within plasma, or are attached to the wall or internalized into tissue, becomes crucial for the drug delivery to microenvironment. It was found that the most efficient way of payload delivery is by internalization of a drug vectors into microenvironment, e.g. tumor. In this optimal scenario, payload release is controlled exclusively by diffusion in tissue and plasma may only affect the efficiency of drug delivery by a wash of the payload from microenvironment. The internalized vector also provides the largest ZOI that measures the microenvironment fraction affected by therapeutically significant drug concentration. The interesting fact in such scenario is that AUCs in plasma and tissues may be different by few orders of magnitude, suggesting that plasma concentration may not always be the adequate measure of drug exposure and efficacy in microenvironment, as it is commonly used in classical PK studies.
In case of an adhered vector, drug delivery characteristics are between systemic drug delivery and the internalized vector, as the vector is exposed at the same time to plasma flow and diffusion through the vessel wall. An adhered vector developed a short-lived ZOI that was many times smaller than the internalized vector and relatively small AUC. Although ZOI and AUC in microenvironment were not as efficient as for the internalized vector, the adhered vector provided more payload to microenvironment than systemic delivery. As expected, the systemic delivery is completely dependent on flow and the drug extravasation is almost unaffected by payload diffusivity in microenvironment. Although no significant ZOI or AUC was found for the case of the systemic delivery, ZOI and AUC could be significant upon a longer exposure. But that would also be true for the adhering and internalized vectors.
Vaupel et al. (Citation1989) have summarized and compared blood flow in normal tissues and tumors in different organs. Their data show that flow rate decreases from 10 to 0.02 ml/g/min (by 500 times) in healthy tissues in the following order (as an example): thyroid > kidney > liver > brain > skin > adipose tissue. At the same time, the comparison shows that tumor tissues have lower flow rate that ranges from 1 to 0.01 ml/g/min. That big range of flow rates means large differences in tR and how efficiently payload is washed out from immobilized vectors. Also, those ranges might influence nanotherapeutic efficacy modulating payload delivery depths in tissues. The flow rate could have less than a dramatic effect for systemic circulation, because drug concentration in plasma entering and leaving a capillary is almost constant. But, in case of drug vectors, it is different, because the payload release is local to a capillary and its microenvironment, also with a removal of payload from the microenvironment. At this point, this analysis can draw a connection to the EPR effect (Maeda, Citation2001) helping partially in explaining why nanotherapeutics frequently provides benefits to cancer treatment over a systemic delivery.
Conclusions
The results show that diffusion and convective transports play important roles at different domains affecting the drug delivery efficiency. Considering the efficiency of payload delivery by nanotherapeutics into microenvironment, the best delivery method would be internalization of drug vectors. As drug vectors have limited diffusivity in microenvironment due to their size, the diffusion of payload will be controlling drug delivery. Based on the investigated examples, by the increasing association or internalization of drug vector into microenvironment, the payload release will be decoupled from the plasma compartment. For the same reason, plasma concentrations might be inadequate to assess the efficacy of drug delivery. In opposite to internalized or adhered vectors, circulating drug vectors (if they leak payload) conform to systemic drug delivery patterns. The results help to elucidate the transport aspect that is pertinent to PK of payload delivered by nanotherapeutics and may find use in drug delivery and nanomedicine.
Supplementary material available online
Supplementary Figures S1 and S2 and Table S.
Supplemental Material.pdf
Download PDF (616 KB)Declaration of interest
The authors acknowledge support from the Houston Methodist Research Institute, Ministry of Education and Science of Serbia (OI 174028, III 41007), City of Kragujevac, and EU grant FP7-ICT-2007 project (224297, ARTreat); the National Institute of Health (U54CA143837 – M.F., K.Y., U54CA151668 – M.F.), the Ernest Cockrell Jr. Distinguished Endowed Chair (M.F.), and the US Department of Defense (W81XWH-09-1-0212) (M.F.).
M.F. serves on the Board of Directors of NanoMedical Systems, Inc., ArrowHead Research Corporation, and discloses potential financial interest in the companies. All other authors declare no competing financial interests.
References
- Davis ME. (2008). Nanoparticle therapeutics: an emerging treatment modality for cancer. Nat Rev Drug Discov 7:771–82
- Ferrari M. (2010). Frontiers in cancer nanomedicine: directing mass transport through biological barriers. Trends Biotechnol 28:181–8
- Geissmann F, Gordon S, Hume DA, et al. (2010). Unravelling mononuclear phagocyte heterogeneity. Nat Rev Immunol 10:453–60
- Gordon AN, Fleagle JT, Guthrie D, et al. (2001). Recurrent epithelial ovarian carcinoma: a randomized phase III study of pegylated liposomal doxorubicin versus topotecan. J Clin Oncol 19:3312–22
- Jain RK, Stylianopoulos T. (2010). Delivering nanomedicine to solid tumors. Nat Rev Clin Oncol 7:653–64
- Kojic M, Filipovic N, Slavkovic R, et al. (1998, 2009). PAK-FS – finite element program for fluid flow and fluid-solid interaction. Kragujevac: University of Kragujevac and R&D Center for Bioengineering
- Kojic M, Filipovic N, Stojanovic B, et al. (2008). Computer modeling in bioengineering: theoretical background, examples and software. Chichester, England, John Wiley and Sons. pp 131–156
- Kojic M, Milosevic M, Kojic N, et al. (2011a). On diffusion in nanospace. J Serbian Soc Comput Mech 5:104–18
- Kojic M, Ziemys A, Milosevic M, et al. (2011b). Transport in biological systems. J Serbian Soc Comput Mech 5:101–28
- Kojic M, Milosevic M, Kojic N, et al. (2014). A multiscale MD-FE model of diffusion in composite media with internal surface interaction based on numerical homogenization procedure. Comput Methods Appl Mech Eng 269:123–38
- Maeda H. (2001). The enhanced permeability and retention (EPR) effect in tumor vasculature: the key role of tumor-selective macromolecular drug targeting. Adv Enzyme Regul 41:189–207
- Maeda H, Wu J, Sawa T, et al. (2000). Tumor vascular permeability and the EPR effect in macromolecular therapeutics: a review. J Control Release 65:271–84
- McVie JG. (1984). The role of pharmacokinetics in (combination) chemotherapy. Cancer 54:1175–8
- Müller M, dela Peña A, Derendorf H. (2004). Issues in pharmacokinetics and pharmacodynamics of anti-infective agents: distribution in tissue. Antimicrob Agents Chemother 48:1441–53
- Norvaisas P, Ziemys A. (2014). The role of payload hydrophobicity in nanotherapeutic pharmacokinetics. J Pharm Sci 103:2147–56
- Nugent LJ, Jain RK. (1984). Extravascular diffusion in normal and neoplastic tissues. Cancer Res 44:238–44
- O'Brien M, Wigler N, Inbar M, et al. (2004). CAELYX Breast Cancer Study Group: reduced cardiotoxicity and comparable efficacy in a phase III trial of pegylated liposomal doxorubicin HCl (CAELYX/Doxil) versus conventional doxorubicin for first-line treatment of metastatic breast cancer. Ann Oncol 15:440–9
- Park K. (2013). Facing the truth about nanotechnology in drug delivery. ACS Nano 7:7442–7
- Presant C, Wolf W, Waluch V, et al. (1994). Association of intratumoral pharmacokinetics of fluorouracil with clinical response. Lancet 343:1184–7
- Simovic S, Prestidge CA. (2007). Nanoparticle layers controlling drug release from emulsions. Eur J Pharm Biopharm 67:39–47
- Singhal S, Henderson R, Horsfield K, et al. (1973). Morphometry of the human pulmonary arterial tree. Circ Res 33:190–7
- Vaupel P, Kallinowski F, Okunieff P. (1989). Blood flow, oxygen and nutrient supply, and metabolic microenvironment of human tumors: a review. Cancer Res 49:6449–65
- Wacker MG. (2014). Nanotherapeutics – product development along the “nanomaterial” discussion. J Pharm Sci 103:777–84
- Wolf W, Presant CA. (2004). Tumor-based pharmacokinetics has greater significance for anticancer drugs than does blood-based pharmacokinetics. Clin Pharmacol Ther 76:508–8
- Yokoi K, Kojic M, Milosevic M, et al. (2014). Capillary-wall collagen as a biophysical marker of nanotherapeutic permeability into the tumor microenvironment. Cancer Res 74:4239–46
- Ziemys A, Kojic M, Milosevic M, et al. (2011). Hierarchical modeling of diffusive transport through nanochannels by coupling molecular dynamics with finite element method. J Comput Phys 230:5722–31