Abstract
Information from health care encounters across the entire health care spectrum, when consistently collected, analysed and applied can provide a clearer picture of patients’ history as well as current and future needs through a better understanding of their morbidity burden and health care experiences. It can facilitate clinical activity to target limited resources to those patients most in need through risk adjustment mechanisms that consider the morbidity burden of populations, and it can help target quality improvement and cost saving activities in the right places. It can also open the door to a new chapter of evidence-based medicine around multi-morbidity. In summary, it can support a better integrated health system where primary care can provide continuous, coordinated, and comprehensive person-centred care to those who could benefit most. This paper explores the potential uses of information collected in electronic health records (EHRs) to inform case-mix and predictive modelling, as well as the associated challenges, with a particular focus on their application to primary care.
KEY MESSAGE:
Information consistently collected in electronic health records over time and across providers can improve patient and population level health care.
Case-mix and predictive modelling tools use this information to improve the planning and delivery of care.
Structural, financial, knowledge and cultural barriers can pose challenges in the implementation of a health information technology strategy.
INTRODUCTION
This article highlights the key points presented by the primary author at the inaugural Barbara Starfield Memorial Lecture delivered 29 June 2013 at the 20th World Organization of Family Doctors (WONCA) World Conference in Prague, Czech Republic.
Following a review of the importance of capturing information from electronic health records (EHR), various methods of analysing the EHR data are described. These methods include the measurement of multi-morbidity as well as the application of case-mix and predictive modelling tools. Such tools transform the EHR data into actionable information and several applications of such information are discussed. Successful implementation of a health information technology strategy faces numerous challenges, which are also outlined prior to the conclusion.
THE ROLE OF INFORMATION FROM ELECTRONIC HEALTH RECORDS
Information on the health of patients and populations, delivered in a timely manner, is essential to all those involved in delivering and paying for care. This can be facilitated through improved recording and transfer of information (a structural element), and application of such information in the ongoing care of a patient and decisions regarding allocation of resources (a process element).
Numerous methods exist to analyze data and apply the resulting information of the relative morbidity burden of individuals and populations. provides an overview of the process through which information can improve health care.
Analysing EHR data
A detailed EHR, at the individual patient and clinician level, can offer a comprehensive historical record. Yet, the analysis of data to produce information can produce further benefits to patient and population care. Tools to transform routinely collected EHR data into actionable information can support the clinician's decision making process to prioritize and provide better tailored care. They can also provide a better understanding of the need for and use of health care resources, which is of interest to clinicians, payers of care and policymakers (Citation1).
Measuring morbidity, case-mix and risk adjustment. The terms ‘case-mix’ and ‘risk adjustment’ are used to describe the process through which the health status (e.g. morbidity burden) of an individual or population is measured and taken into consideration when evaluating care or allocating resources. Classically this has been done by considering the age and sex of patients; however this explains a limited amount of variation in care. For example, it has been shown to explain only 10% of variation in prescribing (Citation2). Being able to assess the morbidity burden based on the analysis of detailed EHRs of individual patients over time and across providers can provide a much more comprehensive picture, and can significantly improve the ability to explain variations in care. Compared to age and sex, morbidity can explain almost six times more variation in general practice referrals and eight times more variation in prescribing (Citation2,Citation3).
Predictive modelling. Predictive modelling is a statistical process that uses regression analysis to identify patterns and predict the likelihood of future events such as hospitalization and risk of being high cost in the future (Citation4,Citation5). A comprehensive measure of morbidity burden when incorporated into predictive models, facilitates the identification of individual high-risk patients for clinical interventions as well as helps estimate health care resource use for entire populations (Citation6,Citation7). Tailored reports can then provide targeted Information to clinical and non-clinical decision makers and clinicians on patients, populations and providers of care.
Potential applications of case-mix and predictive modelling
There are numerous purposes to which the assessment of morbidity burden can be applied. Three applications are described in more detail below:
supporting clinical judgment in assessing and predicting individual patient health needs (patient profiling);
improving coordination amongst multiple clinicians; and
research around multi-morbidity.
Patient profiling. Risk profiling and risk stratification refer to the use of clinical judgment, clinical thresholds or statistical techniques such as predictive modelling to identify individuals or groups of patients with an increased risk of unwanted outcomes who would most likely benefit from targeted interventions. When patients present, traditionally clinicians have used clinical judgment and clinical thresholds to identify patients who are at risk and need greater attention. However, patients may be missed, such as those who do not present frequently or those who see multiple clinicians. Clinicians may also be unduly influenced by the recurrent presentation of some patients who may not be those at highest risk of significant medical complications. Fragmented systems with diminished continuity of care and clinicians with large caseloads and short appointments contribute to this challenge. Therefore, predictive modelling tools can improve traditional screening methods by using information from EHRs to help proactively identify patients at higher risk and support clinical judgment.
Care for these patients, who typically suffer from multiple, chronic conditions, can be tailored to intervene before the patient becomes a high-risk case. Often, it is not the existing high risk individuals who can benefit most from intervention, but those who are anticipated to be high risk in the future where clinical interventions can mitigate those patients from progressing to becoming high risk. Identification of these patients is facilitated by predictive modelling based on these patients’ previous health care experiences.
Once patient populations have been stratified based on their predicted risk, targeted interventions can be applied based on the patients’ needs (). For example, information to enable patients to self-manage their conditions can be offered to those at lowest risk, while health coaching and lifestyle management programmes can be offered to those at moderate risk. For those at highest risk, case management and individualized care plans can be developed, including active monitoring by a clinician.
Figure 2. Stratification of populations facilitates targeted interventions. Source: Johns Hopkins Health Care, 2006.
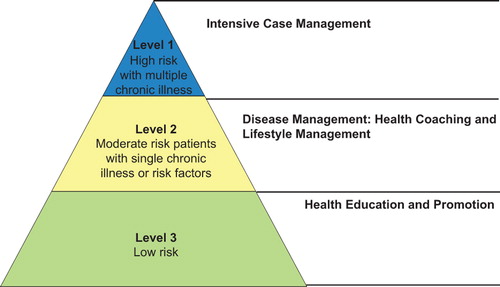
If patients are already high risk, interventions can aim to reduce the probability of an adverse event such as a preventable hospital admission. Such events are undesirable for patients, are costly for the health system, and are indicators of poor quality of care.
Improving coordination. EHRs can also record how many unique clinicians the patient has seen and where most care was provided. This information can help identify patients who are at risk of lack of continuity and coordination of care. An assessment of referral behaviour begins with the referring clinician. Yet often the clinician is unaware of the frequency or types of referrals they make or whether their referrals are appropriate. To be able to reflect on their referral and make a fairer comparison with their colleagues, they need to be provided with information regarding the morbidity profile of their patient load.
The analysis of information from EHRs on clinician prescribing and, where possible, pharmacy dispensing, can also help identify issues around pharmacy use, which may include identifying potential drug interactions, untreated conditions or gaps in treatment.
Research around multi-morbidity. To date most clinical guidance is based on single disease conditions and most pharmaceutical evidence has been based on relatively young patients with limited or no comorbidity. This is no longer reflective of the population that many health systems need to care for. As such, the imperative is to develop evidence based on patients with multi-morbidity (Citation8). Detailed EHRs that can capture the complexity of patients and tools that enable the analysis and ongoing evaluation of these patients will be of increasing importance as populations’ age and multi-morbidity becomes more common.
CHALLENGES
Implementing an information exchange strategy is not easy. Significant challenges can be encountered along the way including structural, financial, knowledge-based and cultural barriers.
Access
First and foremost, clinicians need access to computers and an EHR tool. Who pays for the investment in health technology needs to be aligned with the overall benefits, which can include improved equity in the provision of care, improved quality of care and reduced costs. Trends indicate that countries are at various stages of implementing EHRs and although challenges remain around the implementation of health information exchange strategies, overall primary care is progressing in its use of information technology (Citation9,Citation10). In countries where there has been leadership and financial support provided by government, the adoption of information technology in health care has been accelerated (Citation10).
Standardization
Non-standardized data information systems can make the sharing of data between the various stakeholders difficult and unreliable. This is where policymakers need to be active and develop a national eHealth strategy with a national data dictionary, in other words, a common language for health records to talk to one another. Advocacy and involvement on the part of clinicians in the design of such a strategy is essential. Such a strategy should also set minimum data collection requirements and standards (Citation11). Ideally, this would cover all sectors relevant to health including primary care, secondary care and social care. In addition to interoperability, attention needs to be given to ensure information silos are avoided. Having patients’ information on a clinic computer, in a hospital system, disease registry, or a payer's computer without providing an overall picture is of limited value.
Information governance
There are also important information governance issues including patient confidentiality and data ownership, which need to be carefully addressed (Citation12). Solutions for handling privacy have been developed with encryption protocols being applied to health care information. Informed consent of patients regarding who has access to their EHR is important. However, opt-in mechanisms require significant investment to inform patients and may result in reduced participation. Implied consent and opt-out mechanisms may capture more participants; however, decisions regarding what information is shared, with whom, and how, need careful consideration.
Clinician involvement
Clinicians need to recognize the value of health information technology in their clinical practice. Beginning in medical school, the use and benefits need to be taught, and should continue through postgraduate training and continuous professional development programmes to keep clinicians abreast of developments. There also needs to be regular feedback to clinicians on the information that is gathered, particularly on how this can improve clinical care. There has been considerable debate on whether the practice of medicine is becoming too technical and less patient-focused. Understandably, clinicians want to interact with their patient, not a computer screen. Health information technology should not be a barrier to personal interaction with patients, rather a tool that augments and supports the clinician in this information age. Payment mechanisms for clinicians need to incorporate incentives for the use of EHRs, which must allow clinicians to code with ease without distracting from the consultation. Yet incentives that lead to gaming and a distortion of data, or worse a distortion of care, for financial gain must be avoided (Citation13).
There is a growing appreciation of what measuring multi-morbidity, applying case-mix tools, and undertaking predictive modelling with comprehensive EHR information can be used for. However, the availability of information is not sufficient to improve the delivery of primary care (Citation12,Citation13). Clinicians need to ensure that data are completely and accurately recorded and the resulting information is applied in their clinical practice, whilst policies are required to standardize data capture systems and incentivize professionals to use them.
CONCLUSION
Health care and health needs are changing rapidly. Health information tools to improve clinical, managerial, financial and policy decisions are needed. Advances in information technology present a significant opportunity to improve how care is delivered, assessed and paid for.
Information, when adequately collected, analysed, and applied, provides a clearer picture of patients’ needs through a better understanding of their morbidity burden and experiences across the entire health care spectrum. It enables clinical activity to be more fairly compared, and can help the targeting of quality improvement and cost saving activities in the right places. It can open the door to a new chapter of evidence based medicine around multi-morbidity. In summary, it can support a better health system where primary care can provide continuous, coordinated, and comprehensive person-centred care.
Concerted national efforts are needed to support the use of EHRs to improve care. Health information technology needs to be viewed as a common good. To achieve this, both government support and clinical leadership, in particular from primary care, are essential.
Acknowledgements
The content of this paper reflects that of the keynote Barbara Starfield Memorial Lecture given during the 20th World Organization of Family Doctors’ (Wonca) World Conference in June 2013 by Karen Kinder. The authors should like to acknowledge Normalie Barton for her assistance in preparing the manuscript.
Declaration of interest: The authors alone are responsible for the content and writing of the paper.
The Johns Hopkins University has copyrighted software based on the ACG System that is one of the tools available to help analyse health care data as described in this article. Royalties are paid to the university when this software is licensed. The corresponding author is a faculty member of the Johns Hopkins University.
REFERENCES
- Starfield B, Kinder K. Multimorbidity and its measurement. Health Policy 2011;103:3–8.
- Omar RZ, O’Sullivan C, Petersen I, Islam A, Majeed A. A model based on age, sex, and morbidity to explain variation in UK general practice prescribing: Cohort study. BMJ 2008;337:a238.
- Sullivan CO, Omar RZ, Ambler G, Majeed A. Case-mix and variation in specialist referrals in general practice. Br J Gen Pract. 2005; 55:529–33.
- Lemke KW, Weiner JP, Clark JM. Development and validation of a model for predicting inpatient hospitalization. Med Care 2012; 50:131–39.
- Orueta JF, Nuno-Solinis R, Mateos M, Vergara I, Grandes G, Esnaola S. Predictive risk modelling in the Spanish population: A cross-sectional study. BMC Health Serv Res. 2013;13:269.
- Murphy SM, Castro HK, Sylvia M. Predictive modeling in practice: Improving the participant identification process for care management programs using condition-specific cut points. Popul Health Manag. 2011;14:205–10.
- Weir S, Aweh G, Clark RE. Case selection for a Medicaid chronic care management program. Health Care Financ Rev. 2008;30: 61–74.
- Smith SM, Soubhi H, Fortin M, Hudon C, O’Dowd T. Managing patients with multimorbidity: Systematic review of interventions in primary care and community settings. BMJ 2012;345:e5205.
- Schoen C, Osborn R, Squires D, Doty M, Rasmussen P, Pierson R, et al. A survey of primary care doctors in ten countries shows progress in use of health information technology, less in other areas. Health Aff. 2012;31:2805–16.
- Davis K, Doty MM, Shea K, Stremikis K. Health information technology and physician perceptions of quality of care and satisfaction. Health Policy 2009;90:239–46.
- Protti D. Reflections on international EHR journeys. Healthcare Information Management and Communications 2009; 23:6–9.
- Banfield M, Gardner K, McRae I, Gillespie J, Wells R, Yen L. Unlocking information for coordination of care in Australia: A qualitative study of information continuity in four primary health care models. BMC Fam Pract. 2013;14:34.
- Soler JK, Pringle M. Research using electronic patient records in general practice. The EGPRN meeting in Bertinoro, Italy, May 2009. Eur J Gen Pract. 2010;16:186–9.