Abstract
Our objective was to describe a new endpoint for amyotrophic lateral sclerosis (ALS), the Combined Assessment of Function and Survival (CAFS). CAFS ranks patients’ clinical outcomes based on survival time and change in the ALS Functional Rating Scale–Revised (ALSFRS-R) score. Each patient's outcome is compared to every other patient's outcome, assigned a score, and the summed scores are ranked. The mean rank score for each treatment group can then be calculated. A higher mean CAFS score indicates a better group outcome. Historically, ALS clinical trials have assessed survival and function as independent endpoints. Combined endpoints have been used in other diseases to decrease the confounding effect of mortality on analysis of functional outcomes. We explored the application of a similar approach in ALS, the CAFS endpoint, which was used as a pre-specified secondary analysis in a phase II study of dexpramipexole. Those results and some hypothetical examples based on modeling exercises are presented here. CAFS is the primary endpoint of a dexpramipexole phase III study in ALS. In conclusion, the CAFS is a robust statistical tool for ALS clinical trials and appropriately accounts for and weights mortality in the analysis of function.
Trial registration: ClinicalTrials.gov identifier: NCT01281189.
Introduction
Evaluations of function and survival are equally important in characterizing disease progression in amyotrophic lateral sclerosis (ALS). The use of survival as the primary endpoint requires large, lengthy trials whereas the use of functional decline as the primary endpoint leads to statistical analysis issues such as missing functional data due to death and loss-to-follow-up from disability (Citation1 − 3). Many ALS clinical trials have tested potential therapies using either function or survival as the primary study endpoint but have yielded disappointing results on survival and no success on improving function (Citation4 − 6). Whether these trials have failed because of the clinical outcome measures and analysis (Citation4,Citation5) or due to challenges in effectively targeting ALS pathophysiology remains uncertain.
In analyzing trial outcomes, all functional outcome data are missing after a participant dies. For statistical analysis, these data must somehow be inferred. Functional outcome scores can be reported as a value of zero after death, but this may underestimate function. Functional data can be imputed using the last observation carried forward approach, but in a disease such as ALS where function declines over time, carrying forward a functional value obtained before death will overestimate function (Citation7). Techniques such as the mixed-effects model slopes analysis assume that missing data can be predicted by scores before death or drop–out (i.e. that the missing data are non-informative), which may not be valid and could lead to biased results.
Individually, both function and survival provide only partial information about disease progression and response to therapy. As ALS progresses, the ALS Functional Rating Scale–Revised (ALSFRS-R) total score declines; however, no score predicts imminent death, likely because of disease heterogeneity. In some ALS trials, coprimary endpoints have been chosen (e.g. function and forced vital capacity or survival and function) (Citation8); however, statistical analyses of each endpoint are required to be performed independently, with success requiring significant changes in both primary endpoints.
A combined endpoint that evaluates function and survival together would provide a more accurate indication of an intervention's effect than independent analyses. However, because each of these outcomes can affect the results of the other, most standard techniques for the analysis of function do not adequately account for missing functional data due to deaths. The Combined Assessment of Function and Survival (CAFS) is a novel endpoint that evaluates function while appropriately accounting for missing data due to deaths in ALS. CAFS ranks each subject according to their outcome, with the worst outcome assigned to the subject who dies first in the study and the best outcome assigned to the subject who survives with the least functional decline. The CAFS analysis is non-parametric and does not rely on statistical assumptions required for many of the standard techniques such as linearity or data imputation.
In general, combined endpoints are advantageous because they: 1) more comprehensively estimate the overall clinical benefit of a particular treatment; 2) allow simultaneous analysis of multiple equally important outcome measures without relying on multiple comparisons or coprimary endpoints; 3) account for effect modification and confounding of the outcome measures being combined; 4) offer additional statistical power in many scenarios; and 5) appropriately adjust for missing data owing to deaths and drop-outs without the assumptions of traditional parametric analyses (such as imputing a score of 0 for death) (Citation9 − 11).
In other diseases, combined outcomes draw together multiple measures of disease progression into a single outcome. Since 1984, when a key description of joint outcome analysis modeling was published (Citation12), combined endpoints have been used extensively in clinical trials in rheumatology (Citation13), human immunodeficiency virus (Citation9), and cardiovascular research (Citation14) (). Cardiovascular trials use combined measures consisting of 3 − 4 endpoints (Citation14), and in rheumatology studies, the American College of Rheumatology 20% improvement response criterion is accepted as a pivotal trial endpoint (Citation13). A recent review of HIV studies revealed wide adoption of a combined endpoint (Citation9) first proposed by Finkelstein and Schoenfeld in 1999 (Citation15). In this analysis, ranking of study participants was based on pairwise comparisons of participants using 1) time to death, and 2) time to loss of virologic response to generate individual rank scores. Additional strategies to co-analyze longitudinal data and survival have since been proposed (Citation16 − 18).
Table I. Examples of published composite endpoints and analyses for clinical trials.
Based on the Finkelstein and Schoenfeld methodology (Citation15), the CAFS can be viewed as an analysis of ALSFRS-R that adjusts for mortality. The aim of this report is to examine the application of the CAFS measure as a novel endpoint in clinical trials of ALS, using preliminary data from a phase II trial of dexpramipexole in ALS and hypothetical simulations.
Methods
Use of CAFS as an endpoint in ALS
CAFS is used to compare each study participant's outcome to others in the trial in a series of pairwise comparisons, based on function and survival. For each pairwise comparison, a study participant is assigned a score and then the summed scores are ranked for all participants (). Details of the scoring and ranking process are given below.
Figure 1. Example of the Combined Assessment of Function and Survival calculation using a hypothetical study with six patients. ALSFRS-R: Amyotrophic Lateral Sclerosis Functional Rating Scale–Revised.
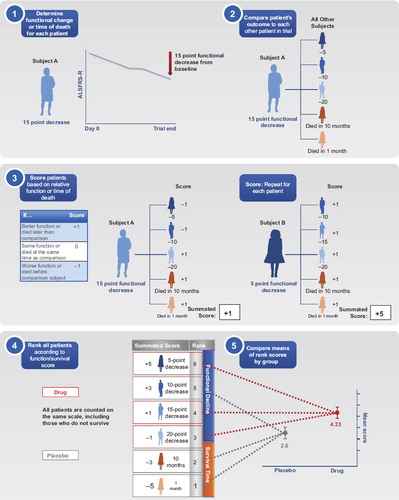
CAFS scoring. To calculate a participant's CAFS, each participant is compared individually to all other participants in the trial. The summary score for each participant is the sum of the comparisons (+ 1, 0, − 1) against all other participants. For each pairwise comparison of patients, the participant who fares better earns a point, and the one who fares worse loses a point (). In the case of a tie, no points are added or subtracted. If both participants die, the one surviving longer fared better; if only one survives then that participant fared better; and if both participants survive, the one with the smaller decline in ALSFRS-R from baseline fared better. If a participant discontinues early, comparison to each other participant uses time to death if the comparator died before the patient's discontinuation time; otherwise, the comparison is based on the last ALSFRS-R time-point available for both participants.
CAFS ranking. Next, participants’ summary scores are ranked (). In general, the ranking has the following characteristics: 1) the first patient who dies will have the lowest score and is ranked the lowest; 2) the last to die is ranked above all others who die; 3) among survivors, the participant with the greatest decline in ALSFRS-R is ranked just above the last patient who died; 4) the surviving participant with the least decline in ALSFRS-R is ranked highest; 5) when comparing ALSFRS-R decline among two survivors, the decline is measured at the longest time that both were followed.
The average rank score is then calculated for each treatment group. A higher mean rank score indicates that participants in that treatment group, on average, fared better.
CAFS analysis. The statistical significance of between-treatment-group differences is tested using a non-parametric statistic called the generalized Gehan-Wilcoxon rank test. If a CAFS analysis indicates a statistically significant effect in the treatment group, function and survival can be analyzed individually to help determine whether the improved outcome for the group was driven by prolonged survival, improved function, or both. If treatment groups differ in baseline characteristics, an analysis of covariance (ANCOVA) can also be performed on the CAFS ranks to adjust for baseline prognostic or demographic factors. These tests can be pre-specified in the analysis plan.
CAFS interpretation. For an individual study participant, the CAFS rank indicates outcome relative to others in the study. The group with the highest mean CAFS rank has, on average, better outcomes during the study. Thus, greater separation in mean CAFS rank between treatment groups implies a larger effect size since a larger proportion of patients in one group had a higher CAFS rank than those in the other group.
The absolute difference between group mean rank scores cannot be compared across studies because it is dependent upon study size. Larger studies will have higher mean ranks because more participants are included in the ranking.
The CAFS analysis has been adopted as the primary endpoint for the phase III EMPOWER trial (ClinicalTrials.gov identifier: NCT01281189), evaluating 150 mg twice daily (b.i.d.) dexpramipexole versus placebo in over 900 subjects. For this study, the pre-specified primary analysis of the CAFS ranks will be conducted after 12 months’ treatment using an ANCOVA model with treatment as a fixed effect and adjusted for the following baseline covariates: ALSFRS-R, duration from symptom onset to the first dose of study treatment, site of onset (bulbar or others), and concomitant use of riluzole. The generalized Gehan-Wilcoxon rank test will be performed as a supportive analysis. Subsequent component analyses of function and survival will be used to determine which parameter(s) drive the overall effect on the CAFS results. The direction and magnitude of the function and survival components of the CAFS will inform the interpretation of the results.
Results
CAFS application in a phase II study
CAFS was used in a two-part phase II study of dexpramipexole for ALS as a pre-specified sensitivity analysis (Citation19). In the second part of this study, 92 participants were randomized to receive 300 mg or 50 mg dexpramipexole as twice-daily divided doses for 24 weeks () (Citation19). Analysis of ALSFRS-R slope favored the 300-mg dose group but may have underestimated the effect due to imbalanced survival. Survival analysis revealed a large (68%) reduction in the mortality hazard rate in the 300-mg dose but this did not reach statistical significance (p = 0.07). The CAFS analysis favored dexpramipexole 300 mg and reached statistical significance (p = 0.046). An exploratory ANCOVA on the CAFS ranks (adjusting for prognostic baseline variables: ALSFRS-R score, time from symptom onset, site of onset, and riluzole use) also favored the dexpramipexole 150-mg b.i.d. group (p = 0.012) (Citation20).
Table II. Dexpramipexole phase II, part 2 study results (Citation19).
CAFS simulation for a phase III study
We ran statistical simulations using the CAFS to evaluate its statistical power in the dexpramipexole phase III EMPOWER study under hypothetical result scenarios (Citation21). The simulations and power calculations were based on the planned sample size (804 patients) and 12 months of follow-up. Hypothetical treatment groups were 150 mg dexpramipexole or placebo b.i.d. We generated 1000 probabilistic datasets for four hypothetical trial outcomes and analyzed the ALSFRS-R slope, survival, and CAFS endpoints.
The simulations were performed using a model derived from placebo group data from the celecoxib study in ALS (Citation22,Citation23). The shared parameter model of Vonesh (Citation24), which has two components, a slopes model for ALSFRS-R and a survival model (Weibull distribution), was fitted to the celecoxib data to obtain parameters to use for the simulation. In this model, the ALSFRS-R slopes and survival models share a parameter, and thus the estimation of treatment effect on slopes is influenced by treatment effect on survival and vice versa.
The estimates derived from the shared parameter model and used for the simulation analysis were as follows:
Placebo ALSFRS-R slope (fixed-effect mean ± standard deviation) from the celecoxib trial was − 1.1 ± 0.84 units/month.
The fixed effect for baseline hazard parameter was modified to give a simulated 1-year mortality rate of 20% (projected rate for the EMPOWER study).
The fixed-effect parameter for treatment effect on ALSFRS-R slope was varied to give active-treatment-group effect sizes ranging from 4% to 24% (i.e. percent reduction in ALSFRS-R slope of the dexpramipexole group relative to the placebo group).
The fixed-effect parameter for treatment effect on mortality was varied to give treatment group hazard rate reductions ranging from 50% to − 15% (i.e. a 15% increase in hazard for mortality).
A fixed-effect parameter for a constant drop-out rate per month was included, which induced 20% drop-outs by 1 year.
Based on these simulations, the hypothetical scenarios (explained in detail below) provide an illustration of the use of CAFS under these conditions (). These simulations demonstrate that CAFS has similar statistical power to an analysis of function, and more accurately reflects the overall treatment effect.
Table III. Power of EMPOWER study based on simulation of various hypothetical scenarios for outcome of ALSFRS-R and survival.
The four hypothetical scenarios were that the drug has: 1) a balanced benefit on both function and survival; 2) moderate functional effect and strong survival effect; 3) moderate functional effect and a) no survival effect or b) negative survival effect; and 4) no effect on function and a beneficial effect on survival (). In the first scenario, where the drug has a balanced benefit on both function and survival (, case 1), the slopes model has slightly more power than the CAFS (95% vs. 91%). However, the CAFS analysis may be more valid than the slopes analysis using the ALSFRS-R data alone, since the CAFS results are not dependent on assumptions associated with parametric models such as non-informative drop-outs and linearity. In the second scenario, where the drug has a disproportionate effect on survival (, cases 2a, b), the CAFS approach provides better power than using the standard slope analysis of ALSFRS-R. The ALSFRS-R decline alone may underestimate the effect of the drug on function as a result of missing data due to deaths. In the third scenario, where the drug moderately affects function and has no effect on mortality (, case 3a), the slopes model has more power than the CAFS analysis and would be preferable if the assumptions of the parametric statistical model can be verified. In addition, in the third scenario, the drug has a negative effect on mortality (, case 3b) and the CAFS analysis has low power; thus it avoids falsely reporting a positive impact of the drug, which reflects the increased risk of death with treatment in that scenario. In the fourth scenario, the drug has a strong effect on mortality and no effect on function (, case 4) and, in such a case, the CAFS lacks power relative to a pure survival analysis.
Discussion and conclusions
The data presented in this manuscript show that the use of the CAFS in ALS trials can capitalize on the established advantages of combined endpoints that permit more than one clinically meaningful outcome to be assessed by means of a single analysis.
Because a drug might have a disproportionate effect on function or survival, a trial designed with either survival or function as the primary outcome could fail because the wrong primary endpoint was chosen. Choosing coprimary endpoints does not mitigate this risk, as significant effects on both outcomes for the trial would need to be observed for the study to be considered positive. Such a requirement could dramatically increase sample size and, therefore, cost and time. However, from a clinical perspective, a novel ALS drug that either reduces functional decline or increases survival would be considered beneficial. Thus, the use of a combined endpoint may be the most appropriate analysis option.
The CAFS offers several major benefits in assessing the effect of a study drug. It provides a balanced analysis of a drug that may have disparate effects on function and survival; if a drug has a benefit on one endpoint (function or survival) and a deleterious effect on the other, the magnitude of treatment group differences in mean CAFS ranks will be appropriately attenuated. It may add statistical power and overcomes problems with missing functional data owing to death or study drop-outs that are not adequately addressed using standard techniques for the analysis of function.
Our hypothetical scenarios demonstrate the overall robustness of the CAFS: 1) it matches the power of individual analyses of function and survival when the treatment effect on each is similar; 2) it provides greater power when there is a strong effect on one component and only a modest effect on the other; 3) it avoids a false-positive result if there is an effect on function but a negative effect on survival. However, combined endpoints such as the CAFS do present a few new challenges (Citation9,Citation10). First, because the CAFS is a non-parametric rank analysis, the magnitude of the difference in CAFS scores between treatment groups cannot be directly compared across trials. Secondly, analyses of the component data for function and survival are required to understand the specific clinical effects of the study drug.
Unlike combined endpoints for time-to-event analyses where the component factors are equally weighted as ‘events’, i.e. cardiovascular trials where death, myocardial infarction, and some other risk factor are grouped together, the CAFS test specifically weights mortality as the most clinically important outcome.
In conclusion, the CAFS is an appropriate, informative, and powerful endpoint for ALS studies. It can be interpreted as a combined analysis of death and functional change or as an analysis of functional outcome that is appropriately corrected for mortality in ALS.
Acknowledgements
Medical writing assistance was provided by Aruna Seth of UBC Scientific Solutions and funded by Biogen Idec, Inc.
Declaration of interests: J. D. Berry has served as a consultant to Biogen Idec, Inc. and as a paid speaker for Oakstone Publishing. He is a site co-investigator for the EMPOWER study. He receives research support from the Muscular Dystrophy Association and the ALS Therapy Alliance. R. Miller served on a Biogen Idec advisory board. M. E. Cudkowicz is the principal investigator of the ongoing EMPOWER study and receives a grant from Biogen Idec, Inc. for this role. In the past 2 years, she has served as a consultant for Teva Pharmaceuticals, Millenium, and Trophos (Data and Safety Monitoring Board) and has served on an advisory board for Biogen Idec, Inc. L. H. van den Berg has served on a Biogen Idec, Inc. advisory board and has received travel grants from Baxter. D. A. Kerr and Y. Dong are employees of Biogen Idec, Inc. E. Ingersoll and D. Archibald are employees of Knopp Biosciences LLC.
References
- Moore DH, Katz JS, Miller RG. A review of clinical trial designs in amyotrophic lateral sclerosis. Neurogen Dis Manage. 2011;1:481–90.
- Shefner JM. Designing clinical trials in amyotrophic lateral sclerosis. Phys Med Rehabil Clin N Am. 2008;19:495–508.
- Berry JD, Cudkowicz ME. New considerations in the design of clinical trials for amyotrophic lateral sclerosis. Clin Investig. 2011;1:1375–89.
- Gordon PH, Corcia P, Lacomblez L, Pochigaeva K, Abitbol JL, Cudkowicz M, . Defining survival as an outcome measure in amyotrophic lateral sclerosis. Arch Neurol. 2009;66:758–61.
- Kaufmann P, Levy G, Thompson JL, Delbene ML, Battista V, Gordon PH, . The ALSFRS-R predicts survival time in an ALS clinic population. Neurology. 2005;64:38–43.
- Bhatt JM, Gordon PH. Current clinical trials in amyotrophic lateral sclerosis. Expert Opin Investig Drugs. 2007;16:1197–207.
- Bensimon G. Survival endpoint: pro. Amyotroph Lateral Scler. 2002;3:S35–6.
- European Medicines Agency. European Medicines Agency guidelines. [27 August 2012]. Available from: http://www.ema.europa.eu/ema/.
- Wittkop L, Smith C, Fox Z, Sabin C, Richert L, Aboulker JP, . NEAT-WP4. Methodological issues in the use of composite endpoints in clinical trials: examples from the HIV field. Clin Trials. 2010;7:19–35.
- Ferreira-González I, Permanyer-Miralda G, Busse JW, Bryant DM, Montori VM, Alonso-Coello P, . Methodologic discussions for using and interpreting composite endpoints are limited, but still identify major concerns. J Clin Epidemiol. 2007;60:651–7.
- Freemantle N, Calvert M. Weighing the pros and cons for composite outcomes in clinical trials. J Clin Epidemiol. 2007;60:658–9.
- O’Brien PC. Procedures for comparing samples with multiple endpoints. Biometrics. 1984;40:1079–87.
- Wong WK, Furst DE, Clements PJ, Streisand JB. Assessing disease progression using a composite endpoint. Stat Methods Med Res. 2007;16:31–49.
- Lim E, Brown A, Helmy A, Mussa S, Altman DG. Composite outcomes in cardiovascular research: a survey of randomized trials. Ann Intern Med. 2008;149:612–7.
- Finkelstein DM, Schoenfeld DA. Combining mortality and longitudinal measures in clinical trials. Stat Med. 1999;18:1341–54.
- Henderson R, Diggle P, Dobson A. Joint modeling of longitudinal measurements and event time data. Biostatistics. 2000;1:465–80.
- Elashoff RM, Li G, Li N. An approach to joint analysis of longitudinal measurements and competing risks failure time data. Stat Med. 2007;26:2813–35.
- Moye LA, Davis BR, Hawkins CM. Analysis of a clinical trial involving a combined mortality and adherence dependent interval censored endpoint. Stat Med. 1992;11:1705–17.
- Cudkowicz M, Bozik ME, Ingersoll EW, Miller R, Mitsumoto H, Shefner J, . The effects of dexpramipexole (KNS-760704) in individuals with amyotrophic lateral sclerosis. Nat Med. 2011;17:1652–6.
- Rudnicki SA, Berry JD, Ingersoll E, Archibald D, Cudkowicz ME, Kerr DA, . Dexpramipexole effects on functional decline and survival in subjects with amyotrophic lateral sclerosis in a phase II study: subgroup analysis of demographic and clinical characteristics. Amyotroph Lateral Scler. 2013;14:44–51.
- Archibald D, Ingersoll EW, Mather JL, Schoenfeld D, Kerr D, Dong Y, . Statistical modeling to illustrate the contribution and effects of differential mortality and functional change on joint rank test outcomes in ALS. Amyotroph Lateral Scler. 2011;12:107.
- Cudkowicz ME, Shefner JM, Schoenfeld DA, Zhang H, Andreasson KI, Rothstein JD, . Trial of celecoxib in amyotrophic lateral sclerosis. Ann Neurol. 2006;60:22–31.
- Healy BC, Schoenfeld DA. Comparison of analysis approaches for phase III clinical trials in amyotrophic lateral sclerosis. Muscle Nerve. 2012;46:506–11.
- Vonesh EF, Greene T, Schluchter MD. Shared parameter models for the joint analysis of longitudinal data and event times. Stat Med. 2006;25:143–63.
- Tseng C-H, Wong WK.Schluchter MD. Analysis of a composite endpoint with longitudinal and time-to-event data. Stat Med. 2011;30:1018–27.