Abstract
Objectives:
To illustrate how claims data can be used to (1) develop outcome scores that predict response to a traditional treatment and (2) estimate the economic impact of individualized assignment to a newer treatment based on the outcome score. An example application is based on two treatments for attention deficit hyperactivity disorder (ADHD): osmotic-release oral system methylphenidate (OROS-MPH) and lisdexamfetamine dimesylate (LDX).
Methods:
Adolescents with ADHD initiating OROS-MPH (n = 6320) or LDX (n = 6394) were selected from the MarketScan claims database. A model was developed for predicting risk of switching/augmentation with OROS-MPH using multiple baseline characteristics. The model was applied to an independent sample to stratify patients by their predicted risk and, within each stratum, risk of switching/augmentation and ADHD-related total costs were compared between OROS-MPH and LDX patients using inverse probability of treatment weighting.
Results:
The prediction model resulted in substantial stratification, showing risk of switching/augmentation with OROS-MPH ranging from 11.3–42.1%. In the two strata where OROS-MPH had highest risk of switching/augmentation, LDX had significantly lower risk of switching/augmentation than OROS-MPH (by 7.0–8.2%) and lower ADHD-related annual total costs (by $264–$625 per patient).
Limitations:
The current study has used the risk of switching/augmentation as a proxy measure for treatment efficacy to establish the prediction model. Future research using a clinical measure for ADHD symptoms is warranted to verify the findings.
Conclusions:
Combining multiple patient characteristics into a predicted score for treatment outcomes with a traditional treatment can help identify subgroups of patients who benefit most from a new treatment. In this analysis, ADHD patients with a high predicted score for switching/augmentation with OROS-MPH had a lower rate of switching/augmentation with LDX. Assigning OROS-MPH and LDX treatments based on the predicted scores that are heterogeneous in a patient population may help improve clinical outcomes and the cost-effectiveness of care.
Introduction
Comparative effectiveness research has traditionally focused on the average effects of treatments in relatively broad patient populations. However, patients often have heterogeneous responses to therapies. As there is generally no one-size-fits-all treatment choice, identifying and assigning treatments to subgroups of patients who are most likely to benefit from them may improve effectiveness and reduce costsCitation1–3. The generation and application of such evidence is often referred to as individualized, personalized, or, more recently, stratified medicine, and holds the promise of improving health outcomes while limiting healthcare costsCitation1,Citation2,Citation4–7.
The role of individualized medicine in clinical practice has become increasingly important. Many of the most advanced examples come from oncology, which has benefitted from a wealth of molecular markers. For example, cetuximab and panitumumab are only recommended for metastatic colorectal cancer patients with the wild-type KRAS geneCitation8,Citation9. Trastuzumab should be used only in HER2-positive breast cancer patientsCitation10,Citation11. Identification of heterogeneous patient subgroups can help to tailor treatment selection in clinical practiceCitation9. However, the conventional methods for identifying patient subgroups, i.e., pre-specified or post-hoc subgroup analyses, face the dilemma of a priori vs post-hoc specificationCitation12–15. Pre-specified subgroup analysis provides statistical rigor but is restricted by the number of subgroups to be tested and offers limited learning from the data because of the limitations on the number of characteristics that could be considered. Although post-hoc exploratory subgroup analysis provides an opportunity to learn from the data, the multiple testing involved makes it prone to low statistical powerCitation12,Citation14,Citation16.
This dilemma has been addressed by a personalized medicine approach, which analyzes post-hoc subgroups defined by a risk score combining multiple patient characteristics using clinical trial dataCitation5–7. The method systematically selects and combines multiple baseline covariates to define subgroups through a regression model. Compared to separate post-hoc subgroups defined by single characteristics, this regression-based approach limits the potential for selecting patient characteristics based on the researchers’ subjective perceptions and the loss of power due to multiple testing for many characteristics. Although a model is used to combine characteristics, the evaluation of the model and the assessment of its performance for patient stratification are non-parametric. Once established, such models could be used to guide treatment selection for individual patients. The value of assigning treatments based on these models could be evaluated by plotting a selection impact curve that estimates the population response as a function of risk scores estimated from the models and comparing that to the existing practiceCitation4,Citation17.
In this study, we have adopted the methods for personalized medicine developed by Cai and colleaguesCitation5–7, and the selection impact curve developed by Song and PepeCitation17 and applied them to the real-world administrative claims data using attention deficit hyperactivity disorder (ADHD) as an example.
ADHD is a common psychiatric disorder with an estimated prevalence among adolescents of 6–10% in the US, 3–9% in the UK, and ∼3% worldwideCitation3,Citation18,Citation19. Psychostimulants, mainly composed of methylphenidate and amphetamine, are recommended as first-line medications for ADHDCitation20,Citation21. Concerta® (Osmotic-release oral system methylphenidate: OROS-MPH) and Vyvanse® (lisdexamfetamine dimesylate: LDX, an amphetamine) are the two most commonly used long-acting drugs from each classCitation22. In the US, OROS-MPH was launched in 2000, and LDX was launched in 2007, when OROS-MPH was considered the market leader for long-acting methylphenidate formulations. The current study aims to identify subgroups of adolescents with ADHD who are most likely to benefit from LDX or OROS-MPH using a stratified medicine method, and to compare the impact of assigning treatment based on stratified medicine vs the real-world practice (the observed treatments with LDX and OROS-MPH in claims) on two outcomes: risk of switching/augmentation and ADHD-related total costs. To better illustrate our method, we also compared the results from stratified medicine with those from pre-defined subgroups analyses.
Methods
Data source
The study was conducted using the Thomson Reuters MarketScan® Commercial Claims and Encounter Research database (2007–2009), a data source from ∼100 payers in all census regions, with 20 million individuals annuallyCitation23. The database contains information on enrollment, medical claims, and pharmacy claims.
Study cohorts
Adolescents (aged 13–17) initiating LDX or OROS-MPH on or after 06/29/2007 (the launch of LDX in the US) were selected. The earliest prescription date of LDX or OROS-MPH was defined as the index date. Patients were excluded if they filled other prescriptions for ADHD on the index date. Patients were further required to be continuously enrolled in the healthcare plan for at least 6 months before (baseline period) and 12 months after (study period) the index-date and have ≥1 ADHD diagnosis (ICD-9 code of 314.0 x)Citation24 during this 18-month period. Patients were categorized into one of two study cohorts based on the drug received on the index date: OROS-MPH or LDX. In addition, patients were classified into two pre-defined sub-populations based on whether they received any other ADHD medications during the baseline period.
Baseline variables and study outcomes
Baseline variables were assessed during the 6-month pre-index period and included age, gender, baseline use of ADHD medications, comorbidities, as well as all-cause and ADHD-related (i.e., services associated with ADHD diagnosis code of 314.0 x) medical resource utilization ().
Study outcomes, risk of switching/augmentation (used as a proxy measure for treatment efficacy), and ADHD-related total costs were measured during the 12-month study period. Switching was defined as use of another ADHD medication within 30 days of discontinuing the index drug; augmentation was defined as use of another ADHD medication while on the index drug. ADHD-related total costs, based on reimbursements from the managed care plans to providers, included costs for medical services associated with ADHD diagnoses (ICD-9 code of 314.0 x) and ADHD prescription drug () costs. All costs were estimated from a third-party payer’s perspective and were inflation-adjusted to 2009 dollars.
Statistical methods
The prediction model described below was developed using R software version 2.11.1 (http://cran.r-project.org/), and the remainder of the analyses were performed using SAS® 9.2 (SAS Institute, Inc., Cary, NC). A result was considered statistically significant if the p-value was less than 0.05 (based on two-tailed tests).
Comparisons of study outcomes in the overall population and in the pre-defined sub-populations
Risk of switching/augmentation and ADHD-related total costs were estimated and compared between the OROS-MPH vs LDX cohorts in the overall population using weighted Chi-square and weighted t-tests, respectively, with inverse probability of treatment weighting (IPTW) to adjust for potential confoundingCitation25–28. The probability of receiving OROS-MPH and LDX was estimated using a logistic regression with baseline characteristics as the independent variables. Baseline characteristics were re-evaluated after IPTW to assess the balance of the two study cohorts. Within each patient sub-population, study outcomes were compared between OROS-MPH and LDX using IPTW. p-values for trends across the pre-defined sub-populations were estimated using generalized linear models with binomial distribution and normal distributions for risk of switching/augmentation and ADHD-related costs, respectively, adjusting for baseline characteristics using IPTW and index drugs.
Stratified medicine
A stratified medicine approach was used to identify patient subgroups with different risk of switching/augmentation if treated with OROS-MPH vs LDX (Appendix 2). First, OROS-MPH patients were randomly selected to create the training sample, which was comprised of half of the OROS-MPH patients; the remaining OROS-MPH patients and all of the LDX patients formed the validation sample. Based on the training sample, a prediction model was established to estimate risk of switching/augmentation with OROS-MPH including all baseline variables as potential predictors. The Least Absolute Shrinkage and Selection Operator (LASSO)Citation29 method with a 10-fold cross-validation was used to select the best model and generate coefficient estimates for the retained variables. The model was set up to predict the risk of switching/augmentation associated with OROS-MPH because this treatment was approved earlier and was considered the more established treatment paradigm. Next, the model was applied to the validation sample to generate scores predicting risk of switching/augmentation associated with OROS-MPH; patients were then stratified into quartiles based on those scores. The predicted risk was compared to the observed risk associated with OROS-MPH to validate the model. Within each stratum, the observed risk of switching/augmentation and ADHD-related total costs were compared between LDX and OROS-MPH using weighted Chi-square tests or weighted t-tests with IPTW to adjust for baseline differences. A more conservative assessment, accounting for the multiple comparisons across strata, used the Holm method to control the familywise type I error rateCitation30. Lastly, the p-values for trend across quartiles were estimated based on generalized linear models with binomial distribution and normal distributions for risk of switching/augmentation and ADHD-related costs, respectively, adjusting for baseline characteristics using IPTW and index drugs.
Treatment allocation
The ADHD-related total costs (based on 2009 costs) and risk of switching/augmentation were estimated and compared among different treatment allocation strategies:
Real-world practice: the observed outcomes without any adjustment.
Pre-defined sub-populations: weighted mean outcomes estimated assuming that LDX was assigned to the pre-defined sub-population where its benefit vs OROS-MPH was more pronounced, while OROS-MPH was assigned to the other sub-population.
Stratified medicine: weighted mean outcomes estimated assuming that a certain proportion (0%, 25%, 50%, 75%, or 100%) of patients with the lowest predicted risk of switching/augmentation with OROS-MPH were assigned to that treatment; the remainder were assigned to LDX.
In addition, a hypothetical scenario of reducing the pharmacy costs of OROS-MPH by 20% with the remaining costs unchanged was also considered to simulate the potential price decrease due to the recent availability (05/2011) of generic OROS-MPH.
Results
Description of study cohorts
A total of 12,714 adolescents with ADHD who initiated treatment with OROS-MPH (n = 6320) and LDX (n = 6394) were identified. At baseline, patients in the LDX cohort were significantly more likely to use ADHD medications and have all-cause and ADHD-related outpatient and psychotherapy visits ().
Table 1. Baseline characteristics for adolescents.
Comparisons of study outcomes in the overall population and the pre-defined sub-populations
In the overall population, compared with OROS-MPH, LDX was associated with a 4.5% lower adjusted risk of switching/augmentation (18.4% vs 22.9%; p < 0.0001) and $116 lower adjusted annual ADHD-related total costs per patient ($1456 vs. $1572; p < 0.0001). LDX was also associated with significantly lower adjusted risk of switching/augmentation in the pre-defined sub-populations with and without prior treatment (4.6% and 3.4%, respectively) ().
Figure 1. Comparison of study outcomes for LDX vs OROS-MPH†. (a) Overall population‡; (b) Pre-defined sub-populations.‡,$. †Study outcomes were measured over the 12-month post-index period. ‡Mean and p-values were estimated using weighted t-tests for continuous variables. Tests adjusted for baseline characteristics by using IPTW. $p-values for trends across the pre-defined sub-populations for risk of switching/augmentation and ADHD-related costs were estimated based on generalized linear models with binomial distribution and normal distributions, respectively, adjusting for baseline characteristics using IPTW and index drugs. Both p-values were <0.001. *p-value significant at α=0.05.
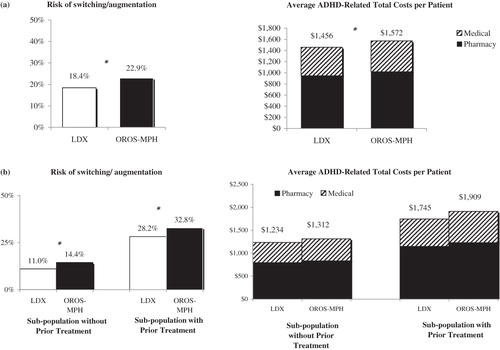
Stratified medicine
Baseline variables retained in the prediction models by LASSO are presented in . Use of ADHD medications, presence of comorbidity, and frequent use of medical services at baseline were associated with a higher risk of switching/augmentation with OROS-MPH treatment.
Table 2. Prediction model for switching/augmentation on OROS-MPH.
Stratifying patients into quartiles based on the predicted risk of switching/augmentation with OROS-MPH resulted in substantial heterogeneity in the observed risk associated with OROS-MPH, ranging from 11.3–42.1% across quartiles. The observed risks with OROS-MPH were similar to the predicted values in each quartile, and the p-value from a Hosmer-Lemeshow test was greater than 0.05, an indication of good predictive power ().
Table 3. Comparison of outcomes for LDX vs OROS-MPH. Observed and predicted risk of switching/augmentation.
In the quartiles with higher risk of switching/augmentation with OROS-MPH, the risk associated with LDX was lower than OROS-MPH (by 8.2% in quartile 3, p = 0.0263 and 0.0526 with and without adjustment, and 7.0% in quartile 4, p = 0.0139 and 0.0456 with and without adjustment). ADHD-related costs were also lower comparing LDX vs OROS-MPH in these quartiles (by $265 per patient per year in quartile 3, p = 0.0227 and 0.0908 with and without adjustment, and $625 in quartile 4, p = 0.0394 and 0.1182 with and without adjustment) (). Based on the Whole Acquisition Costs, a 30-day supply would cost $572 for OROS-MPH (54 mg) and $447 for LDXCitation31. The estimated mean costs of index drug in the claims analyses were $636–$845 for OROS-MPH and $626-$873 for LDX across different quartiles ().
Table 4. Comparison of outcomes for LDX vs OROS-MPH. ADHD-related costs.
Treatment allocation
Real-world practice was associated with a lower risk of switching/augmentation (19.9% vs 20.2%) and lower ADHD-related total costs ($1481 vs $1495 per patient per year) than treatment allocation based on the pre-defined sub-populations (i.e., assigning patients using baseline ADHD medications to LDX and patients without baseline medications to OROS-MPH). Among all the allocations based on stratified medicine, treating all patients with LDX was the most cost-effective option, with a risk of switching/augmentation of 18.3% and $1449 ADHD-related total costs per patient (). In addition, it was also more cost-effective compared to the real-world practice and treatment allocation based on the pre-defined sub-populations.
Figure 2. Outcomes achievable with individualized treatment with LDX and OROS-MPH. LDX, lisdexamfetamine dimesylate; OROS-MPH, osmotic-release oral system methylphenidate; ADHD, attention deficit hyperactivity disorder. The squares connected by the dashed black line illustrate different ways of allocating patients based on the stratified medicine approach; black diamond illustrates treatment allocation by pre-defined sub-populations (i.e., sub-populations with and without prior ADHD treatments); white diamond represents the observed real-world practice.
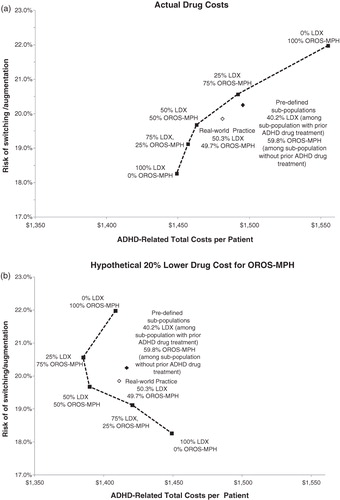
In the hypothetical case in which the pharmacy costs of OROS-MPH were reduced by 20%, the treatment strategy with 50% LDX and 50% OROS-MPH resulted in lower ADHD-related total costs per patient ($1390 vs $1411) and a lower risk of switching/augmentation (19.7% vs 19.9 %) compared to the real-world practice ().
Discussion
Recently developed methods for personalized medicine have been successfully applied to clinical trial data to identify patients who benefit most from a particular treatmentCitation5–7,Citation32. We have shown that this method could be adapted to real-world administrative claims data. In the example of ADHD, this approach identified patient subgroups with heterogeneous responses to OROS-MPH and LDX. This is also the first study applying this methodology to psychiatric disorders. When multiple baseline characteristics were combined to stratify patients, a dramatic stratification was produced, with risk of switching/augmentation on OROS-MPH ranging from 11.3–42.1%. Patients who were most likely to switch or augment with OROS-MPH showed significantly lower risk of such events with LDX (by 7–8%), a greater difference than that observed in the overall population and pre-defined sub-populations (∼4%).
Stratified medicine can help inform decision-makers in their attempts to optimize patient care by making tradeoffs between costs and effectiveness. In the current example, real-world treatment assignments were not optimal in terms of either effectiveness or costs compared to treatment allocations based on risk stratification. The value of stratified medicine is exemplified in the hypothetical scenario where the pharmacy costs of OROS-MPH decreased by 20% (a possible scenario, given that generic OROS-MPH is already available). Under this assumption, assigning 50% of the patients who were predicated to have highest risk of switching/augmentation with OROS-MPH to LDX and the rest to OROS-MPH was associated with better treatment outcomes and lower ADHD-related costs than real-world practice.
The stratified medicine method described here could be used to increase the application of real-world observational databases to future comparative effectiveness research intended to optimize patient care. The method could also use measures other than augmentation or switching, e.g., hospitalization or composite benefit-risk measures, as study outcomes. In addition, as alternatives, models could be developed to directly estimate risk scores for differences in responses between OROS-MPH and LDX, or simply for responses to LDX. However, given the established nature of OROS-MPH as a treatment and the potential correlations between responses to the two treatments, it is more informative to stratify patients based on responses to OROS-MPH. Future studies can explore different modeling strategies.
The results of stratified medicine will be beneficial to a variety of healthcare professionals. The importance of identifying and analyzing outcomes heterogeneity in patient subgroups has already been recognized by some healthcare payers and technology assessment authorities such as the National Institute for Health and Clinical Excellence (NICE) and France’s Transparency CommissionCitation2,Citation33. For example, a recent briefing paper from NICE emphasizes the importance of including subgroups in cost-effectiveness analyses and decision-makingCitation2. Payers could optimize the value of healthcare by allocating treatments based on the stratified medicine results to improve outcomes and reduce costs in different therapeutic areas. Such an approach could help them focus further research efforts on cost-effective interventions based on personalized treatments. The findings from stratified medicine could also be formatted into index cards or computer-based programs to assist physicians and patients in selecting the most beneficial treatments. However, before applying the findings from an observational study of stratified medicine in real-world decision-making, an important next step is to validate these findings using independent data.
The study is subject to certain limitations. First, as in any observational study, there may be unobserved confounding between the two study cohorts. Second, because the claims data have limited measures of clinical symptoms, the risk of switching/augmentation was used as the treatment outcome here. Future research using a real-world database with rich clinical information (e.g., ADHD symptoms relief) is warranted to verify our findings. Since stratified medicine involves the interpretation of treatment effects across multiple strata, adjustment for multiple comparisons can provide a more conservative assessment of statistical significance in exploratory analyses. In the present study, adjustment for multiple testing using the Holm procedure identified fewer statistically significant outcome differences between treatments within strata. Lastly, we used quartiles as an example to illustrate patient stratification. Future studies could use smoothingCitation5,Citation7,Citation34, which can avoid stratification and treatment allocation by a defined number of subgroups. However, because treatments in claims are not randomized, it is important that the adjustment for selection bias be made, or at least assessed, locally, rather than relying on a single propensity score model fit to the full data. Such two-level smoothing, for the localized propensity score and for the localized assessment of IPTW adjusted treatment effects, was beyond the scope of the current study.
Conclusion
The individualization of medical care plays an increasing role in informing treatment selection to improve outcomes and reduce costs. We have illustrated how stratified medicine, through the targeted use of new treatments based on risk stratification of patients using traditional treatments, can be informed by analyses of real-world claims data using ADHD as an example. In the example application, risk stratification identified patients with heterogeneous responses to a traditional agent (OROS-MPH) and indicated how targeted use of a newer agent (LDX) could potentially improve treatment outcomes while reducing the costs compared to current practice.
Transparency
Declaration of funding
This study was funded by Shire Development LLC., Wayne, PA, USA.
Declaration of financial/other interests
J.E.S., H.Y., K.P., K.A.B., J.X., and E.Q.W. have disclosed that they are employees of Analysis Group, Inc., a company that received funding from Shire Development LLC to conduct this study. M.H.E., J.S., and P.H. have disclosed that they are employees of Shire Development LLC. Shire develops and markets psychiatric drugs including treatments for ADHD.
Acknowledgments
No assistance in the preparation of this article is to be declared.
References
- Cortese DA. A vision of individualized medicine in the context of global health. Clin Pharmacol Ther 2007;82:491-3
- National Institute for Health and Clinical Excellence. Briefing paper for methods review workshop on identifying sub-groups and exploring heterogeneity. London: National Institute for Health and Clinical Excellence, 2007preprint
- The National Collaborating Centre for Mental Health. Attention deficit hyperactivity disorder - Diagnosis and management of ADHD in children, young people and adults. London: NICE clinical guideline 72, 2008
- Janes H, Pepe MS, Bossuyt PM, et al. Measuring the performance of markers for guiding treatment decisions. Ann Intern Med 2011;154:253-9
- Tian L, Cai T, Wei LJ. Identifying subjects who benefit from additional information for better prediction of the outcome variables. Biometrics 2009;65:894-902
- Zhao L, Cai T, Tian L, et al. Stratifying subjects for treatment selection with censored event time data from a comparative study. Harvard University Biostatistics Working Paper Series. 2010; Working Paper 122. http://biostats.bepress.com/harvardbiostat/paper122
- Cai T, Tian L, Wong PH, et al. Analysis of randomized comparative clinical trial data for personalized treatment selections. Biostatistics 2011;12:270-82
- Karapetis CS, Khambata-Ford S, Jonker DJ, et al. K-ras mutations and benefit from cetuximab in advanced colorectal cancer. N Engl J Med 2008;359:1757-65
- Dahabreh IJ, Terasawa T, Castaldi PJ, et al. Systematic review: anti-epidermal growth factor receptor treatment effect modification by KRAS mutations in advanced colorectal cancer. Ann Intern Med 2011;154:37-49
- Bilous M, Dowsett M, Hanna W, et al. Current perspectives on HER2 testing: a review of national testing guidelines. Mod Pathol 2003;16:173-82
- Hanna W. Testing for HER2 status. Oncology 2001;61(2 Suppl):22-30
- Lagakos SW. The challenge of subgroup analyses–reporting without distorting. N Engl J Med 2006;354:1667-9
- Peto R. Misleading subgroup analyses in GISSI. Am J Cardiol 1990;66:771-2
- Pocock SJ, Assmann SE, Enos LE, et al. Subgroup analysis, covariate adjustment and baseline comparisons in clinical trial reporting: current practice and problems. Stat Med 2002;21:2917-30
- Assmann SF, Pocock SJ, Enos LE, et al. Subgroup analysis and other (mis)uses of baseline data in clinical trials. Lancet 2000;355:1064-9
- Hernandez AV, Boersma E, Murray GD, et al. Subgroup analyses in therapeutic cardiovascular clinical trials: are most of them misleading? Am Heart J 2006;151:257-64
- Song X, Pepe MS. Evaluating markers for selecting a patient's treatment. Biometrics 2004;60:874-83
- Pastor PN, Reuben CA. Diagnosed attention deficit hyperactivity disorder and learning disability: United States, 2004-2006. Vital Health Stat 2008;10:1-14
- Polanczyk G, de Lima MS, Horta BL, et al. The worldwide prevalence of ADHD: a systematic review and metaregression analysis. Am J Psychiatry 2007;164:942-8
- Heal DJ, Smith SL, Findling RL. ADHD: current and future therapeutics. Curr Top Behav Neurosci 2012;9:361-90
- Wolraich ML, Wibbelsman CJ, Brown TE, et al. Attention-deficit/hyperactivity disorder among adolescents: a review of the diagnosis, treatment, and clinical implications. Pediatrics 2005;115:1734-46
- Market Watch -ADHD Market. Focus on brands. 2011. http://www.pm360online.com/MarketWatch_1111. Accessed December 19, 2011
- Thomson Reuters MarketScan®. Commercial claims and encounter research database. Cambridge, MA: Cambridge MTHC, 2011
- ICD9Data.com. Attention deficit disorder of childhood. 2012. http://www.icd9data.com/2012/Volume1/290-319/300-316/314/default.htm. Accessed April 20, 2012
- Lunceford JK, Davidian M. Stratification and weighting via the propensity score in estimation of causal treatment effects: a comparative study. Stat Med 2004;23:2937-60
- Rosenbaum PR, Rubin DB. Constructing a control-group using multivariate matched sampling methods that incorporate the propensity score. Am Stat. 1985;39:33-8
- Polsky D, Mandelblatt JS, Weeks JC, et al. Economic evaluation of breast cancer treatment: considering the value of patient choice. J Clin Oncol 2003;21:1139-46
- Nishida Y, Takahashi Y, Nakayama T, et al. Comparative effect of olmesartan and candesartan on lipid metabolism and renal function in patients with hypertension: a retrospective observational study. Cardiovasc Diabetol 2011;10:74
- Tibshirani R. Regression shrinkage and selection via the Lasso. J Roy Stat Soc B (Meth) 1996;58:267-88
- Holm S. A simple sequentially rejective multiple test procedure. Scand J Statist 1979;6:65-70
- ReadyPrice®. Greenwood Village, CO: MicroMedex Division, Thomson Health Care, 2011
- Dorresteijn JAN, Visseren FLJ, Ridker PM, et al. Estimating treatment effects for individual patients based on the results of randomised clinical trials. BMJ 2011;Oct 3:343:d5888
- de Sahb-Berkovitch R, Woronoff-Lemsi MC, Molimard M. Assessing cancer drugs for reimbursement: methodology, relationship between effect size and medical need. Therapie 2010;65:373–7, 367-72
- Uno H, Cai T, Tian L, Wei LJ. Graphical procedures for evaluating overall and subject-specific incremental values from new predictors with censored event time data. Biometrics. 2011 Dec;67(4):1389-96
Appendix 1. Baseline medications and comorbidities
Appendix 2. Diagram of stratified medicine method

LDX, lisdexamfetamine dimesylate; OROS-MPH, osmotic-release oral system methylphenidate