ABSTRACT
Inhalation rate is an essential factor for determining the inhaled dose of air pollutants. Here, accelerometers were used to develop regression equations for predicting the minute ventilation rate () to estimate the daily inhalation rate in young children. Body acceleration and heart rate were measured in 29 Japanese preschool children (6 yr of age) during nine different levels of activities (lying down, sitting, standing, playing with plastic bricks, walking, building with blocks, climbing stairs, ball tossing, and running) using the Actical omnidirectional accelerometer, the ActivTracer triaxial accelerometer, and a heart rate monitor. Measurements were calibrated against the
measured by the Douglas bag method. ActivTracer accelerometer measurements gave a strong correlation with
(Pearson's r = 0.913), which was marginally stronger than that for the Actical counts (r = 0.886) and comparable to the correlation between heart rate and logarithmic
(r = 0.909). According to the linear regression equation, the
for lying down, sitting, standing, playing with plastic bricks, walking, and running was overestimated by 14–60% by the Actical and by 14–37% by the ActivTracer. By comparison, for building with blocks, climbing stairs, and ball tossing, the
was underestimated by 19–23% by the Actical and by 13–18% by the ActivTracer. When these three activities were excluded, a stronger correlation was found between the
and ActivTracer measurements (r = 0.949); this correlation was 0.761 for the three excluded activities. Discriminant analysis showed that the ratio between vertical and horizontal acceleration obtained by the ActivTracer could discriminate walking from building with blocks, climbing stairs, and ball tossing with a sensitivity of 75%. The error in estimating
was considerably improved for the ActivTracer measurements by the use of two regression equations developed for each type of activity.
Respiratory ventilation rate is an essential factor for assessing the health risk from air pollutants because it allows the dose of air pollutants delivered to the respiratory system to be determined. When establishing standards or criteria related to the management of health risks from hazardous environmental pollutants, the particular vulnerability of young children to environmental pollutants and their pattern of exposure should be considered. However, there are limited data on the respiratory ventilation rate of young children in daily life. To help address this problem, accelerometers were used to develop regression equations for predicting the to estimate the daily inhalation rate in young children.
INTRODUCTION
Respiratory ventilation rate is an essential factor for determining the daily inhaled dose of air pollutants, which is important information for the establishment of health criteria or guideline values for air quality. In recent years, the particular vulnerability of children to environmental pollutants and age-related differences in exposure have become a concern in health risk assessment and management.Citation1–3 In Japan, an inhalation rate of 15 m3 · day−1 for a 50-kg adult is commonly used to estimate the daily inhaled dose of air pollutants.Citation4 However, no standard inhalation rate value has been established for children. To adequately assess adverse health risks to children, the inhalation rate of young children in daily life needs to be determined.
The doubly labeled water (DLW) method and the time-activity-ventilation method are experimental approaches that have been used to estimate daily inhalation rates during the daily activities of free-living individuals.Citation5–11 The DLW method is the most accurate method to measure the average total energy expenditure (EE) of people over several days and is based on the rates of disappearance of a dose of water containing the stable isotopes 2H and 18O. Although the DLW approach provides an accurate estimation of the daily inhalation rate, it is an expensive method and requires the collection of urine samples for 2–3 weeks. By comparison, the time-activity-ventilation method estimates the volume of air inhaled by a person over a defined period of time as a time-weighted average of the minute ventilation rate (V E) for each level of activity during the period. The daily inhalation rate using this approach is expressed by the following equation:
Another limitation of the time-activity-ventilation method is the availability of suitable techniques to obtain the intensity and duration of physical activity of young children during daily life. Self-report activity diaries and heart rate monitoring are the traditional tools used to determine the level of physical activity for estimating the of free-living people. The disadvantages of self-reporting are that it tends to be subjective and is not practical for the assessment of young children, who are unable to accurately self-estimate and record their physical activity levels.Citation13–15 Even if an observer records the activities of children, it is still not possible to accurately measure the intensity and duration of the activities of children, which can vary within a few seconds.Citation16,Citation17 Heart rate is a valid predictor of
Citation18, but its limitations include interruption of heart rate measurement by electrode displacement and discomfort from the electrodes, which can reduce the compliance of children and their parents with the study requirements.Citation15 To overcome these issues in monitoring the activity of children, an accelerometer could be a practical alternative for measuring the intensity, frequency, and duration of physical activity of free-living people. The advantages of an accelerometer-based approach are that it is noninvasive, it is not affected by the wearing of clothes, and it can provide data on a second time scale over long periods of time without interfering with normal activities. Many studies have examined the reliability and validity of these devices in children under laboratory and field conditions and determined that they provide valid measures of EE and oxygen (O2) consumption in children.Citation19–31
The primary goal of this study was to develop a regression equation to predict from accelerometer measurements to estimate the daily inhalation rate in young children. First evaluated was the validity of accelerometer measurements to predict
by calibrating the Actical omniaxial accelerometer and the ActivTracer triaxial accelerometer against the
measured at different levels of activity by the Douglas bag method. The performance of the accelerometers was also evaluated by comparing the accelerometer measurements with heart rate.
METHODS
Participants and Study Design
The study presented here was conducted from December 2006 through March 2007. The study population comprised 29 Japanese preschool children (16 boys and 13 girls) 6 yr of age (mean age, 6.5 ± 0.2 years) who were recruited from a single kindergarten class thanks to the cooperation of the administrative personnel. The children's mean height was 115.4 cm (SD, 5 cm; range, 103.4–124.6 cm) and mean body weight was 20.6 kg (SD, 2.4 kg; range, 17–26.3 kg); these data were obtained at the start of the experiment. The V
E associated with nine different levels of physical activity were measured in a laboratory setting. Body acceleration and heart rate were measured by using activity and heart rate monitors and these measures were calibrated against the values. Written informed consent was obtained from the parents of each participant. The ethics committee of the National Institute (Tsukuba, Ibaraki, Japan) approved the experimental protocol for Environmental Studies.
Procedure
The experiment was conducted in a recreation room of the kindergarten from 2:00 to 6:00 p.m. Room temperature was set between 23 and 25 °C throughout the experiment to avoid the influence of cold temperature on the metabolism of the participants.Citation32,Citation33 All participants had fasted for at least 2 hr before the experiment to avoid an increase in metabolic rate.Citation33 At the start of the experiment, participants first lay on a floor mat without movement for at least 30 min to allow their metabolic rate to stabilize. After this equilibrating period, expired breath was collected for the resting state over 10 min. The participants then sequentially performed eight activities: (1) sitting in a chair, (2) standing, (3) playing with plastic bricks, (4) building with blocks, (5) walking, (6) climbing stairs (up and down), (7) tossing a ball, and (8) running. When the participants were sitting or standing, they were asked to keep calm and watch a cartoon video for 10 min. The children played for 15 min with the plastic bricks while sitting in a chair at a desk. When building with blocks, participants were allowed to move freely within a 1.9- by 2.4-m area of free space for 5 min; this activity included standing, squatting, walking, and lifting and carrying blocks. For climbing stairs, participants repeated climbing up and down four steps for 2 min. For ball tossing, a handball was thrown to the participant from a set distance every 7 sec for 3 min; participants were asked to catch the ball and throw it back. For walking, participants were instructed to walk continuously at their normal pace along a straight course of 10 m for 4 min. Running was performed for 3 min under the same conditions as walking, but participants were encouraged to maintain a speed of more than 100 m · min−1.Citation34 The average speed was 69 ± 6.4 m · min−1 for walking and 133 ± 17 m · min−1 for running. The activities were chosen because they are commonly performed by children and cover a wide range of activity levels on the basis of observations in the kindergarten and because they were thought not to interrupt the breath sampling described below.
The during the nine different activities was measured by the Douglas bag method. During each activity, participants wore facemasks to collect expired breath. Expired breath was collected through tubes (2.5-cm inner diameter × 80-cm length) into the 50-L Douglas bag near the end of each activity, after O2 consumption (
) had stabilized. Previous studies have reported that
and heart rate almost reach a plateau 1–2 min after the start of a 5-min endurance run in children 5 yr of age.Citation35,Citation36 The durations of the activities and sampling periods are summarized in .
Table 1. Time periods for performance of physical activity and sampling of exhaled breath
Instruments
Body acceleration was recorded throughout the experiment by using an Actical accelerometer (27 × 28 × 10 mm, 17 g, Mini-mitter, Inc.) and an ActivTracer accelerometer (type AC-210A, 48 × 67 × 16 mm, 57 g, GMS, Inc.). These two acceleration monitors were selected because of superior technical support and the following reasons. The Actical was selected because it was small, lightweight, and was considered to be suitable for invasive monitoring of activities of young children. The ActivTracer was selected because triaxial accelerometers have been reported to capture the principal acceleration component, which varies considerably with type of activity (e.g., between arm work and walking)Citation21,Citation22 and would thus more accurately measure the free-living activities of children than uniaxial accelerometers. This notion is supported by a previous report that the output from a triaxial accelerometer provides a stronger correlation with than that of a uniaxial accelerometer.Citation23
The Actical accelerometer has an omnidirectional sensor that is sensitive to movements in all directions and is most sensitive to vertical movement when mounted on the hip. The sensor detects movements in the 0.5- to 3.2-Hz frequency range and generates an analog voltage. The voltage is amplified, filtered, and then digitized by an analog-to-digital converter to create a digital value. The process is repeated 32 times per second (32 Hz), or every 31.25 msec, and the 32 digitized values in 1 sec are summed. The resulting 1-sec value is divided by 4 and then added to an accumulated activity value for the time intervals (epoch) specified by the user. The Actical accelerometer provides the measurements in terms of activity counts.
The ActivTracer accelerometer contains a triaxial accelerometer that detects movements in the anteroposterior (x-axis), mediolateral (y-axis), and vertical (z-axis) directions. The sensor detects the movements in each of the three directions every 40 msec with a band-pass filter of 0.3–100 Hz. The measurement range of the sensor is 0 to ±4 G for the vertical direction and 0 to ±2 G for the anteroposterior and mediolateral directions, and the resolution is 0.002 G. The output measure of the ActivTracer is the average of absolute values for acceleration (mG) in each axis direction and a synthesis of acceleration in three directions (xyz, vector synthetic) for the time interval defined by the user. Note that the outputs of the two accelerometers are not comparable because each provides acceleration measurement through a different data processing algorithm.
During the experiment, participants wore the two accelerometers on the hip by using a belt (). Heart rate was also monitored (ActiHR, Mini-mitter, Inc.) to compare the validity of the measurement for predicting with the accelerometer measurement. The sensor and lead of the heart rate monitor were attached to the participant's chest using disposable electrode pads in accordance with the manufacturer's instructions. Acceleration and heart rate were monitored every 15 sec.
Figure 1. Children wearing Actical and ActivTracer accelerometers on their hips with a belt. Heart rate was monitored at the same time using an ActiHR attached to the participant's chest. Dashed lines indicate the location of the sensor and lead of the heart rate monitor under the clothing.
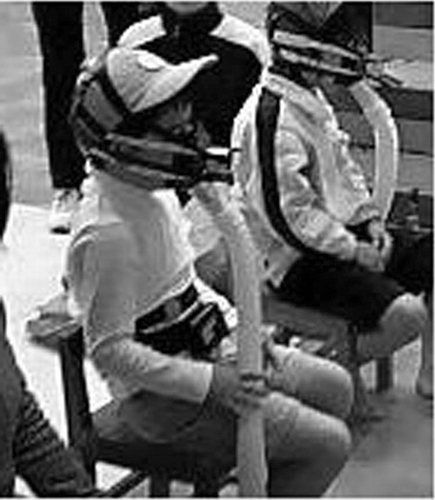
The volume of expired breath was measured with a dry gas flow meter (Shinagawa Company). To measure and carbon dioxide (CO2) production by participants, the O2 and CO2 concentrations in expired breath were analyzed by using a portable gas analyzer (AR-1, ARCO System, Inc.), which was calibrated and verified with outdoor air before each sample analysis.
Data Analysis
The volume of expired breath measured at ambient temperature and pressure and saturated with water vapor (ATPS condition) was converted to the volume at standard temperature and pressure under dry conditions (STPD condition). With the exception of the data for running, if the respiratory quotient (which is expressed as the ratio between CO2 production [] and
) was outside of the range 0.75–0.99, the V
E data were excluded from the analysis because the participant was in a state of hyperventilation or the exhaled breath had leaked from the facemask during breath sampling. Mean height, body weight, and
values were compared between boys and girls by using the Student's t test. For comparison of acceleration among multiple activities, one-way analysis of variance (ANOVA) was conducted, followed by the Scheffe test for the individual comparison if the result of ANOVA was significant.
Linear regression analysis was performed to develop equations to predict from the synthetic acceleration from the accelerometers and heart rates with all of the participants and activities combined. The regression equations were presented as follows:
Using the vertical and horizontal acceleration measured by the ActivTracer, linear discriminant analysis was performed to identify variables that strongly discriminate between the different types of activities. This analysis was used to dichotomize the type of activity into two groups, group 0 and group 1, and group was included in the analysis as a categorical dependent variable. The vertical activity counts (z) and the ratio between vertical and horizontal acceleration (z/[x 2 +y 2]0.5) were included as independent variables. The significance of the discriminant function was evaluated with Wilks′ λ. All statistical analyses were performed using SPSS software version 15 for Windows (SPSS, Inc.). Statistical significance was set at P <0.05.
The physical activity ratio (PAR), which is the energy cost of an activity per unit time, was calculated to classify V
E according to the intensity of physical activity on the basis of energy consumption. The PAR was calculated by dividing the EE (kcal · min−1) for a specific activity by the estimated basal metabolic rate (BMR).Citation38,Citation39 EE was calculated from the (L · min−1) and
(L · min−1) as described previously.Citation40 BMR was calculated by dividing the EE during the resting state by 1.1 on the assumption that the thermic effect of food is 10% of the BMR.Citation32 PAR was categorized as sedentary (PAR <1.5), light (1.5 ≤ PAR <3), moderate (3 ≤ PAR <6), and vigorous (6 ≤ PAR) according to values given in the literature for the energy cost of physical activities.Citation26 All data in the text, tables, and figures are presented as mean ± 1 SD.
RESULTS
The (STPD),
(STPD), accelerometer measurements, and heart rates of participants during the nine physical activities are summarized in and . The
ranged from 0.18 ± 0.02 L · kg−1 · min−1 when the participant was lying down to 1.07 ± 0.29 L · kg−1 · min−1 during running. There were no gender-associated differences (P > 0.05, t test) in the height or weight of participants or in
during the various activities.
Table 2. Summary of V
E and
at STPD and PAR during nine different activities
Table 3. Summary of accelerometer measurements and heart rate during nine different activities
Synthetic acceleration ranged from 1 ± 3 counts · sec−1 during sitting to 1960 ± 370 counts · sec−1 during running when measured with the Actical accelerometer and from 7.4 ± 5.2 during lying down to 1024 ± 126 mG · sec−1 during running with the ActivTracer accelerometer. The ActivTracer detected body acceleration in all participants during all nine activities. By comparison, the Actical detected body acceleration in only 78% of participants when lying down, 61% during sitting, 90% during standing, 75% while playing with plastic blocks, and in 100% of participants during all other activities. A significant difference was observed among the accelerations for resting, sitting, standing, and building with blocks when measured with the ActivTracer, whereas no significant difference was observed among the accelerations for these four activities when measured with Actical (ANOVA, P <0.05). Heart rates ranged from 98 ± 9 beats · min−1 when the participant was lying down to 177 ± 14 beats · min−1 during running.
Calibration
There was a strong correlation between the experimentally measured using the Douglas bag method and the synthetic acceleration measured by the Actical and ActivTracer; Pearson's correlation coefficients were r = 0.886 (n = 223, P <0.001) for the Actical and 0.913 (n = 222, P <0.001) for the ActivTracer. Heart rates showed a high linear correlation with the logarithmic
(r = 0.909, n = 223, P <0.001).
The mean percentage differences between experimentally measured and that estimated from synthetic acceleration and heart rates were calculated using linear regression models (). The synthetic acceleration overestimated
from lying down through playing with plastic bricks by 24–60% for the Actical and by 14–37% by the ActivTracer.
values for walking and running were overestimated by 11–20% for the Actical and by 11–24% for the ActivTracer. By comparison, the Actical underestimated the
values for building with blocks, ball tossing, and stair climbing by 19–23%, and the ActivTracer underestimated these values by 13–18%. When predicted from heart rates,
for lying down through to playing with plastic bricks was overestimated by 14–19%, whereas that for walking through to running was underestimated by 2–11%, with the exception of ball tossing, which was within an overestimation of 1%. The overall mean differences between the observed and predicted
were greater for the Actical (13% ± 33%) and ActivTracer (9% ± 24%) than for those calculated from heart rates (4% ± 26%).
Table 4. Difference between observed and estimated
from Actical and ActivTracer accelerometer measurements and heart rate using a single regression equation
Additional regression analysis showed that when building with blocks, ball tossing, and stair climbing were excluded, the correlation between and synthetic acceleration was improved, with r = 0.949 (n = 146, P <0.001, y = 0.0004x+ 0.22) for the Actical and r = 0.962 (n = 145, P <0.001, y = 0.00086x+ 0.20) for the ActivTracer. For the group of activities including building with blocks, ball tossing, and stair climbing, the correlation between V
E and synthetic acceleration was r = 0.781 (n = 77, P <0.001, y = 0.0004x+ 0.44) for the Actical and r = 0.761 (n = 77, P <0.001, y = 0.00094x+ 0.35) for the ActivTracer ().
Activity Type by Using Vertical and Horizontal Activity Counts
The linear discriminant analysis showed a significant difference between walking and the group of activities comprising building with blocks, stair climbing, and ball tossing (Wilks′ λ= 0.897, P <0.005). The discriminant function obtained was
The standardized canonical coefficient was greater for the ratio between vertical and horizontal acceleration than for vertical acceleration alone, indicating that the ratio was a more significant discriminator than the vertical acceleration. The sensitivity of discrimination was 75% for group 1 (walking) and 70% for group 0 (other activities). The correct classification rate was 75% for walking, as category 1, and was 60, 64, and 89% for the category 0 activities building with blocks, stair climbing, and ball tossing, respectively.
The for each activity was recalculated in two steps for data obtained with the ActivTracer. When the synthetic acceleration of a given activity was between 96 and 754 mG · sec−1 (the range of accelerometer measurements by the ActivTracer from building with blocks through to ball tossing), the activity was classified as walking or other activities using the previously described discriminant function. The
then was estimated from the synthetic acceleration measured by the ActivTracer using the regression equation developed for the group of activities to which the activity of interest was classified. As a result, the mean difference between observed and estimated
was+10% for lying down,+1% for sitting, −1% for standing, −6% for playing with plastic bricks and building with blocks,+16% for walking, −12% for stair climbing, −0.3% for ball tossing, and+1% for running (). The overall mean percentage difference was 0.2% ± 19% (−0.01 ± 0.12 L · kg−1 · min−1).
Figure 3. Percent difference between measured with a Douglas bag and that predicted from synthetic accelerometer measurements by the ActivTracer during nine different activities. Percent differences using the two regression equations were compared with those using the single regression equation. Activity: 1 = lying down, 2 = sitting, 3 = standing, 4 = playing with plastic bricks, 5 = building with blocks, 6 = walking, 7 = stair climbing, 8 = ball tossing, and 9 = running.
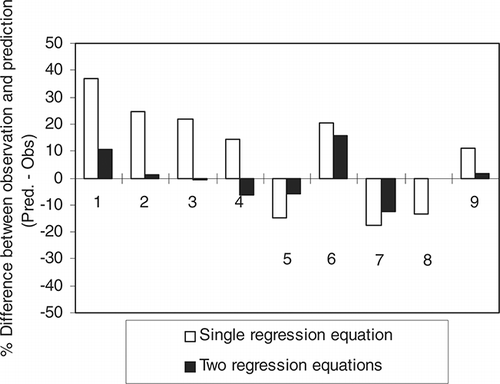
Classification of
The mean PAR was 1.16 ± 0.07 for sitting, 1.20 ± 0.11 for standing, 1.38 ± 0.20 for playing with plastic bricks, 2.79 ± 0.48 for walking, 3.00 ± 0.56 for building with blocks, 4.84 ± 0.68 for climbing stairs, 4.54 ± 0.91 for ball tossing, and 5.79 ± 1.05 for running. The PAR indicates a sedentary state for sitting, standing, and playing with plastic bricks; a light-to-moderate level of physical activity for building with blocks and walking; moderate physical activity for stair climbing and ball tossing; and moderate-to-vigorous physical activity for running. The thresholds corresponding to PAR values of 1.5, 3, and 6, which are the cutpoints between sedentary-to-light, light-to-moderate, and moderate-to-vigorous levels of physical activity intensity were 132, 478, and 1783 counts · sec−1 for the Actical measurements and 71, 412, and 1093 mG · sec−1 (category 1) and 31, 218, and 593 mG · sec−1 (category 0) for the ActivTracer measurements.
DISCUSSION
In the study presented here, mean values were obtained for Japanese preschool children 6 yr of age during the resting state and during sedentary through to vigorous physical activity. The weight and height of the participants in this study were similar to those reported for 6-yr-old children by the Ministry of Health, Labor, and Welfare, Japan.Citation41 The
values presented here for sitting and standing, which were equivalent to 5.00 ± 0.64 and 5.23 ± 0.67 L · min−1, respectively, at body temperature, ambient pressure, and saturated with water vapor (BTPS) were consistent with the
values of 4.98 ± 0.56 and 5.48 ± 0.44 L · min−1 (BTPS) in children 4–6 yr of age reported by Honda.Citation42
The accelerometer measurements were also validated for estimating the of young children during daily life. To the authors' knowledge, this study is the first to use accelerometer-based activity measurements to estimate
for different levels of activity. There was a strong positive linear correlation between synthetic acceleration from the accelerometers and
from the resting state through to vigorous physical activity, indicating that accelerometers can be used to estimate
.
The comparison between the Actical and ActivTracer accelerometers presented here revealed smaller percentage differences between observed and predicted values with the ActivTracer, especially from resting through to light levels of physical activity. This result suggests that the ActivTracer more accurately measures physical activity and hence than does the Actical. This difference is largely because of the differences in the ability of the Actical and ActivTracer to sense body acceleration. The ActivTracer detected body acceleration during resting and sedentary activities in all participants and revealed significant differences among the accelerations for resting, sitting, standing, and building with blocks. In contrast, the Actical failed to detect body movement in all participants during these activities and could not detect differences in acceleration among the activities. On the basis of the correlation coefficients for the single linear regression model, heart rate was a better predictor of
than the synthetic acceleration measurements from the accelerometers, but there was poor agreement between observed and predicted
from the resting state through to light levels of activity.
The primary limitation of accelerometers for estimating is that a single regression model does not accurately predict the
associated with activities during daily life. This study showed that the
for building with blocks, stair climbing, and ball tossing was underestimated by using the synthetic acceleration from the Actical and ActivTracer accelerometers. The experimentally observed
was significantly higher for these three activities than for walking, although activity counts for all of these activities varied similarly. This variability in the relationship between
and synthetic acceleration resulted in marked differences between observed data and estimated values derived using the single regression model. Similar results have been reported in studies validating the use of accelerometer-based activity monitors for determining EE.Citation43,Citation44 The underlying explanation is that static work or upper body activities such as lifting, carrying, and throwing objects results in increased EE or
, Citation45–49 and thus an increase in
, without a proportional increase in body movement. This discrepancy cannot be captured by synthetic acceleration alone.
It was shown that the type of activity can be discriminated by using three-dimensional motion data obtained from a triaxial accelerometer. Discriminant analysis revealed that the ratio between vertical and horizontal acceleration and vertical acceleration alone were reasonably effective in discriminating walking from playing with plastic bricks, climbing stairs, and ball tossing as a group but were less effective in discriminating between playing with plastic bricks and climbing stairs. The study presented here also demonstrated that prediction of can be improved through discrimination of the type of activity using the three-dimensional motion data and use of one of two regression models specific for the type of activity. Using this approach, the percentage difference between observed and predicted
was improved for each activity by an average of 14% and was even smaller than those predicted from heart rate ( and ).
This approach for improving the accuracy of estimating is essential for the more accurate assessment of the short-term or daily dose of air pollutants through inhalation, especially from the resting state through to light physical activity, because people tend to spend the greatest proportion of time at these levels of activity.Citation50,Citation51 For example, for lying down, the 37% overestimation in
resulting from the single regression equation with all activities combined is estimated to result in an overestimation of 8.6% in daily inhalation volume given the assumption that in 1 day children spend 10.2 hr in a resting state; 4 hr in a sedentary state; and 8.3, 1.3, and 1.2 hr in light, moderate, and vigorous levels of physical activity, respectively.Citation52 By comparison, the 10% overestimation in
for lying down calculated by the two regression equations is estimated to result in only a 2% overestimation in daily inhalation volume using the same assumptions.
Misclassification of the activity type by the discriminant function still leads to inaccurate estimations of for walking and climbing stairs. Using the assumption described above, a 16% error in the estimation for light-level activities (e.g., walking) is roughly equivalent to 7% of the total daily inhalation volume. However, an overestimation of
can be canceled out, at least in part, by underestimation of V
E for other light-to-moderate activities (e.g., building with blocks and stair climbing). Underestimation of
for climbing stairs will not cause considerable error because generally people spend only brief amounts of time in this type of activity during daily life.
The study presented here demonstrates that accelerometer measurements are a valid predictor of in children and overcome some of the limitations of traditional approaches. The traditional approach using self-report activity diaries has limited objectivity and accuracy in quantifying the intensity of physical activity of free-living people. For example, BenderCitation16 conducted a study of 65 children aged 10–16 yr together with their parents and reported a poor correlation between proxy records of children's physical activity by parents and the metabolic equivalent value on the basis of accelerometer measurements as an objective measure of physical activity (r ≤ 0.383). In another study, LichtmanCitation53 reported that the actual daily EE, measured by the DLW method, was overestimated by 50% in self-report activity diaries for adults. The inherent inability of self-reports to accurately quantify the intensity of physical activity could thus result in errors in estimating the daily inhalation rate. The validity of accelerometers in estimating
was estimated by comparing measurements from them with measurements from the Douglas bag method, and it was found that the correlation between accelerometer measurement and
is strong and that the error in estimating
using the regression equations derived from ActivTracer measurements is between −12% and+16% depending on the activity. Also, this difference was smaller than those estimated from the heart rate measurements.
Standards for categorizing have not been defined for children. Here,
was classified in relation to the level of physical activity for children in terms of the PAR. Differences in this classification structure between studies might lead to differences in the
corresponding to the activity level of interest and the estimates of the time spent in activities of different intensity categories. A previous studyCitation54 presented V
E corresponding to the level of physical activity intensity described in terms of metabolic equivalents (METs), which are expressed as a multiple of the resting metabolic rate for an activity. One MET is generally considered equivalent to the resting
of 3.5 mL · kg−1 · min−1 in adults. However, in children, the resting metabolic rate is known to exceed 3.5 mL · kg−1 · min−1.Citation26,Citation55 Puyau et al.Citation24 reported that use of the adult default value resulted in significant differences between the observed PAR and estimated MET and that this difference was greater in younger children and at higher levels of EE. Thus, use of the adult default value would inaccurately reflect the level of intensity of physical activities in young children.
One of the shortcomings of this study is the small number of subjects. Further studies with a larger study group would more accurately represent the population of 6-yr-old children in Japan. Repeated measurements of are also needed to obtain a more reliable assessment of
in young children. The conclusions presented here are based on nine different levels of physical activity in a laboratory setting and provide strong groundwork for further analysis and research. Additional studies covering a more comprehensive range of activities are needed to confirm the validity of this approach for prediction of
and to better understand the factors that contribute to variability in accelerometer measurements.
ACKNOWLEDGMENTS
The authors thank Seiko Yamaguchi for her technical advice on V E measurements and Naoko Yoshida, Mai Yamanishi, Makiko Yamamoto, Mamiko Iwasaki, and Satomi Hara for their assistance with the laboratory work. The authors thank all of the participants, their parents and teachers, and the administration of the kindergarten for their kind participation and support. The Ministry of Environment of Japan supported this study from 2005 through 2006. The contents of this article are solely the responsibility of the authors and do not necessarily represent official views of the ministry. The peer reviewer provided excellent comments that resulted in an improved paper.
REFERENCES
- Strategic Approach to International Chemical Management (SAICM), Report of the International Conference on Chemicals Management on the Works of Its First Session http://www.chem.unep.ch/ICCM/meeting_docs/iccm1_7/7%20Report%20E.pdf (http://www.chem.unep.ch/ICCM/meeting_docs/iccm1_7/7%20Report%20E.pdf) (Accessed: 19 April 2010 ).
- A Framework for Assessing Health of Environmental Exposures to Children; EPA/600/R-05/093F; U.S. Environmental Protection Agency; National Center for Environmental Assessment: Washington, DC, 2006 http://www.epa.gov/ncea (http://www.epa.gov/ncea) (Accessed: 19 April 2010 ).
- 2006 . “ Principle for Evaluating Health Risks in Children Associated with Exposure to Chemicals ” . In Environmental Health Criteria 237 , Geneva , , Switzerland : World Health Organization .
- 1997 . Report on Committee for Setting Goal for Reducing the Level of Dioxin in the Atmosphere , Tokyo , , Japan : Japan Environment Agency .
- Brochu , P. , Robitaille , D. , Francois , J. and Brodeur , J. 2007 . Physiological Daily Inhalation Rates for Free Living Individuals Aged 1 Month to 96 Years Using Data from Doubly Labeled Water Measurements: A Proposal for Air Quality Criteria, Standard Calculations and Health Risk Assessment . Hum. Ecol. Risk Assess. , 12 : 675 – 701 .
- Spiers , C.E. , Little , D.E. , Trim , S.C. , Johnson , T.R. , Linn , W.S. and Hackney , J.D. 1992 . Activity Patterns in Elementary and High School Students Exposed to Oxidant Pollution . J. Expo. Anal. Environ. Epidemiol. , 2 : 277 – 293 .
- Allan , M. and Richardson , G.M. 1998 . Probability Density Functions Describing 24-Hour Inhalation Rates for Use in Human Health Risk Assessment . Hum. Ecol. Risk Assess. , 4 : 379 – 408 .
- Layton , D.W. 1993 . Metabolically Consistent Breathing Rates for Use in Dose Assessment . Health Phys. , 64 : 23 – 36 .
- McCurdy , T. 2000 . Conceptual Basis for Multi-Route Intake Dose Modeling Using an Energy Expenditure Approach . J. Expo. Anal. Environ. Epidemiol. , 10 : 86 – 97 .
- 1997 . Exposure Factors Handbook , Washington , DC : U.S. Environmental Protection Agency; National Center for Environmental Assessment; Office of Research and Development .
- 2008 . Child-Specific Exposure Factors Handbook , Washington , DC : U.S. Environmental Protection Agency; National Center for Environmental Assessment; Office of Research and Development . EPA/600/R-06/096F
- Brochu , P. , Robitaille , D. , Francois , J. and Brodeur , J. 2006 . Physiological Daily. Inhalation Rates for Free Living Individuals Aged 2.6 Month to 96 Years Based on Doubly Labeled Water Measurements: Comparison with Time-Activity-Ventilation and Metabolic Energy Conversion Estimates . Hum. Ecol. Risk Assess. , 12 : 736 – 761 .
- Sallis , J.F. 1991 . Self Report Measures of Children's Physical Activity . J. Sch. Health. , 61 : 215 – 219 .
- Coleman , K.J. , Saelens , B.E. , Wiedrich-Smith , M.D. , Finn , J.D. and Epstein , L.H. 1997 . Relationships between TriTrac-R3D Vectors; Heart Rate, and Self Report in Obese Children . Med. Sci. Sports Exerc. , 29 : 1535 – 1542 .
- Terblanche , A.P.S. , Ozkaynak , H. , Spengler , J.D. and Butler , D.A. 1991 . Relationship between Self Reported Activity Levels and Actual Heart Rates in Teenagers . Journal of the Air & Waste Management Association , 41 : 942 – 946 .
- Bender , J.M. , Brownson , R.C. , Elliott , M.B. and Haire-Joshu , D.L. 2005 . Children's Physical Activity: Using Accelerometers to Validate a Parent Proxy Record . Med. Sci. Sports Exerc. , 37 : 1409 – 1413 .
- Bailey , R.C. , Olson , J. , Pepper , S.L. , Porszasz , J. , Barstow , T.J. and Cooper , D.M. 1995 . The Level and Tempo of Children's Physical Activities: An Observational Study . Med. Sci. Sports Exerc. , 27 : 1033 – 1041 .
- Adams , W.C. 1993 . Measurement of Breathing Rate and Volume in Routinely Performed Daily Activities; Contract No. A033-205 , Sacramento , CA : Final Report; California Environmental Protection Agency; Air Resources Board .
- Welk , G.J. 2000 . Measurement Issues in the Assessment of Physical Activity in Children . Res. Q. Exerc. Sport. , 71 : 59 – 73 .
- Meijer , G.A. , Westerterp , K.R. , Koper , H. and Hoor , F.T. 1989 . Assessment of Energy Expenditure by Recording Heart Rate and Body Acceleration . Med. Sci. Sports Exerc. , 21 : 343 – 347 .
- Bouten , C.V. , Westerterp , K.R. , Verduin , V. and Janssen , J.D. 1994 . Assessment of Energy Expenditure for Physical Activity Using a Triaxial Accelerometer . Med. Sci. Sports Exerc. , 26 : 1516 – 1523 .
- Eston , R.G. , Rowlands , A.V. and Ingledew , D.K. 1998 . Validity of Heart Rate; Pedometry and Accelerometry for Predicting Energy Cost of Children's Activities . J. Appl. Physiol. , 84 : 362 – 371 .
- Ott , A.E. , Pate , R.R. , Trost , S.G. , Ward , D.S. and Saunders , R. 2000 . The Use of Uniaxial and Triaxial Accelerometers to Measure Children's “Free Play” Activity . Pediatr. Exerc. Sci. , 12 : 360 – 370 .
- Puyau , M.R. , Adolph , A.L. , Vohra , F.A. and Butte , N.F. 2002 . Validation and Calibration of Physical Activity Monitors in Children . Obes. Res. , 10 : 150 – 157 .
- Rodriguez , G. , Beghin , L. , Michaud , L. , Moreno , L.A. , Turck , D. and Gottrand , F. 2002 . Comparison of the TriTrac-R3D Accelerometer and a Self-Report Activity Diary with Heart-Rate Monitoring for the Assessment of Energy Expenditure in Children . Br. J. Nutr. , 87 : 623 – 631 .
- Puyau , M.R. , Adolph , A.L. , Vohra , F.A. , Zakeri , I. and Butte , N.F. 2004 . Prediction of Activity Energy Expenditure Using Accelerometers in Children . Med. Sci. Sports Exerc. , 36 : 1625 – 1631 .
- Pfeiffer , K.A. , McIver , K.L. , Dowda , M. , Almeida , M.J. and Pate , R.R. 2006 . Validation and Calibration of the Actical Accelerometer in Preschool Children . Med. Sci. Sports Exerc. , 38 : 152 – 157 .
- Trost , G. , Ward , D.S. , Moorehead , S.M. , Watson , P.D. , Riner , W. and Bruke , J.R. 1998 . Validity of the Computer Science and Applications (CSA) Activity Monitor in Children . Med. Sci. Sports Exerc. , 30 : 629 – 633 .
- Plasqui , G. , Joosen , A.M. , Kester , A.D. and Westerterp , K.R. 2005 . Measuring Free Living Energy Expenditure and Physical Activity with Tri-Axial Accelerometry . Obes. Res. , 13 : 1363 – 1369 .
- Freedson , P.S. , Pober , D. and Janz , K.F. 2005 . Calibration of Accelerometer Output for Children . Med. Sci. Sports Exerc. , 37 : S523 – S530 .
- Freedson , P. and Miller , K. 2000 . Objective Monitoring of Physical Activity Using Motion Sensors and Heart Rate . Res. Q. Exerc. Sport. , 71 : 21 – 29 .
- Kobayashi , S. 1997 . Nutrition Standards and Recommendations: Recent Trends in RDA, RDI and Dietary Guidelines and Their Background , 86 – 96 . Tokyo , , Japan : Kenpakusya .
- Kashiwazaki , H. , Dejima , Y. and Suzuki , T. 1990 . Influence of Upper and Lower Thermoneutral Room Temperatures on Fasting and Post-Prandial Resting Metabolism under Different Outdoor Temperature . Eur. J. Clin. Nutr. , 44 : 405 – 413 .
- Yoshizawa , S. 2002 . Aerobic Capacity in Young Children , 61 – 71 . Tokyo , , Japan : Kyorin-Shoin .
- Yoshizawa , S. , Honda , H. , Urushihara , M. and Nakamura , N. 1981 . The Study on Aerobic Work Capacities of Preparatory School Children III . Jpn. J. Phys Fitness Med. , 30 : 73 – 85 .
- Hatano , Y. , Ono , M. and Miyazaki , Y. 1981 . Heart Rate Response and Work Intensity on Treadmill Running in Preschool Children . Rep. Res. Center Phys. Ed. , 9 : 127 – 136 .
- Bland , J.M. and Altman , D.G. 1986 . Statistical Methods for Assessing Agreement between Two Methods of Clinical Measurement . Lancet , 1 : 308 – 310 .
- 2004 . “ Human Energy Requirements. Report of a Joint FAO/WHO/UNU Expert Consultation ” . In Food and Nutrition Technical Report Series 1 , 35 – 52 . Geneva , , Switzerland : U.N. Food and Agriculture Organization .
- 2007 . “ Dietary Reference Values for Food Energy and Nutrients for the United Kingdom ” . In Report on Health and Social Subjects 41; 16th Impression , 15 – 38 . London , , United Kingdom : Department of Health; The Stationery Office .
- Weir , J.B. 1949 . New Methods for Calculating Metabolic Rate with Special Reference to Protein Metabolism . J. Physiol. , 109 : 1 – 9 .
- 2006 . The National Health and Nutrition Survey in Japan, 2003 , 318 – 319 . Tokyo , , Japan : Ministry of Health, Labor, and Welfare of Japan .
- Honda , H. , Watanabe , N. , Ito , K. , Nakamura , N. and Yoshizawa , S. 1995 . Changes in the Effects of 18 Month Endurance Run Training on Aerobic Work Capacity in Young Children . Jpn. J. Phys. Fitness Med. , 44 : 251 – 266 .
- Hendelman , D. , Miller , K. , Baggett , C. , Debold , E. and Freedson , P. 2000 . Validity of Accelerometry for the Assessment of Moderate Intensity Physical Activity in the Field . Med. Sci. Sports Exerc. , 32 : S442 – S449 .
- Tanaka , C. , Tanaka , S. , Kawahara , J. and Midorikawa , T. 2007 . Triaxial Accelerometry for Assessment of Physical Activity in Young Children . Obesity , 15 : 1233 – 1244 .
- Debusk , R.F. , Valdez , R. , Houston , N.R.N. and Haskell , W. 1978 . Cardiovascular Responses to Dynamic and Static Effort Soon after Myocardial Infarction . Circulation , 58 : 368 – 375 .
- Toner , M.M. , Sawka , M.N. , Levine , L. and Pandolf , K. 1983 . Cardiorespiratory Responses to Exercise Distributed between the Upper and Lower Body . J. Appl. Physiol. , 54 : 1403 – 1407 .
- Vander , L.B. , Franklin , B.A. , Wrisley , D. and Rubenfire , M. 1984 . Cardiorespira-tory Responses to Arm and Leg Ergometry in Woman . Phys. Sports Med. , 12 : 101 – 106 .
- Hangerman , F.C. , Lawrence , R.A. and Mansfield , M.C. 1988 . A Comparison of Energy Expenditure during Rowing and Cycling Ergometry . Med. Sci. Sports Exerc. , 20 : 479 – 488 .
- Pendergast , D.R. 1989 . Cardiovascular; Respiratory, and Metabolic Responses to Upper Body Exercise . Med. Sci. Sports Exerc. , 21 : S121 – S125 .
- 2005 NHK National Time Use Survey; NHK Broadcasting Culture Research Institute: Tokyo, Japan, 2006 http://www.nhk.or.jp/bunken/book/book_data/bookdata_06020701.html (http://www.nhk.or.jp/bunken/book/book_data/bookdata_06020701.html) (Accessed: 19 April 2010 ).
- Hubal , E.A.C. , Sheldon , E.A.C. , Burke , J.M. , McCurdy , T.R. , Barry , M.R. , Rigas , M.L. , Zartarian , V.G. and Freeman , N.C.G. 2000 . Children's Exposure Assessment: A Review of Factors Influencing Children's Exposure, and the Data Available to Characterize and Assess that Exposure . Environ. Health Perspect. , 108 : 475 – 486 .
- Kawahara , J. , Tanaka , C. , Tanaka , S. , Aoki , Y. and Yonemoto , J. 2008 . Estimation of Daily Inhalation Rate of Preschool Children by Using Tri-Axial Accelerometer . Epidemiology , 19 : S139
- Lichtman , S.W. , Pisarska , K. , Berman , E.R. , Pestone , M. , Dowling , H. , Weisel , H. , Heshka , S. , Matthews , D.E. and Heymsfield , S.B. 1992 . Discrepancy between Self-Reported and Actual Caloric Intake and Exercise in Obese Subjects . New Engl. J. Med. , 327 : 1893 – 1898 .
- 1994 . Methods for the Derivation of Inhalation Reference Concentrations and Application of Inhalation Dosimetry , Washington , DC : U.S. Environmental Protection Agency . EPA/600/8-90/066F
- 2005 . Dietary Reference Intakes for Energy, Carbohydrate, Fiber, Fat, Fatty Acids, Cholesterol, Protein, and Amino Acids , 880 – 935 . Washington , DC : National Academy .