ABSTRACT
In this study the performance of the American Meteorological Society and U.S. Environmental Protection Agency Regulatory Model (AERMOD), a Gaussian plume model, is compared in five test cases with the German Dispersion Model according to the Technical Instructions on Air Quality Control (Ausbreitungsmodell gemäβ der Technischen Anleitung zur Reinhaltung der Luft) (AUSTAL2000), a Lagrangian model. The test cases include different source types, rural and urban conditions, flat and complex terrain. The predicted concentrations are analyzed and compared with field data. For evaluation, quantile-quantile plots were used. Further, a performance measure is applied that refers to the upper end of concentration levels because this is the concentration range of utmost importance and interest for regulatory purposes. AERMOD generally predicted concentrations closer to the field observations. AERMOD and AUSTAL2000 performed considerably better when they included the emitting power plant building, indicating that the downwash effect near a source is an important factor. Although AERMOD handled mountainous terrain well, AUSTAL2000 significantly underestimated the concentrations under these conditions. In the urban test case AUSTAL2000 did not perform satisfactorily. This may be because AUSTAL2000, in contrast to AERMOD, does not use any algorithm for nightly turbulence as caused by the urban heat island effect. Both models performed acceptable for a nonbuoyant volume source. AUSTAL2000 had difficulties in stable conditions, resulting in severe underpredictions. This analysis indicates that AERMOD is the stronger model compared with AUSTAL2000 in cases with complex and urban terrain. The reasons for that seem to be AUSTAL2000's simplification of the meteorological input parameters and imprecision because of rounding errors.
This study contributes to the understanding of dispersion modeling and demonstrates the advantage of detailed meteorological data. It also helps air quality regulators and planners to identify the most appropriate model to use. It is indicated that AERMOD is more suitable for air quality planning and regulation, when all required meteorological information is available, because its predictions are mostly closer to field observations. Furthermore AUSTAL2000 predicted concentrations that showed a narrow range and triggered far less impacts from the source.
INTRODUCTION
Dispersion modeling is an important process tool in air quality regulation and planning throughout the world. Dispersion models simulate the dispersion of gases and particles and help to predict the concentrations of pollutants in the atmosphere. They are a cost- and time-effective alternative to field measurements.
Dispersion models have to be reliable. Inaccurate predictions can lead to faulty decisions. An underestimation of pollutant concentrations can result in wrong decisions and can thus yield conditions that are deemed unhealthy for the population. On the other hand, an overestimation can result in excessive restrictions and high costs for a facility.
Two models are compared in this study. The American Meteorological Society and U.S. Environmental Protection Agency Regulatory Model (AERMOD) and the Dispersion Model according to the Technical Instructions on Air Quality Control (Ausbreitungsmodell gemäβ der Technischen Anleitung zur Reinhaltung der Luft) (AUSTAL2000) are short-range dispersion models for travel distances up to 50 km within the atmospheric boundary layer. They are open source and freely available. AERMOD was developed by the American Meteorological Society (AMS) and the U.S. Environmental Protection Agency (EPA), who formed together the AMS and EPA Regulatory Model Improvement Committee (AERMIC). Since 2005, AERMOD is the preferred regulatory model of the EPA. AUSTAL2000 was developed for the German Federal Environmental Agency (Umweltbundesamt). It is the reference dispersion model for short-range transport in Germany and is compliant with Annex 3 of the Technical Instructions on Air Quality Control (TA Luft)Citation1 and guideline 3945, part 3 of the Association of German Engineers.Citation2
This paper gives on overview of the performance comparison of AERMOD and AUSTAL2000 in five test cases. These cases include different source types, rural and urban conditions, flat and complex terrain. For this study the predicted concentrations of each model are compared with field data using quantile-quantile (Q-Q) plots. A performance measure is used that uses the important upper end of the concentration ranges.
THE MODELS
AERMOD
AERMOD is a steady-state dispersion model because the meteorological conditions are assumed to be consistent during the modeling period of 1 hr and horizontally homogenous. However, it accounts for vertical variations of meteorological parameters in the planetary boundary layer. This model is designed for stationary sources and emissions are assumed to be continuous and neutral or positively buoyant. AERMOD can handle multiple point, area and volume sources. It does not differentiate between different pollution types, but algorithms for dry and wet deposition are incorporated.Citation3
Under stable and neutral stratification with a Monin–Obukhov length (L) greater than 0, AERMOD assumes the concentration distribution to be horizontally and vertically of Gaussian type. During convective and neutral conditions with L < 0, AERMOD presumes Gaussian distribution for the horizontal but bi-Gaussian distribution for the vertical dimension. It also incorporates the three-plume concept for calculations in the convective boundary layer, which treats the emitted plume as if it came from three different sources: a direct source that accounts for the part of the emissions that is carried directly to the ground, an indirect source that accounts for the initial reflection at the mixing height and all subsequent reflections, and a penetrated source that refers to the part that penetrates the elevated inversion and reenters it.Citation4
In mountainous terrain, AERMOD simulates a plume according to the concepts of the critical dividing streamline that defines which plumes flow over the hill and which flow around it.Citation5,Citation6 Downwash effects are calculated with the Plume Rise Model Enhancement (PRIME) algorithm. The estimation of the plume rise incorporates the effects of velocity deficit, enhanced dilution from the turbulent wake, radiative heat loss, streamline deflection, and vertical wind shear. Schulman et al.Citation7 deliver a detailed description of the PRIME algorithm.
With its urban mode, AERMOD accounts for the dispersive nature of the urban thermal effects that form during nighttime in the boundary layer when stable rural air flows over a warmer urban surface. AERMOD scales this effect by population size.
AERMOD uses a large amount of meteorological information, including the surface friction velocity, Dear-dorff convective velocity, vertical potential temperature gradient, height of the convectively generated boundary layer, height of the mechanically generated boundary layer, L, surface roughness length (z 0), wind speed, wind direction, temperature, and the measurement heights of wind and temperature. Further, it uses the data of radio soundings for each recorded height of measurement, including wind direction, wind speed, temperature, standard deviation of the wind direction, and the standard deviation of the wind speed.Citation3,Citation8 Foken explains the meteorological parametersCitation9 and Cimorelli et al.Citation4 give a description of AERMOD's model formulation.
Two input data processors are regulatory components of the integrated AERMOD modeling system: the meteorological data preprocessor AERMETCitation8 and the terrain preprocessor AERMAP.Citation10 Of the nonregulatory components of this modeling system, only the Building Profile Input Program for PRIME (BPIPPRIME)Citation7 was used. The version of AERMOD used in this study is 09292 from October 2009.
AUSTAL2000
Like AERMOD, AUSTAL2000 is a steady-state dispersion model that is designed for long-term sources and continuous buoyant plumes. AUSTAL2000 is also capable of using multiple point, area, volume, and line sources. This model includes dry deposition algorithms and considers the conversion of nitric oxide (NO) to nitrogen dioxide (NO2). It is also able to make predictions about the frequency of odor nuisance.Citation11 However, only data of sulfur dioxide (SO2) and sulfur hexafluoride (SF6) measurements were used in the test cases of this study.
Unlike the Gaussian dispersion model AERMOD, AUSTAL2000 is a Lagrangian dispersion model that simulates the dispersion of air pollutants by utilizing a random walk process. According to Sawford,Citation12 a Lagrangian simulation “has greater potential for application” as it mimics the behavior of particles. The direction and velocity of dispersion are estimated by wind field vectors. Additionally, the vector of the turbulent velocity is randomly varied for every particle by using a Markov process. The random element varies with the intensity of turbulence. The concentration is determined by counting the particles in a given volume.Citation2
Like AERMOD, AUSTAL2000 is capable of calculating terrain and contains its own algorithm to assess the effects of building downwash.Citation11 AUSTAL2000 does not differentiate between rural or urban areas.
AUSTAL2000 requires less meteorological information than AERMOD: z 0, wind measurement height, wind direction, wind speed, and the stability classes according to Klug–Manier. The Klug–Manier classesCitation11 represent the German standard stability classification for the atmosphere, similar to the Pasquill stability classesCitation13 in the
United States. All of these meteorological data come from ground-based measurements and no information from upper air soundings is utilized.
The wind measurement height and z 0 are provided in the input file. If z 0 is not provided by the user, AUSTAL2000 will calculate it using an internal database of roughness lengths and the coordinates of the area.Citation11
AUSTAL2000 uses the register of roughness lengths and the integrated wind field component TALdia, which creates wind field libraries for complex terrain and for cases with buildings. Detailed information about the model is to be found in the program documentation by Janicke.Citation11 The version of AUSTAL2000 used in this study is 2.4 from January 2009.
TEST CASES
Five test cases based on U.S. field studies were used for this paper, each one with different features. gives a short summary.
Table 1. Summary of the test cases used in this study
Two test cases were constructed from the Bowline field study.Citation14 One case was without any buildings in the modeling domain, the other included a power plant building to assess the effects of building downwash. The Bowline power plant is located on the western shore of the Hudson River, near Haverstraw, Rockland County, NY. Four receptors were installed in the vicinity of the power plant to measure the actual SO2 concentration. Data were collected for the entire year of 1981, resulting in 8760 data points of hourly averages. The wind was predominantly from the northwest, with wind speeds between 5.7 and 8.8 m/sec the most common. The background concentration was also measured and deducted. This was also done in all of the other SO2 field studies.
A third test case for the two models was created from the observations of the former Westvaco paper plant.Citation15 It includes complex terrain. The paper plant is in the small town of Luke in hilly Allegany County, MD. Eleven SO2 samplers were placed in the surrounding area at a distance between 800 and 1500 m and data were collected from December 1980 to November 1981, resulting in 8760 hr of data. Westerly winds prevailed, with wind speeds generally from 3.6 to 5.7 m/sec.
Because AERMOD has an urban mode to account for the urban thermal effects during nighttime, the Indianapolis test case (no. 4) was created from a field study done by Murray and BowneCitation16 to test the model performance in an urban environment. Indianapolis is the capital of the state of Indiana. AERMOD needs information about the population size for its urban mode. A population of 700,000 was used, which is an approximation of the population size in 1988. The tracer SF6 was released into the stack of the Perry-K generating station. The SF6 concentration in the lower atmosphere is negligible, making it a direct source and a good tracer. In September and October 1985 a total of 170 hr of data were collected. During the measurements southerly winds with speeds from 5.7 to 8.8 m/sec were the most frequent.
In the Duke Forest field study, a volume source was simulated by a nonbuoyant release of SF6 from an array of point sources (no. 5). The study was conducted on an open field site at Duke Forest, NC, over 11 days in January 1995.Citation17 In the 38 hr of data, the wind most frequently came from the southeast and northwest with wind speeds commonly in the range of 5.7–8.8 m/sec.
PERFORMANCE MEASURES
There are several ways to evaluate the performance of models. Because the high end of ground-level concentrations is typically of interest in air quality regulation and planning,Citation18 this study focuses on plots and a performance measure that refers to peak concentrations of air pollutants.
The predicted concentrations of both models are compared with observation data. Generally care needs to be taken when predicted concentrations are compared with measured ones. The former reflect the increase of concentration in response to the source emissions, whereas the latter also include the background concentrations. However, in the SO2 field databases used in this study, the background concentration was deducted. The atmospheric concentration of the tracer SF6 is insignificant.
Q-Q plots rank the predicted and observed concentrations from maximum to minimum and then pair them by their rank.Citation19 Perfect rank correlation will be expressed by data points along the 1:1 line. A good model would yield data points close to this line. A Q-Q plot provides the opportunity to assess, over the full range of concentrations, how close the model's predictions are to the observed data.
The robust highest concentration (RHC) was calculated and used as an element of another indicator. The RHC is a smoothed estimation of the peak concentrations. It is preferred over the actual maximum values because these are highly variable and could be caused by unusual events.Citation18
The formula given by Perry et al.Citation20 is
where x{n} is the nth largest value, n is the number of values to characterize the upper end of the concentration values, and x is the average of the n − 1 largest values. Cox and TikvartCitation18 suggested selecting n = 26, which is used for most cases in this study.
The fractional bias (FB) is also used to evaluate the model performance. According to Cox and Tikvart,Citation18 the FB is symmetrical and bounded. Further, it “is a dimensionless number which makes it convenient for combining the results from data categories having significantly different concentration levels.” It is given by
where OB is the RHC of the observed field data and PR is the RHC of the predicted concentrations. The FB ranges from −2 (for extreme overprediction) to +2 (for extreme underprediction). Values around zero represent predictions that are close to field measurements.
RESULTS AND DISCUSSION
The first of the two test cases with the Bowline power plant represents the situation without buildings. shows the annual average of the SO2 concentration predictions of AERMOD and AUSTAL2000. The overall direction of the concentration distribution shows similarities in both models. Wind data are the key meteorological parameter for these models and lead to higher SO2 concentrations in the southeast and, to a lesser degree, in the northwest of the plant. Compared with AUSTAL2000, the highest concentrations of AERMOD are much closer to the source. AERMOD predicts higher concentrations with the highest value of 7.4 μg/m3; AUSTAL2000's maximum is less than half as high with 3.3 μg/m3. It should be mentioned that both models predicted the ground-level concentration maximum in a distance of more than 1 km from the source, but all four samplers were closer than 1 km. The predicted concentrations of the Gaussian AERMOD are smoothly dispersed. However, the concentrations of AUSTAL2000 are spread in patches because of the Lagrangian approach and the use of its default quality level.
Figure 1. Predicted SO2 concentration distribution (annual average) of (a) AERMOD and (b) AUSTAL2000 for the Bowline test case without buildings. × = the source.
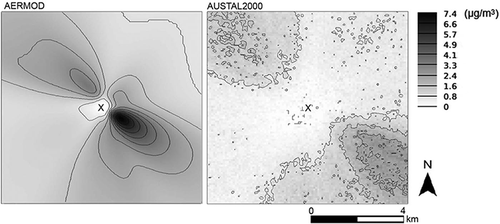
The distribution significantly changed when both models took the power plant building into account. They predicted higher levels of SO2 downwind. AERMOD predicted a peak concentration nearly 3 times as high with 21.6 μg/m3 and AUSTAL2000 more than doubled it with 7.5 μg/m3. For both models the concentration maximum was closer to the source because of the building downwash effects. Once again, in this case AERMOD predicted higher concentrations for the annual average than AUSTAL2000 ().
Figure 2. Predicted SO2 concentration distribution (annual average) of (a) AERMOD and (b) AUSTAL2000 for the Bowline test case with the power plant building. × = the source.
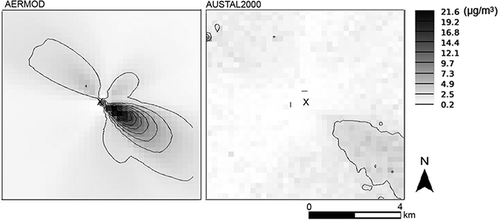
The Q-Q plot for the test case without buildings using the data of all samplers is shown in . AUSTAL2000 constantly underpredicts the SO2 concentrations and exhibits only a limited range of values. AERMOD also underpredicts the concentration; however, it has a broader range for SO2 and more hours with impacts from the source. Although the peak concentrations of both models are acceptable, their predictions for lower concentrations differ strongly from the observation data. This is especially true for AUSTAL2000. Nevertheless, the upper end of the predicted values is most important for regulatory purposes.
Figure 3. Q-Q plot for all of the receptor points of the Bowline test case with simple terrain (1-hr average). ▪ = AERMOD, ▽ = AUSTAL2000. The solid line indicates the 1:1 rank correlation of the distributions. The dotted lines illustrate the factor of 2 over-and underestimates.
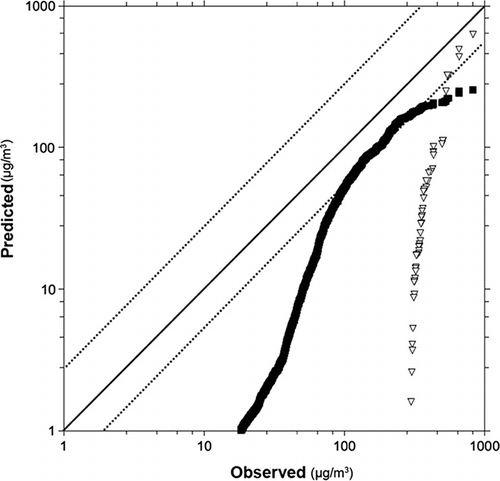
Looking at the FB (), AERMOD, over the entire year, moderately underpredicted the SO2 pollution with a FB of 1.01 for the test case without building downwash. AUSTAL2000's underprediction was less strong with a FB of 0.44. Both models performed rather poorly under very stable and stable conditions, exhibiting extreme under-prediction. When neutral conditions prevailed, AERMOD had a FB of 0.89 and AUSTAL2000 a FB of 1.23. In convective conditions, the two models produced contrasting results. AERMOD modestly overpredicted with a FB of −0.63 and AUSTAL2000 underpredicted with a FB of 1.06. However, AUSTAL2000 overestimated the concentration in very unstable conditions with a FB of −1.33 and AERMOD overestimated with a FB of −1.05.
Table 2. FB for each test case and atmospheric stability
Huber and SnyderCitation21 observed in a wind tunnel study that downwind near an elevated source such as a smoke stack the main influence on the plume is the enhancement of dispersion in the vertical dimension. They also noted that in very low winds and strong atmospheric stability, the large horizontal wind meander has a far greater influence than the building. Both models considered this and performed better when they included the power plant building in their calculations. AERMOD slightly overestimated the high-end concentrations whereas the underprediction of AUSTAL2000 improved slightly, as portrayed in . The slopes and ranges for both models are quite similar to the test case without buildings.
Figure 4. Q-Q plot for all of the receptor points of the Bowline test case with the power plant building (1-hr average). ▪ = AERMOD, ▽ = AUSTAL2000. Solid and dotted lines symbolize the same as in .
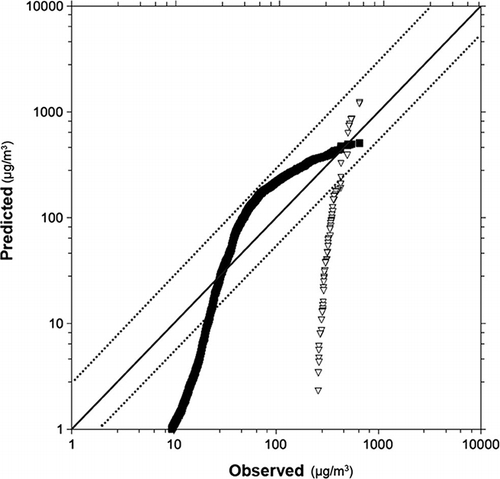
The comparison of the FB of both Bowline test cases emphasizes that the two models gained precision by including the power plant building. AERMOD underestimated the concentrations with a FB of 0.28 and AUSTAL2000 overestimated them with a FB of −0.74 over the whole year. For the test case that includes the power plant building. AERMOD performed considerably better in very stable conditions with a FB of 0.25 and a FB of only 0.03 for stable conditions. Nevertheless, AUSTAL2000 continued to seriously underpredict the SO2 concentration. Both models showed improved predictions for neutral stratifications with AERMOD having a FB of 0.19 and AUSTAL2000 having a FB of −0.77. AUSTAL2000's results were better than AERMOD's in unstable conditions because its FB was reduced from 1.06 to 0.12. The same can be stated for very unstable conditions because AUSTAL2000's FB improved from −1.33 to −0.37 whereas the quality of AERMOD's FB remained about the same.
In the Westvaco test case, in which elevated terrain was integrated, AERMOD did particularly well whereas AUSTAL2000 fell short. The Q-Q plot in displays, for all of the samplers, that AERMOD only slightly differs from the observed concentrations in the upper end. Its slope is almost similar to the 1:1 line for values higher than 100 μg/m3, proving that AERMOD is clearly able to handle complex terrain. However, AUSTAL2000 shows a strong underestimation, a short range of concentrations, and a steep slope as in the Bowline cases.
Figure 5. Q-Q plot for all of the receptor points of the Westvaco test case (1-hr average). ▪ = AERMOD, ▽ = AUSTAL2000. Solid and dotted lines symbolize the same as in .
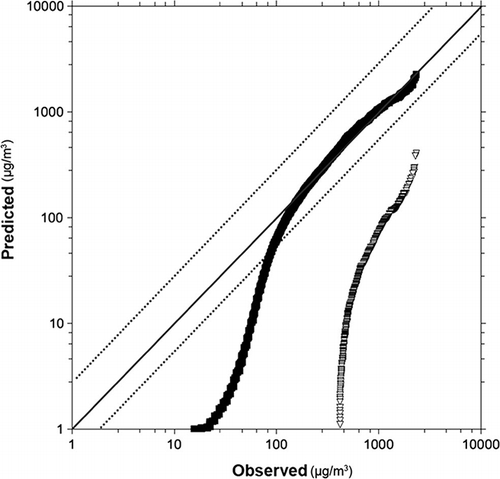
AERMOD performed very well with a FB of 0.15 for the whole year. Contrastingly, AUSTAL 2000 strongly underpredicted the SO2 pollution with a FB of 1.49. In stable stratifications, AERMOD was reliable with a FB of only 0.12 for very stable conditions and 0.59 for stable conditions. AUSTAL2000 had difficulties calculating concentrations in stable conditions, leading to strong underpre-diction. AERMOD modestly underestimated in neutral stratifications with a FB of 0.74, whereas AUSTAL2000 had a FB of 1.61. About the same can be stated for unstable conditions. In very unstable conditions AERMOD was very accurate with a FB of 0.07 whereas that of AUSTAL2000 was 1.21.
The strongest performance difference between the models was observed for the Indianapolis test case. AUSTAL2000 strongly underestimated the concentrations. This is especially the case for nighttime hours with stable stratification, when it did not predict any hours with impacts from the source. During these nightly conditions weak urban thermal effects are formed that are caused by the heat island effect, which AERMOD takes into account by using its urban mode. The fact that AUSTAL2000 fails to consider this effect might be one of the reasons for its rather poor performance. As Paine et al.Citation22 already observed for the Indianapolis database, AERMOD performed well for convective conditions at all downwind samples. During stable conditions it underestimated the tracer, especially the very low concentrations within 1 km of the stack.
Although AERMOD underpredicted with a FB of 0.43 in the Duke Forest test case, AUSTAL2000 predicted the tracer concentrations very well with a FB of 0.01. The reason for AUSTAL2000's very good predictions might be that there is no plume rise, because the tracer was emitted by a nonbuoyant volume source, and the receptors are placed very close to the source with a maximum distance of approximately 200 m.
CONCLUSIONS
In most of the test cases, AERMOD's predictions are closer to the field observations than those of AUSTAL2000, although the former does not mimic the random walk of particles and uses the “less natural” Gaussian approach. Both models underpredicted the concentrations for the Bowline test case with simple terrain. Their performance considerably improved with the inclusion of the power plant building, indicating that the downwash effect near a source is an important factor. Whereas AERMOD handled complex topography well, AUSTAL2000 significantly underestimated the concentrations. AERMOD's good results in mountainous terrain are according to Perry et al.Citation20 because of its definition and incorporation of the vertical structure of the boundary layer and the use of the dividing streamline concept. In the only urban test case of this study, AUSTAL2000 did not perform well. The reason may be the lack of consideration of nightly turbulences caused by the heat island effect in the city. Both models worked acceptably for a nonbuoyant volume source, with AUSTAL2000 predicting the tracer concentrations well and AERMOD moderately underpredicting the tracer in the Duke Forest study. It should be mentioned that Fernando et al.Citation23 noticed that for bigger scale volume and area sources such as agricultural burnings, buoyancy effects play a dominant role and the current buoyancy algorithm in AERMOD only works for point sources. Because it can be difficult to obtain the emission rates of an area source, Faulkner et al.Citation24 analyzed the influence of changes in the emission factor in software containing the algorithm of AERMOD and AUSTAL2000 among others. Faulkner et al.Citation24 used ammonia measurements of a 24-hr period as a database. They observed that AUSTAL2000 had a tendency to predict lower concentrations than AERMOD.
Looking at the FB overall, AERMOD tends toward a slight underestimation. AERMOD showed no clear tendencies toward overestimation or underestimation regarding its performance in different atmospheric stratifications. However, Perry et al.Citation20 reported that AERMOD “is still somewhat challenged” in predicting lower values during stable conditions. In this study, AUSTAL2000 commonly had difficulties in stable conditions, resulting in strong underpredictions.
The reasons for AUSTAL2000's lower performance in complex terrain seem to be its simplification of the meteorological parameters and imprecision because of rounding errors. AERMOD uses a vast amount of meteorological information, whereas AUSTAL2000 only uses the z 0, wind measurement height, wind direction, wind speed, and the stability classes according to Klug–Manier. The German model also assumes the z 0 is constant over the whole measuring period, whereas AERMOD accepts hourly values for z 0. Detailed information about z 0 seems to be important because Faulkner et al.Citation25 proved that AERMOD is very sensitive to changes in z0. To assess atmospheric stability, AERMOD directly uses L. AUSTAL2000 only uses L indirectly because it follows the instructions from the TA Luft and calculates them from Klug–Manier classes and z 0.Citation1 The use of Klug–Manier classes is convenient for the user because L can be difficult to obtain. Nevertheless, this procedure is prone to large rounding errors and the direct use of such data leads to fewer uncertainties.
Generally speaking, this analysis indicates that AERMOD is the stronger model compared with AUSTAL2000 in complex and urban terrain. In cases with simple terrain, both models lead to acceptable results. Given the specific conditions and scope of the investigation, a model user has to evaluate whether he/she can get the meteorological data required to operate AERMOD. For cases of poor meteorological data coverage, AUSTAL2000 could be an alternative. There is also the option to use a prognostic model such as the Fifth-Generation National Center for Atmospheric Research/Pennsylvania State Mesoscale Model to generate meteorological data for AERMOD. This procedure has shown to yield higher predicted concentrations.Citation26
Another aspect worth mentioning is the duration of a calculation (CPU time). The complex algorithm of AUSTAL2000 makes a run, especially for complex terrain, very time-consuming, which is another relative advantage of AERMOD.
Dispersion modeling is a valuable tool for air quality regulators and planners and delivers predictions of environmental impacts in the atmosphere. AERMOD performed this task rather well, although the output data must, as in any other case of predictive models, be interpreted with great care.
ACKNOWLEDGMENTS
The authors thank Bruce A. Egan of Egan Environmental, Steve R. Hanna of Hanna Consultants, and the EPA for providing us with the field databases. This study would not have been possible without the data. The authors also thank the air quality experts of the South Coast Air Quality Management District for their friendly support, Hayley Chamberlain and Lillian Harris for language editing of the manuscript, and the reviewers for their careful work.
REFERENCES
- German Federal Ministry for Environment, Nature Conservation and Nuclear Safety . 2002 . First General Administrative Regulation for the Federal Emission Control Law/Instructions for Pollution Control—TA Luft (in German) . Gemeinsames Ministerialblatt. , 24 : 511 – 605 .
- 2000 . Guideline 3945, Part 1. Environmental Meteorology—Atmospheric Dispersion Models—Particle Model , Düsseldorf , , Germany : Verein Deutscher Ingenieure .
- 2004 . User's Guide for the AMS/EPA Regulatory Model—AERMOD , Research Triangle Park , NC : U.S. Environmental Protection Agency . EPA-454/B-03-001
- Cimorelli , A.J. , Perry , S.G. , Venkatram , A. , Weil , J.C. , Paine , R.J. , Wilson , R.B. , Lee , R.F. , Peters , W.D. and Brode , R.W. 2005 . AERMOD: a Dispersion Model for Industrial Source Applications. Part I: General Model Formulation and Boundary Layer Characterization . J. Appl. Meteorol. , 44 : 682 – 693 .
- Shepphard , P.A. 1956 . Airflow over Mountains . Quart. J. Roy. Meteorol. Soc. , 82 : 528 – 529 .
- Snyder , W.H. , Thompson , R.S. , Eskridge , R.E. , Lawson , R.E. , Castro , I.P. , Lee , J.T. , Hunt , C.R. and Ogawa , Y. 1985 . The Structure of the Strongly Stratified Flow over Hills: Dividing Streamline Concept . J. Fluid Mech. , 152 : 249 – 288 .
- Schulman , L.K. , Strimaitis , D.G. and Scire , J.S. 1997 . Addendum to ISC3 User's Guide—the Prime Plume Rise and Building Downwash Model , Palo Alto , CA : Electric Power Research Institute .
- 2004 . User's Guide for the AERMOD Meteorological Preprocessor (AERMET) , Research Triangle Park , NC : U.S. Environmental Protection Agency . EPA-454/B-03-002
- Foken , T. 2006 . Micrometeorology , Berlin , Heidelberg : Springer Verlag .
- 2004 . User's Guide for the AERMOD Terrain Preprocessor (AERMAP) , Research Triangle Park , NC : U.S. Environmental Protection Agency . EPA-454/B-03-003
- 2009 . AUSTAL2000—Program Documentation of Version 2.4 , Dunum , Germany : Janicke Consulting .
- Sawford , B.L. 1985 . Lagrangian Statistical Simulation of Concentration Mean and Fluctuation Fields . J. Climate Appl. Meteorol. , 24 : 1152 – 1166 .
- Pasquill , F. 1961 . The Estimation of the Dispersion of Windborne Material . Meteor. Mag. , 90 : 33 – 49 .
- Schulman , L.L. and Hanna , S.R. 1986 . Evaluation of Downwash Modifications to the Industrial Source Complex Model . J. Air Pollut. Control Assoc. , 36 : 258 – 264 .
- Strimaitis , D.G. , Paine , R.J. , Egan , B.A. and Yamartino , R J. 1987 . EPA Complex Terrain Model Development: Final Report , Research Triangle Park , NC : U.S. Environmental Protection Agency; Office of Air Quality Planning and Standards . EPA/600/3-88/006
- Murray , D.R. and Bowne , N.E. 1988 . Urban Power Plant Plume Studies , Palo Alto , CA : Electric Power Research Institute . EA-5468
- Hanna , S.R. , Egan , B.A. , Purdum , J. and Wagler , J. 1999 . Evaluation of ISC3, AERMOD, and ADMS Dispersion Models with Observations from Five Field Sites , San Antonio , TX : Hanna Consultants .
- Cox , W. and Tikvart , J. 1990 . A Statistical Procedure for Determining the Best Performing Air Quality Simulation Model . Atmos. Environ. , 24 : 2387 – 2395 .
- Chambers , J.M. , Cleveland , W.S. , Kleiner , B. and Tukey , J.A. 1983 . Graphical Methods for Data Analysis (Bell Laboratories) , 47 – 73 . Belmont , CA : Wadsworth International Group and Duxbury Press .
- Perry , S.G. , Cimorelli , A.J. , Paine , R.J. , Brode , R.W. , Weil , J.C. , Venkatram , A. , Wilson , R.B. , Lee , R.F. and Peters , W.D. 2005 . AERMOD: a Dispersion Model for Industrial Source Applications. Part II: Model Performance against 17 Field Study Databases . J. Appl. Meteorol. , 44 : 694 – 708 .
- Huber , A.H. and Snyder , W.H. 1982 . Wind Tunnel Investigation of the Effects of a Rectangular-Shaped Building on Dispersion of Effluents from Short Adjacent Stacks . Atmos. Environ. , 12 : 2837 – 2848 .
- Paine , R.J. , Lee , R.F. , Brode , R. , Wilson , R.B. , Cimorelli , A.J. , Perry , S.G. , Weil , J.C. , Venkatram , A. and Peters , W.D. 1988 . Model Evaluation Results for AERMOD , Research Triangle Park , NC : U.S. Environmental Protection Agency .
- Fernando , H.J.S. , Lee , S.-M. and Dumitrescu , C. 2008 . Effects of Prescribed Fires on Air Quality in the Yuma-San Louis Area , Tempe , AZ : Department of Mechanical and Aerospace Engineering; Arizona State University .
- Faulkner , W.B. , Powell , J.J. , Lange , J.M. , Shaw , B.W. , Lacey , R.E. and Parnell , C.B. 2007 . Comparison of Dispersion Models for Ammonia Emissions from a Ground-Level Area Source . Trans. ASABE , 50 : 2189 – 2197 .
- Faulkner , W.B. , Shaw , B.W. and Grosch , T. 2008 . Sensitivity of Two Dispersion Models (AERMOD and ISCST3) to Input Parameters for a Rural Ground-Level Area Source . Journal of the Air & Waste Management Association , 58 : 1288 – 1296 . doi: 10.3155/1047-3289.58.10.1288
- Touma , J.S. , Isakov , V. , Cimorelli , A.J. , Brode , R.W. and Anderson , B.J. 2007 . Using Prognostic Model-Generated Meteorological Output in the AERMOD Dispersion Model: an Illustrative Application in Philadelphia, PA . Journal of the Air & Waste Management Association , 57 : 586 – 595 . doi: 10.3155/1047-3289.57.5.586