ABSTRACT
This paper describes a near-field validation study involving the steady-state, U.S. Environmental Protection Agency (EPA) guideline model AERMOD and the nonsteady-state puff model CALPUFF. Relative model performance is compared with field measurements collected near Martins Creek, PA—a rural, hilly area along the Pennsylvania-New Jersey border. The principal emission sources in the study were two coal-fired power plants with tall stacks and buoyant plumes. Over 1 yr of sulfur dioxide measurements were collected at eight monitors located at or above the two power plants' stack tops. Concurrent meteorological data were available at two sites. Both sites collected data 10 m above the ground. One of the sites also collected sonic detection and ranging measurements up to 420 m above ground. The ability of the two models to predict monitored sulfur dioxide concentrations was assessed in a four-part model validation. Each part of the validation applied different criteria and statistics to provide a comprehensive evaluation of model performance. Because of their importance in regulatory applications, an emphasis was placed on statistics that demonstrate the model's ability to reproduce the upper end of the concentration distribution. On the basis of the combined results of the four-part validation (i.e., weight of evidence), the performance of CALPUFF was judged to be superior to that of AERMOD.
Use of the nonsteady-state CALPUFF model in the near field (<50 km) for regulatory applications has been limited because of the lack of appropriate model validation studies. Considered an alternative model by EPA, use of CALPUFF for regulatory purposes in the near field must be supported by a relevant performance evaluation using measured air quality data. This validation study should help address the lack of information on the performance of CALPUFF in near-field applications. The potential problem with the use of the robust high concentration as a metric in model validations is also examined.
INTRODUCTION
Currently, there is a lack of detailed performance evaluations to support the use of CALPUFF in the near field. A U.S. Environmental Protection Agency (EPA) clarification memo statesCitation1:
“There has been no comprehensive demonstration made that the CALPUFF modeling system performs as well or better than AERMOD for near-field regulatory applications in complex wind situations based on field study data.”
This validation study addresses this deficiency by revisiting the Martins Creek air quality database. The study area encompasses portions of the Delaware River and the elevated terrain on either side of the river valley. The model evaluation utilized the following datasets collected from May 1, 1992 through May 19, 1993: hourly sulfur dioxide (SO2) measurements from eight ambient monitors, hourly SO2 emissions data from four facilities in the area, and hourly meteorological measurements from two locations. Although the emissions from four facilities have been included in the validation, over 98% of the SO2 emissions originate from two of the sources—the Martins Creek Power Plant and the Portland Power Plant. The Martins Creek databases have been used in previous model validation studies involving several different models, although none included CALPUFF.Citation2–5
The criteria used to judge a model's accuracy in this validation study reflect those used historically. These methods included recommendations in EPA's “Guideline on Air Quality Models”Citation6 and other techniques used by EPA and other AERMOD model validation studies.Citation2,Citation3,Citation5 An overall emphasis was placed on statistics that demonstrate a model's ability to reproduce the upper end of the concentration distribution. The model validation consisted of the following four parts:
1. | The actual monitored concentrations were compared with each model's predicted high and second-high 1-, 3-, and 24-hr and annual impacts. | ||||
2. | The monitored concentrations were compared with model predictions applying the statistical methodology used for long-term monitoring datasets in the principal AERMOD validation studies.Citation2,Citation3 | ||||
3. | The monitored concentrations were compared with model predictions following guidance in the EPA document “Protocol for Determining the Best Performing Model,” as recommended in Sections 3.2.1(a) and 3.2.2(d) of the “Guideline on Air Quality Models.”Citation6,Citation7 | ||||
4. | Several large datasets of predicted and monitored 1-hr concentrations were compared using statistical performance metrics calculated by the BOOT Statistical Model Evaluation Software Package, version 2. These evaluation techniques are also recommended in Sections 3.2.1(a) and 3.2.2(d) of the “Guideline on Air Quality Models.”Citation6,Citation8 |
The overall ability of CALPUFF and AERMOD to predict SO2 concentrations was judged on their performance in each of these four components (i.e., weight of evidence).
STUDY LOCATION AND DATASETS
shows a regional view of the Martins Creek, PA, validation study area—a rural, hilly area 90 km west of New York City, NY, on the New Jersey-Pennsylvania border. provides a closer look at the study area and its geographic features. The Delaware River transects the region. Both power plants in the study area—Martins Creek and Portland—are located on the Delaware River. The elevated terrain on either side of the river rises 400–500 ft above the valley floor in the northern portion of the study area near the Portland Power Plant. Further south near the Martins Creek Power Plant, major terrain features such as Scotts Mountain to the east of the Delaware River rise up to 1000 ft above the valley floor. The valley ridge to the west of the Martins Creek Power Plant is lower, ranging from 500 to 600 ft above the valley floor. Kittatinny Ridge, visible in the upper left corner of the figure, is the highest terrain feature in the area with elevations over 1500 ft above mean sea level (amsl), approximately 1200 ft above the Delaware River.
Figure 2. Location of SO2 sources, monitors, and meteorological stations used in the model validation study.
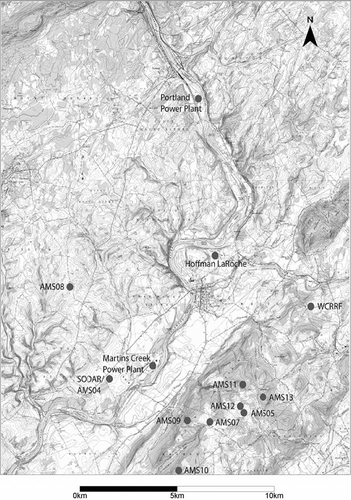
is an aerial photo that provides a greater understanding of the region's terrain, monitoring locations, and the spatial relationship between the two large power plants included in the validation. The photo was taken looking south through the center of the study area. The Portland Power Plant and Delaware River are in the foreground and the Martins Creek Power Plant is in the background. The high terrain to the left of Martins Creek Power Plant is Scotts Mountain, NJ, the location of seven of the eight SO2 monitors. On the high terrain along the right border of the photo is the location of the eighth SO2 monitor. This was the only monitor located in Pennsylvania. The distance between the four emission sources and eight monitors varied from 3 to 17 km.
Figure 3. Photo of the Portland Power Plant in the foreground and the Martins Creek Power Plant in the background.
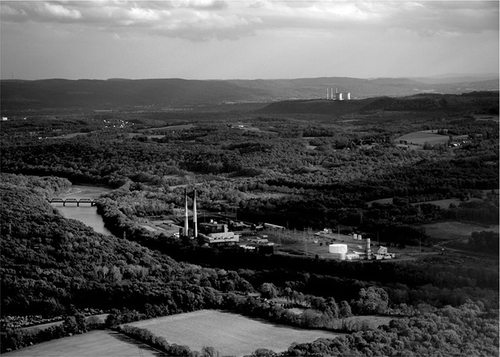
Sources and Emissions Data
The locations of the four SO2 sources in the model validation are shown in They are the Martins Creek Power Plant, the Portland Power Plant, the Warren County Resource Recovery Facility (WCRRF), and the Hoffmann-LaRoche Cogeneration Facility. Continuous emissions monitor (CEM) data, sometimes in combination with hourly load data, and fuel sulfur content were used to generate hourly SO2 emissions, stack temperatures, and exit velocities for these four facilities. All stacks were above their calculated Good Engineering Practice stack heights except for those at the Martins Creek Power Plant. However, the amount of downwash on these stacks has been judged to be extremely small. The previous AERMOD validation studies have classified the Martins Creek data as a non-downwash database.Citation2,Citation3
Review of the CEM emissions data used in previous Martins Creek validation studies discovered a significant error in the SO2 emission rate assigned to Portland Unit 1. The monthly emissions used in the previous validation studies were compared with data submitted by the previous owners of the Portland Power Plant to the Pennsylvania Department of Environmental Resources and EPA Region III.Citation9–11 On the basis of these data sources, the total emissions of SO2 from Unit 1 between May 1, 1992 and May 19, 1993 was approximately twice as high as that used in the previous validation studies (11,737 vs. 5773 t). As a result, the hourly emissions data of Unit 1 were adjusted to better reflect its true emissions during the validation period. No inaccuracies were found with Portland Unit 2’s hourly CEM SO2 emissions data.
The source characteristics of the Martins Creek Power Plant, the Portland Power Plant, the WCRRF, and the Hoffmann-LaRoche Cogeneration Facility are summarized in . Of the 52,101 t of SO2 emitted from these four facilities during the validation period (May 1, 1992 to May 19, 1993), over 98% were due to the Martins Creek Power Plant Units 1 and 2 and Portland Power Plant Units 1 and 2. Although they were potentially major emitters of SO2, Martins Creek Units 3 and 4 were relatively minor sources during the validation period. Units 3 and 4 were shut down approximately 85% of the time during the validation and operated at an 80% load or higher during only 5% of the validation period.
Table 1. Source characteristics and SO2 emissions
SO2 Monitoring Data
Also shown in is the network of eight SO2 monitoring stations where measurements were collected. These monitors are identified as AMS-5, AMS-7, AMS-8, AMS-9, AMS-10, AMS-11, AMS-12, and AMS-13. The AMS-5, AMS-7, AMS-9, AMS-10, AMS-11, AMS-12, and AMS-13 monitors were all sited on Scotts Mountain in Warren County, NJ. These seven monitors are 3–5.8 km east to southeast of the Martins Creek Power Plant and 13–17 km south of the Portland Power Plant. The elevations of these monitors range from 1120 to 1236 ft amsl, well above the heights of the Martins Creek stacks (840 ft amsl) and the Portland Power Plant stacks (694 ft amsl). Therefore, these monitors represent a complex terrain plume/receptor relationship. Although no violations of the SO2 National Ambient Air Quality Standards were measured during the of Warren County where these seven monitors are located was designated as a nonattainment area for SO2 in 1987 by EPA.Citation12 The designation was based on a screening modeling analysis, not monitoring data.
The AMS-8 monitor is located west of the Delaware River in Pennsylvania, 5.5 km to the northwest of the Martins Creek Power Plant and 11 km southwest of the Portland Power Plant. This monitor was not included in the previous AERMOD validation studies at this location.Citation2,Citation3 AMS-8 is unique because it is west of the Delaware River and is located on terrain at 810 ft amsl, much lower in height than the other seven SO2 monitors. The Martins Creek and Portland Power Plant stack tops are at the same approximate height as AMS-8. Although emissions from the Portland Power Plant contribute to some of the high concentrations at the complex terrain monitors, review of the wind direction data collected at AMS-8 and the sonic detection and ranging (SODAR) site strongly suggest that emissions from the Portland Power Plant are predominately responsible for all of the top 25 1-hr concentrations measured at AMS-8.
As with the previous validation studies, the lowest hourly concentration reported at any monitor was used as the hourly background concentration to reflect the regional-scale contributions from distant sources. The measurement at a monitor after background was subtracted was the value compared with the model predictions. No one monitor dominated the determination of the background values. The method of calculating background introduced uncertainty in the monitor's relatively low annual concentrations. Additional uncertainties result from the detection level of the SO2 monitors (∼16 μg/m3) and the zero baseline drift as high as 26 μg/m3 of the monitors.Citation2 As a result, this validation study focused on the much higher magnitude shorter-term averaging times that are relatively insensitive to these uncertainties.
Meteorological Data
Similar to previous validation studies at this site, meteorological data collected from May 1, 1992 through May 19, 1993 were used.Citation2–5 The SODAR and 10-m tower data from AMS-4 were used by CALPUFF and AERMOD's meteorological preprocessor programs (CALMET and AERMET). The AMS-4 site is located within the Delaware River Valley at a base elevation of 320 ft amsl (see ). The SODAR data consist of wind speed and wind direction at 30-m height increments from 90 to 420 m above ground. The 10-m tower data consist of 10-m wind speed, wind direction, standard deviation of the horizontal wind direction (σ-theta) and temperature data. Wind measurements taken at AMS-8 at a base elevation of 810 ft amsl were also input into CALMET. Temperature, wind speed, and wind direction were collected at the 10-m level at AMS-8.
Examining wind roses generated from the available meteorological data provides a better understanding of the complex wind flows within the modeling domain. contains the following four wind roses: (1) the AMS-4 10-m tower, (2) the 150-m SODAR, (3) the 300-m SODAR, and (4) the AMS-8 10-m tower data. As would be expected in a river valley, the 10- and 150-m (840-ft amsl) SODAR measurements at AMS-4 show a pronounced northeast/southwest axis corresponding to an up-valley/down-valley flow. The higher-level 300-m SODAR wind rose from AMS-4 data shows minimal terrain influence. Because winds at this 300- m level (1300 ft amsl) are above the highest terrain on either side of the Delaware River Valley, they reflect much more of a synoptic wind pattern. The 10-m AMS-8 wind rose, measured at the ridge location, most closely resembles the regional, synoptic wind direction distribution of the 300-m SODAR data, not those of the in-valley AMS-4 10-m tower data or 150-m SODAR data. In combination, these wind roses demonstrate that the significant terrain features in the area are capable of generating terrain-induced winds and nonsteady-state wind fields.
MODEL SETUP AND PROCESSING OF METEOROLOGICAL DATA
CALPUFF
CALMET version 5.8 was used to develop the wind fields for the model validation. Along with the 10-m data from AMS-4 and AMS-8, the SODAR data from AMS-4 were incorporated into CALMET using the subroutine PROF2UP. PROF2UP constructs a CALMET ready UP.DAT vertical wind and temperature file by incorporating the SURFACE and PROFILE files from the AERMET meteorological preprocessor. The SURFACE and PROFILE files were generated with the AMS-4 10-m tower data and SODAR measurements. Within the surface layer, wind and temperature profiles are assumed to follow surface similarity theory. Above the surface layer, simple profiling assumptions are used including winds that are constant with height and temperatures that decrease with typical atmospheric lapse rates. The change in mixing height over time determines where extrapolated profiles transition from below the surface layer to above the surface layer up to the top of the CALMET domain.
The effects of terrain on wind flow were accounted for in CALMET by setting options to run CALMET in diagnostic mode and by using a grid resolution small enough to resolve the terrain in the study area.
The CALMET 37.8- by 40-km modeling domain had a horizontal grid resolution of 200 m. The atmosphere was divided into 12 layers in the vertical. The grid cells were centered at the following heights above ground: 10, 30, 60, 107.5, 165, 225, 285, 360, 700, 1250, 1850, and 2600 m. This level of detail allowed CALMET to better incorporate the multilevel SODAR measurements at AMS-4. A TERRAD of 2.7 km was selected. Because of the complex terrain and the relatively narrow river valley near the sources, small values appropriate for near-field transport were assigned R1, R2, RMAX1, and RMAX2 weighing factors (RMAX1 and RMAX2 = 2 km, and R1 and R2 = 1 km). CALMET is unable to assign these interpolation values to individual meteorological stations; therefore, these weighing factors were also used at AMS-8.
The SO2 concentration predictions at the eight monitoring locations were made by CALPUFF version 5.8. The atmospheric chemistry option that includes conversion of SO2 to sulfate was turned off. The CALPUFF options that utilized similarity-theory-based dispersion coefficients (MISP = 2) and the probability density function convective boundary layer conditions (MPDF = 1) were selected. As a result, CALPUFF used a plume dispersion methodology similar to AERMOD. The EPA default switch that utilizes the partial plume path coefficient treatment in the dispersion calculations for receptors located in elevated terrain was selected. A complete description of the CALPUFF modeling setup methodologies and results are contained in the report “Validation of CALPUFF in the Near-Field,” available at the New Jersey Department of Environmental Protection (NJDEP) website.Citation13
AERMOD
The input file from EPA's 2003 AERMOD evaluation study was input into AERMOD, version 07026.Citation2 AERSURFACE (version 08009) was used to determine the surface characteristics (surface roughness, albedo, and Bowen ratio) for the area surrounding the AMS-4 meteorological tower. Surface roughness was determined in 30° sectors for a 1-km radius circle and the Bowen ratio and albedo were based on the average characteristics over a 10- by 10-km square centered on the observing site. The meteorological data were processed with the AERMET meteorological preprocessor (version 06341) to provide the “surface” meteorological data file for input into AERMOD.Citation14 The AERMET “profile” file was obtained from the EPA website (http://www.epa.gov/scram001/7thconf/aermod). A complete description of the AERMOD modeling setup methodologies and results is available at the NJDEP website.Citation13
MODEL UNCERTAINTY
A brief discussion on the sources of model uncertainty is appropriate before presenting the results of the model validation. There are four basic sources of uncertainty in air quality model validation studies: (1) model formulation uncertainty (how accurately do the model equations simulate atmospheric physics), (2) uncertainty in the representativeness of the model input data, (3) uncertainty in the measurements of the model input and monitoring data, and (4) uncertainty caused by the limitation that models can only characterize a portion of the naturally occurring variations in the atmosphere.Citation15,Citation16
Although a detailed analysis of these four factors is beyond the scope of this paper, there are several sources of uncertainty easily identified in this study. The assumption by AERMOD that meteorological data from AMS-4 are representative of the wind fields throughout the entire modeling grid is a source of uncertainty. The same uncertainty applies to CALPUFF, but to a lesser degree because of CALMET's use of data from AMS-8 and its ability to generate nonsteady-state wind fields on the basis of local terrain. The measurement uncertainties in quantifying the hourly SO2 emissions, especially from Portland Power Plant Unit 1, and the accuracy of the eight monitors' SO2 measurements are other sources of error in model predictions. This validation involves a very limited number of elevated point sources and a sparse, relatively nearby network of monitors. As a result, the effect of discrepancies between the measured wind direction and the actual wind direction can be significant. A plume traveling downwind typically expands at an angle of approximately 10°. In this situation, a discrepancy as small as 2° between the measured and actual wind direction can result in a large disagreement between the observed and modeled concentration.Citation16
MODEL VALIDATION RESULTS
Model Validation Part 1
Part 1 of the model validation included the comparison of predicted model concentrations with the network-wide actual high and second-high monitored values. The results of these comparisons are shown in . Although the CALPUFF predictions are marginally above and below the monitored values, all of the AERMOD predicted ratios except one represent an underprediction. The composite ratios for the two models reflect this fact. The CALPUFF composite ratio for all four averaging times is 1.01, whereas AERMOD's ratio is 0.85. The results suggest that AERMOD has a greater tendency to underpredict actual maximum concentrations than CALPUFF.
Table 2. Modeled high and second-high impact compared with network-wide observed high and second-high concentration
Model Validation Part 2
Part 2 of this validation study follows methods used in two previous AERMOD validation studies conducted at the Mar-tins Creek location.Citation2,Citation3 One of the primary statistical metrics used in the two previous studies was the robust high concentration (RHC). The RHC is designed to represent a “smoothed” estimate of the highest concentration on the basis of an exponential fit to the upper tail end of the concentration distribution. The RHC attempts to represent a stable estimate of the highest concentration, one that mitigates the unwanted influence of unusual events. As stated in the 2005 AERMOD validation study, “for regulatory applications, a good model would produce a concentration distribution parallel to the slope of the measured distribution and produce high-end concentrations (RHCs) that are similar to that of the observations.”Citation3
The RHC for modeling validation purposes was first defined in a paper by Cox and Tikvart.Citation17 The RHC is calculated in Equationeq 1 as follows:
where X(N) is the Nth highest value, X is the average of the N − 1 highest values, and N is the number of values exceeding a threshold value.
Validation studies that have used the RHC routinely select a value of N = 26 as the best representation of the upper-end distribution of concentrations.Citation2,Citation3 Although use of 26 as the value for N has been suggested in several documents, each noted that its selection is entirely arbitrary.Citation7,Citation17 The guidance given on the selection of N states that the value needs to adequately define the upper tail end of the distribution of concentrations. Because the upper tail-end distribution of monitored concentrations will vary by averaging time and location, the number of samples needed to define the upper end of the concentration distribution (N) will vary. Visual examination allows for the selection of a value for N that best defines the slope of the upper tail-end distribution. This technique will result in the calculation of a more accurate RHC.Citation18
An example of the importance of the value selected for N is given in This figure displays the 51 highest 24-hr concentrations measured at AMS-8, the monitor with the highest 24-hr concentrations. The figure also shows the calculated 24-hr RHCs for four values of N (6, 11, 16, and 26). The calculated RHCs range from 162.6 to 210.8 μg/m3. In this instance, a RHC value based on N = 16 was selected as most appropriate. The rapid increase in 24-hr monitored values beginning with the 16th highest concentration best defines the upper tail-end distribution.
lists the ratio of AERMOD and CALPUFF's network-wide RHC predictions for 3- and 24-hr time periods to the network-wide monitored RHC values for the same time periods. Although both models made fairly accurate predictions of the maximum 3- and 24-hr RHCs on a network-wide basis, CALPUFF's performance is superior to AERMOD's because its ratios are closer to 1. AERMOD shows a tendency to underpredict. The use of a more appropriate value for N in calculating the RHC and the inclusion of AMS-8 do contribute to an improvement in AERMOD's performance for this metric as compared with what was reported in the previous validation studies at Martins Creek.Citation2,Citation3
Table 3. Modeled RHC compared with network-wide observed RHC
Model performance was also judged with the network-wide quantile-quantile (Q-Q) plots. All of the 1-, 3-, and 24-hr observations and model predictions were ranked independent of time and space. The Q-Q plots are shown in Overall, the distribution of the predictions of AERMOD and CALPUFF are similar for all three averaging periods. Both models' predictions fall within a factor of 2 of the observations with the exception of the low-end distribution of 24-hr predictions. The underprediction by the models of the lowest monitored 24-hr concentrations is primarily because of the detection limit and the method of determining SO2 background in this study. The model predictions do deviate from each other at the upper end of the distributions. Concentrations at the upper end of the distribution predicted by CALPUFF tend to be higher than the AERMOD predictions for all three averaging times.
Model Validation Part 3
Part 3 of this validation study follows guidance in the EPA document “Protocol for Determining the Best Performing Model.”Citation7 The “Guideline on Air Quality Modeling” cites this document as providing statistical techniques for the evaluation of model performance in predicting peak monitored concentrations.Citation6 As with Part 2 of the validation study, the RHC is utilized for comparison of modeled concentrations to monitored concentrations. An additional statistic applied was the fractional bias (FB), a fundamental measure of the discrepancy between the average monitored and model-predicted concentrations and is given in Equationeq 2.
A FB of −0.67 is equivalent to an overprediction by a factor of 2, a FB of 0.67 is equivalent to an underprediction by a factor of 2. A FB of zero represents, on average, a perfect model prediction. A well-performing model should have a FB of less than 0.3.Citation19
The protocol requires the use of a composite performance measure (CPM). The CPM is calculated using the absolute fractional bias (AFB), a measure of the absolute discrepancy between monitored and modeled concentrations. A model's CPM has an operational and scientific component. The operational component is based on the highest measured RHC at any monitor and the highest model-predicted RHC at any monitor. This is done for the 3-and 24-hr averaging times. The scientific component only examines the 1-hr average RHCs under various atmospheric conditions. The operational component is considered the more important of the two and is given twice the weight of the scientific component. The algebraic expression for the CPM is given as
where (AFB)i,j is the AFB for meteorological category i at station j, (AFB)3 is the AFB for 3-hr averages, and (AFB)24 is the AFB for 24-hr averages.
The protocol also recommends that a bootstrap procedure be used to determine if the CPM difference (standard error) between models is statistically significant. The bootstrap technique involves resampling the monitoring and model prediction data and creating hundreds of artificial “trial” years. However, as discussed earlier, an arbitrary selection of N = 26 to define the slope of the upper tail end of a distribution when calculating the RHC is inappropriate. The range of monitored concentrations should be evaluated for each averaging time on an individual basis and a value of N should be selected that best defines the slope of the tail-end distribution of concentrations. Such an effort when conducted visually and involving hundreds of trial years is clearly impractical.Citation18
Operational Component
The comparisons between the highest monitored RHC and modeled RHC at an individual monitor are given in . Both models overpredict the monitored 3-hr RHC and underpredict the monitored 24-hr RHC. CALPUFF's predicted 3-hr RHC occurred at AMS-12. This was the only instance when either model was able to predict a maximum RHC at the same location as the monitored maximum RHC. The average AFB on the basis of the operational component comparison was 0.25 for AERMOD and 0.21 for CALPUFF. The lower average AFB indicates a slightly better performance by CALPUFF.
Table 4. Individual monitor's modeled RHC compared with the observed RHC
Scientific Component
The scientific portion of the CPM examined the 1-hr RHC for monitors located in two types of terrain under three different atmospheric stabilities. The first set of monitors was located on terrain well above the stack height of the principal sources of interest, the Martins Creek and Portland Power Plants. These monitors included AMS-5, AMS-7, AMS-9, AMS-10, AMS-11, AMS-12, and AMS-13. Monitor elevations ranged from 1120 to 1236 ft amsl and represent a complex terrain plume/receptor relationship. The second set comprised measurements taken near the stack-top elevation of the Martins Creek (840 ft amsl) and Portland Power Plant stacks (694 ft amsl). Only the SO2 monitor at AMS-8 (810 ft amsl) met that requirement.
Each hour of the evaluation period was designated as one of three sets of atmospheric stability: unstable, neutral, and stable. The classification of atmospheric stability was based on the Monin–Obukhov length (L) data contained in the AMS-4 “surface” meteorological file that were input into AERMOD. A value of 0.1 m approximates the average surface roughness surrounding the AMS-4 site. Assuming that value, the relationship of L to atmospheric stability was as follows: values of L between zero and −65 were designated unstable, values of L less than -65 and greater 65 were designated neutral, and values of L between zero and 65 were designated stable.Citation20
For the complex terrain monitors, shows that CALPUFF is relatively accurate in predicting the 1-hr RHCs during unstable and stable conditions but overpredicts during neutral conditions. AERMOD's performance is erratic. It severely underpredicts the 1-hr RHC during unstable conditions, the stability during which the highest 1-hr RHC occurred at the monitors. AERMOD shows overprediction of the monitors' RHCs during neutral and stable conditions.
Table 5. One-hour observed RHC compared with modeled RHC as a function of atmospheric stability
also compares the AERMOD and CALPUFF 1-hr RHC predictions to the 1-hr RHC of the stack-top monitor AMS-8. CALPUFF accurately predicts the 1-hr RHC during unstable conditions, whereas it underpredicts the 1-hr RHC during neutral and stable conditions. AERMOD moderately to severely underpredicts the 1-hr RHCs at this monitor during all three atmospheric stabilities. As discussed earlier, meteorological measurements suggest that emissions from the Portland Power Plant are responsible for the AMS-8 high SO2concentrations. The lack of SODAR data collected in the valley near the Portland Power Plant likely contributed to CALPUFF's inaccuracies in predicting maximum concentrations at the stack-top monitor (AMS-8). If such meteorological data were available, it would help CALPUFF to better characterize the complex winds in the modeling grid. As a steady-state model, AERMOD's predictions would not improve with this additional meteorological data because it cannot utilize more than one SODAR profile at a time.
The average AFB on the basis of the scientific component comparison was 0.58 for AERMOD and 0.37 for CALPUFF. If one considers the average FB instead of the average AFB for each model, the CALPUFF average FB of −0.04 is relatively unbiased, whereas the AERMOD average FB of 0.38 shows a bias toward underprediction.
CPM
Each model's AFBs were averaged for the operational component and scientific component and the CPMs were calculated. The lower CPM of CALPUFF (0.26) as compared with AERMOD's (0.36) signifies it is the better performing model.
Model Validation Part 4
The fourth part of the model validation study used the BOOT Statistical Model Evaluation Software Package, version 2, as distributed with the Model Validation Kit.Citation8 The original BOOT software program was based on recommendations by Hanna, but it has been upgraded to include additional performance measures and the implementation of the American Society for Testing and Materials (ASTM; 2000) model evaluation procedure. However, the ASTM procedures, best suited for tracer test experiments, were not used because of the long-term and limited number of monitors.Citation21–23
In this analysis, the BOOT program was applied to 1-hr predicted and monitored concentrations. The following statistical measures were evaluated: FB, the underpredicting component of the FB (FBFN), the overpredicting component of the FB (FBFP), and the normalized mean square error (NMSE). FBFP and FBFN are always positive and, when combined, are equivalent to the FB. Whereas the FB is a measure of mean relative bias or systematic bias, the NMSE is a measure of mean relative bias and random scatter. A NMSE value of zero indicates no scatter between observed and predicted concentrations (i.e., a perfect model). A NMSE less than 1 implies the magnitude of the scatter is less than the mean concentration.
Several large datasets were evaluated with the BOOT software. The first dataset was a comparison between the ranked maximum measured 1-hr concentrations at any of the eight monitors (background subtracted) with the maximum model prediction at any of the eight SO2 monitors for the same hour. Unlike the other parts of this validation study in which only the highest monitored values were of interest, uncertainties in the measurements at low SO2 concentrations are a concern. To remove the influence of the low-end, inexact monitoring values on the calculated statistics, only hours monitored in which the maximum concentrations were 42 μg/m3 and higher were included in the network-wide, ranked 1-hr dataset. This criterion removes the possible adverse effects of the SO2 monitor's 16-μg/m3 detection threshold and baseline (zero) drifts up to a magnitude of 26 μg/m3.Citation2 After exclusion of hours with these lower concentrations, a total of 2448 hr remained in the dataset. The BOOT statistics were calculated on these independent of time and space data pairs.
The 2448 hr were then divided into atmospheric stability categories using the L data from AMS-4 using the same methodology discussed in the scientific component of part 3 of the validation. On the basis of this metric, 810 hr were classified as unstable, 630 hr were classified as neutral, and 1008 hr were classified as stable.
summarizes the results of the models' performance for the three atmospheric stability regimes. The average of the observed network-wide 1-hr concentrations for each of the three stabilities are fairly uniform: 94.4 μg/m3 (stable), 101.8 μg/m3 (unstable), and 104.7 μg/m3 (neutral). Overall, CALPUFF did a better job of matching the average observed 1-hr concentration during each of the three stabilities than AERMOD. The CALPUFF NMSEs for all three stabilities are lower than those of AERMOD, especially during stable conditions.
Table 6. Summary of BOOT performance measures for 1-hr observed and modeled concentrations
AERMOD shows significant underprediction during the hours of unstable and neutral atmospheric stabilities. An FBFP of zero indicates that AERMOD underpredicted every hour during unstable and neutral conditions. AERMOD FB and FBFN during stable conditions show a pronounced overprediction of observed concentrations. This is not unexpected given the large overpredictions by AERMOD of the average 1-hr observed concentrations during stable conditions. The overprediction FBFP component of the FB far exceeds the underprediction component FBFN.
In , the FBs listed for each of the three stabilities reflect more accurate model predictions by CALPUFF than AERMOD. The FB values indicate that CALPUFF has a tendency to overpredict during unstable hours but makes relatively accurate predictions during neutral and stable conditions. The values of FBFP and FBFN demonstrate a more even distribution between under- and overprediction of 1-hr concentrations by CALPUFF as compared with AERMOD, the predictions of which for a given stability are dominated by under-prediction (unstable and neutral) or overprediction (stable). The overprediction by AERMOD in the stable case exceeds a factor of 2 (FB =-0.683). These results suggest that AERMOD may be producing the right overall concentration distribution for the wrong reasons.
The confidence limits of each model's FBs in were calculated using the bootstrap resampling technique. The boot-strapping algorithm was run to generate the 95% confidence limits to test whether, when compared with observations, each model's FB significantly differs from zero (i.e., the degree of bias in the predictions). Also tested was whether the differences in FBs between the two models significantly differed from zero (i.e., are the models predictions significantly different from each other?).
graphically depicts the FB confidence limits for the three 1-hr ranked network datasets of unstable, neutral, and stable conditions. Each bar represents the 95% confidence limits on the basis of the 2.5th through 97.5th percentile of the cumulative distribution function of the data. The darker shading represents the median to the 97.5th percentile, whereas the lighter shade represents the 2.5th percentile to the median. The narrower the bar, the more confidence there is in the model's predictions. For all three stabilities, the CALPUFF FB confidence limits are closer to zero than AERMOD's, indicating more accurate predictions. Because there is no overlap in the confidence limits, the CALPUFF FBs are significantly more accurate than the AERMOD FBs for all three stabilities.
Figure 7. One-hour FB confidence limits as a function of atmospheric stability: (a) unstable, (b) neutral, and (c) stable.
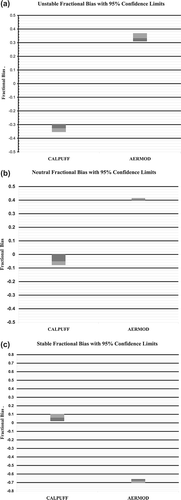
For two of the stabilities (neutral and stable), CALPUFF's FB confidence limits approach or cross the zero line, which indicates little bias in the predictions during those stabilities. Conversely, for all three stabilities, the AERMOD FB confidence limits do not meet the zero line, which suggests that the FBs are significantly different from zero and indicates a model bias in predicting concentrations during each of the stabilities.
CONCLUSIONS
Parts 1–3 of this model validation focused on model performance when predicting the high-end concentrations that are used to assess compliance with the ambient air quality standards. CALPUFF consistently showed high-end predictions and RHCs near measured levels with no obvious tendency to under- or overpredict. Although AERMOD's predictions are also relatively close to monitored levels on an absolute basis, the data demonstrate a tendency for AERMOD to underpredict the highest 3- and 24-hr monitored concentrations and the calculated 3- and 24-hr RHCs.
The ability to predict the 1-hr RHCs as a function of atmospheric stability and terrain elevation showed mixed results by both models. In complex terrain, CALPUFF showed high accuracy in predicting the 1-hr RHC during unstable conditions, a modest overprediction in stable conditions, and a large overprediction during neutral conditions. AERMOD's moderate overprediction of the 1-hr RHC during neutral and stable conditions contrasted with its severe underprediction of the unstable case, the atmospheric regime that produced the highest 1-hr RHC at the complex terrain monitors. At the stack-top monitor, both models underpredicted the 1-hr RHC for all stabilities except for CALPUFF in the unstable case.
Although an important metric in parts 2 and 3, problems were identified with the use of RHC in validation studies. Selecting an accurate value of N that represents the upper-end distribution of concentrations must be done independently for each dataset. In addition, determining whether the uncertainty of the two models' RHC predictions are statistically significant using a bootstrap technique is extremely difficult given the variation of N needed to define each resampled “trial” year's RHC value.
Evaluation of the large 1-hr datasets with the BOOT Statistical Model Evaluation Software Package in part 4 of the model validation found some of the same model behaviors as occurred when predicting the highest 1-hr concentrations. The NMSE indicated less scatter in the CALPUFF predictions for all three atmospheric stabilities. For all three atmospheric datasets, CALPUFF's FBs were closer to zero than AERMOD's. Consistent with the findings in part 3 of validation, AERMOD showed a bias toward underpredicting during unstable and neutral conditions and overpredicting during stable conditions. Although CALPUFF tended to overpredict during unstable conditions, its accuracy in predicting 1-hr concentrations during neutral and stable conditions was excellent.
The 95% confidence level of each model's FB indicated that CALPUFF's accuracy in predicting monitored concentrations was significantly better than that of AERMOD for all three stability datasets. In two of the stability datasets, the CALPUFF FB 95% confidence levels were at or very close to being insignificantly different from zero. Conversely, in all three cases, AERMOD's FB was significantly different than zero.
When judged on various comparisons and statistical measures applied in this validation study, the overall performance of CALPUFF at this location was superior to that of AERMOD.
REFERENCES
- Brode , R.W. and Anderson , B. 2008 . Technical Issues Related to CALPUFF Near-Field Applications , Research Triangle Park , NC : Memorandum; U.S. Environmental Protection Agency .
- AERMOD: Latest Features and Evaluation Results . EPA-454/R-03-003 . 2003 . U.S. Environmental Protection Agency; Office of Air Quality Planning and Standards: Research Triangle Park, NC
- Perry , S.G. , Cimorelli , A.J. , Paine , R.J. , Brode , R.W. , Weil , J.C. , Venkatram , A. , Wilson , R.B. , Lee , R.F. and Peters , W.D. 2005 . AERMOD: a Dispersion Model for Industrial Source Applications. Part II: Model Performance against 17 Field Study Databases . J. Appl. Meteor. , 44 : 694 – 708 .
- Ratte , M.A. and Murray , D.R. Air Quality Model Performance Evaluation and Comparison Study . TRC Project 14715-R61 . 1994 . Prepared by TRC Environmental Corporation, Windsor, CT, for Pennsylvania Power and Light Company: Allentown, PA
- 2007 . ADMS 4 Building & Complex Terrain Validation Martins Creek Steam Electric Station , Cambridge , , United Kingdom : Cambridge Environmental Research Consultants .
- Guideline on Air Quality Models. CFR, Part 51, Title 40, Appendix W, 2005.
- Protocol for Determining the Best Performing Model . EPA-454/R-92-025 . 1992 . U.S. Environmental Protection Agency; Office of Air Quality Planning and Standards: Research Triangle Park, NC
- Chang , J.C. and Hanna , S.R. 2005 . Technical Descriptions and User's Guide for the BOOT Statistical Model Evaluation Software Package July 10 Version 2.0
- 1992 PEDS Data Worksheet; Letter from J. West, MetEd/GPU, to T. DiLazaro, Pennsylvania Department of Environmental Resources Bethlehem District Office; February 22, 1993.
- 1993 PEDS Data Worksheet; Letter from J. West, MetEd/GPU, to T. DiLazaro, Pennsylvania Department of Environmental Resources Bethlehem District Office; March 7, 1994.
- Second Response of Reliant Energy, Inc., to the U.S. Environmental Protection Agency's January 10, 2001 Request for Information on the Seward, Portland, and Titus Stations; Letter from D.J. Jezouit, Baker Botts LLP, Counsel for Reliant Energy, to R.P. Killian of Region III; May 3, 2001.
- 1987 . Designation of Areas for Air Quality Planning Purposes; Revision to Section 107 Attainment Status Designations for the State of New Jersey (Final Rule) . Fed. Regist. , 52 : 49408 – 49411 .
- Validation of CALPUFF in the Near-Field; New Jersey Department of Environmental Protection: Trenton, NJ, 2010 http://www.state.nj.us/dep/baqp/petition/Exh%2012%20Validation_doc_050710_final.pdf (http://www.state.nj.us/dep/baqp/petition/Exh%2012%20Validation_doc_050710_final.pdf) (Accessed: 2010 ).
- Modeling Protocol in Support of Project Sequoia . TRC Project No. 160909 . 2008 . Prepared by TRC for PPL Corporation: Allentown, PA
- Fox , D.G. 1984 . Uncertainty in Air Quality Modeling . Bull. Amer. Meteor. Soc. , 65 : 27 – 36 .
- Canepa , E. and Irwin , J.S. 2005 . Air Quality Modeling. Volume II: Advanced Topics , Edited by: Zannetti , P. 503 – 556 . Pittsburgh , PA : EnviroComp Institute and A&WMA .
- Cox , W. and Tikvart , J. 1990 . A Statistical Procedure for Determining the Best Performing Air Quality Simulation Model . Atmos. Environ. , 24 : 2387 – 2395 .
- Cox, W., Air Quality Analysis Group, EPA OAQPS, personal communication, 2009 and 2010.
- Chang , J.C. and Hanna , S.R. 2004 . Air Quality Model Performance Evaluation . Meteorol. Atmos. Phys. , 87 : 167 – 196 .
- Brode , R. AERMET Training Class . Presented by U.S. Environmental Protection Agency; Office of Air Quality Planning and Standards, Air Quality Modeling Group to the NESCAUM Permit Modeling Committee . May 31 .
- Hanna , S. 1989 . R Confidence Limits for Air Quality Model Evaluation as Estimated by Bootstrap and Jackknife Resampling Methods . Atmos. Environ. , 23 : 1385 – 1398 .
- Standard Guide for Statistical Evaluation of Atmospheric Model Performance . D6589-00 . 2000 . American Society for Testing and Materials: West Conshohocken, PA
- Irwin , J.S. , Carruthers , D. , Stocker , J. and Paumier , J. 2003 . Applications of ASTM D6589 to Evaluate Dispersion Model Performance . Int. J. Environ. Pollut. , 20 : 4 – 10 .