ABSTRACT
Sampling and handling artifacts can bias filter-based measurements of particulate organic carbon (OC). Several measurement-based methods for OC artifact reduction and/or estimation are currently used in research-grade field studies. OC frequently is not artifact-corrected in large routine sampling networks (e.g., U.S. Environmental Protection Agency (EPA)'s Chemical Speciation Network). In some cases, the OC artifact has been corrected using a regression method (RM) for artifact estimation. In this method, the y-intercept of the regression of the OC concentration on the fine particle (PM2.5) mass concentration is taken to be an estimate of the average OC sampling artifact (net of positive and negative artifacts). This paper discusses options for artifact correction in large routine sampling networks. Specifically, the goals are to (1) articulate the assumptions and limitations inherent to the RM, (2) describe other artifact correction approaches, and (3) suggest a cost-effective method for artifact correction in large monitoring networks. The RM assumes a linear relationship between measured OC and PM mass: a constant slope (OC mass fraction) and a constant intercept (RM artifact estimate). These assumptions are not always valid. Additionally, outliers and other individual data points can have a large influence on the RM artifact estimates. The RM yields results within the range of measurement-based methods for some datasets and not for others. Given that the adsorption of organic gases increases with atmospheric concentrations of organics, subtraction of an average artifact from all samples (e.g., across multiple sites) will underestimate OC for lower-concentration samples (e.g., clean sites) and overestimate OC for higher-concentration samples (e.g., polluted sites). For relatively accurate, simple, and cost-effective artifact OC estimation in large networks, the authors suggest backup filter sampling on at least 10% of sampling days at all sites with artifact correction on a sample-by-sample basis as described herein.
This paper discusses options for OC sampling artifact correction in EPA's Chemical Speciation Network and elsewhere. Trip/field blanks account for artifacts associated only with transport, handling, and storage, but not artifacts that result from active sampling. Several organic artifact correction methods exist, including a linear RM that requires no additional sampling or chemical analysis. Previously unstated assumptions and limitations of this RM and guidance for those who wish to use it are described. However, the authors do not recommend the RM for future network operation; instead, intermittent artifact measurement and correction on a sample-specific basis is suggested.
INTRODUCTION
The U.S. Environmental Protection Agency (EPA)'s national Chemical Speciation Network (CSN; consisting of the Speciation Trends Network [STN] and the State and Local Air Monitoring Stations [SLAMS]) and the Inter-agency Monitoring of Protected Visual Environments (IMPROVE) network provide speciated fine particle (PM2.5) measurements for approximately 200 urban and 170 rural locations across the United States. One of the major PM2.5 components measured in these networks is particulate organic carbon (OC). Positive and negative sampling artifacts complicate OC measurement. Quartz-fiber filters (QFFs) are used to collect samples for OC analysis because they withstand the high temperatures used in thermal-optical carbon analysis.Citation1,Citation2 However, QFFs have large surface areas, and sorption of organic vapors (a positive artifact) on these filters is well documented (i.e., by the presence of OC on a QFF behind a front filter that removes the particles such that the QFF is exposed to particle-free airCitation3). In urban areas, adsorbed vapors frequently contribute on the order of 30–40% (1–3 μg/m3) of the OC collected on a QFF (20–40 cm/sec face velocity; 24-hr collection). Organic vapor adsorption can exceed 50% (0–2 μg/m3) in rural areas where OC concentrations are lower or in cases where samples are collected for shorter time periods or low flow rates (i.e., when particle loadings are smaller).Citation4 (Note that in experiments in which standard mixtures of gases and particles were sampled with increasing collection time, the mass of adsorbed vapor collected on a QFF was larger for longer collection times, but this adsorption artifact was a smaller percentage of the total collected OC for longer collection times, presumably because the particle deposition rate is constant and adsorption slows as the adsorbed phase approaches equilibrium with the gas phase.Citation4) Changes in ambient concentrations of organic vapors, temperature, and relative humidity (RH) can alter the partitioning of semi-volatile organic compounds, leading to additional adsorption of organic vapors on the filter and collected particles (positive artifact) or volatilization of adsorbed vapors and collected organic particulate matter (PM) from the filter (negative artifact). The pressure drop across the filter that can develop as particles are collected could also induce volatilization. Calculations and measurements suggest this effect is smaller than adsorption (i.e., 10% of collected OC) for typical ambient conditions, sampling face velocities, and collection times.Citation5–7 However, the magnitude of negative artifacts is particularly difficult to assess. For example, when adsorbable gases are removed with a denuder, collected particles are exposed to clean air, which enhances volatile losses. Also, PM lost by volatilization is not necessarily collected on a downstream QFF.Citation6 Measurement methods that account for adsorption and volatilization (described below) require considerable care and attention to detail. More measurements of this type collocated with undenuded QFF measurements are needed to document the net OC artifact on a QFF (by direct comparison) and to further validate simpler OC measurement methods.
Substrate handling, transport, and storage can also introduce positive and negative artifacts. Before sampling, QFFs are preheated in an oven at high temperature to remove any organic contaminants. CSN and IMPROVE QFF filters are prefired at 900 °C for 3–4 hr.Citation8,Citation9 These clean substrates can adsorb organic vapors during handling, transport, and storage. These “handling” artifacts are usually accounted for by laboratory, trip, and field blanks. For example, CSN filters are exposed to ambient air for up to several hours during these periods. After sampling, filters can adsorb additional organic gases or collected OC can volatilize if the equilibrium partitioning changes (e.g., if high temperatures are encountered during transport, handling, and storage, or if filters are somehow exposed to high or low organic concentrations). For these reasons, most research-grade studies take great care to store and transport clean and collected substrates in well sealed containers and at reduced temperatures. For the national networks, all CSN filters are shipped to and from the sites in their sampling cassettes in sealed Ziploc plastic bags in coolers with blue ice by overnight carrier with temperatures not to exceed 4 °C. Filters are stored cold ( 0 °C) before and after sampling. IMPROVE filters are sent and received between field sites and the University of California (UC)–Davis in their sampling cassettes in sealed Ziploc plastic bags at ambient temperature by standard U.S. mail in plastic containers designed for durability but not thermal stability. Shipping between UC–Davis and the Desert Research Institute (DRI), where IMPROVE filters are prepared, stored, and analyzed, is done at reduced temperatures. These filters are stored at DRI below 0 °C.
Accurate measurement of particulate OC on QFFs requires that artifacts are reduced and/or quantified. Research-grade field projects, which are limited in duration and well staffed (e.g., PM Supersite Experiments),Citation5 use denuder systems or backup filters (dynamic blanks) to correct for artifacts on a sample-by-sample basis. To date, these approaches have been considered too difficult and costly for large routine monitoring networks (e.g., CSN, IMPROVE). CSN samples for carbon analysis are collected on a single QFF. Field and trip blanks are used to assess artifacts associated only with transportation, handling, and storage, but artifacts associated with active sampling are not measured. Field and trip blanks are each collected with approximately 10% of samples. They are not subtracted from the reported OC concentrations, but they are reported in EPA's database (Aerometric Information Retrieval System) and are frequently subtracted by analysts. Statistical summaries of trip and field blanks are also available.Citation10 In the IMPROVE network, quartz backup filters (QQBs) are collected with every sample at six sites to provide a measure of the adsorption artifact. These backup filters are averaged monthly across the six sites and are subtracted from all samples at all sites on a peak-by-peak basis (i.e., OC1, OC2, OC3,…; each peak represents carbon evolved at a different temperature step during thermal-optical analysis).Citation2 (Note: To obtain better agreement between CSN and IMPROVE monitoring networks for OC and elemental carbon, EPA has converted all carbon measurements at the 54 STN sites and eventually at SLAMS sites to IMPROVE sampling and analysis protocols. Some differences, such as shipping protocols, remain unchanged [http://www.epa.gov/ttn/amtic/specurg3000.html].)
Regression Method for Organic Artifact Estimation
Solomon et al.Citation11 applied a linear regression approach to correct CSN network OC measurements for organic artifacts because sampling artifacts were not measured (only trip and field blanks were collected). In the regression method (RM) approach, the QFF OC concentrations (front-filter OC without blank correction) were regressed on PM2.5 mass concentrations (measured gravimetrically on Teflon filters). This method assumes that when the PM2.5 mass is zero, OC mass also should be zero. Therefore, the linear regression of measured OC (QFF; y-axis) on PM2.5 mass (Teflon; x-axis) should have a y-intercept that represents the average net (positive and negative) OC artifact integrated across handling, shipping, storage, and sampling effects. Examples of the RM are provided below.
In this method, an average OC artifact estimate (a constant value given by the y-intercept of the regression of OC on PM2.5 mass) is subtracted from each QFF OC concentration for the set of samples included in the regression. When a negative intercept results based on the RM, the absolute value of the intercept is added to the front QFF because, in this case, volatilization is assumed to be greater than adsorption. This method assumes that the Teflon filters on which PM2.5 mass is measured adsorb a negligible quantity of organic vapors (a reasonable assumptionCitation12), that volatile losses (e.g., of OC and nitrate) from Teflon filters are negligible, and that PM2.5 mass measurements are accurate. The RM has been used by several researchersCitation11,Citation13,Citation14 and is attractive because it provides a “net artifact estimate” at low cost relative to the need to collect and analyze additional filters and/or use denuders. This paper explores additional assumptions and limitations of the RM and discusses biases that result from the subtraction of an average artifact—an issue that also pertains to other artifact correction approaches in current use.
Measurement-Based Artifact Correction Methods
Several artifact correction methods have been used to estimate “true” or “artifact-corrected” particulate OC concentrations when OC is measured on QFFs.Citation7,Citation11,Citation15,Citation16 Good agreement (within ∼10%) has been found between research-grade measurements of OC that (1) correct for adsorption through backup filter subtraction and (2) correct for adsorption and volatilization using a denuder followed by an adsorbent and simultaneously measure denuder breakthrough in a second channel (i.e., Pittsburgh Air Quality Study [PAQS] Citation5 and ACE-AsiaCitation6). These and other approaches are described below.
Figure 1. PAQS artifact-corrected OC calculated as Q – QQB (□) and Q – TQB (Δ) and from the denuder sampler (Equationeq 5). Adapted with permission from Subramanian et al.Citation5 First published in Aerosol Science and Technology, 38 (suppl 1), 27-48, 2004. Copyright 2004 Taylor & Francis, Aerosol Science and Technology.
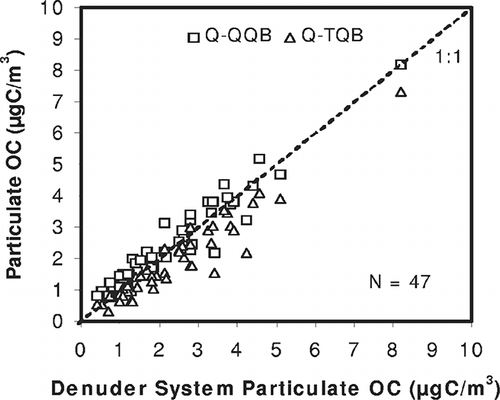
Filter with Trip or Field Blanks
Trip and/or field blanks are usually used to estimate OC artifacts associated with the transport, handling, and storage of filters. However, these blanks do not experience changes that are associated with sampling, such as changes in ambient organic vapor concentration, increases in pressure drop related to filter loading, and changes in ambient temperature during sampling. Trip blanks are transported and stored with samples but are not exposed to ambient air outside of the laboratory. Field blanks are treated similarly to trip blanks; however, they are mounted in the sampler usually for seconds to minutes and then resealed in their original shipping container. CSN field blanks are handled in this way. In the most realistic field-blank protocols, air is pulled through the sampler for a few minutes with the field blank in place. Averaged trip or field-blank OC is usually subtracted from samples when OC is measured on a single QFF.
Filter with Backup Filter
In this approach, the OC adsorption artifact on a QFF is taken to be the OC measured on a concurrently collected quartz fiber backup filter, also called a “dynamic blank” (). Unlike field and trip blanks, dynamic blanks remain in the sampler throughout sampling and experience essentially all of the sampling, transport, handling, and storage conditions as the front filter. The dynamic blank is the OC measured on a QFF downstream of a QFF (QQB), or OC measured on a QFF downstream of a Teflon filter (TQB), the latter in a parallel sampling port. The quartz or Teflon front filter removes particles so that the backup filter collects only QFF adsorbable vapors. Artifact-corrected OC is calculated as the OC on the front QFF (Q) minus the OC on the concurrently collected backup filter (QQB or TQB; ).
Figure 2. (a) Dynamic blank sampler and (b) denuder system configurations used to estimate OC artifact during PAQS. Filters are labeled as follows: Q = QFF, T = Teflon filter, QQB = quartz quartz backup, TQB, Teflon quartz backup, ADS = CIG adsorbent.
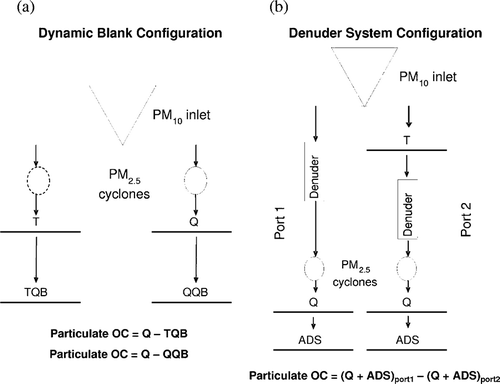
This approach assumes that the amounts of organic vapor adsorbed on the front and the backup filter are equal and that OC lost from particles as a result of volatilization is negligible.Citation4 Evidence suggesting that volatilization from undenuded QFFs is small was reported by studies operating side-by-side denuder and filter samplers,Citation5,Citation6,Citation18 although it must be recognized that there are only a few studies of this type and that they are extremely difficult to do well. Adsorbed OC can be lower on backup filters than on front filters as discussed below. Turpin et al.Citation4 recommend collection of a dynamic blank (backup filter) with each sample and subtraction on a sample-by-sample basis because the amount of adsorption depends on the ambient concentration, the composition of semi-volatile organics, and on temperature, which also varies from sample to sample. Kirchstetter et al.Citation3 suggest selecting front and backup QFFs from the same lot before sampling because of interlot variability of QFF adsorption capacity.
Differences between TQB and QQB OC artifact estimates have long been recognized and are not completely understood. One might expect less adsorption on a TQB filter because of the smaller surface area and larger pressure drop across a front Teflon filter than across a front QFF. However, OC on a QQB is typically lower than OC on a TQB,Citation3,Citation12 especially for samples with low sample volumes. Two studies comparing artifact-corrected OC obtained from TQB and QQB approaches () with a denuder-based approach () concluded that the TQB approach is more accurate for low sample volumes (e.g. 4-hr duration, 10–20 L/min, 47-mm filters).Citation5,Citation6 This finding is consistent with the argument that it takes longer for a front QFF than a front Teflon filter to reach equilibrium with the sampled airstream and before that time the backup filter behind the QFF is exposed to a reduced concentration of organic vapors.Citation3,Citation7,Citation12,Citation19,Citation20 Differences between TQB and QQB artifact estimates are smaller for larger sample volumes. Subramanian et al.Citation5 found better agreement between the QQB approach and the denuder-based method for 24-hr samples (16.7 L/min, 47-mm filters), as shown in . The QQB approach appears to be a reasonable choice for CSN-style sampling.
Denuder-Filter-Adsorbent
A denuder upstream of the QFF (port 1; ) has been used to substantially reduce the concentrations of organic vapors passing through the QFF.Citation5,Citation6,Citation16,Citation18 This reduces the adsorption artifact but also disturbs the equilibrium partitioning of semi-volatile compounds and can induce volatile loss of semi-volatile compounds from collected particles. If the denuder is 100% efficient at removing adsorbable vapors, OC collected on an adsorbent downstream of the denuder and filter (ADS, port 1 of ) can be attributed to volatile losses from collected particles. If the denuder is not 100% efficient at removing vapors that can adsorb to the QFF and ADS (i.e., if denuder breakthrough occurs), then organic gases that pass through the denuder can also adsorb on the QFF and adsorbent. The degree of denuder breakthrough can be determined in a parallel sampling port (port 2; ) that is identical to port 1 except for the placement of a Teflon filter upstream of the denuder. In port 2, particle-free ambient air (containing organic gases) enters the denuder, which is followed by a QFF and an adsorbent. OC measured on the QFF and adsorbent in port 2 is a measure of adsorbed vapor present because of denuder breakthrough. Artifact-corrected OC is then calculated as the sum of OC on the front QFF (Q) and adsorbent (ADS) in port 1 minus the sum of OC on the QFF (Q) and adsorbent (ADS) in port 2.
The two-channel denuder-QFF-adsorbent method, which corrects for denuder breakthrough, is considered the best current method for estimating ambient concentrations of OC in air, at least in principle, because it accounts for adsorption and volatilization. However, maintaining adequate quality control in such a system is extremely challenging. This approach is similar to the denuder-adsorbent filter method preferred for aerosol nitrate measurement.Citation21 If denuder breakthrough is not measured, denuder sampling (port 1 only) assumes (1) the denuder removes 100% of gases adsorbed by a QFF and ADS and (2) the ADS collects 100% of the organic vapors volatilized from the collected particles during sampling.Citation6 It is important to note that with even modest denuder breakthrough, the contribution of breakthrough gases to ADS can equal or exceed the contribution of particulate OC volatilized from Q because organic vapor concentrations typically greatly exceed organic PM concentrations in the atmosphere.Citation22 Denuder collection efficiencies decrease with increasing temperature and RH and are composition-dependent. Thus, field measurement of denuder breakthrough (e.g., as described above [port 2]) is important. The use of efficient adsorbants also makes it difficult to prevent contamination of the ADS during transport, handling, and storage. Several types of denuders (e.g., parallel plate, annular, and honeycomb) containing adsorbent materials such as carbon-impregnated cellulose fiber (CIF), carbon-impregnated glass fiber (CIG), polyurethane foam (PUF), activated carbon monolith honeycomb, and polystyrene divinylbenzene resin (XAD)Citation5,Citation6,Citation16 are com monly used. QFFs, CIG, CIF, XAD, and PUF also are used as the adsorbent downstream of the QFF.Citation5,Citation6,Citation16,Citation23,Citation24 De nuder collection efficiencies for filter-adsorbable organic vapors of 80–100% have been reported.Citation5,Citation6,Citation16,Citation23,Citation24
EXPERIMENTAL WORK
Objectives
The purpose of this paper is to discuss options for artifact correction in large routine sampling networks. Specifically, this paper discusses the assumptions and limitations inherent to the RM, compares RM performance with measurement-based methods for a few studies, provides some guidance to those using the RM, describes other approaches to artifact correction, and suggests a strategy for artifact correction in large networks going forward. To accomplish these goals, datasets from PAQS, the Relationship of Indoor and Outdoor Personal Air (RIOPA) study, and a six-site STN/IMPROVE comparison study were used. Key measurement parameters are summarized in .
Table 1. OC measurements during the PAQS, the RIOPA study, and the six-site STN/IMPROVE comparison study
PAQS Samples
Twenty-four-hour integrated concentrations of PM2.5 mass and chemical composition were measured daily for 14 months during PAQS.Citation5,Citation25–28 In general, PM2.5 at the primary PAQS site was heavily influenced by long-distance transport and atmospheric processing.Citation27 OC (<2.5 μm in aerodynamic diameter) was measured daily using filter plus backup filter approaches (QQB and TQB) () and a two-port denuder-filteradsorbent sampler (activated carbon monolith honeycomb denuder, MastCarbon, Ltd.; CIG adsorbent, Schleicher & Schuell) that allowed correction for denuder breakthroughCitation5 (). Artifact-corrected OC was calculated with Equationeqs 3 Equation Citation5. Artifact-corrected OC calculated from Equationeq 3 (Q - QQB) agreed best with artifact-corrected OC concentrations obtained by the denuder sampler for this study (Equationeq 5; ; 24-hr samples).Citation5
All sample lines were operated at 16.7 L/min (47-mm diameter filters mounted to provide a 29-cm/sec face velocity). QFF (Pallflex; 550 °C in air; >4 hr) and CIG filters (360 °C in ultrahigh purity nitrogen; >12 hr) were baked before use. QFF substrates were stored at room temperature in clean, sealed glass jars. CIGs were initially stored at room temperature and later in the study were stored frozen. CIG substrate storage temperature did not have a significant effect on the handling blanks.Citation5 After collection, all samples were stored cold (−20 °C), and OC was measured by thermal-optical transmittance.Citation5 CIGs were also analyzed thermally (330 °C in He).Citation5 Note that in the results reported by Subramanian et al.,Citation5 PAQS filter data were corrected by the average field blank, whereas in this work, uncorrected filter data were used.
RIOPA Samples
As part of RIOPA,Citation29–32 48-hr integrated outdoor PM2.5 samples were collected outside of homes in Elizabeth, NJ; Houston, TX; and Los Angeles County, CA (summer 1999 to spring 2001). Many homes were particularly close (<200 m) to identified outdoor sources, such as congested highways, gas stations, refineries, and truck loading facilities.Citation30 Samples for PM2.5 mass (gravimetric analysis) were collected on a Teflon filter,Citation29,Citation31 OC (<2.5 μm aerodynamic diameter) was collected on a QFF, and the adsorption artifact was measured on a TQB.Citation32 Artifact-corrected OC was calculated from Equationeq 4.
All samples were collected at 10 L/min (37-mm diameter; 25-cm/sec face velocity). Pallflex QFFs were prebaked (550 °C, >2 hr) and stored in Petri dishes lined with baked aluminum foil in sealed plastic bags at room temperature until sampling.Citation32 QFFs were removed from the sampler immediately after sampling, stored frozen on-site in the same container as initially used, transported cold (blue ice) the next day by express carrier, and stored frozen until analysis. OC was measured by thermal-optical transmittance.Citation32
Six-Site STN/IMPROVE Comparison Study
Time-integrated 24-hr measurements of PM2.5 mass, QFF OC, and other PM2.5 species were measured using collocated STN and IMPROVE samplers approximately every third day in the six-site STN/IMPROVE comparison study.Citation33 Sites were located at three urban STN and three rural IMPROVE locations. The urban and rural sites were paired and located as follows: Beacon Hill, Seattle, WA and Mount Rainier, WA; Phoenix, AZ and Tonto, AZ; and Haines Point, Washington, DC and Dolly Sods, WV.
Data from October 2001 to October 2002 were used to calculate RM artifact estimates. OC and PM2.5 mass were measured on 47-mm diameter QFF and Teflon filters, respectively, by the following STN samplers: an Andersen reference ambient air sampler (10.4-cm/sec QFF face velocity, 23.6-cm/sec Teflon face velocity; Dolly Sods, WV, and Haines Point, Washington DC), a MetOne spiral aerosol speciation sampler (9.5-cm/sec face velocity; Phoenix, AZ, and Tonto, AZ), and a URG mass aerosol speciation sampler (23.6-cm/sec face velocity; Beacon Hill, WA, and Mount Rainier, WA). All samplers were operated at manufacturer-recommended flow rates. Details regarding the CSN (STN) speciation samplers can be found elsewhere.Citation11,Citation34 All QFFs (Whatman; prebaked at 900 °C) were stored below freezing in the laboratory before and after sampling, transported cold using blue ice, and removed from the samplers typically within 3 days. During handling, samples are exposed to room air in a large warehouse for up to a couple of hours. Samples were analyzed by thermal-optical transmittance.Citation35
RESULTS
Artifact Estimates
In PAQS and RIOPA ( and ) the field blanks, which only provide a measure of OC artifacts on QFFs because of transit, handling, and storage, are 3–4% of front QFF OC, or 0.10–0.22 μgC/m3. This is a modest contribution to the total measured artifact (i.e., TQB, QQB, denuder). For PAQS the average total measured artifact is 0.48–1.03 μgC/m3, or 15–34% of front QFF OC depending on the method used to estimate the OC artifact. For RIOPA, the average total measured artifact is 31–43% or 1.65–2.18 μgC/m3 depending on the city (). Field blanks were a much higher percentage of front QFF OC in the six-city STN/IMPROVE comparison study (). For Haines Point, Dolly Sods, Phoenix, and Tonto, the site average field blanks are 1.3–1.4 μgC/m3, which is 27–54% of front QFF OC. For Beacon Hill and Mount Rainier, the site average field blanks are 0.1–0.3 μgC/m3 (7–11% of front QFF OC). The use of a different type of filter or differences in sample handling and storage might explain the higher field blanks for CSN samples. Note, as seen here and reported elsewhere,Citation11 CSN field blanks are sampler specific with URG having the lowest blank values and Anderson and MetOne having higher and more similar values.
Table 2a. PAQS artifacts. Regression of measured QFF OC concentration (μgC/m3) on PM2.5 (μg/m3) mass by standard LLSR, Deming regression (accounting for measurement uncertainties), and LLSR and Deming regression after outlier removal by robust regression for PAQS
Table 2b. RIOPA artifacts. Regression of measured QFF OC concentration (μgC/m3) on PM2.5 (μg/m3) mass by standard LLSR, Deming regression (accounting for measurement uncertainties), and LLSR and Deming regression after outlier removal by robust regression for RIOPA sites
Table 2c. Six-site STN/IMPROVE comparison study. Regression of measured QFF OC concentration (μgC/m3) on PM2.5 (μg/m3) mass by standard LLSR, Deming regression (accounting for measurement uncertainties), and LLSR and Deming regression after outlier removal by robust regression
PAQS and RIOPA studies included denuders and backup filters, enabling estimates of OC sampling artifacts and transport, handling, and storage artifacts. Specifically, the undenuded RIOPA and PAQS backup filters provide estimates of the positive sampling artifact together with the handling blank. Differences between undenuded QFF OC and PAQS artifact-corrected OC obtained using Equationeq 5 provide estimates of the net artifact, accounting for adsorption and volatilization during sampling and the handling blank. RM performance is examined against these more comprehensive artifacts in the following paragraph.
The simplest application of the RM is to regress OC on mass using linear least-squares regression (LLSR) as shown in the first row of for each dataset. These results are discussed here, and alternative approaches are discussed below. The corresponding data are displayed in . (Note that the regression line shown in is not LLSR, but Deming, after outlier removal, which is discussed later.) The RM OC artifact estimate for PAQS by LLSR (36% of QFF OC; 1.1 ± 0.1 μgC/m3) is similar to artifact measurements made during PAQS (i.e., 15–33%; 0.48 –1.03 μgC/m3 for QQB, TQB, and denuder; and ) and those made at similar face velocities and OC loadings in other studies.Citation6,Citation7,Citation12,Citation13 Note that although the standard deviation in TQB or QQB values across days () reflects the daily variations in the size of the sampling artifact, the variations in the artifact estimate across methods (QQB, TQB, denuder) gives some perspective on the inherent uncertainly in artifact measurements.
Table 3. OC estimates by denuder (Equationeq 5), by backup filter subtraction (Q-TQB and Q-QQB), by RM (Deming robust regression), and uncorrected front QFF OC for PAQS and RIOPA
Figure 3. Measured front QFF OC (μgC/m3) and PM2.5 mass (μg/m3) for (a) PAQS, (b–d) RIOPA sites, and (e–j) the six-site STN/IMPROVE comparison study. Dashed circles are outliers in x or y identified by robust regression. The solid line is the Deming linear regression of y on x performed without outliers. The RM estimate is the Deming regression y-intercept value after outlier removal by the robust regression (). The dashed line is the RM artifact estimate (y-intercept of regression; see also ). In the RM, this value is subtracted from each sample. Note OC concentrations that fall below this line will be negative after RM artifact correction. Dashed squares indicate points not identified as outliers but with a large influence on the regression.
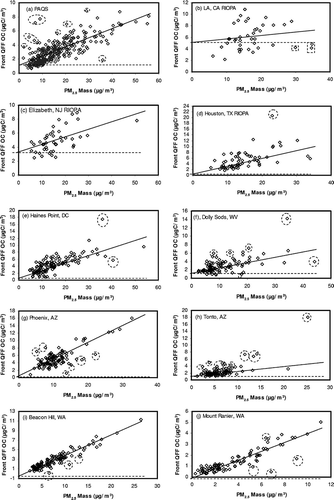
RM artifact estimates were substantially greater than measurement-based artifact estimates when the method was individually applied to data from two of the three RIOPA cities. For the RIOPA California dataset the RM artifact estimate was 87% of QFF OC (5.3 ± 1.0 μgC/m3), for New Jersey it was 62% of QFF OC (3.1 ± 0.5 μgC/m3), and for Texas it was 27% of QQF OC (1.4 ± 0.7 μgC/m3); the measurement-based artifact at those sites (TQB) was 31–43% (1.7–2.2 μgC/m3). After subtraction of the RM artifact estimate from the Los Angeles County QFF OC values, 16 of 40 artifact-corrected OC concentration estimates were below zero (; data below dashed line will be negative after RM artifact subtraction). These results indicate that, relative to the backup filter method, the RM approach overestimated the OC artifact and underestimated OC at RIOPA sites. It should be noted that there is substantial scatter in the RIOPA data (r2 = 0.02–0.36 for RIOPA datasets; r2 = 0.35–0.87 for others), which adds uncertainty to the intercept estimate. This scatter is likely because many RIOPA study homes are in close proximity to sources. Because the contribution of local sources is expected to be quite variable, the OC mass fraction in the RIOPA datasets is expected to be more variable. Also, the number of samples in the RIOPA datasets is smaller (N = 40–61) than in the other datasets (N = 99–301). The limitations and assumptions of the RM approach are examined in the next section.
RM Limitations
x and y Value Uncertainties
One limitation of the RM is that standard LLSR takes into consideration uncertainties only in the y variable. However, PM2.5 mass (x-axis) and front-filter OC (y-axis) variables have inherent measurement uncertainties, and this can affect y-intercept values. The Deming regression provides a more exact solution of the linear least-squares problem and allows for incorporation of measurement uncertainties in both variables.Citation37–40 Measurement uncertainties are study specific. They were similar for PAQS, RIOPA, and the six-site STN/IMPROVE comparison study. Measurement uncertainties used here for front-filter OC (7%) and PM2.5 mass (5%) were estimated by propagation of error and included a 5% sampling uncertainty (driven by variations in flow rateCitation32) and an analytical uncertainty of 5% for OCCitation5,Citation32,Citation36 and 1% for PM2.5 massCitation29 determined from replicate analysis. Larger uncertainties of 9 and 14% (reported as average relative difference, %) have been reported for CSN PM2.5 mass and OC, respectively, on the basis of collocated samples.Citation17,Citation41 For the Deming regressions performed in this study, uncertainties estimated by propagation of error (7% for OC and 5% for PM2.5 mass) were used because they were available for all three studies.
RM artifact estimates (y-intercepts) obtained by Deming and by LLSR were virtually identical with the exception of Houston (), Phoenix, and Tonto (), where Deming regressions produced lower artifact estimates than LLSR, although not statistically different. (Note that Deming and LLSR results were never significantly different given the large uncertainties in the intercepts.) In general, Deming regression is preferred over standard LLSR whenever x and y variables have uncertainties. If the uncertainty in x is much smaller than the uncertainty in y, similar slopes are expected from LLSR and Deming.
Outliers and Influential Points
A second limitation of the RM is that a few values can have a disproportionate influence on the slope and intercept in linear regression, especially when the dataset is small. For the purpose of obtaining the integrated net artifact estimate by the RM, outliers should be identified statistically and excluded from the regression. In this work least-trimmed squares robust regression was used to identify outliers in x and y (SAS; SAS Institute, Inc., version 9.1). Measurements with a standardized robust residual greater than 3 (default setting) were considered outliers.
The removal of outliers (circled data in ) had only a modest effect on the RM artifact estimate (y-intercept) for most examined datasets (; compare LLSR with robust and Deming with Deming robust). The exceptions were Phoenix and Tonto (STN), where the Deming regression intercept changed from 0.75 ± 0.38 to 0.21 ± 0.30 for Phoenix and from −0.08 ± 0.31 to 1.43 ± 0.14 for Tonto with removal of outliers. Note that Phoenix and Tonto had some of the larger intercept uncertainties of the study, although the coefficients of determination (r2) between OC and mass did not distinguish these sites from the others.
Even after statistically identifying and removing outliers, a few unusual sampling days can have a disproportionate influence on the RM artifact estimate, as demonstrated for the RIOPA California dataset (, “Deming w/o influential points”). For example, the removal of three data points in the lower right would reduce the RM artifact estimate (y-intercept) from 5.2 to 2.9 μg/m3, bringing it closer to the TQB estimate of 1.9 μg/m3. The removal of the six data points in the upper left would reduce the RM artifact estimate from 5.2 to 4 μg/m3. Certainly, points should not be removed subjectively (i.e., without a statistical basis), but this example illustrates the observation that RM artifact estimates have larger uncertainties for some datasets than others. The likelihood that a few unusual sampling days will alter the RM artifact estimate for an entire dataset is greater for datasets with low r2 and small N. It should be noted that even when outlier identification was performed using a narrower residual distance for outlier identification (SAS DEFFITS = 1, Studentized = 1.5) so that four outliers were identified, the RM artifact estimate for the California dataset remained above 5 μg/m3. The RIOPA Texas RM estimate was stable with respect to outliers and did not seem to be unduly influenced by individual points.
RM Assumptions
Linearity
The inherent assumptions of LLSR are that y values (front QFF OC concentrations) vary linearly with x values (PM2.5 mass concentrations) so that the slope and the intercept (RM artifact estimate) are constant. Because the slope in the RM method is the OC mass fraction (OC/PM2.5 mass), the RM assumes that the OC mass fraction is independent of PM mass. For example, if high PM2.5 mass concentrations are driven by high sulfate or nitrate without a concurrent increase in OC, the RM estimate (y-intercept) likely would be biased high because the OC mass fraction is lower on high-concentration days than on low-concentrations days, creating a nonlinear QFF OC-mass relationship. The opposite effect would occur when high PM2.5 mass concentration days are driven by high wood smoke alone (i.e., in this case, the RM estimate is expected to be biased low). In fact, this (high mass dominated by nitrate) might explain the nonlinear shape () and the very high RM artifact estimate found for the Los Angeles RIOPA data. Unfortunately this cannot be confirmed directly because nitrate was not measured in RIOPA, but RIOPA species mass balances have a larger unexplained fraction (primarily nitrate and water) on the highest 25th percentile PM2.5 concentration days.Citation32 In contrast, one might argue that the highest mass concentrations could be driven by wood smoke at sites such as Mount Rainier (from home heating, camping, and logging-associated burns outside of the park) and Beacon Hill, a Seattle neighborhood (from home heating) ( and ). If true, this would decrease the RM artifact estimate. The RM artifact estimate is quite small for Mount Rainier and negative for Beacon Hill, consistent with this scenario. However, if a nonlinearity exists in these OC-mass plots, it is not visually obvious. There are other possible explanations for the very small and negative RM artifact estimates at Mount Rainier and Beacon Hill, respectively. For example, volatile losses could be greater or the concentrations of adsorbable vapors could be smaller at these sites than at the other sites. These sites used the URG sampler, which operated at a higher face velocity.
Independence
The RM treats the organic artifact as a constant value, independent of the PM2.5 mass concentration or the sample loading. This will not be true if days with higher OC (and higher PM2.5 mass) also have higher concentrations of quartz-adsorbable OC vapors. Certainly adsorption theory and gas-particle partitioning theory suggest that days with higher particulate OC also have higher gaseous OC and on these days adsorption to QQFs is greater. Consistent with theory, adsorbed OC has been reported to increase with increasing front QFF OC at several locations,Citation4,Citation12,Citation13 including PAQS and RIOPA cities (). This increase is not typically linear. The observation of higher adsorbed OC loadings on higher concentration days is relevant to the RM artifact correction approach in two ways: (1) the RM involves subtracting a constant artifact estimate from all samples and therefore does not account for this dependence, and (2) the general increase in the OC artifact with concentration could make the OC-PM mass relationship nonlinear. The extension of the RM to negative artifacts (Beacon Hill) is also problematic in this regard. It is unlikely that the quantity of OC lost to volatilization will be independent of the amount of collected OC.
Figure 4. Measured organic adsorption artifact OC (TQB or QQB) and front-filter OC for (a) PAQS and (b–d) RIOPA sites. The increase in measured organic adsorption artifact with increasing front-filter OC (a measure of the organic pollution level) was significant (linear regression t test, α= 0.05) for PAQS and RIOPA sites. An increase in the actual organic adsorption artifact with organic pollution level violates an assumption of the RM of organic artifact estimation.
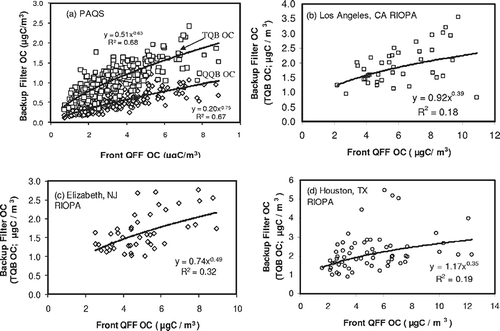
PM2.5 Mass
The RM also assumes that PM2.5 mass measurements (x values) are accurate. Although Teflon filters adsorb a negligible quantity of organic vapors, their higher pressure drop makes them more susceptible to volatile losses than QFFs.Citation12 Volatile losses of nitrate also can affect the accuracy of PM2.5 mass measurements. If PM2.5 mass measurements are biased low, RM artifact estimates will be biased high.
DISCUSSION
Subtraction of an Average Artifact
Given an increase in the adsorption artifact with increasing concentrations of organics, no matter what artifact correction method is used, subtraction of a single average artifact estimate from all samples (collected at a single site across all seasons or across multiple sites) would constitute an oversubtraction (i.e., underestimation of OC) at the lower OC concentrations and an undersubtraction (i.e., overestimation of OC) at the higher OC concentrations. For example, subtraction of a single average artifact estimate across multiple sites would result in systematic bias, with oversubtraction at cleaner sites and undersubtraction at more polluted sites. Note that IMPROVE subtracts an averaged backup filter (QQB) across multiple sites.
RM Discussion
The RM was initially expected to provide an average artifact estimate with the consequences described above. However, the RM actually provided an artifact estimate that more closely resembles the artifact experienced by the higher OC concentration values for the PAQS dataset, where the RM performed quite well (). This likely occurs because higher concentration data have greater leverage in regression, especially for log-normally distributed data. For PAQS, the RM yielded artifact-corrected OC concentrations within the range of those obtained by the measurement-based methods for the mean of the highest 25% of PM2.5 mass concentration days, within 5% of “Q – QQB” and “Denuder.” However, for the lowest 25% of PM2.5 mass concentration days, RM-corrected OC concentrations were approximately 30% below Q – QQB and denuder.
A goal of this work was to develop criteria that could be used to identify under what conditions (i.e., for what datasets) the RM could be reliably applied. It was hypothesized that the better RM performance for PAQS than for RIOPA occurs because of the higher OC-mass correlations (r2). PAQS sampling occurred at a location dominated by regional aerosol, with a sizable secondary component. In contrast, RIOPA sampling occurred at locations strongly influenced by nearby sources (i.e., many homes were located within 200 m of local sources of organics and PM—roadways, gas stations, and refineries.) As a result, RIOPA samples have much larger variations in composition and source contributions than PAQS samples, and the proportion of the variation (r2) in front QFF OC that is explained by PM2.5 mass is higher for PAQS than RIOPA (). The number of samples in each RIOPA dataset is also small (N = 40–61). To test this hypothesis, simulated data were generated by Monte Carlo methods (1000 measurements generated to have a desired slope of 0.40 and y-intercept of 0.1 μgC/m3 and 4 different σ-square values for the random error term in the regression model). Calculated y-intercepts were compared with the desired value. This analysis of simulated data demonstrated that good RM performance can be obtained even at low r2(0.14) if the RM assumptions hold (linear relationship between OC and PM mass: artifact and OC mass fraction are independent of PM mass concentration), and that RM estimates can have substantial bias even for high r2 if these same assumptions are violated. PAQS and RIOPA datasets violate the assumptions of independence because the adsorption artifact is higher on higher concentration days. The poorer performance for RIOPA datasets might result from additional violations of the RM assumptions, the variable influence of nearby sources causing variations in the OC mass fraction, or because a few data points can have a large impact on the regression, especially for small N.
When RM artifact estimation was performed (Deming robust) on the six-site STN/IMPROVE comparison study dataset (; ), artifact estimates for three sites (Haines Point [urban], 1.40 ± 0.26 μg/m3, 32% of QFF OC; Dolly Sods and Tonto [rural], 1.4–1.6 μg/m3, 55–67% of QFF OC) were reasonably consistent with expectations based on the literature (net positive artifact of 0.5–4 μg/m3, 20–50% of front QFF OC in urban areas, 30–60% in remote)Citation4 and uncertainties in the intercept were modest (10–20%). These values were only a little higher than the average field blanks. (Field blanks averaged 1.3–1.4 μg/m3). In contrast, the RM estimate for Beacon Hill (r2 = 0.9) was negative (RM: −0.31 ± 0.11 μgC/m3; −11% of front QFF OC). RM estimates for Mount Rainier and Phoenix were small (0.02–0.21 μgC/m3; 2–4% of front QFF OC), with uncertainties greater than 100%. The average field blanks for Mount Rainier and Beacon Hill were also small (0.1–0.3 μgC/m3), whereas the Phoenix field blank (1.4 μgC/m3) was quite a bit larger than the RM estimate. Outlier removal substantially alters the RM artifact estimate for Phoenix (from 0.75 to 0.21 μgC/m3) and Tonto (from -0.08 to 1.43 μgC/m3).
Because sampling artifacts were not measured, the accuracy of the RM artifact estimates for the six-site STN/IMPROVE comparison study cannot be assessed, nor can it be determined whether or not the organic artifacts at these sites are dominated by the field blank. If the organic artifacts are dominated by the field blanks, artifact OC will be independent of PM mass, an assumption of the RM method that was violated for PAQS and RIOPA. The lower RM artifact estimates in Phoenix, Mount Rainier, and Beacon Hill could occur because of more volatilization or less adsorption (during sampling and/or transport and storage) at these sites or for these samplers. For example, temperatures can be quite high in Phoenix. Alternatively, the RM artifact estimate could be biased low because one or more assumptions of the RM were violated. For example, at Beacon Hill and Mount Rainier wood smoke is a substantial contributor to PM mass on many poor air quality days in the fall and winter.Citation42 It is logical then that the OC mass fraction might be particularly high on high-PM days and this would violate the assumption of linearity. RM artifact estimates for Mount Rainier and Phoenix are small and their uncertainties are large. Even if these artifact estimates were accurate, subtraction would not be recommended because the small correction would introduce larger uncertainties.
RM Guidance
When using OC values measured on a single QFF, one must decide whether to subtract the field blank, use the uncorrected values, or subtract the RM artifact estimate. This paper articulates the assumptions and limitations of the RM, leaving the reader better informed about the method. Examples of datasets for which the RM provided reasonable and unreasonable artifact estimates are provided. Unfortunately, criteria that could be used to determine objectively and with confidence when the use of the RM leads to better data quality were not able to be defined, nor could the uncertainties that would be introduced by application of the RM to the CSN or IMPROVE network be quantified with confidence. Although the authors are not recommending the RM, the following insights are provided for those who would like to explore it further:
1. | The RM approach is best applied to sites that represent OC concentrations at least at neighborhood and regional scales as defined by BlanchardCitation43 be cause samples collected at sites that are sometimes strongly influenced by a local source are particularly likely to violate the assumption of linearity. Even for these sites, the assumption of linearity will be violated if the sampling artifact is proportional to the OC concentration. If multiple locations are used to increase N, the concentration range and chemical characteristics of the aerosol (gas and particle phases) at the sites must be similar. | ||||
2. | A Deming regression should be used to account for uncertainties in x and y variables. | ||||
3. | Remove outliers in x and y using standard statistical tests (e.g., those used herein). | ||||
4. | Consider the possibility that the artifact might vary with changes in composition/source mix. If the dataset is quite large, analyses could be segregated by season or by OC mass fraction. Each subset would still need to contain a wide range of PM mass and OC concentrations. | ||||
5. | Evaluate the stability of the RM artifact estimate. How sensitive is the answer to the presence/absence of random subsets of data? | ||||
6. | Consider the possibility that the dataset might violate the inherent assumption of linear regression (that y values vary linearly with x values) because the slope (OC mass fraction) is not constant or the OC artifact is higher on high-concentration days. Nonlinearity might not be visually apparent when there is substantial scatter in the data. Additionally, if OC is a highly variable fraction of PM, the artifact could be also, and subtracting an average value would introduce considerable error in individual measurements. | ||||
7. | Be aware that accurate PM mass measurements are necessary (but not sufficient) for RM use. | ||||
8. | The testing of hypothesized criteria for RM use should include two-channel denuder, undenuded QFF, and accurate PM2.5 mass measurements. |
Another Option: Intermittent Backups, Sample-Specific Correction
Organic artifact estimates can be obtained by including backup filters at every site in a monitoring network. In large monitoring networks using 24-hr sampling periods, QQB filters could be preloaded in filter cassettes, thus requiring no additional field instrumentation or on-site filter exchange. If daily backup filter collection at all sites for all days is too costly given current budget constraints for large networks, backup filters can be collected on fewer days. In the latter case, it would be best to collect backup filters at all sites, although it is possible that sites could be grouped, noting that differences in temperature, source mix, concentration, and RH are likely to induce site-by-site differences in the size of the artifact. Note that it is preferable to include backup filters at all sites on fewer days rather than all days at fewer sites, and it is important that backup filters be collected across all seasons. The central limit theorem provides guidance regarding the number of samples needed for a daughter population (e.g., that were obtained from intermittent backup filter measurement) to resemble the distribution of the parent population. Datasets with lower correlations and larger measurement uncertainties require more samples.Citation44
For large datasets, when collecting backup filters at all sites, but on fewer days, artifact-corrected OC concentrations would be obtained by subtracting the measured artifact (QQB OC) from the front QQF OC on a sample-by-sample basis for samples in which the backup filter was included in the measurement. For samples with no backup filter, artifact-corrected OC concentrations would be predicted from front QFF OC as illustrated for PAQS in . (This approach also worked for RIOPA.) Specifically, the artifact-corrected OC was determined from the QFF OC and the regression of artifact-corrected OC (Q – QQB) on front QFF OC based on the more limited dataset. Note the regression of was unchanged when only every tenth sample was plotted. For example, a measured front QFF OC value of 5 μgC/m3 translates to an artifact-corrected OC concentration (Q – QQB) of 4.2 μgC/m3 for PAQS. This approach accounts for the fact that the adsorption artifact varies with OC concentration and can even account for the nonlinear nature of the artifact, which the RM method does not. Also, predictions are more robust because they are made using the entire regression relationship rather than being based on the intercept alone. Like the RM, taking into consideration uncertainties in x and y and excluding outliers will provide an optimal regression equation.
Figure 5. LLSR of artifact-corrected OC (Q – QQB or Q – TQB; y-axis) on front QFF OC (measured on Q; x-axis), demonstrating intermittent backup filter collection with every tenth sample for PAQS. The regression lines shown correspond well with their full dataset equivalents (PAQS Q – QQB: y = 0.89x – 0.14, r2 = 0.99; PAQS Q – TQB: y = 0.81x – 0.43, r2 = 0.97; N = 301). For days without backup filter measurements, particulate OC can be estimated from regression equations such as these.
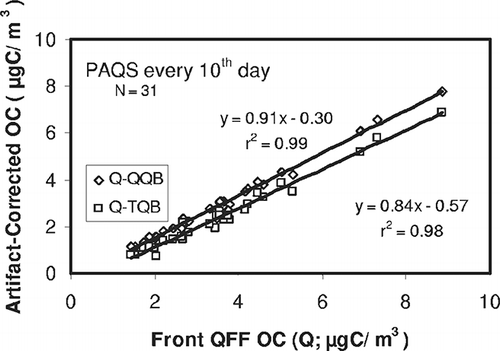
CONCLUSIONS
Future Network Operations
CSN and IMPROVE have several available options to quantify and/or reduce OC artifacts associated with sampling and with filter handling, transit, and storage. Subtraction of field blanks that have been loaded in the sampler and had air pulled through them briefly would correct for organic artifacts associated with filter handling, storage, and transport but would not correct for sampling artifacts. Field-blank values might be more similar to backup filter values if they are left passively exposed to ambient air for longer periods, as suggested elsewhere.Citation45 However, active sampling of particle-free ambient air, as is done with a backup filter, provides a “dynamic blank” that better mimics conditions encountered by samples. Alternatively, two-channel denuder sampling could be performed, as shown in , provided that suitable denuder and sorbent materials are available. This approach provides high-quality OC measurements (Equationeq 5; ) but only when operated with considerable care and verified through fastidious quality control; thus, this approach is impractical for a large routine network. Having said that, many more measurements of this type should be made across seasons and geographical regions for the purpose of evaluating the accuracy of simpler approaches. This is particularly important because negative artifacts are difficult to quantify.
Daily backup filter sampling is simple to perform and provides a sample-by-sample estimate of the adsorption artifact, enabling correction of front-filter OC by an estimate of the organic adsorption artifact experienced by that sample (Equationeqs 3 and Equation4; ). When batches of front and backup filters are transported and stored together, these backup filters also include artifacts encountered during transport, handling, and storage. Thus, when the backup filter is subtracted, it is not necessary to also subtract a field blank. Backup filter sampling on selected days provides a low-cost approach for CSN and other monitoring networks. Given that an increase in the ambient concentration of organics produces an increase in the adsorption artifact, no matter what artifact correction method is used, subtraction of a single average artifact estimate from all samples would constitute an oversubtraction (underestimation of OC) at the lower OC concentrations in the dataset and an undersubtraction (overestimation of OC) at the higher OC concentrations in the dataset. This could cause bias in reported annual average OC at particularly clean or polluted sites when the same network average value is subtracted from OC values at clean and polluted sites. The following protocol avoids this problem:
1. | Replace most QFF field blanks with backup QFF (QQB for 24-hr samples such as used in the CSN) so that approximately 10% of the samples collected at all sites include an adsorption estimate (dynamic bank). (Use TQB for samplers operating at low flow rates or for short sampling periods.) Compare OC artifacts across sites and seasons (plot backup-filter OC vs. front-filter OC) to determine to what extent data can be combined (to increase N). | ||||
2. | For all samples with concurrently collected backup filters, subtract the backup filter from its corresponding front QFF on a sample-by-sample basis (Equationeqs 3 or Equation4) to provide artifact-corrected OC values. | ||||
3. | Use the relationship between artifact-corrected OC and front QFF OC to provide artifact-corrected OC estimates (see example above) on a sample-by-sample basis for all samples in the dataset. | ||||
4. | Perform concurrent research-grade two-port denuder measurements () at selected sites concurrently with simpler methods for continued artifact investigation. Sites should be chosen to represent a range of composition and meteorological conditions within the network. |
ACKNOWLEDGMENTS
The authors thank the PAQS and RIOPA investigators and field teams who created the data used in this work. A special thanks is given to Dr. Adam Reff, Dr. Ann Marie Carlton, and Dr. Cliff Davidson. EPA, through its Office of Research and Development, collaborated in the research described here. It has been subjected to agency review and approved for publication.
REFERENCES
- Birch , M.E. and Cary , R.A. 1996 . Elemental Carbon-Based Method for Monitoring Occupational Exposures to Particulate Diesel Exhaust . Aerosol Sci. Technol. , 25 : 221 – 241 .
- Chow , J.C. , Watson , J.G. , Pritchett , L.C. , Pierson , W.R. , Frazier , C.A. , Purcell , R.G. and The , DRI. 1993 . Thermal/Optical Reflectance Carbon Analysis System: Description, Evaluation and Application in US Air Quality Studies . Atmos. Environ. , 27 : 1185 – 1201 .
- Kirchstetter , T.W. , Corrigan , C.E. and Novakov , T. 2001 . Laboratory and Field Investigation of the Adsorption of Gaseous Organic Compounds onto Quartz Filters . Atmos. Environ. , 35 : 1663 – 1671 .
- Turpin , B.J. , Saxena , P. and Andrews , E. 2000 . Measuring and Simulating Particulate Organics in the Atmosphere: Problems and Prospects . Atmos. Environ. , 34 : 2983 – 3013 .
- Subramanian , R. , Khlystov , A.Y. , Cabada , J.C. and Robinson , A.L. 2004 . Positive and Negative Artifacts in Particulate Organic Carbon Measurements with Denuded and Undenuded Sampler Configurations . Aerosol Sci. Technol. , 38 : 27 – 48 .
- Mader , B.T. , Schauer , J.J. , Seinfeld , J.H. , Flagan , R.C. , Yu , J.Z. , Yang , H. , Lim , H.-J. , Turpin , B.J. , Deminter , J.T. , Heidemann , G. , Bae , M.S. , Quinn , P. , Bates , T. , Eatough , D.J. , Huebert , B.J. , Bertram , T. and Howell , S. 2003 . Sampling Methods Used for the Collection of Particle-Phase Organic and Elemental Carbon during ACE-Asia . Atmos. Environ. , 37 : 1435 – 1449 .
- McDow , S.R. and Huntzicker , J.J. 1990 . Vapor Adsorption Artifact in the Sampling of Organic Aerosol: Face Velocity Effects . Atmos. Environ. , 24 : 2563 – 2571 .
- 2000 . DRI Standard Operating Procedure: Thermal/Optical Reflectance Carbon Analysis of Aerosol Filter Samples , Reno , NV : Desert Research Institute .
- 2003 . Standard Operating Procedure for the Determination of Organic, Elemental, and Total Carbon in Particulate Matter Using a Thermal/Optical-Transmittance Carbon Analyzer , Research Triangle Park , NC : Research Triangle Institute .
- Flanagan, J., Research Triangle Institute, Research Triangle Park, NC. Personal communication, April 11, 2011. Data available at http://www.epa.gov/ttn/amtic/ (http://www.epa.gov/ttn/amtic/)
- Solomon, P.A.; Mitchell, W.; Gemmill, D.; Tolocka, M.; Norris, G.; Wiener, R.; Eberly, J.; Rice, J.; Homolya, J.; Scheffe, R.; Vanderpool, R.; Murdoch, R.; Natarajan, S.; Hardison, E. Evaluation of PM2.5 Chemical Speciation Samplers for Use in the U.S. EPA National PM2.5 Chemical Speciation Network; EPA-454/R-01-005/NTIS PB#2001-105814; 2000 http://www.epa.gov/ttn/amtic/files/ambient/pm25/spec/fourcty.pdf (http://www.epa.gov/ttn/amtic/files/ambient/pm25/spec/fourcty.pdf) (Accessed: 2011 ).
- Turpin , B.J. , Hering , S.V. and Huntzicker , J. J. 1994 . Investigation of Organic Aerosol Sampling Artifacts in the Los Angeles Basin . Atmos. Environ. , 28 : 3061 – 3071 .
- Kim , B.M. , Cassmassi , J. , Hogo , H. and Zeldin , M.D. 2001 . Positive Organic Carbon Artifacts on Filter Medium during PM2.5 Sampling in the South Coast Air Basin . Aerosol Sci. Technol. , 34 : 35 – 41 .
- Tolocka , M.P. , Solomon , P.A. , Mitchell , W. , Norris , G.A. , Gemmill , D.B. , Wiener , R.W. , Vanderpool , R.W. , Homolya , J.B. and Rice , J. 2001 . East Versus West in the US: Chemical Characteristics of PM2.5 during the Winter of 1999 . Aerosol Sci. Technol. , 34 : 88 – 96 .
- Frank , N.H. 2006 . Retained Nitrate, Hydrated Sulfates, and Carbonaceous Mass in FMR Fine PM for 6 Eastern US Cities . Journal of the Air & Waste Management Association , 56 : 500 – 511 .
- Eatough , D.J. , Wadsworth , A. , Eatough , D.A. , Crawford , J.W. , Hansen , L.D. and Lewis , E.A. 1993 . A Multiple-System, Multichannel Diffusion Denuder Sampler for the Determination of Fine Particulate Organic Material in the Atmosphere . Atmos. Environ. , 27 : 1213 – 1219 .
- Flanagan , J.B. , Peterson , M.R. , Jayanty , R.K.M. and Rickman , E.E. 2002 . Analysis of PM2.5 Speciation Network Carbon Blank Data . Presented at the Symposium on Air Quality Measurement Methods and Technology . November 2002 , San Francisco , CA .
- Cheng , Y. , He , K.B. , Duan , F.K. , Zheng , M. , Ma , Y.L. and Tan , J.H. 2009 . Measurement of Semivolatile Carbonaceous Aerosols and Its Implications: a Review . Environ. Int. , 35 : 674 – 681 .
- Mader , B.T. and Pankow , J.F. 2001 . Gas/Solid Partitioning of Semivolatile Organic Compounds (SOCs) to Air Filters. 3. An Analysis of Gas Adsorption Artifacts in Measurements of Atmospheric SOCs and Organic Carbon (OC) When Using Teflon Membrane Filters and Quartz Fiber Filters . Environ. Sci. Technol. , 35 : 3422 – 3432 .
- Hart , K.M. and Pankow , J.F. 1994 . High-Volume Air Sampler for Particle and Gas Sampling. 2. Use of Backup Filters to Correct for the Adsorption of Gas-Phase Polycyclic Aromatic Hydrocarbons to the Front Filter . Environ. Sci. Technol. , 28 : 655 – 661 .
- Hering , S.V.L. , Lawson , D.R. , Allegrini , I. , Febo , A. , Perrino , C. , Possanzini , M. , Sickles , J.E. II. , Anlauf , K.G. , Wiebe , A. , Appel , B.R. , John , W. , Ondov , J. , Wall , S. , Braman , R.S. , Sutton , R. , Cass , G.R. , Solomon , P.A. , Eatough , D.J. , Eatough , N.L. , Ellis , E.C. , Grosjean , D. , Hicks , B.B. , Womach , J.D. , Horrocks , J. and Knapp , K.T. 1988 . The Nitric Acid Shootout: Field Comparison of Measurement Methods . Atmos. Environ. , 22 : 1519 – 1539 .
- Fraser , M.P. , Grosjean , D. , Grosjean , E. , Rasmussen , R. and Cass , G. 1996 . Air Quality Model Evaluation Data for Organics. 1. Bulk Chemical Composition and Gas/Particle Distribution Factors . Environ. Sci. Technol. , 30 : 1731 – 1743 .
- Tang , H. , Lewis , E.A. , Eatough , D.J. , Burton , R.M. and Farber , R.J. 1994 . Determination of the Particle Size Distribution and Chemical Composition of Semi-Volatile Organic Compounds in Atmospheric Fine Particles with a Diffusion Denuder Sampling System . Atmos. Environ. , 28 : 939 – 947 .
- Lewtas , J. , Booth , D. , Pang , Y. , Reimer , S. , Eatough , D.J. and Gundel , L.A. 2001 . Comparison of Sampling Methods for Semi-Volatile Organic Carbon (SVOC) Associated with PM2.5 . Aerosol Sci. Technol. , 34 : 9 – 22 .
- Wittig , A.E. , Anderson , N. , Khlystov , A.Y. , Pandis , S.N. , Davidson , C. and Robinson , A.L. 2004 . Pittsburgh Air Quality Study Overview . Atmos. Environ. , 38 : 3107 – 3125 .
- Polidori , A. , Turpin , B.J. , Lim , H.J. , Cabada , J.C. , Subramanian , R. , Robinson , A.L. and Pandis , S.N. 2006 . Local and Regional Secondary Organic Aerosol: Insights from a Year of Semi-Continuous Measurements at Pittsburgh . Aerosol Sci. Technol. , 40 : 861 – 872 .
- Cabada , J.C. , Pandis , S.N. , Subramanian , R. , Robinson , A.L. , Polidori , A. and Turpin , B. 2004 . Estimating the Secondary Organic Aerosol Contribution to PM2.5 Using the EC Tracer Method . Aerosol Sci. Technol. , 38 : 140 – 155 .
- Cabada , J.C. , Rees , S. , Takahama , S. , Khlystov , A.Y. , Pandis , S.N. , Davidson , C.I. and Robinson , A.L. 2004 . Mass Size Distributions and Size Resolved Chemical Composition of Fine Particulate Matter at the Pittsburgh Supersite . Atmos. Environ. , 38 : 3127 – 3141 .
- Meng , Q.Y. , Turpin , B.J. , Korn , L. , Weisel , C.P. , Morandi , M. , Colome , S. , Zhang , J. , Stock , T. , Spektor , D. , Winer , A. , Zhang , L. , Lee , J.H. , Cui , W. , Giovanetti , R. , Kwon , J.M. , Alimokhtari , S. , Shendell , D. , Jones , J. and Maberti , S. 2005 . Influence of Outdoor Sources of Indoor and Personal Fine Particle Concentrations: Analyses of RIOPA Data . J. Expo. Anal. Environ. Epidemiol. , 15 : 17 – 28 .
- Weisel , C.P. , Zhang , J. , Turpin , B.J. , Morandi , M.T. , Colome , S. , Stock , T.H. , Spektor , D.M. , Korn , L. , Winer , A. , Alimokhtari , S. , Kwon , J. , Mohan , K. , Harrington , R. , Giovanetti , R. , Cui , W. , Afshar , M. , Maberti , S. and Shendell , D. 2005 . The Relationships of Indoor, Outdoor and Personal Air (RIOPA) Study: Study Design, Methods, and Quality Assurance/Control Results . J. Expo. Anal. Environ. Epidemiol. , 15 : 123 – 137 .
- Meng, Q. Ph.D. Dissertation, Rutgers University, New Brunswick, NJ, 2004.
- Polidori , A. , Turpin , B. , Meng , Q.Y. , Lee , J.H. , Weisel , C. , Morandi , M. , Colome , S. , Stock , T. , Winer , A. , Zhang , J. , Kwon , J. , Alimokhtari , S. , Shendell , D. , Jones , J. , Farrar , C. and Maberti , S. 2006 . Fine Organic Particulate Matter Dominates Indoor-Generated PM2.5 in RIOPA Homes . J. Expo. Sci. Environ. Epidemiol. , 16 : 321 – 331 .
- Solomon , P.A. , Egeghy , P. , Crumpler , D. , Rice , J. , Homolya , J. , Frank , N. , Klamser-Williams , Pitchford , M. , Ashbaugh , L. , McDade , C. , Orourke , J. , Flanagan , J. and Rickman , E. Multi-Site Comparison of Mass and Major Chemical Components Obtained by Collocated STN and IMPROVE Chemical Speciation Network Monitors . In Proceedings of the American Association for Aerosol Research (AAAR) Annual Meeting . Mount Laurel , NJ . AAAR . Abstract 8E2
- Particulate Matter (PM2.5) Speciation Guidance, Final Rule; U.S. Environmental Protection Agency; 1999 http://www.epa.gov/ttn/amtic/files/ambient/pm25/spec/specfinl.pdf (http://www.epa.gov/ttn/amtic/files/ambient/pm25/spec/specfinl.pdf) (Accessed: 2011 ).
- Peterson , M.R. , Flanagan , J.B. and Jayanty , R.K.M. 2003 . Standard Operating Procedure for the Determination of Organic, Elemental, and Total Carbon in Particulate Matter Using a Thermal/Optical Transmittance Carbon Analyzer , Research Triangle Park , NC : Environmental and Industrial Sciences Division; Research Triangle Institute .
- Kim , E. , Hopke , P.K. and Qin , Y. 2005 . Estimation of Organic Carbon Blank Values and Error Structures of the Speciation Trends Network Data for Source Apportionment . Journal of the Air & Waste Management Association , 55 : 1190 – 1199 .
- Deming , W.E. 1943 . Statistical Adjustment of Data , New York : John Wiley and Sons .
- Cornbleet , P.J. and Gochman , N. 1979 . Incorrect Least-Squares Regression Coefficients in Method-Comparison Analysis . Clin. Chem. , 25 : 432 – 438 .
- Turpin , B.J. and Huntzicker , J. J. 1995 . Identification of Secondary Organic Aerosol Episodes and Quantitation of Primary and Secondary Organic Aerosol Concentrations during SCAQS . Atmos. Environ. , 29 : 3527 – 3544 .
- Chu , S.-H. 2005 . Stable Estimate of Primary OC/EC Ratios in the EC Tracer Method . Atmos. Environ. , 39 : 1383 – 1392 .
- Flanagan , J.B. , Jayanty , R.K.M. , Rickman , E.E. and Peterson , M.R. 2006 . PM2.5 Speciation Trends Network: Evaluation of Whole-System Uncertainties Using Data from Sites with Collocated Samplers . Journal of the Air & Waste Management Association , 56 : 492 – 499 .
- Wu , C.-F. , Larson , T.V. , Wu , S.-Z. , Williamson , J. , Westberg , H.H. and Liu , L.-J. 2007 . Source Apportionment of PM2.5 and Selected Hazardous Air Pollutants in Seattle . Sci. Total Environ. , 386 : 42 – 52 .
- Blanchard , C. 2004 . Particulate Matter Science for Policy Makers, a NARSTO Assessment , Cambridge , , United Kingdom : Cambridge University . Ch. 6
- Triola , M.F. 2001 . Elementary Statistics , 8th , New York : Addison-Wesley Longman .
- Chow , J.C. , Watson , J.G. , Chen , L.-W.A. , Rice , J. and Frank , N.H. 2010 . Quantification of PM2.5 Organic Carbon Sampling Artifacts in US Networks . Atmos. Chem. Phys. , 10 : 5223 – 5239 .