Abstract
The perpetual activity of the cerebral cortex is largely supported by the variety of oscillations the brain generates, spanning a number of frequencies and anatomical locations, as well as behavioral correlates. First, we review findings from animal studies showing that most forms of brain rhythms are inhibition-based, producing rhythmic volleys of inhibitory inputs to principal cell populations, thereby providing alternating temporal windows of relatively reduced and enhanced excitability in neuronal networks. These inhibition-based mechanisms offer natural temporal frames to group or “chunk” neuronal activity into cell assemblies and sequences of assemblies, with more complex multi-oscillation interactions creating syntactical rules for the effective exchange of information among cortical networks. We then review recent studies in human psychiatric patients demonstrating a variety alterations in neural oscillations across all major psychiatric diseases, and suggest possible future research directions and treatment approaches based on the fundamental properties of brain rhythms.
La perpetua actividad de la corteza cerebral está sustentada en gran medida por la variedad de oscilaciones que genera el cerebro, las que abarcan un número de frecuencias y sitios anatómicos, así como correlatos conductuales. En primer lugar se revisan los hallazgos de estudios animales que muestran que la mayoría de las formas de los ritmos cerebrales se basan en la inhibición, produciendo descargas rítmicas de estímulos inhibitorios a las principales poblaciones celulares, proporcionando por lo tanto ventanas temporales que alternan una excitabilidad relativamente reducida o aumentada en los circuitos neuronales. Estos mecanismos basados en la inhibición ofrecen marcos temporales naturales para agrupar o “fragmentar” la actividad neuronal en conjuntos celulares y secuencias de conjuntos, con interacciones más complejas de multi-oscilación creando reglas sintácticas para el cambio efectivo de información entre los circuitos corticales. Luego se revisan los estudios en pacientes psiquiátricos que demuestran una variedad de alteraciones en las oscilaciones neurales en las principales enfermedades psiquiátricas, y sugieren posibles orientaciones en las investigaciones a futuro y aproximaciones terapéuticas basadas en las propiedades fundamentals de los ritmos cerebrales
L'activité permanente du cortex cérébral est largement basée sur la grande variété d'oscillations que le cerveau génère incluant un grand nombre de fréquences et de localisations anatomiques, ainsi que leurs corrélats comportementaux. Nous présentons tout d'abord les recherches sur les études animales montrant que la plupart des formes des rythmes cérébraux sont basées sur l'inhibition, produisant des volées rythmiques de signaux inhibiteurs vers les populations cellulaires principales, fournissant alors des fenêtres temporales alternatives d'excitabilité relativement réduites et plus importantes dans les réseaux neuronaux. Ces mécanismes inhibiteurs offrent des cadres temporaux naturels à une grosse activité neuronale ou activité groupée dans des ensembles de cellules et des séquences d'ensembles de cellules, avec des interactions multi-oscillatoires plus complexes créant des règles syntaxiques pour l'échange efficace d'information parmi les réseaux corticaux. Nous analysons ensuite des études récentes de patients psychiatriques qui montrent des altérations variées des oscillations neurales dans toutes les principales maladies psychiatriques. De possibles directions de recherche future ainsi que des approches de traitement fondées sur les propriétés fondamentales des rythmes cérébraux sont proposées.
Overview
The initial portion of this manuscript is devoted to a basic introduction to a number of neurophysiological concepts as background to an understanding of neuronal oscillations (used here nearly interchangeably with “rhythms”) in the brain. This initial portion may require some in-depth knowledge in neurophysiology, though we hope to introduce concepts in intuitive ways and by adding a glossary (see Glossary in separate Box) for convenience. We will go on to explain how these oscillatory rhythms interact with each other and allow for information coding, demonstrating that they are central to brain function. Therefore, a knowledge of the role of neural rhythms is critical to a full understanding of brain function and the impact disturbances of brain rhythms can have on patients. With this background in hand, we then move to a larger perspective aimed specifically at findings and future directions in psychiatric disease.
Introduction: a system of brain rhythms
Computation in the cerebral cortex of all mammals has two essential features: local-global communication and persistent activity.Citation1-Citation3 Due to the bidirectional and highly branched connectivity of neurons throughout the mammalian brain, the results of local computations are broadcast to widespread areas so that multiple structures are informed simultaneously around any given local activity. The inverse is also true: local circuits are under the continuous control of global brain activity, usually referred to by terms such as “brain state,” “top-down” or “attentional” control.Citation4,Citation5 The second fundamental feature of the cerebral cortex is its persistent activity, ie, an ability to ignite and maintain a long-lasting trace after the initial input has already vanished.Citation6 For example, a transient perturbation, such as hearing someone's name in a particular context, can trigger internally generated brain activity for several minutes. In the case of sleep, which is an extreme example of persistent activity, since activity is maintained free of external inputs, a transient perturbation can have a lasting impact. Both local-global communication and persistent activity require special structural and dynamic organization.
Local-global interactions and persistent activity can be maintained by the interactive systems of brain oscillations.Citation7 The cerebral cortex is perpetually active as reflected by the ever-changing landscape of the electroencephalogram (EEG). Traditional quantitative investigation of the EEG is performed by calculating the spectral power distribution of long-duration recordings, ie, the relative amplitudes, or “energies” of the various frequencies comprising the EEG or other extracellularly recorded signal. ( ). A striking aspect of the extracellular signal is its self-similarity (“fractal” nature) in both space and time, wherein the fundamental features of the extracellular signal recorded by microelectrodes or large scalp electrodes over different cortical structures are the same, even though the recorded volume of neurons differs in orders of magnitude. Thus, certain fundamental aspects of function are maintained across location and scale.Citation8,Citation9 Such a distribution is telltale of complex (or “pink”) noise,Citation10 which led many investigators to suggest that brain dynamics are essentially chaotic, driven by noise fluctuations.Citation11 However, this conclusion is valid only when brain activity is surveyed over very long periods of time. However, many of the most interesting brain-related phenomena from perception to action occur in relatively short time windows such as subseconds rather than minutes or hours; therefore, these short windows are the most relevant for the investigation of brain dynamics involved in cognitive activity. Examined from such a temporal perspective, the brain patterns that characterize these cognitive moments may have some nonoscillatory or irregular components, but are typically largely oscillatory in nature and return reliably to the same states after the information is processed. Even in such short time windows several rhythms and nonrhythmic patterns can coexist. Most often the frequencies of the various rhythms have a noninteger relationship with each other and the resulting interference patterns lead to the appearance of “noise.” Neuronal networks in the mammalian forebrain support several oscillatory bands (families of oscillations) that span from approximately 0.05 Hz to 500 Hz (Figure 1). Importantly, there are a number of boundary lines drawn to delineate cortical oscillations which have been empirically found to act relatively independently. The frequencies occupied by these bands have relatively constant relationships to each other on a natural logarithmic scale (Figure 1) and tend to have constant ratios between any given pair of neighboring frequencies (Figure 1). Constrained largely by the slow axon conduction velocity of the neurons, when the available time is short, as is the case of higher frequency oscillations, the participating neurons are confined to a small volume of nervous tissue. In contrast, during slow oscillations many neurons in a large volume of tissue can be recruited to the rhythm. Mainly due to this structural constraint, when multiple rhythms are present simultaneously, the phase of the slow rhythm(s) modulates the power of the faster one(s). This “cross-frequency phase coupling,” first demonstrated between theta (0,4 to 9 Hz) and gamma (γ, 30 to 90 Hz) oscillations,Citation12,Citation13 is a general mechanism for all known rhythms ( .)Citation14-Citation16 and it undergirds a hierarchical organization of brain rhythms.Citation17
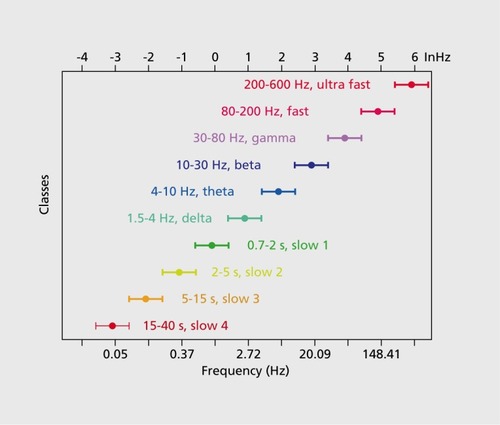
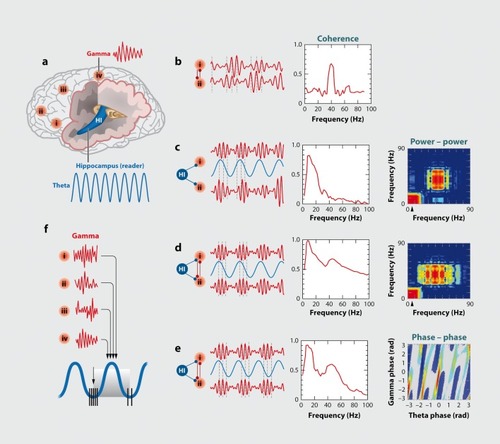
Preservation of brain rhythms in the mammalian order
The spectral features of the EEG or local field potentials (LFP) recorded from animals with small or large brains are similar, and all known oscillations in humans are present in all other mammals investigated to date, γ oscillations have the same frequency range (30 to 90 Hz) and, importantly, have the same intermittent nature and likely the same mechanisms in animals with small and large brains.Citation13 Slow oscillations (0.5 to 2 Hz)Citation18 have been observed in the neocortex of all mammals tested. Similarly, sleep spindles have not only the same frequency (12 to 18 Hz) but the duration of the spindles is also similar.Citation19-Citation21 The ultra-slow (0.1 Hz) rhythm () involved large areas of the neocortex and is easily detectable with functional magnetic resonance imaging (fMRI) as correlated and anticorrelated brain regions in this frequency range gives rise to the “default” patterns of cortical activity (ie, those brain activity patterns observed in the absence of specific inputs or tasks) now frequently seen in human subjects.Citation22 The ultra-slow fluctuation of cortical network excitability is robust and has been observed also in monkeys,Citation23 cats,Citation24 and ratsCitation21 (Figure 3). Thalamocortical alpha (α) oscillations (8 to 12 Hz) are the characteristic dynamic of sensory and motor systems in their “idling” or non-directed state. In humans, the specific members of the α family rhythms are known as a oscillations of the visual system, mu (μ) rhythms of the sensorimotor system, and tau (τ) rhythms of the auditor)' system.Citation17,Citation25 Similar α mechanisms have been detected in the gustatory cortex, even in the absence of taste inputs.Citation26 In primates with a large cortical domain dedicated to vision, eye closure induces widespread α oscillation in the occipital-posterior part of the neocortex.Citation27 In rodents, where face-whisker representation in the somatosensory cortex is particularly large, the μ rhythm occurs synchronously over the parietal-frontal areas during immobility.Citation28 Sniffing induces θ phase-modulated μ patterns in the olfactory bulb and cortex with similar frequencies and temporal dynamic in multiple species.Citation29
Hippocampal θ oscillations are perhaps the only known rhythm whose frequency scales inversely with the size of the brain. The 6- to 12-Hz θ oscillations in rodentsCitation30,Citation31 slow down to 4 to 6 Hz In carnivores.Citation32,Citation33 θ frequency of all species investigated is slowest in humans (1 to 4 Hz),Citation34-Citation36 and its very existence has been questioned by some reports in epileptic patients.Citation37,Citation38
The preservation of frequencies in the various mammalian species is an important argument in favor of time as the most important organization principle in brain dynamics. While brain rhythms vary little across species (30% to 100%), brain size increases hundreds- to thousands-fold from the smallest- to the largest-brained mammal.Citation39 This scaling process places serious and critical constraints on brain development. While the modular neocortex can volumetrically expand multiple-fold, time-related issues appear to have fundamentally shaped brain phylogenesis. To preserve timing in the face of multiplying cortical modules, disproportionally more long-range axonal pathways and more effective axon myelination are deployed.Citation40 By contrast, a potential argument for the decreasing frequency of hippocampal θ oscillations In mammals with larger brains is that the hippocampus is a single cortical module,Citation41,Citation42 and its growth is limited by the axon conduction delays. Pyramidal neurons of the CA3 region of the hippocampus Innervate a very large volume of the hippocampus,Citation41,Citation43,Citation44 connecting distant peer neurons and requiring long axonal lengths and, consequently, longer delays. The increasing delays may contribute to the slowing of the θ rhythm as the structure grows.
It is critical to emphasize that preservation of cortical rhythms across species does not reflect the brain's inability to change timing mechanisms, and rhythms can adapt effectively according to the needs of given species. For example, central pattern generators for walking and respiratory rhythms vary according to ecological needs from 0.5/min in large aquatic mammals to 100/min in mice. Instead, the constancy of brain oscillations across species seems to reflect the importance of timing as well as an inheritance of the same coding mechanisms. The preservation of temporal constants that govern brain operations across several orders of magnitude of time scales suggests that the architectural aspects of the brain, such as scaling the ratios of neuron types, modular growth, system size, intersystem connectivity, synaptic path lengths, and axon caliber are subordinated to a temporal organizational priority.
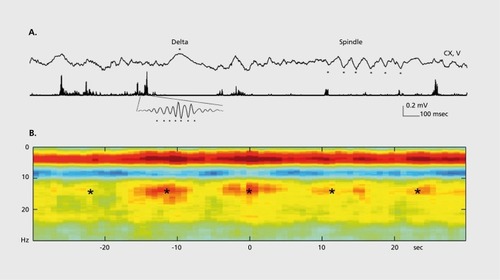
Brain oscillations provide framework for a neural syntax
Most, if not all, network oscillations are based on inhibition wherein populations of principal neurons are paced by repetitive trains of inhibitory postsynaptic potentials. These rhythmic inhibitory volleys provide windows of alternating reduced and enhanced excitability of principal cells in a temporally coordinated manner.Citation17,Citation45 Indeed, segregation of excitatory principal cells into functional groups, often referred to as cell assemblies and assembly sequences,Citation46 is perhaps the most important service performed by the large family of inhibitory neuron classes in the cortex.Citation47,Citation48 Inhibition-based oscillations may do so by providing a natural means to “stop” signals of neuronal information flow by temporally silencing principal cells and “chunking” streams of messages into shorter frames, as evidenced by the observation that oscillations have well-defined onsets and offsets with characteristic maximum and minimum spiking activity in the information-transmitting principal cells.Citation49 This stop-start parsing function of neuronal oscillators and their hierarchical cross-frequency coupling organization (see section below as well as Figure 2), in turn, can support syntactical rules, known to both sender and receiver, making communication more straightforward than interpreting long uninterrupted messagesCitation50 or stochastic patterns of spikes.
In general, syntax is a set of principles that allows the generation of rich combinations from a limited number of elements using a minimal number of rules. It has been hypothesized that the fundamental element of neuronal syntax is an assembly of neurons discharging together in a γ cycle.Citation51-Citation53 The most important role of the cell assembly is to bring together sufficient numbers of peer neurons so that their collective spiking can bring above discharge threshold the proper population of downstream postsynaptic neurons.Citation52 Consequently, from the point of view of the downstream (“reader” or “integrator”) target cells, collective activity of upstream neurons is classified as a single eventCitation53 only if their spikes occur within the time-integrating window (ie, within the membrane time constant of the neurons, tends to be 10 to 30 msec; if signals occur within this time scale, they will be combined as a unitary event).Citation54 Spikes of upstream neurons which fire outside the integration time window must be part of another event, or a separate assembly. The temporal window or the membrane time constant also corresponds to the duration of the γ cycle (a 40-1 Iz oscillation yields cycle lengths of 25 ms) and is also closely related to other biophysical properties of neurons, including the time constant of γ-aminobutyric acid (GABA)A and α-amino-3-hydroxy-5-methyl- 4-isoxazolepropionic acid (AMPA) receptorsCitation55 and the critical time window of spike-timing-dependent plasticity.Citation56-Citation57
Multiple γ cycles, each containing their own cell assembly, can be thought of as being “neural letters” and these letters can then be combined to create “words” and later “sentences.” More precisely: discrete episodes or packets of γ oscillations, which are typically shortlasting,Citation5,Citation15,Citation45,Citation58,Citation59 are often grouped by slower oscillations via cross-frequency phase coupling (Figure 2). Citation12,Citation14,Citation15,Citation60-Citation62 This packeting can be thought to associate the “letters” contained in the series γ cycles to form a neural “word.” An example would be a γ “burst” which might be cross-frequency coupled to 0 and therefore present in a single θ cycle.Citation63-Citation66 Then slower rhythms In which θ waves nest can bind such words into “neural sentences,” ie, longer messages of information, coordinated across large brain territories.
In summary, the hierarchical nature of cross-frequency interactions may reflect a mechanism of syntactical organization. Importantly, the LFP γ oscillatory episodes can be exploited as a proxy for assembly organization and for monitoring physiological and disease-related alterations of neuronal communication.
Brain oscillations support inter-regional communication
As discussed above, efficient communication requires that messages are transmitted by syntactical rules known to both sender and receiver. In human-made systems, transfer of messages from source (sender) to target (reader) is usually considered a unidirectional operation in which an ever-ready recipient mechanism stands by for receiving messages. However, brain networks have evolved their own self-organized (“spontaneous”) patterns, which can effectively gate or bias whether the information conveyed by the sensors or sender network is amplified or ignored.Citation53,Citation67
In order to better illustrate these phenomena, we will start with sensory systems which are not “ever-ready” reading mechanisms but rather have coevolved with specialized motor systems that are dedicated to allowing those sensory systems to most efficiently operate. These dedicated motor outputs, such as licking, sniffing, whisking, touching, saccadic eye movements, twitching of the inner ear muscles, or other gating mechanisms assist their specific sensory systems by optimizing the orientation of the sensors and, therefore, maximizing their ability to sample the environment. In addition to optimizing the sensors, top-down mechanisms provide further amplification and filtering in short time windows. Such active mechanisms can create transient gain adjustments, which enhance the ability of the sensory system to process inputs selectively.Citation68-Citation72 Such “active gain” is perhaps best illustrated in the case of olfaction, where the detection of an odorant in a particular phase of the sniff cycle is several-fold more effective than presentation in the same odorant at other phases.Citation71 Such transient gain adjustments are likely to play a critical role in vision, where saccadic eye movements allow for an intermittent and active sampling of the visual input. This mechanism may explain why stationary pictures evoke barely detectable spike rate changes in higher visual areas,Citation73 whereas movie clips that are scanned by frequent saccades robustly activate those same cells.Citation74 In summary, the sequence of sensation appears to be as follows: generation of an internal plan, corresponding motor-based adjustment of the sensor to allow selective amplification of the preplanned inputs and suppression of other streams of information, then finally detection. When unexpected stimuli impinge on such a system it of course means the initial processing of information was suboptimal, and the system adapts by replanning for optimal adjustments of the sensors and detection of key details. Oftentimes, this active sampling mechanism is referred to as attention or selective attention,Citation75 although the biological origin and mechanisms of the effector mechanisms are rarely discussed.Citation67
We hypothesize that, analogous to the sensory systems, higher-order areas of the brain adopted similar readerinitiated mechanisms for efficient processing of afferent information. For example, transfer of neural information from the hippocampus (the “sender”) to the neocortex (the “reader”) during slow-wave sleep can be initiated by the transition of neocortical neurons to a depolarized/active state during the neocortical slow oscillation which occurs during slow-wave sleep.Citation21,Citation76-Citation78 These fluctuations of neocortical (receiver) excitability can bias the spike content of hippocampal (sender) sharp wave-ripple oscillations,Citation79,Citation80 thereby allowing the hippocampus to then signal back and reciprocally affect those neocortical populations that are still actively spiking in the persisting depolarized state of the slow oscillation. In the waking brain, the directionality is opposite: now the dialogue is initiated by the reader hippocampus via θ-phase control of (sender) neocortical network dynamics in the form of local γ oscillations.Citation15 This scheme has the additional advantage of allowing the receiver to specify that the self-organized γ oscillations at the many sender modules across the cortex, can arrive at the hippocampus at the phase of the θ cycle when the reader hippocampal networks are in their most sensitive, plastic state.Citation81 Exchange of information between different stages of the visual system appears to follow similar rules,Citation58,Citation82 suggesting that the reader-initiated transfer of neural messages from a sender is a general rule in the brain. This, again, is in contrast to the notion of a passively waiting receiver system, showing that the brain has evolved unique communication schemes, utilizing oscillations and their mutual interactions as a tool to aide an efficient communication scheme.
Hierarchy of brain oscillators is formed by cross-frequency coupling
Brain rhythms can interact with each other by multiple mechanisms, including phase-phase, phase-amplitude and amplitude-amplitude envelope coupling (Figure 2). In the simplest scenario oscillators of similar frequency within the same or different anatomical structures can entrain each other by a mechanism known as phase coupling. Phase coupling can be measured by coherence or preferably by more advanced methods, which are independent of the amplitude fluctuations and based exclusively on phase. A well-known example of phase-phase coupling is the coherent θ oscillations throughout the hippocampus-entorhinal cortex system. Multiple regions can generate θ oscillations and all layers form θ dipoles that fuse into a global “monolithic” single θ oscillator. This occurs despite the fact that the θ rhythm generators of isolated regions oscillate at different frequencies.Citation31 Typically, when oscillators of similar frequencies are coupled, the overall frequency is determined by the fastest one.Citation83 The computational advantages of phase synchronization have been illustrated by numerous experiments in various species, and excellent reviews summarize those findings.Citation4,Citation83-Citation85
A temporally less precise, but nevertheless important, interaction between oscillators of similar frequency is expressed by the temporal covariation of their power, known as amplitude comodulation or power-power coupling. In this case, phase constancy between the waves may not be present but, instead, the power (amplitude) envelopes of the oscillators are correlated (comodulation of power). This power-power synchrony of two or multiple oscillators in various networks can be coordinated by the joint phase biasing of the power of the faster oscillations by the slower rhythm, known as crossfrequency phase-amplitude (CFPA) coupling or nested oscillations. One reason why slow oscillations can impact faster ones in multiple brain areas has to do with the conduction velocities of cortical neurons. Compared with faster oscillators, slower oscillators involve more neurons in a larger volumeCitation86 and are associated with larger membrane potential changes because in longer time windows spikes of many more upstream neurons can be integrated.Citation61,Citation87 Cross-frequency phase-amplitude coupling was first described between hippocampal θ and γ rhythms,Citation12,Citation88,Citation89 and extended subsequently to across-structure coupling. Citation14,Citation60,Citation90-Citation97 Gamma power can also be phasemodulated by α,Citation97,Citation98 spindle,Citation99 delta,Citation100 switching between UP and DOWN states of slow oscillationsCitation61,Citation77,Citation101 and ultraslowCitation23 oscillations.Citation13,Citation16,Citation17,Citation84,Citation102,Citation103 The principle of cross-frequency phase-amplitude coupling generalizes to all known frequency bands in the mammalian cortex and has been reported between all co-occurring oscillators in interactive circuits at frequencies from as low as 0.025 Hz to as high as 500 Hz.Citation21,Citation104,Citation105 For example, the occurrence of hippocampal “ripples” (f 40 to 200 Hz) is coupled to dendritic layer sharp waves and phase-modulated by sleep spindles (12 to 16 Hz). In turn, the spindle-modulated sharp wave-ripple complex is phasecoupled to neocortical slow oscillations (0.5 to 1.5 Hz)Citation77-Citation101; and all these rhythms are modulated by the ultraslow (0.1 Hz) oscillationCitation21 (Figure 3).
Several studies have demonstrated the physiological utility of cross-frequency coupling. For example, the strength of θ-γ coupling in the hippocampus and striatum of the rat was affected by task demands.Citation94,Citation95 The magnitude of coupling between a 4-Hz oscillation and y power in the prefrontal cortex increased in the working memory phase of a choice task.Citation106 In patients implanted with depth electrodes, the magnitude of θ-γ coupling in the hippocampal region varied with working memory load.Citation107 In an auditory task, γ power in the frontal and temporal sites was phase-locked mainly to θ oscillations, whereas over occipital areas phase modulation was strongest by the α rhythm in a visual task.Citation108 Computational models have suggested that θ phase-nested γ waves can support multi-item working memory, allowing for the discrete representation and sequencing of individual items or places.Citation51,Citation63 This is indeed a physiologically viable mechanismCitation107,Citation109 since γ cycle represented items within the θ cycle may be bound together by NMDA receptors.Citation66,Citation110
Yet another cross-frequency interaction is referred to as cross-frequency phase-phase or n:m coupling, when there is an integer relationship between the frequencies of the two rhythms. In n:m phase locking one observes m events associated with the “driven” cycle of one frequency occurring at n different times or phases in the “stimulus” cycle of the other.Citation111 When multiple oscillators are present simultaneously, phase-phase coupling may not be immediately obvious. However, since the repeating sequence n:m pattern is periodic in time, the distribution of the difference between phases of the oscillators can be calculated for different n:m ratios. In the case of significant phase-phase coupling between two rhythms the distribution of n:m combination deviates from a uniform distribution because the phase differences between the two rhythms oscillate around some constant value. The peaks in the phase difference distributions are known as “Arnold tongues” or synchronization tongues,Citation112 and are regarded as regions of synchronization.Citation113 Phase-phase cross-frequency synchronization has been described between θ-γ rhythms in both rats and humansCitation114,Citation115 () and between beta (β) -γ oscillations in the human magnetoencephalogram (MEG) in a working memory task.Citation98
The phenomenon of cross-frequency coupling firmly demonstrates the hierarchical organization of multiple brain rhythms in both space and time and implies that time in the brain is represented at multiple correlated scales.Citation7,Citation16,Citation84,Citation102,Citation103 Computational models have explored potential theoretical advantages of cross-frequency coupling,Citation4,Citation51,Citation116,Citation117 and the mechanisms of cross-frequency coupling may form the backbone of a neural syntax, which allows for both segmentation and linking of spike trains into cell assemblies (“letters”) and assembly sequences (neural “words”).Citation53
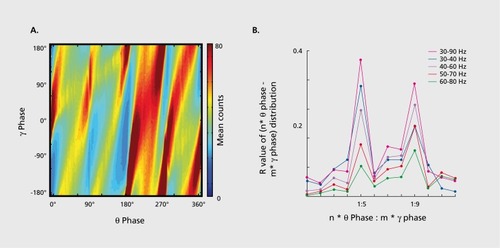
Spike content of brain rhythms
While local field potentials provide reliable information about the group actions of neurons, they do not fully represent the true common currency of interneuronal communication: action potentials, or “spikes” that the cell “fires.” While local field potential oscillations can be taken as a signal regarding the action potential-generating status of a particular population of neurons, it is mainly the action potential output of the neuron that can inform its downstream partners. Interest in brain rhythms has greatly increased recently largely due to our better understanding of the spike content of oscillations.Citation7 These combined spike-field experiments further illustrate that time in the brain is coded at multiple temporal scales and we will discuss representative experiments below.
One such example comes in the form of hippocampal “place cells”: neurons (which are actually pyramidal and granule cells of the hippocampus) that show an orderly firing of action potentials specifically correlated with the location of the rat in its environment. Assemblies of such neurons provide enough spatial information that they can be used to define a particular position of space.Citation118,Citation119 Furthermore, in a running/moving animal there is a constantly updating process of prediction of the places the animal will visit in the future by the firing of a spatially organized series hippocampal place cell neurons representing places directly ahead of the animal along its anticipated path.Citation66,Citation120 Within a given θ cycle, the neurons active at the trough of that cycle appear to represent the current location of the rat, neurons active earlier in the cycle seem to represent already-visited places and later-activated neurons represent future locations () The temporal relationships of these predictively activated neurons are governed by a “compression rule”: within the θ cycle, the spike timing sequence of neurons predicts the upcoming sequence of locations in the path of the rat, with larger time lags representing proportionally larger distances or travel timesCitation17,Citation66,Citation121 (Figure 5). The time compression mechanism has important consequences on the assembly organization of hippocampal neurons. Because of the small time offsets between the place cell spikes within the γ cycle, the summed spikes of many overlapping place neurons will generate a group oscillation whose frequency is slower than the oscillation frequency of the constituent neurons. As a result, an interference pattern emerges between the larger γ frequency oscillation and the cycle created by the action potentials of active single neurons, known as “phase precession” of place cellsCitation122 (Figure 5). A consequence of the correlation of absolute and γ phase-related time scales of spikes is that the distance traveled from the beginning of the place field can be instantly inferred from the γ phase of the place cell spikesCitation66,Citation120,Citation122,Citation123 (Figure 5).
The within-γ cycle time lags between place neurons are largely responsible for determining the globally coherent γ oscillation in the hippocampal system.Citation31 -Citation121,Citation124,Citation125 An important corollary of the relation between different time scales is that place cells continue to represent the same positions and distances in the same environment even when the running speed of the rat varies,Citation126,Citation127 since the oscillation frequency of place cells increases in proportion to the velocity.Citation127,Citation128 Another ramification is that the natural upper limit of distance coding by γ-scale time lags (~50cm for neurons in the dorsal hippocampus)Citation120,Citation123 is limited by the duration of the γ cycle (120-150 msec in the rat). Objects and locations > 50 cm ahead of the rat are initially less distinguishable on this neuronal spike-timing map from more distant landmarks, but as the animal approaches, they are progressively better resolved by the interleaved cell assemblies. The within-θ cycle temporal lags between neurons limit how many cell assemblies can nest in a given θ period (7 to 9, as reflected by the number of γ cycles per θ cycle).Citation12,Citation14,Citation51,Citation63,Citation129
Because of this temporal limitation, the hippocampus functions as a “spatial zoom” device, so that distance resolution scales with the size of the environment; place fields are small in small enclosures and large in large environments, appropriately scaling to the information at hand.Citation128,Citation130,Citation131
Assuming that place locations can be regarded as analogues to other discrete items,Citation51,Citation120 the temporal compression mechanism is then a limiting factor of the “register capacity” of the memory “buffer.” Citation51,Citation65,Citation110,Citation132 Recall from long-term episodic memory can enter conscious working memory in “chunks” of 7±2 items at a time in such a way that the spatiotemporal resolution of events near to the recalled event is higher than the resolution for the far past or far future, relative to the recalled event.Citation51,Citation128 Only by moving the content of recall forward in perceived time, do subsequent events emerge with high contextual resolution.Citation120
The within-θ cycle delays between place cells are secured by perisomatic inhibition.Citation127,Citation133 As a result of this cycle-based organization, almost the entire phase space of the θ cycle is utilized, by the firing of neurons representing, past, present and future places (Figure 5). Optogenetic suppression of parvalbumin-immunoreactive, perisomatic interneurons impaired the phase distribution of place cells and increased their tendency to fire synchronously near the trough.Citation134 Pharmacological activation of cannabinoid (CB1) receptors can also impair the proper timing between place cells without affecting the spatial tuning of the hippocampal cells. Despite the intact hippocampal spatial map, the animal under the influence of cannabinoid cannot solve a spatial memory task, presumably because the downstream reader networks of hippocampal neurons cannot decode the time-jittered spikes.Citation135
The above examples from work on the hippocampus demonstrate that representation of multiple time scales is a fundamental feature of cortical function. Similar coding strategies may support the emergence of “grid cells”Citation136 (neurons found to be active not only in particular places in the environment but occupying an array of places which are regularly spaced in a grid of equilateral triangles) in the entorhinal cortexCitation14,Citation137-Citation140 and other complex functions in the prefrontal cortex and other structures.Citation141-Citation144
Temporal compression is also at play while the network is not receiving new sensory input, or is “offline.” As described earlier, “place cells” are neurons which fire when the animal is physically in certain places in the environment and in the waking animal. During behavioral pauses just before an animal runs across an environment, the sequence of anticipated place cell firings during the animal's future run are “preplayed” in a compressed manner during sharp wave -ripple (SPW-R) events which last 80-150 ms.Citation104 They are also “replayed” after reaching the end of the run but this time in reverse sequence, as if the path is retracted.Citation145,Citation146 Such compressed replay is also routinely observed in non-REM sleep.Citation147,Citation148 The bidirectional re-enactment of temporal sequences during SPW-Rs is critical for memory consolidationCitation64,Citation66,Citation149-Citation154 and may also contribute to creative associations in the subsequent waking episodes.Citation155,Citation156 Similar time-compressed off-line replay of waking activity has been also documented in the neocortexCitation80,Citation157-Citation161 and striatum,Citation162,Citation163 suggesting that multiple time-scale representation is a general phenomenon in the brain.
Thus, neuronal oscillations organize the spiking activity of multiple neurons in a number of manners, which appear to allow for prediction, recall, consolidation, and creative association. Furthermore, this appears to be a phenomenon utilized more broadly than by only the spatial processing system and may underlie a great deal of efficient neural information handling.
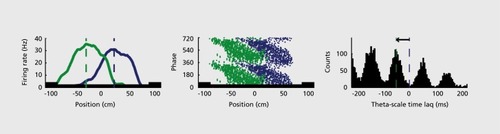
Oscillations can promote both spike synchrony and asynchrony
Oscillations and synchrony are often used synonymously and an often-expressed objection against the utility of network oscillations is that rhythmically discharging neurons are predictable and synchronized spikes are largely redundant.Citation164-Citation166 In line with this reasoning, the most synchronized network patterns occur during sleep, anesthesia and various diseases, such as epilepsy and Parkinson's disease.Citation167 In contrast to these states, the effectiveness of a representation system is characterized by high variability with minimal interactions among the constituents, furthermore improvement of behavioral performance in behavioral tasks is often characterized by increased interspike variability and reduced spike correlation among neurons.Citation109,Citation165,Citation168-Citation172 This view appears to be at striking odds with suggestions that neuronal oscillations enhance the coding ability of neurons.Citation4,Citation5,Citation17,Citation58,Citation122
There seems to be a compromise between the information processing capacity of neuronal networks and the need for some level of synchronization of their neurons for efficient communication. Fast communication among pyramidal neurons in the cortex is established by AMPA-type glutamate receptors, which are weak and have high probability of transmission failures.Citation173 Therefore, in order to convey information to peer neurons, pyramidal cells should come together in transient coalitions, or cell assemblies.Citation46,Citation53 As discussed above, the goal of the temporal assembly formation is to discharge the downstream neurons. Thus, for effective communication there is a requirement for a minimum level of synchrony and it may be that γ cycle-defined cell assemblies represent a useable packet of information, which contain varying specific content.Citation53
Cross-frequency coupling between local γ oscillations and the more global slower rhythms is established by phase synchrony. However, this coupling mechanism does not imply that the slower oscillation magnifies the synchrony in the γ period. Quite the contrary, γ cycle-embedded cell assemblies are distributed across the phase space of the modulating slow oscillation, as demonstrated above for θ oscillations. In inhibition-based oscillations, the recruitment of assemblies is deliberately protracted over time. Instead of narrowing the time window of synchronization, multiple assemblies are separated in nested γ cycles within the slower, modulating θ cycle. In contrast to the intuitive “oscillations-enhance-synchrony” picture, temporal synchrony among principal neurons is lowest during θ oscillations and highest during slow-wave sleep. Another counterintuitive observation is that the coefficient of variation (CV) of the interspike intervals of hippocampal principal cells is also largest during θ-associated exploration behaviors and smallest during slow-wave sleep.Citation174 The potential mechanism underlying such “paradoxical” asynchrony and spike variability-promoting functions of θ oscillation is its ability to temporally coordinate excitatory and inhibitory synaptic inputs to neurons.Citation12,Citation31,Citation175 Modeling studies have demonstrated that temporally precise correlated fluctuations of excitatory and inhibitory currents can reduce or cancel each others' action and decorrelate the synaptic current of postsynaptic neurons.Citation166,Citation176 As a result, spike timing variability can increase and synchrony across neurons can be reduced, due the strong θ-correlated excitatory and inhibitory inputs impinging upon most pyramidal cells. Under this hypothesis, precise temporal coordination and synchrony have different meanings, since sequential but temporally precise activity of neurons are deemed asynchronous or “polychromous”Citation177 not synchronous. Similar to observations in other systems,Citation109,Citation168,Citation169 enhanced irregularity of spike intervals should have computational advantageous in the hippocampus. It will be important to learn whether such features are specific to hippocampal θ oscillations or can be generalized to other types of rhythms as well.
Relationship of neural oscillations and spiking clynar psychiatric disease
In the sections above we have laid out the fundamental role played by oscillations in information processing and coding in the brain. We have illustrated that oscillations play a number of roles, all apparently subserving efficient information handling, including coordinating the activities of neurons both in small and large regions of brain, allowing for a unique method of transmission of information from sender regions to receiver regions and creating “packets” of information in the form of assemblies of neurons firing action potentials within certain phases of the oscillations, yet in a manner that maintains high information content. Thus, it appears oscillations may be fundamental to cognition and brain function overall.
The temporal coordinating properties of network oscillations are of course not only relevant to the healthy brain, but also with the pathological processes of psychiatric disease. Psychiatric disease includes symptoms of disordered emotion, perception, and reality testing, not to mention the somewhat less well-defined personality disorders and other characlerologic disorders wherein much more subtle disruptions of socialization, attachment and overall personal functioning are described. Despite the fact that many symptoms in psychiatric disease do not lie in the domains classically studied by electrophysiologically-oriented neuroscientists, such as visual perception, spatial processing, or learning and memory, it has become clear that all psychiatric disorders have a basis in the brain and likely electrophysiologic processes.Citation178,Citation179 Indeed neural circuits from invertebrates to vertebrates and from the cortex to the limbic system have been shown to consistently share many fundamental properties, including basic principles of connectivity, oscillatory activity, and oscillation-related control of assembly spike timing.
We believe that neuronal oscillations provide the right level of inquiry for strengthening the link between research in animals and psychiatric disease and therefore we review briefly below the work-in-progress in this field and discuss possible future directions.
Brain oscillations as quantifiable phenotypes
Brain oscillations are among the most heritable traits in mammals,Citation180 as known since the earliest twin studies of the human EEG.Citation181-Citation185 During early ontogeny brain physiology undergoes dramatic changes,Citation186-Citation188 and these changes continue into adulthood.Citation92,Citation189 However, despite these developmental/maturational changes, within-subject patterns are remarkably stable when retested after several months, much more so than variability across subjects.Citation190 Healthy adults show a remarkably stable power spectral pattern in the 8 to 16 Hz band during sleep, which allows >90% correct discrimination among individuals,Citation191,Citation192 independent of the level of education or general intelligence.Citation193 Monozygotic twins show high similarity of spontaneous EEG for all frequencies and brain areas with close to correlations levels of r = 0.9 across pairs. The concordance within heterozygotic twins is less but still higher than between non-twin siblings.Citation191,Citation194-Citation197 Not only self-organized (“spontaneous”) but also reactive patterns are under strong genetic control, as shown by a high index of heritability (0.9) of visually induced y-band (45-85 Hz) activityCitation198 () Similar to human studies, the various oscillatory patterns studied in rodents also show highly reliable genetic control.Citation199-Citation207 The quantitatively reliable discrimination between brains by physiological means lead to the suggestions that they can be used for “fingerprinting” individuals.Citation191 Such characterization may be further improved when brain oscillations are not considered separately but as a system and when cross-frequency coupling mechanisms are also taken into consideration.
If fingerprinting of individuals is possible by EEG and magneto-encephalogram (MEG), it is certainly a useful way to characterize neurological and mental diseases from the perspective of brain activity. Such “rhythmopathies,” “oseillopathies” or “dysrhythmias” may reflect malfunctioning networks.Citation167,Citation208 While EEG is one of the oldest diagnostic tools for identifying and characterizing certain neurological and psychiatric diseases, recent progress in understanding the origin and physiological significance of brain rhythms has renewed interest in this area of clinical research.Citation209
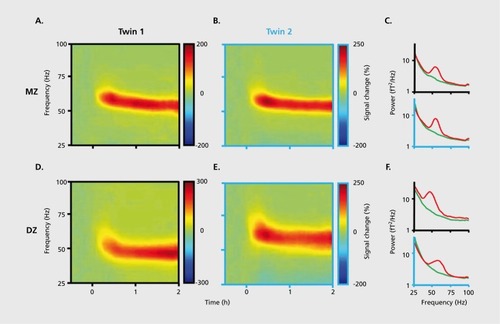
Oscillation phenotypes of psychiatric diseases
A great number of recent publications report impaired γ-band oscillations in schizophrenic patients in a variety of behavioral tasks, including the ability of cortical fields to “passively” reflect or follow externally imposed auditory frequencies, evoked responses after single stimuli and transcranial magnetic stimulation-induced response.Citation61,Citation210-Citation212 Furthermore, schizophrenic patients both have decreased power of localized γ oscillations but also have perturbed γ synchronization across hemispheres or other large anatomical distances.Citation213,Citation214 In addition, many recent reports have correlated degree of γ oscillation disturbance with degree of schizophrenia symptoms including the cognitive impairment classical in schizophrenia, positive symptoms (ie, hallucinations and delusions) and negative symptoms (ie, affective blunting, anhedonia, avolitional state).Citation213,Citation215,Citation217 Finally, these γ disturbances are also present in first-degree relatives of schizophrenic patients without classical schizophrenia symptoms per se and therefore may be a traceable endophenotype.Citation211,Citation218,Citation219
The rekindled interest in the study of γ oscillations mainly lies in the understanding of the critical involvement of parvalbumin-immunoreactive basket interneurons in γ oscillationsCitation13 and the relatively selective degeneration of this parvalbumin class of neurons in schizophrenia.Citation220 Schizophrenic patients also show a significant reduction in sleep spindlesCitation221,Citation222 and θ-γ phase coupling.Citation223 γ oscillation disturbances may also point to alterations in the glutamatergic system, given that glutamate neurons are the primary backbone of neural signaling. Indeed data have shown that glutamate-targeted treatments for schizophrenia may have efficacy in schizophrenic patients.Citation224
While schizophrenia is perhaps the most pervasive and debilitating psychiatric disease, depression is the most prevalent, with roughly 20% lifetime incidence in Western populations and it inflicts the third largest measured disease burden of all illnesses in the world.Citation225,Citation226 Numerous studies have noted oscillation-related differences between depressed patients versus controls: increased α and β rhythm power, increased β asymmetric a in the frontal aspects of the hemispheres, and sensory-evoked responses.Citation227,Citation228 Such differences may be leveraged in better treating this debilitating and sometimes-lethal disease. At the moment, while a variety of treatments exist for depression, the current state of the art in drug-based treatment is based on statisticallyinformed trial and error.Citation229 This is due to the fact that there is no reliable means of determining which medication will work for which individual patient. A number of researchers have begun to use EEG to not only point to differences between depressed patients and healthy controls, but have also begun to find correlates between brain rhythms and likelihood of response.Citation227,Citation228 Treatment response is predicted by a number of electrical parameters including baseline α power, α power asymmetry, frontal θ concordance measures within a few days of treatment, evoked responses to auditory stimuli and combinations of these measures. In general these parameters are able to predict responsivity at rates of approximately 60% to 80% accuracy.Citation227,Citation228 A particularly clinically relevant question is whether a particular measure can predict which medication to choose for a particular patient and a few studies have found that a measure called LDAEP (loudness-dependence of the auditory evoked potential) can do just that differentiating between patients likely to respond to serotonergic versus non-serotonergic antidepressants.Citation228,Citation230
Relatively less attention has been given to bipolar disorder, despite its severity. However, there have been findings of altered induced responses in the β and γ bands.Citation231-Citation253 In alcoholic patients (after long abstinence from alcohol), resting β is increased, α is low in certain subsets, α-band interhemispheric coupling is greater, γ is decreased in visual tasks, and evoked responses are less.Citation234 Attention-deficit hyperactivity disorder patients have been consistently found to have increased frontal θ power and increased γ-β ratio compared with controls,Citation35 γ power in response to auditory stimuli have also been found to be increased.Citation236 Abnormalities of α and β rhythms have also been found in personality-disordered patients.Citation237-Citation239 Patients with autism spectrum disorders (ASD) also have remarkably altered EEG/MEG patterns, often time characterized by “disorganized” but high γ power and reduced rhythm.Citation211,Citation240-Citation242
Advancing disease understanding
We believe that, while investigation of oscillations in the brain can also deepen our understanding of the pathophysiology of mental disease, progress has been disappointingly slow, with discoveries of new psychotherapeutic drugs practically at a standstill, and development of homologous relevant animal models being extremely challenging.Citation243 Working under the principle that cognition and perception are supported by brain-generated ensemble patterns in cortical networks and that impairment of proper temporal organization underlies the various deficits associated with psychiatric and neurological disorders, then studying network oscillations should be an effective and novel method for both furthering our understanding of the basis of neuropsychiatry disease and for finding new treatments. Network oscillations have a combination of properties, which allows them to be particularly appropriate targets for further mechanistic and therapeutic research. First, as discussed above, network oscillations are robust pheno types whose general properties are well preserved throughout mammalian evolution. Second, rhythms vary within a small but reliable range from individual to individual in a manner that has been born out by data to relate to function and disease. Third, on a shorter time scale oscillations are strongly influenced by both overt and cognitive behaviors. Fourth, different rhythms are specific to particular brain structures and, finally, they have the right temporal scale for the assessment of cell assembly patterns. These features, when combined, suggest that reliably measurable signals correlating with specific disease and functional impairment can be detected in specific regions of the brain in response to specific behaviors and stimuli and can be reliably studied in animals to further our understanding, with an aim towards novel therapeutics.
A likely helpful approach to efforts to bridge animal and human investigations will be to more actively build a library of shared phenotypes between animals and humans. There are a number of animal disease models for various psychiatric diseases, which have been characterized along various levels of assessment. The types of characterizations carried out in animals are limited in humans. Conversely, human phenotypes, such as the oscillatory phenotypes described above, have not been carefully assessed in experimental animals, either in specific disease models or in wild-type animals. Further building our database of disease-related oscillation phenotypes shared between animals and humans represent a rational first step in any efficient effort to understand mechanisms of neuropsychiatry disease at the level of neuronal communication. Clear human-to-animal links have been made in other domains of human diseaseCitation244 and neuroscience,Citation245 and have yielded efficient and clinically relevant findings. Such an effort towards understanding how psychiatric disease rests upon the fundamental scaffolding of brain function, ie, fast time-scale oscillatory- and assembly-based action may be taken on piecemeal the way most of research is done now, but it may be most usefully pursued more systematically in the form of the efforts of a large institution or consortium.
A novel path for treatment
The predominant mode of psychiatric treatment today is based on pharmacology and it may be useful to consider network level phenotypes as an intermediate link between disease and drug action. Brain network-specific oscillations and cross-frequency coupling of their interactions can be quantified effectively in both sleeping and task-solving animals, and since network patterns are specifically and differentially affected by a large spectrum of psychotropic drugs,Citation104,Citation246 they can be used in early screening. Unlike the often-varying drug responses between humans and animal models across many measures,Citation243 the pharmacological profiles of network oscillations are identical in all mammalian species. For more detailed analysis, large-scale recordings of multiple single neurons in the behaving animal can be used to assess both the mechanistic network level effects of both drugs that are already known to be effective in humans and also novel/other agents, which may have novel mechanisms on the same systems. Such work would of course be informed by work on network alterations in human disease and once animal-based discoveries are made they can be translated back to the human.
In addition, the oscillation-centric approach offers an alternative to pharmaceutical-based interventions: direct assessment and potential correction of aberrant brain activity based on the measurement of that activity itself. Such approaches would certainly be appealing in cases of drug resistance, but are potentially useful as even firstline treatment, especially if they can remain noninvasive. In fact, a version of such therapies have existed for years in the form of biofeedback, where patients are presented with various transforms of their brain rhythms and requested to alter brain rhythms in a particular direction using cognitive control. With varying degrees of practice, patients are able to learn to self-alter their brain oscillations as a proxy for improving other symptoms such as anxiety. Although such feedback treatments have been used for decades, the therapeutic outcomes have been controversial and suboptimal.Citation247 In light of new knowledge about oscillatory activity in the intact brain and in disease states, carefully controlled and targeted trials are now warranted.
For more extreme or difficult to control symptoms, aberrant brain activity can in principle be restored by appropriately patterned electrical stimulation. Furthermore, in many diseases, symptoms recur irregularly and unpredictably and are often separated by long symptomless intervals.Citation225 In such instances, closed-loop feedback brain control that leaves other aspects of brain functions unaffected is desirable. Effective clinical application of closed-loop treatment has two fundamental requirements.Citation248-Citation251 The first is recording and identifying causal pathophysiological network patterns. The second requirement is closed-loop feedback stimulation of the target circuits whose activation can interfere with the emerging pathological pattern. shows a proof of principle for this approach. The detected pathophysiological pattern is the thalamocortical spike-and-wave pattern in a genetic model of generalized, absence seizures in the rodent. The spike components of the pattern can be readily detected by surface or scalp recordings and used as a trigger to trigger an effector mechanism. Using transcranial electrical stimulation (TES) or optogenetic activation of the neocortex as effectors, closed-loop feedback could effectively reduce the duration of seizure episodes.Citation172,Citation252 Noninvasive, closed-loop stimulation may also prove effective affecting identifiable brain states. For example, “synthetic” sleep spindles can be induced by TES during sleep in schizophrenic patients with an attempt to supplement the low incidence of spindles in this disease. A recent studyCitation253 used feedback auditory stimulation to temporarily improve depressive symptoms in hypersomnic-type depressive patients, although in that study brain activity was monitored by a human operator. The authors systematically detected delta or slow waves during stage 3 sleep and once such rhythms were detected, sound stimulation was administered that did not awaken the patient but did reduce slow waves for several minutes. As a result of reducing the “depth” of sleep, depression symptoms decreased transiently but significantly.
In addition, decreased levels of sleep spindles have been detected in patients with schizophrenia.Citation221 Assuming that the deficit of sleep spindles is causal to the disease,Citation222 closed-loop stimulation of thalamic activity and resultant of sleep spindles can be used either in hospital settings or the patient's home. Another example might be specific suppression of excess γ activity specifically in the primary sensory cortices of schizophrenic patients with the goal of reducing hallucinations. However, long-term reliable and on-line monitoring of low amplitude brain activity, especially from outside the brain parenchyma remains a major challenge. In selected very deeply affected patients, subdural or depth recording might perhaps be a viable option to treat debilitating symptoms and might even be paired with the already-successful invasive deep brain stimulators for extremely treatment resistant depressed patients.Citation254,Citation255 In a recent pilot study, oscillation phenotypes were examined to determine which patients might be responsive candidates for deep stimulation treatment, finding that greater frontal θ “cordance” prior to surgery predicts greater improvement of depression in response to subcallosal anterior cingulate cortex stimulation.Citation256
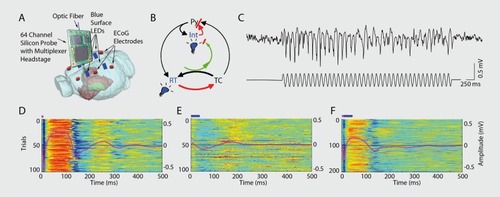
Conclusion
Given that large pharmaceutical companies are progressively reducing or eliminating research on mental disease due to the difficulty in finding new drugs, new treatments may well need to come via fundamentally different approaches to therapeutics. We have presented here alternative ideas for therapies such as closed-loop feedback that may not only circumvent the problem of decreased pharmaceutical dollars being tunneled towards psychiatric disease, but may well affect thus-far untreatable aspects of these complex diseases due to the fact that they likely work via fundamentally different means. In sum, we submit that approaching psychiatric disease from the perspective of oscillations and assembly-related fast timescale neural activity will lead at the very least to new understanding of the underpinnings of psychiatric symptoms, and possibly also represent a road to new and useful therapies.
Glossary
Brain oscillations: | = | Periodic fluctuation of excitability in groups of neurons. The coherent changes in the membrane potential of neurons create an extracellular current which can be measured by electrical recordings from the brain or scalp (such as by EEG). Since most brain rhythms are paced by inhibition, they are natural mechanisms for carrying information in temporal frames. |
Phase of oscillation: | = | Phase is the time-variant angle of the oscillatory cycle, expressed in degrees from 0 to 360 or radians. The phase repeats with every cycle of an oscillation. |
Phase space: | = | The mathematical domain of the cycle of an oscillation. In other words the entire mathematical/conceptual space covered by the combination of all phases of a particular cyclical oscillation. This “space” can be used to conceptually address events or properties happening across all aspects, or phases, of the oscillation (ie, action potentials, or comodulation of other oscillations). |
Spectral power: | = | After data is broken down into a number of component oscillations (each of a particular frequency) using a Fourier Transformation (or similar), the spectral power distribution is the series of numbers signifying the amplitude of each component oscillation. For example a pure ft) Hz sine wave has a power spectrum showing zero power everywhere but at 10-Hz. Neural data has a complex power spectrum and the peaks can characterize the dominant frequency In a given brain state. |
Local field potential (LFP): | = | Intraparencyhmal equivalent of EEG. Composed of electric current contributions from all active cellular processes within a volume of brain tissue which superimpose at a given recording location in the extracellular medium to generate an extracellular potential Ve (a scalar measured in Volts) with respect to a reference potential. The difference in Ve between two locations gives rise to an electric field (a vector whose amplitude is measured in Volts per distance). Hie proper physical term of LFP should be local potential because the field is a vector. Power Law of local field potential: A relationship between the energy of the extracellular signal and its temporal frequency. A descending straight-line on the log-log plot (power vs frequency) would be an indication of a power law that scales as 1/f“. |
Power Law of local field potential: | = | A relationship between the energy of the extracellular signal and its temporal frequency. A descending straight-line on the log-log plot (power vs frequency) would be an indication of a power law that scales as 1/f“. |
Noise (noise fluctuation): | = | Variations which occur ostensibly and practically at random, no clear relationship to oscillations, Inputs or other known system (ie, brain) states. One should distinguish between neuronal and nonneuronal (artifactual) noise. Neuronal “noise” is brain activity not directly related to the measured phenomenon but maybe critical for other phenomena. |
Cross-frequency phase coupling: | = | Phenomenon where the phase of a lower frequency oscillation modulates the amplitudes of a higher frequency oscillation to create “packets” of those higher frequency waves. Correlation/cross-correlation: A measure of the degree of similarity of two waves. |
Anti-correlation: | = | Phenomenon wherein two waves have amplitude fluctuations (aka phase) that are opposite in timing (Ie, one increases when other decreases). |
Neuronal time constant/membrane time constant: | = | An electrical property of a neuron determined by the resistance and capacitance of the neuronal cell membrane. This property determines how quickly electrical deviations from baseline remain present and are quantified as the time it takes for the membrane voltage to relax back from a step response and reach 1-1/e (approx 63.2%) of its asymptotic value. Typically 10-30 ms. |
Sharp wave-ripple: | = | Cross-frequency coupling between a sharp wave (80-150 ms) and amplitude-modulated fast oscillatory (140-200Hz) events. Sharp-wave ripples are believed to be the transfer mechanism of Information from the hippocampus to neocortex during off-line (eg, sleep) states. |
Place cells: | = | Neurons, mostly studied In the hippocampus, which fire action potentials only when the animal is In a specific place in a given environment (that place where a cell fires is called the “place field” of that cell). |
Grid cells: | = | Neurons, notably In the entorhinal cortex, which fire action potentials when the animal Is in any of a number of places which are geometrically arrayed in space in a regular grid fashion. It is conjectured that the outputs of neurons combine to create the place fields of place cells. |
REFERENCES
- DehaeneS.ChangeuxJ-P.Experimental and theoretical approaches to conscious processing..Neuron..20117020022721521609
- TononiG.EdelmanGM.Consciousness and complexity..Science.1998282184618519836628
- SherringtonCS.Man On His Nature.New York, NY; Cambridge, UK: The Macmillan Company; The University Press.1941
- VarelaF.LachauxJ-P.RodriguezE.MartinerieJ.The brainweb phase synchronization and large-scale integration.Nat Rev Neurosci.2001222923911283746
- EngelAK.FriesP.SingerW.Dynamic predictions oscillations and synchrony in top-down processing.Nat Rev Neurosci.2001270471611584308
- BuzsakiG.The structure of consciousness.Nature.200744626717361165
- BuzsakiG.DraguhnA.Neuronal oscillations in cortical networks.Science.20043041926192915218136
- FreemanWJ.RogersU.HolmesMD.SilbergeldDL.Spatial spectral analysis of human electrocorticograms including the alpha and gamma bands.J Neurosci Meth.2000951 11121
- MilsteinJ.MormannF.FriedI.KochC.Neuronal shot noise and Brownian 1/f2 behavior in the local field potential.PLoS One.20094e4338e433819190760
- BakTangWiesenfeldSelf-organized criticality: an explanation of the 1/f noise.Phys Rev Lett.19875938138410035754
- NunezPL.SrinivasanR.Electric Fields of the Brain: the Neurophysics of EEG. 2nd ed. New York, NY: Oxford University Press.2005
- BraginA.JandoG.NadasdyZ.HetkeJ.WiseK.BuzsakiG.Gamma (40100 Hz) oscillation in the hippocampus of the behaving rat.J Neurosci.199515(1 Pt 1)47607823151
- BuzsakiG.WangX-J.Mechanisms of gamma oscillations.Ann Rev Neurosci.20123520322522443509
- ChrobakJJ.BuzsakiG.Gamma oscillations in the entorhinal cortex of the freely behaving rat.J Neurosci.1998183883989412515
- SirotaA.MontgomeryS.FujisawaS.IsomuraY.ZugaroM.BuzsakiG.Entrainment of neocortical neurons and gamma oscillations by the hippocampal theta rhythm.Neuron.20086068369719038224
- SchroederCE.LakatosP.Low-frequency neuronal oscillations as instruments of sensory selection.Trends Neurosci.20093291819012975
- BuzsakiG.Rhythms of the Brain. New York, NY: Oxford University Press.2006
- SteriadeM.Impact of network activities on neuronal properties in corticothalamic systems.J Neurophysiol.20018613911431485
- BISHOPGH.CLAREMH.Potential wave mechanisms in cat cortex.Electroencephalogr Clin Neurophysiol.1956858360213375498
- MorisonRS.DempseyEW.Mechanism of thalamocortical augmentation and repetition.Am J Physiol.1943138297308
- SirotaA.CsicsvariJ.BuhlD.BuzsakiG.Communication between neocortex and hippocampus during sleep in rodents.Proc Natl Acad Sci USA.20031002065206912576550
- RaichleME.SnyderAZ.A default mode of brain function: a brief history of an evolving idea.Neurolmage.20073710831099
- LeopoldDA.MurayamaY.LogothetisNK.Very slow activity fluctuations in monkey visual cortex: implications for functional brain imaging.Cereb Cortex.20031342243312631571
- PopaD.PopescuAT.PareD.Contrasting activity profile of two distributed cortical networks as a function of attentional demands.J Neurosci.2009291191120119176827
- LehtelaL.SalmelinR.HariR.Evidence for reactive magnetic 10-Hz rhythm in the human auditory cortex.Neurosci Lett.19972221111149111741
- FontaniniA.KatzDB.7 to 12 Hz activity in rat gustatory cortex reflects disengagement from a fluid self-administration task.J Neurophysiol.2005932832284015574797
- JurkoMF.GiurintanoLP.GiurintanoSL.AndyOJ.Spontaneous awake EEG patterns in three lines of primate evolution.Behav Biol.1974103773844205545
- BuzsakiG.BickfordRG.ArmstrongDM.et al.Electric activity in the neocortex of freely moving young and aged rats.Neuroscience.1988267357443200427
- BresslerSL.FreemanWJ.Frequency analysis of olfactory system EEG in cat, rabbit, and rat.Electroencephalogr Clin Neurophysiol.19805019246159187
- VanderwolfCH.Hippocampal electrical activity and voluntary movement in the rat.Electroencephalogr Clin Neurophysiol.1969264074184183562
- BuzsakiG.Theta oscillations in the hippocampus.Neuron.20023332534011832222
- GrastyanE.LissakK.MadaraszI.DonhofferH.Hippocampal electrical activity during the development of conditioned reflexes.Electroencephalogr Clin Neurophysiol.19591140943013663816
- ArnoldsDE.Lopes daSilvaFH.AitinkJW.KampA.Hippocampal EEG and behaviour in dog. I. Hippocampal EEG correlates of gross motor behaviour.Electroencephalogr Clin Neurophysiol.19794655257088344
- ArnoldsDE.Lopes da SilvaFH.AitinkJW.KampA.BoeijingaP.The spectral properties of hippocampal EEG related to behaviour in man.Electroencephalogr Clin Neurophysiol.1980503243286160974
- KahanaMJ.SeeligD.MadsenJR.Theta returns.Curr Opin Neurobiol.20011173974411741027
- WatrousAJ.FriedI.EkstromAD.Behavioral correlates of human hippocampal delta and theta oscillations during navigation.J Neurophysiol.20111051747175521289136
- CanteroJL.AtienzaM.StickgoldR.KahanaMJ.MadsenJR.KocsisB.Sleep-dependent theta oscillations in the human hippocampus and neocortex.J Neurosci.200323108971090314645485
- HalgrenE.BabbTL.CrandallPH.Human hippocampal formation EEG desynchronizes during attentiveness and movement.Electroencephalogr Clin Neurophysiol.19784477878178806
- JerisonHJ.Evolution of the Brain and Intelligence. London, UK: Academic Press.1973
- BullmoreE.SpornsO.Complex brain networks: graph theoretical analysis of structural and functional systems.Nat Rev Neurosci.20091018619819190637
- WittnerL.HenzeDA.ZaborszkyL.BuzsakiG.Three-dimensional reconstruction of the axon arbor of a CA3 pyramidal cell recorded and filled in vivo.Brain Struct Funct.2007212758317717699
- BuzsakiG.Hippocampus: network physiology. In: Shepherd G, Grillner S, eds.Handbook of Brain Microcircuits. New York, NY: Oxford University Press, USA.2010165174
- IshizukaN.WeberJ.AmaralDG.Organization of intrahippocampal projections originating from CA3 pyramidal cells in the rat.J Cornp Neurol.1990295580623
- LiXG.SomogyiP.YlinenA.BuzsakiG.The hippocampal CA3 network: an in vivo intracellular labeling study.J Cornp Neurol.1994339181208
- WhittingtonMA.TraubRD.KopellN.ErmentroutB.BuhlEH.Inhibition-based rhythms: experimental and mathematical observations on network dynamics.IntJ Psychophysiol.20003831533611102670
- HebbDO.The Organization of Behavior: a Neuropsychological Theory New York, NY: Wiley1949
- FreundT.BuzsakiG.Interneurons of the hippocampus.Hippocampus.199663474708915675
- KlausbergerT.SomogyiP.Neuronal diversity and temporal dynamics: the unity of hippocampal circuit operations.Science.2008321535718599766
- MasquelierT.HuguesE.DecoG.ThorpeSJ.Oscillations, phase-offiring coding, and spike timing-dependent plasticity: an efficient learning scheme.J Neurosci.200929134841349319864561
- WickelgrenWA.Webs, cell assemblies, and chunking in neural nets: Introduction.Can J Exp Psychol.19995311813110389494
- LismanJE.IdiartMA.Storage of 7 +/- 2 short-term memories in oscillatory subcycles.Science.1995267151215157878473
- HarrisKD.CsicsvariJ.HiraseH.DragoiG.BuzsakiG.Organization of cell assemblies in the hippocampus.Nature.200342455255612891358
- BuzsakiG.Neural syntax: cell assemblies, synapsembles, and readers.Neuron.20106836238521040841
- DestexheA.RudolphM.PareD.The high-conductance state of neocortical neurons in vivo.Nat Rev Neurosci.2003473975112951566
- JohnstonD.WuSM-S.Foundations of Cellular Neurophysiology Cambridge, MA: MIT Press, Bradford Books1994
- MageeJC.JohnstonD.A synaptically controlled, associative signal for Hebbian plasticity in hippocampal neurons.Science.19972752092138985013
- MarkramH.LiibkeJ.FrotscherM.SakmannB.Regulation of synaptic efficacy by coincidence of postsynaptic APs and EPSPs.Science.19972752132158985014
- FriesP.A mechanism for cognitive dynamics: neuronal communication through neuronal coherence.Trends Cogn Sci.2005947448016150631
- GrayCM.SingerW.Stimulus-specific neuronal oscillations in orientation columns of cat visual cortex.Proc Natl Acad Sci USA.198986169817022922407
- CanoltyRT.EdwardsE.DalaiSS.et al.High gamma power is phaselocked to theta oscillations in human neocortex.Science.20063131626162816973878
- HasenstaubA.ShuY.HaiderB.KraushaarU.DuqueA.McCormickDA.Inhibitory postsynaptic potentials carry synchronized frequency information in active cortical networks.Neuron.20054742343516055065
- SteriadeM.Grouping of brain rhythms in corticothalamic systems.Neuroscience.20061371087110616343791
- JensenO.LismanJE.Hippocampal sequence-encoding driven by a cortical multi-item working memory buffer.Trends Neurosci.200528677215667928
- LeeAK.WilsonMA.Memory of sequential experience in the hippocampus during slow wave sleep.Neuron.2002361183119412495631
- LismanJE.Relating hippocampal circuitry to function: recall of memory sequences by reciprocal dentate-CA3 interactions.Neuron.19992223324210069330
- SkaggsWE.McNaughtonBL.WilsonMA.BarnesCA.Theta phase precession in hippocampal neuronal populations and the compression of temporal sequences.Hippocampus.199661491728797016
- SchroederCE.WilsonDA.RadmanT.ScharfmanH.LakatosP.Dynamics of active sensing and perceptual selection.Curr Opin Neurobiol.20102017217620307966
- AhissarE.ArieliA.Figuring space by time. Neuron. 200132185201
- BremmerF.KubischikM.HoffmannK-P.KrekelbergB.Neural dynamics of saccadic suppression.J Neurosci.200929123741238319812313
- HalpernBP.Tasting and smelling as active, exploratory sensory processes.Am J Otolaryngol.198342462496353966
- KepecsA.UchidaN.MainenZF.The sniff as a unit of olfactory processing.Chem Senses.20063116717916339265
- KleinfeldD.AhissarE.DiamondME.Active sensation: insights from the rodent vibrissa sensorimotor system.Curr Opin Neurobiol.20061643544416837190
- QuirogaRQ.ReddyL.KreimanG.KochC.FriedI.Invariant visual representation by single neurons in the human brain.Nature.20054351102110715973409
- Gelbard-SagivH.MukamelR.HarelM.MalachR.FriedI.Internally generated reactivation of single neurons in human hippocampus during free recall.Science.20083229610118772395
- FriesP.ReynoldsJH.RorieAE.DesimoneR.Modulation of oscillatory neuronal synchronization by selective visual attention.Science.20012911560156311222864
- BuzsakiG.Memory consolidation during sleep: a neurophysiological perspective.J Sleep Res.19987(suppl 1)17239682189
- IsomuraY.SirotaA.OzenS.et al.Integration and segregation of activity in entorhinal-hippocampal subregions by neocortical slow oscillations.Neuron.20065287188217145507
- SirotaA.BuzsakiG.Interaction between neocortical and hippocampal networks via slow oscillations.Thalamus Relat Sys.20053245259
- BattagliaFP.SutherlandGR.McNaughtonBL.Hippocampal sharp wave bursts coincide with neocortical “up-state” transitions.Learn Mem.20041169770415576887
- JiD.WilsonMA.Coordinated memory replay in the visual cortex and hippocampus during sleep.Nat Neurosci.20071010010717173043
- HuertaPT.LismanJE.Synaptic plasticity during the cholinergic thetafrequency oscillation in vitro.Hippocampus.1996658618878743
- WomelsdorfT.SchoffelenJ-M.OostenveldR.et al.Modulation of neuronal interactions through neuronal synchronization.Science.20073161609161217569862
- KopellN.ErmentroutGB.Mechanisms of phase-locking and frequency control in pairs of coupled neural oscillators. In: Fiedler B, ed.Handbook of Dynamical Systems Vol 2. Philadelphia, PA: Elsevier Science2002354
- FellJ.AxmacherN.The role of phase synchronization in memory processes.Nat Rev Neurosci.20111210511821248789
- LachauxJ-P.AxmacherN.MormannF.HalgrenE.CroneNE.High-frequency neural activity and human cognition: Past, present and possible future of intracranial EEG research.Prog Neurobiol In press2012
- von SteinA.SarntheinJ.Different frequencies for different scales of cortical integration: from local gamma to long range alpha/theta synchronization.IntJ Psychophysiol.20003830131311102669
- QuilichiniP.SirotaA.BuzsakiG.Intrinsic circuit organization and theta-gamma oscillation dynamics in the entorhinal cortex of the rat.J Neurosci.201030111281114220720120
- BuzsakiG.LeungLW.VanderwolfCH.Cellular bases of hippocampal EEG in the behaving rat.Brain Res.19832871391716357356
- SolteszI.DeschenesM.Low-and high-frequency membrane potential oscillations during theta activity in CA1 and CA3 pyramidal neurons of the rat hippocampus under ketamine-xylazine anesthesia.J Neurophysiol.199370971168395591
- MormannF.FellJ.AxmacherN.et al.Phase/amplitude reset and theta-gamma interaction in the human medial temporal lobe during a continuous word recognition memory task.Hippocampus.20051589090016114010
- DemiralpT.BayraktarogluZ.LenzD.et al.Gamma amplitudes are coupled to theta phase in human EEG during visual perception.Int J Psychophysiol.200764243016956685
- GriesmayrB.GruberWR.KlimeschW.SausengP.Human frontal midline theta and its synchronization to gamma during a verbal delayed match to sample task.Neurobiol Learn Mem.20109320821519808098
- ColginLL.DenningerT.FyhnM.et al.Frequency of gamma oscillations routes flow of information in the hippocampus.Nature.200946235335719924214
- TortABL.KramerMA.ThornC.et al.Dynamic cross-frequency couplings of local field potential oscillations in rat striatum and hippocampus during performance of a T-maze task.Proc Natl Acad Sci U S A.2008105205172052219074268
- TortABL.KomorowskiRW.MannsJR.KopellNJ.EichenbaumH.Theta-gamma coupling increases during the learning of item-context associations.Proc Natl Acad Sci USA.2009106209422094719934062
- WangX-J.Pacemaker neurons for the theta rhythm and their synchronization in the septohippocampal reciprocal loop.J Neurophysiol.20028788911826054
- CohenMX.ElgerCE.FellJ.Oscillatory activity and phase-amplitude coupling in the human medial frontal cortex during decision making.J Cogn Neurosci.20092139040218510444
- PalvaJ.Phase synchrony among neuronal oscillations in the human cortex.J Neurosci.200525396215829648
- PeyracheA.Inhibition recruitment in prefrontal cortex during sleep spindles and gating of hippocampal inputs. Proc.Natl Acad Sci USA.201110817207
- LakatosP.ShahAS.KnuthKH.UlbertI.KarmosG.SchroederCE.An oscillatory hierarchy controlling neuronal excitability and stimulus processing in the auditory cortex.J Neurophysiol.2005941904191115901760
- SterladeM.ContrerasD.Curró DossiR.NunezA.The slow (< 1 Hz) oscillation in reticular thalamic and thalamocortical neurons: scenario of sleep rhythm generation in interacting thalamic and neocortical networks.J Neurosci.199313328432998340808
- JensenO.ColginLL.Cross-frequency coupling between neuronal oscillations.Trends Cogn Sci.20071126726917548233
- anoltyRT.KnightRT.The functional role of cross-frequency coupling.Trends Cogn Sci.20101450651520932795
- BuzsakiG.Network properties of the thalamic clock: role of oscillatory behavior in mood disorders. In: Ba ar E, Bullock TH, eds.Induced Rhythms in the Brain Boston, MA: Birkhäuser1992235250
- KandelA.BuzsakiG.Cellular-synaptic generation of sleep spindles, spike-and-wave discharges, and evoked thalamocortical responses in the neocortex of the rat.J Neurosci.199717678367979254689
- FujisawaS.BuzsakiG.A 4 Hz oscillation adaptively synchronizes prefrontal, VTA, and hippocampal activities.Neuron.20117215316521982376
- AxmacherN.HenselerMM.JensenO.WeinreichI.ElgerCE.FellJ.Cross-frequency coupling supports multi-item working memory in the human hippocampus.Proc Natl Acad Sci USA.20101073228323320133762
- VoytekB.CanoltyRT.ShestyukA.CroneNE.ParviziJ.KnightRT.Shifts in gamma phase-amplitude coupling frequency from theta to alpha over posterior cortex during visual tasks.Front Hum Neurosci.2010419121060716
- GutniskyDA.DragoiV.Adaptive coding of visual information in neural populations.Nature.200845222022418337822
- JensenO.LismanJE.Hippocampal CA3 region predicts memory sequences: accounting for the phase precession of place cells.Learn Mem.1996327928710456097
- RosenblumMG.KurthsJ.PikovskyA.SchaferC.TassP.AbelHH.Synchronization in noisy systems and cardiorespiratory interaction.IEEE Eng Med Biol Mag.19981746539824761
- GuevaraMR.GlassL.Phase locking, period doubling bifurcations and chaos in a mathematical model of a periodically driven oscillator: a theory for the entrainment of biological oscillators and the generation of cardiac dysrhythmias.J Math Biol.1982141237077182
- Garcia-AlvarezD.StefanovskaA.McClintockPVE.High-order synchronization, transitions, and competition among Arnold tongues in a rotator under harmonic forcing.Phys Rev E Stat Nonlin Soft Matter Phys.200877(5 Pt 2)56203
- BelluscioMA.MizusekiK.SchmidtR.KempterR.BuzsakiG.Cross-frequency phase-phase coupling between θ and γ oscillations in the hippocampus.J Neurosci.20123242343522238079
- SausengP.KlimeschW.HeiseKF.et al.Brain oscillatory substrates of visual short-term memory capacity.Curr Biol.2009191846185219913428
- LismanJ.The theta/gamma discrete phase code occuring during the hippocampal phase precession may be a more general brain coding scheme.Hippocampus.20051591392216161035
- NeymotinSA.LazarewiczMT.SherifM.ContrerasD.FinkelLH.LyttonWW.Ketamine disrupts θ modulation of γ in a computer model of hippocampus.J Neurosci.201131117331174321832203
- O'KeefeJ.NadelL.The Hippocampus as a Cognitive Map New York, NY: Clarendon Press1978
- WilsonMA.McNaughtonBL.Dynamics of the hippocampal ensemble code for space.Science.1993261105510588351520
- DragoiG.BuzsakiG.Temporal encoding of place sequences by hippocampal cell assemblies.Neuron.20065014515716600862
- GeislerC.DibaK.PastalkovaE.MizusekiK.RoyerS.BuzsakiG.Temporal delays among place cells determine the frequency of population theta oscillations in the hippocampus.Proc Natl Acad Sci USA.20101077957796220375279
- 0'KeefeJ.RecceML.Phase relationship between hippocampal place units and the EEG theta rhythm.Hippocampus.199333173308353611
- MaurerAP.VanrhoadsSR.SutherlandGR.LipaP.McNaughtonBL.Self-motion and the origin of differential spatial scaling along the septotemporal axis of the hippocampus.Hippocampus.20051584185216145692
- BullockTH.BuzsakiG.McCluneMC.Coherence of compound field potentials reveals discontinuities in the CA1-subiculum of the hippocampus in freely-moving rats.Neuroscience.1990386096192270136
- LubenovEV.SiapasAG.Hippocampal theta oscillations are travelling waves.Nature.200945953453919489117
- HuxterJ.BurgessN.O'KeefeJ.Independent rate and temporal coding in hippocampal pyramidal cells.Nature.200342582883214574410
- GeislerC.RobbeD.ZugaroM.SirotaA.BuzsakiG.Hippocampal place cell assemblies are speed-controlled oscillators. Proc.Natl Acad Sci USA.200710481498154
- DibaK.BuzsakiG.Hippocampal network dynamics constrain the time lag between pyramidal cells across modified environments.J Neurosci.200828134481345619074018
- BuzsakiG.BuhlDL.HarrisKD.CsicsvariJ.CzehB.MorozovA.Hippocampal network patterns of activity in the mouse.Neuroscience.200311620121112535953
- FentonAA.KaoH-Y.NeymotinSA.et al.Unmasking the CA1 ensemble place code by exposures to small and large environments: more place cells and multiple, irregularly arranged, and expanded place fields in the larger space.J Neurosci.200828112501126218971467
- O'KeefeJ.BurgessN.Geometric determinants of the place fields of hippocampal neurons.Nature.19963814254288632799
- HasselmoME.BodelonC.WybleBP.A proposed function for hippocampal theta rhythm: separate phases of encoding and retrieval enhance reversal of prior learning.Neural Comput.20021479381711936962
- MaurerAP.CowenSL.BurkeSN.BarnesCA.McNaughtonBL.Phase precession in hippocampal interneurons showing strong functional coupling to individual pyramidal cells.J Neurosci.200626134851349217192431
- RoyerS.ZemelmanBV.LosonczyA.et al.Control of timing, rate and bursts of hippocampal place cells by dendritic and somatic inhibition. Wat.Neurosci.201215769775
- RobbeD.BuzsakiG.Alteration of theta timescale dynamics of hippocampal place cells by a cannabinoid is associated with memory impairment.J Neurosci.200929125971260519812334
- HaftingT.FyhnM.MoldenS.MoserM-B.MoserEl.Microstructure of a spatial map in the entorhinal cortex.Nature.200543680180615965463
- BurgessN.BarryC.O'KeefeJ.An oscillatory interference model of grid cell firing.Hippocampus.20071780181217598147
- HasselmoME.A model of episodic memory: mental time travel along encoded trajectories using grid cells.Neurobiol Learn Mem.20099255957319615456
- MizusekiK.SirotaA.PastalkovaE.BuzsakiG.Theta oscillations provide temporal windows for local circuit computation in the entorhinal-hippocampal loop.Neuron.20096426728019874793
- MoserEl.KropffE.MoserM-B.Place cells, grid cells, and the brain's spatial representation system.Ann Rev Neurosci.200831698918284371
- BenchenaneK.PeyracheA.KhamassiM.et al.Coherent theta oscillations and reorganization of spike timing in the hippocampal- prefrontal network upon learning.Neuron.20106692193620620877
- DeCoteauWE.ThornC.GibsonDJ.et al.Learning-related coordination of striatal and hippocampal theta rhythms during acquisition of a procedural maze task.Proc Natl Acad Sci USA.20071045644564917372196
- JonesMW.WilsonMA.Theta rhythms coordinate hippocampal-prefrontal interactions in a spatial memory task.PLoS Biol.20053e402e40216279838
- SiapasAG.LubenovEV.WilsonMA.Prefrontal phase locking to hippocampal theta oscillations.Neuron.20054614115115820700
- DibaK.BuzsakiG.Forward and reverse hippocampal place-cell sequences during ripples.Nat Neurosci.2007101241124217828259
- FosterDJ.WilsonMA.Reverse replay of behavioural sequences in hippocampal place cells during the awake state.Nature.200644068068316474382
- BuzsakiG.Two-stage model of memory trace formation: a role for “noisy” brain states.Neuroscience.1989315515702687720
- CarrMF.JadhavSP.FrankLM.Hippocampal replay in the awake state: a potential substrate for memory consolidation and retrieval.Nat Neurosci.20111414715321270783
- KudrimotiHS.BarnesCA.McNaughtonBL.Reactivation of hippocampal cell assemblies: effects of behavioral state, experience, and EEG dynamics.J Neurosci.19991940904101 10234037
- NadasdyZ.HiraseH.CzurkoA.CsicsvariJ.BuzsakiG.Replay and time compression of recurring spike sequences in the hippocampus.J Neurosci.1999199497950710531452
- O'NeillJ.SeniorTJ.AllenK.HuxterJR.CsicsvariJ.Reactivation of experience-dependent cell assembly patterns in the hippocampus. Wat.Neurosci.200811209215
- WilsonMA.McNaughtonBL.Reactivation of hippocampal ensemble memories during sleep.Science.19942656766798036517
- Ego-StengelV.WilsonMA.Disruption of ripple-associated hippocampal activity during rest impairs spatial learning in the rat.Hippocampus.20102011019816984
- GirardeauG.BenchenaneK.WienerSI.BuzsakiG.ZugaroMB.Selective suppression of hippocampal ripples impairs spatial memory. Wat.Neurosci.20091212221223
- MarshallL.HelgadottirH.MolleM.BornJ.Boosting slow oscillations during sleep potentiates memory.Nature.200644461061317086200
- BuzsakiG.SilvaFL.High frequency oscillations in the intact brain.Prog Neurobiol In press2012
- EustonDR.TatsunoM.McNaughtonBL.Fast-forward playback of recent memory sequences in prefrontal cortex during sleep.Science.20073181147115018006749
- HoffmanKL.McNaughtonBL.Coordinated reactivation of distributed memory traces in primate neocortex.Science.20022972070207312242447
- HuberR.GhilardiMF.MassiminiM.TononiG.Local sleep and learning.Nature.2004430788115184907
- JohnsonA.FentonAA.KentrosC.RedishAD.Looking for cognition in the structure within the noise.Trends Cogn Sci.200913556419135406
- akehara-NishiuchiK.McNaughtonBL.Spontaneous changes of neocortical code for associative memory during consolidation.Science.200832296096318988855
- LansinkCS.GoltsteinPM.LankelmaJV.JoostenRNJMA.McNaughtonBL.PennartzCMA.Preferential reactivation of motivationally relevant information in the ventral striatum. J.Neurosci.20082863726382
- PennartzCMA.LeeE.VerheulJ.LipaP.BarnesCA.McNaughtonBL.The ventral striatum in off-line processing: ensemble reactivation during sleep and modulation by hippocampal ripples.J Neurosci.2004246446645615269254
- ShadlenMN.NewsomeWT.The variable discharge of cortical neurons: implications for connectivity, computation, and information coding.J Neurosci.199818387038969570816
- ZoharyE.ShadlenMN.NewsomeWT.Correlated neuronal discharge rate and its implications for psychophysical performance.Nature.19943701401438022482
- RenartA.de la RochaJ.BarthoP.et al.The asynchronous state in cortical circuits.Science.201032758759020110507
- LlinasRR.RibaryU.JeanmonodD.KronbergE.MitraPP.Thalamocortical dysrhythmia: A neurological and neuropsychiatric syndrome characterized by magnetoencephalography.Proc Natl Acad Sci U S A.199996152221522710611366
- CohenMR.MaunsellJHR.Attention improves performance primarily by reducing interneuronal correlations.Nat Neurosci.2009121594160019915566
- MitchellJF.SundbergKA.ReynoldsJH.Spatial attention decorrelates intrinsic activity fluctuations in macaque area V4.Neuron.20096387988819778515
- AbbottLF.DayanP.The effect of correlated variability on the accuracy of a population code.Neural Comput.199911911019950724
- ompolinskyH.YoonH.KangK.ShamirM.Population coding in neuronal systems with correlated noise.Phys Rev E Stat Nonlin Soft Matter Phys.200164(5 Pt 1)51904
- EckerAS.BerensP.KelirisGA.BethgeM.LogothetisNK.ToliasAS.Decorrelated neuronal firing in cortical microcircuits.Science.201032758458720110506
- LismanJE.RaghavachariS.TsienRW.The sequence of events that underlie quantal transmission at central glutamatergic synapses. Wat.Rev Neurosci.20078597609
- MizusekiK.BuzsakiG.Brain state-dependent firing patterns of principal neurons and interneurons in the entorhinal-hippocampal circuit. Paper presented at: Society for Neuroscience Meeting 2012, New Orleans, LA, USA. October 13-17, 2012
- KamondiA.AcsadyL.WangXJ.BuzsakiG.Theta oscillations in somata and dendrites of hippocampal pyramidal cells in vivo: activity-dependent phase-precession of action potentials.Hippocampus.199882442619662139
- ChanceFS.AbbottLF.ReyesAD.Gain modulation from background synaptic input.Neuron.20023577378212194875
- SzatmáryB.IzhikevichEM.Spike-timing theory of working memory.PLoS Comput Biol In press
- NestlerE.HymanS.MalenkaR.Molecular Neuropharmacology: A Foundation for Clinical Neuroscience, Second Edition New York, NY: McGrawHill Professional2008
- KandelE.Psychiatry, Psychoanalysis, And The New Biology Of Mind Arlington, VA: American Psychiatric Publishing2005
- van BeijsterveldtCE.BoomsmaDl.Genetics of the human electroencephalogram (EEG) and event-related brain potentials (ERPs): a review.Hum Genet.1994943193307927323
- RaneyET.Brain potentials and lateral dominance in identical twins.J Exp Psychol.1939242139
- DavisH.DavisPA.Action potentials of the brain in normal persons and in normal states of cerebral activity.Arch Neurol Psychiatry.19363612141224
- LennoxWG.GibbsEL.GibbsFA.The brain-wave pattern, an hereditary trait; evidence from 74 “normal” pairs of twins.J Hered.194536233243
- VogelF.The genetic basis of the normal human electroencephalogram (EEG).Human Genetics.197010911145528299
- LykkenDT.TellegenA.ThorkelsonK.Genetic determination of EEG frequency spectra.Biol Psychol.197412452594473236
- Ben-AriY.Neuro-archaeology: pre-symptomatic architecture and signature of neurological disorders.Trends Neurosci.20083162663618951639
- LeinekugelX.KhazipovR.CannonR.HiraseH.Ben-AriY.BuzsakiG.Correlated bursts of activity in the neonatal hippocampus in vivo.Science.20022962049205212065842
- KhazipovR.SirotaA.LeinekugelX.HolmesGL.Ben-AriY.BuzsakiG.Early motor activity drives spindle bursts in the developing somatosensory cortex.Nature.200443275876115592414
- MatousekM.PetersenI.Automatic evaluation of EEG background activity by means of age-dependent EEG quotients.Electroencephalogr Clin Neurophysiol.1 9733 5603612
- GasserT.BacherP.SteinbergH.Test-retest reliability of spectral parameters of the EEG.Electroencephalogr Clin Neurophysiol.1985603123192579798
- De GennaroL.MarzanoC.FratelloF.et al.The electroencephalographic fingerprint of sleep is genetically determined: a twin study.Ann Neurol.20086445546018688819
- BuckelmullerJ.LandoltH-P.StassenHH.AchermannP.Trait-like individual differences in the human sleep electroencephalogram.Neuroscience.200613835135616388912
- PosthumaD.NealeMC.BoomsmaDl.de GeusEJ.Are smarter brains running faster? Heritability of alpha peak frequency, IQ, and their interrelation.Behav Genet.2001315677911838534
- AnokhinA.SteinleinO.FischerC.et al.A genetic study of the human low-voltage electroencephalogram.Hum Genet.199290991121427795
- van BeijsterveldtCE.MolenaarPC.de GeusEJ.BoomsmaDl.Heritability of human brain functioning as assessed by electroencephalography.Am J Hum Genet.1996585625738644716
- Linkenkaer-HansenK.SmitDJA.BarkilA.et al.Genetic contributions to long-range temporal correlations in ongoing oscillations.J Neurosci.200727138821388918077700
- SmitCM.WrightMJ.HansellNK.GeffenGM.MartinNG.Genetic variation of individual alpha frequency (IAF) and alpha power in a large adolescent twin sample.Int J Psychophysiol.20066123524316338015
- van PeltS.BoomsmaDl.FriesP.Magnetoencephalography in twins reveals a strong genetic determination of the peak frequency of visually induced y-band synchronization.J Neurosci.2012323388339222399760
- VadaszC.CarpiD.JandoG.et al.Genetic threshold hypothesis of neocortical spike-and-wave discharges in the rat: an animal model of petit mal epilepsy.Am J Med Genet.19956055637485236
- Jando'G.CarpiD.KandelA.et al.Spike-and-wave epilepsy in rats: sex differences and inheritance of physiological traits.Neuroscience.1995643013177700522
- MarescauxC.VergnesM.DepaulisA.Genetic absence epilepsy in rats from Strasbourg-a review.J Neural Trans Suppl.1992353769
- FrankenP.MalafosseA.TaftiM.Genetic variation in EEG activity during sleep in inbred mice.Am J Physiol Regul Integr Cornp Physiol.1998275R1127R1137
- oebelsJL.The biology of epilepsy genes.Ann Rev Neurosci.20032659962514527270
- NoebelsJL.Single-gene models of epilepsy.Adv Neurol.19997922723810514817
- TaftiM.Quantitative genetics of sleep in inbred mice.Dialogues Clin Neurosci.2007927317969864
- HellmanK.HernandezP.ParkA.AbelT.Genetic evidence for a role for protein kinase A in the maintenance of sleep and thalamocortical oscillations.Sleep.2010331920120617
- PeetersBW.KerbuschJM.CoenenAM.VossenJM.van LuijtelaarEL.Genetics of spike-wave discharges in the electroencephalogram (EEG) of the WAG/Rij inbred rat strain: a classical mendelian crossbreeding study.Behav Genet.1992223613681616465
- SchulmanJJ.CancroR.LoweS.LuF.WaltonKD.LlinasRR.Imaging of thalamocortical dysrhythmia in neuropsychiatry.Front Hum Neurosci.201156932421863138
- Ba arE.GuntekinB.A review of brain oscillations in cognitive disorders and the role of neurotransmitters.Brain Res.2008123517219318640103
- KwonJS.Gamma frequency-range abnormalities to auditory stimulation in schizophrenia.Arch Gen Psychiatry.1999561001100510565499
- hlhaasPJ.SingerW.Abnormal neural oscillations and synchrony in schizophrenia.Nat Rev Neurosci.20101110011320087360
- HerrmannCS.DemiralpT.Human EEG gamma oscillations in neuropsychiatry disorders.Clin Neurophysiol.20051162719273316253555
- MulertC.KirschV.WhitfordTJ.et al.Hearing voices: a role of interhemispheric auditory connectivity?.World J Biol Psychiatry.20121315315821623667
- MaharajhK.TealeP.RojasDC.ReiteML.Fluctuation of gamma-band phase synchronization within the auditory cortex in schizophrenia.Clin Neurophysiol.201012154254820071232
- SpencerKM.NestorPG.NiznikiewiczMA.SalisburyDF.ShentonME.McCarleyRW.Abnormal neural synchrony in schizophrenia.J Neurosci.2003237407741112917376
- SpencerKM.NiznikiewiczM a.NestorPG.ShentonME.McCarleyRW.Left auditory cortex gamma synchronization and auditory hallucination symptoms in schizophrenia.BMC Neurosci.2009108519619324
- Gonzalez-BurgosG.HashimotoT.LewisDA.Alterations of cortical GABA neurons and network oscillations in schizophrenia.Curr Psych Rep.201012335344
- ClementzBA.BlumenfeldLD.CobbS.The gamma band response may account for poor P50 suppression in schizophrenia.Neuroreport.19978388938939462461
- RassO.ForsythJK.KrishnanGP.et al.Auditory steady state response in the schizophrenia, first-degree relatives, and schizotypal personality disorder.Schizophr Res.201213614314922285558
- LewisDA.HashimotoT.VolkDW.Cortical inhibitory neurons and schizophrenia. Wat.Rev Neurosci.20056312324
- errarelliF.PetersonMJ.SarassoSet al.Thalamic dysfunction in schizophrenia suggested by whole-night deficits in slow and fast spindles.Am J Psychiatry.20101671339134820843876
- FerrarelliF.TononiG.The thalamic reticular nucleus and schizophrenia.Schizophr Bull.20113730631521131368
- LismanJ.BuzsakiG.A neural coding scheme formed by the combined function of gamma and theta oscillations.Schizophr Bull.20083497498018559405
- PatilST.ZhangL.MartenyiF.et al.Activation of mGlu2/3 receptors as a new approach to treat schizophrenia: a randomized Phase 2 clinical trial.Nat Med.2007131102110717767166
- SadockBJ.SadockVA.Kaplan & Sadock Comprehensive Textbook of Psychiatry Philadelphia, PA: Lippincott Williams & Wilkins20004884
- MathersC.FatDM.OrganizationWH.BoermaJT.The Global Burden of Disease: 2004 Update Geneva, Switzerland: World Health Organization2008
- AlhajH.WisniewskiG.McAllister-WilliamsRH.The use of the EEG in measuring therapeutic drug action: focus on depression and antidepressants.J Psychopharmacol.20112511751191 21106608
- losifescuDV.Electroencephalography-derived biomarkers of antidepressant response.Harvard Rev Psychiatry.201119144154
- rivediMH.RushAJ.WisniewskiSR.et al.Evaluation of outcomes with citalopram for depression using measurement-based care in STAR*D: implications for clinical practice. Am J.Psychiatry.20061632840
- HegerlU.JuckelG.Intensity dependence of auditory evoked potentials as an indicator of central serotonergic neurotransmission: a new hypothesis.Biol Psychiatry.1993331731878383545
- OribeN.OnitsukaT.HiranoS.et al.Differentiation between bipolar disorder and schizophrenia revealed by neural oscillation to speech sounds: an MEG study.Bipolar Disord.20101280481221176027
- O'DonnellBF.HetrickWP.VohsJL.KrishnanGP.CarrollCA.ShekharA.Neural synchronization deficits to auditory stimulation in bipolar disorder.Neuroreport.2004151369137215167568
- RassO.KrishnanG.BrennerCA.et al.Auditory steady state response in bipolar disorder: relation to clinical state, cognitive performance, medication status, and substance disorders.Bipolar Disord.20101279380321176026
- RangaswamyM.PorjeszB.Uncovering genes for cognitive (dysfunction and predisposition for alcoholism spectrum disorders: a review of human brain oscillations as effective endophenotypes.Brain Res.2008123515317118634760
- LooSK.MakeigS.Clinical utility of EEG in attention-deficit/hyperactivity disorder: a research update.Neurotherapeutics.2012956958722814935
- YordanovaJ.BanaschewskiT.KolevV.WoernerW.Rothenbergera.Abnormal early stages of task stimulus processing in children with attention-deficit hyperactivity disorder-evidence from event-related gamma oscillations.Clin Neurophysiol.20011121096110811377270
- BauerLO.HesselbrockVM.EEG, autonomic and subjective correlates of the risk for alcoholism.J Stud Alcohol.1993545775898412148
- ChiSE.ParkCB.LimSL.et al.EEG and personality dimensions: A consideration based on the brain oscillatory systems.Pers Individ Diff.200539669681
- HegerlU.SteinM.MulertC.et al.EEG-vigilance differences between patients with borderline personality disorder, patients with obsessive-compulsive disorder and healthy controls.Eur Arch Psychiatry Clin Neurosci.200825813714317990048
- OrekhovaEV.StroganovaTA.NygrenG.et al.Excess of high frequency electroencephalogram oscillations in boys with autism.Biol Psychiatry.2007621022102917543897
- GriceSJ.SpratlingMW.Karmiloff-SmithA.et al.Disordered visual processing and oscillatory brain activity in autism and Williams syndrome.Neuroreport.2001122697270011522950
- BernierR.DawsonG.WebbS.MuriasM.EEG mu rhythm and imitation impairments in individuals with autism spectrum disorder.Brain Cogn.20076422823717451856
- NestlerEJ.HymanSE.Animal models of neuropsychiatric disorders.Nat Neurosci.2010131161116920877280
- WeinbergRA.The Biology of Cancer New York, NY; London, UK: Garland Science2006
- CasalsJB.PieriNCG.FeitosaMLT.et al.The use of animal models for stroke research: a review.Compar Med.201161305313
- AgidY.BuzsakiG.DiamondDM.et al.How can drug discovery for psychiatric disorders be improved?.Nat Rev. Drug Discov.2007618920117330070
- HammondDC.The need for individualization in neurofeedback: heterogeneity in QEEG patterns associated with diagnoses and symptoms.Appl Psychophysiol Biofeedback.201035313619760143
- RosinB.SlovikM.MitelmanR.et al.Closed-loop deep brain stimulation is superior in ameliorating parkinsonism.Neuron.20117237038422017994
- MorrellMJ.Responsive cortical stimulation for the treatment of medically intractable partial epilepsy.Neurology.2011771295130421917777
- HatsopoulosNG.DonoghueJP.The science of neural interface systems.Ann Rev Neurosci.20093224926619400719
- JacksonA.FetzEE.Interfacing with the computational brain.IEEE Trans Neur Syst Rehabil Eng.201119534541
- BerenyiA.BelluscioM.MaoD.BuzsakiG.Closed-loop control of epilepsy by transcranial electrical stimulation.Science.201233773573722879515
- LandsnessEC.GoldsteinMR.PetersonMJ.TononiG.BencaRM.Antidepressant effects of selective slow wave sleep deprivation in major depression: a high-density EEG investigation.J Psychiatr Res.2011451019102621397252
- Holtzheimer 3rdPE.MaybergHS.Deep brain stimulation for treatment-resistant depression.Am J Psychiatry.20101671437144421131410
- GoodmanWK.AltermanRL.Deep brain stimulation for intractable psychiatric disorders.Ann Rev Med.20126351152422034866
- BroadwayJM.HoltzheimerPE.HilimireMR.et al.Frontal theta cardance predicts 6-month antidepressant response to subcallosal cingulate deep brain stimulation for treatment-resistant depression: a pilot study.Neuropsychopharmacology.2012371764177222414813