Abstract
Psychiatric treatment relies on a solid armamentarium of pharmacologic and nonpharmacologic treatment modalities that perform reasonably well for many patients but leave others in a state of chronic disability or troubled by problematic side effects. Treatment planning in psychiatry remains an art that depends on considerable trial and error. Thus, there is an urgent need for better tools that will provide a means for matching individual patients with the most effective treatments while minimizing the risk of adverse events. This review will consider the current state of the science in predicting treatment outcomes in psychiatry. Genetic and other biomarkers will be considered alongside clinical diagnostic, and family history data. Problems inherent in prediction medicine will also be discussed, along with recent developments that support the hope that psychiatry can do a better job in quickly identifying the best treatments for each patient.
El tratamiento psiquiátrico cuenta con un completo arsenal de modalidades terapéuticas farmacológicas y no farmacológicas que funcionan relativamente bien en muchos pacientes, pero que dejan a otros incómodos por importantes efectos secundarios o en un estado de discapacidad crónica. Los planes de tratamiento en psiquiatría continúan siendo un arte que depende en forma considerable del ensayo error. Por lo tanto, existe una urgente necesidad de mejores herramientas que proporcionen los medios para asociar a pacientes individuales con las terapias más efectivas al mismo tiempo que minimicen el riesgo de efectos adversos, Esta revisión aborda el estado actual de la ciencia respecto a la predicción de resultados terapéuticos en psiquiatría. La genética y otros biomarcadores se consideran junto con información clínica, diagnóstica y de la historia familiar. Se discuten problemas inherentes a la predicción en medicina y también los desarrollos recientes que sustentan la esperanza que la psiquiatría pueda realizar un mejor trabajo en la rápida identificación de las mejores terapias para cada paciente.
Les traitements psychiatriques reposent sur un arsenal solide de modalités thérapeutiques pharmacologiques et non pharmacologiques assez efficaces pour de nombreux patients, mais qui en laissent d'autres dans un état d'incapacité chronique ou gênés par des effets indésirables problématiques. La planification d'un traitement en psychiatrie reste un art qui dépend beaucoup d'essais et d'erreurs, il est donc urgent de trouver de meilleurs outils pouvant apparier chaque patient au traitement le plus efficace, tout en minimisant le risque d'événements indésirables. Cet article concerne l'état actuel des connaissances permettant de prédire les résultats des traitements en psychiatrie. Les biomarqueurs génétiques et d'autres marqueurs sont mentionnés aux côtés des données cliniques, diagnostiques et familiales. Nous analysons les problèmes inhérents à la médecine prédictive, parallèlement aux développements récents laissant espérer que la pratique de la psychiatrie peut être améliorée en identifiant rapidement les meilleurs traitements pour chaque patient.
Introduction
Over the past 50 years, clinical psychiatry has been revolutionized by the development of a broad range of psychopharmaceutical agents that can effectively ameliorate symptoms of many major mental illnesses, including schizophrenia, mood disorders, and anxiety disorders. Despite these advances, no curative treatments have yet been developed, so patients must generally take medications for a long period of time—often years—creating a risk for long-term adverse events.Citation1 Although most currently used psychopharmaceutical agents are safe and well-tolerated, side effects can be problematic even with acute use, leading to reduced treatment adherence and poorer outcomes.Citation2 Moreover, response rates for most psychopharmaceutical agents are not optimal, and it can take months for clinicians to find the best medication or combination treatment for individual patients. Even less is known about predictors of response to vital nonpharmacological treatments such as psychotherapy.
Improved prediction of treatment response could have many benefits for patients and reduce the health care costs that accrue from the use of inappropriate or suboptimal treatments. This is a key goal of the personalized medicine movement.Citation3 However, prediction is a challenging endeavor, since many different variables can affect individual treatment outcomes. Among these, diagnosis, comorbidity, and treatment adherence are clearly important. In recent years, considerable effort has gone into developing biomarkers of treatment response that might supplement clinical factors. Genetic markers have received the most attention thanks to the exciting advances in pharmacogenomic research.Citation4
Pharmacogenomics aims to reduce acute and longterm adverse events and optimize response rates by using genetic information to match medications to individual patients.Citation5 Pharmacogenomic research has advanced greatly in recent years, owing to the wealth of data and new technologies that have arisen from the Human Genome Project and related initiatives. However, firm genetic associations have proven to be the exception rather than the rule, perhaps owing to limited sample sizes. A few robust research findings have emerged, but the translation of these findings into better clinical care remains challenging. Other biomarkers are being sought by a variety of methods, including neuroimaging, proteomics, and metabolomics. These areas are still relatively unexplored in psychiatry as sources of information that could inform prediction of treatment outcomes. New advances are expected in the near future that could alter the impact of these kinds of biomarkers in psychiatry.
In this review, the current state of treatment prediction research in psychiatry will be reviewed, with the aim of summarizing progress and highlighting areas where research findings have the best potential for near-term translation to clinical practice. This is not a systemic overview of the literature, but rather an attempt to provide an expert review that summarizes key findings for a nonspecialist audience.
We will begin with an overview of the science of prediction, highlighting some recent developments in multivariate and actuarial methods of relevance to clinical medicine. Next, we will discuss some of the typical end points that clinicians seek to predict, along with optimal study designs. Next we address the ways in which treatment outcomes can be measured in psychiatry, where improvement can seem subjective and difficult to operationalize. After a review of some well-studied predictive factors, including diagnosis, comorbidity, and genetic and other biomarkers, we will conclude with a view to future directions for research and practice.
Prediction science
Prediction of treatment outcomes is a specific case of the broader and very vibrant field of prediction science.Citation6 Fundamentally, a prediction is a statement about the way things will be in the future. Good predictions are based on experience and data, but these can be noisy sources of information. The best predictions arise from statistical methods that fit precise data to valid models describing how the relevant variables contribute to the outcome. Experience tells us that an apple dropped from a tree will fall to the ground; Newtonian mechanics offer a model that makes use of the precise dropping height, gravitational constant, and mass to predict the force with which the apple will impact the ground.
This example highlights several challenges that make the prediction of treatment outcomes more complex than the prediction of an apple's descent: What are the relevant variables? How can they be measured with precision? What is the most valid model that fits the data? The impact of stochastic (chance-wise) variation must also be considered.Citation7 Stochastic variation makes it more difficult to predict outcome in a specific individual than it is to predict average outcomes of a group.
Consider the case of life insurance. Life insurance works since insurers use large sets of actuarial data to predict, with considerable accuracy, how long groups of individuals with particular lifestyles, health histories, and demographic characteristics will live and to set premium rates accordingly. In theory, similar principles apply to prediction in medicine, but amassing the required data in sufficient sample sizes can be challenging. Hie advent of electronic medical records may improve this situation,Citation8 especially in societies where medical care is organized in such a way that all outcomes can be tracked over time without significant attrition. On the other hand, prediction algorithms based on large samples do not help much in predicting an individual outcome, which is most important from the perspective of an individual patient. Insurance companies cannot predict exactly who will survive and who will not, only group outcomes. Similarly, standard medical approaches to predicting mortality generally perform modestly, at best.Citation9
In the best of worlds, predictions for a specific individual could be based on the right combination of clinical findings, biomarker measures, and genetic information. These data could be used to place a given patient in a subclass with well-studied outcomes. Breast cancer offers a good example, where we can already tailor treatment and make a good prognosis on the basis of family history, clinical staging, and expression of estrogen receptors by tumor cells.Citation10 Of course, the brain is a more complex organ than breast, liver, or kidney, but the same principles of prediction can be applied to psychiatric treatment outcomes.
Measurement of prediction in medicine
In medicine, the predictive value of a test depends on three main factors.Citation11 First, and most important, is the likelihood of the outcome of interest given the population from which the individual being tested is drawn, also known as the prior probability. If prior probability is low, even the best tests will return many false positives. Test sensitivity is another important factor, ex pressing how good the test is at detecting the outcome, or the probability of a positive test in someone who will show the outcome of interest. Test specificity expresses how good the test is at differentiating among possible outcomes, or the probability of a negative test in someone who will not show the outcome of interest.
Sensitivity and specificity tend to vary inversely, so the goal is often to find the test value that maximizes both. This can be expressed as the area under the curve (AUG) of a receiver operating curve (ROC), where sensitivity is plotted against specificity (Figure 1). Clinically useful tests usually have AUC values over 80%. In practice, however, it is often more useful to know the positive predictive value (PPV) of a test, which expresses the probability that a positive test correctly detects someone who will develop the outcome of interest:
PPV = true positives/(true positives + false positives)
Related concepts comprise measures that also attempt to address the clinical utility of a test: does the test provide unique information of sufficient impact to affect clinical decision-makingCitation14? One of the bestknown such measures is the number needed to screen (NNS), which estimates the number of patients who would need to be tested for every true positive detected.Citation15 There is no hard rule for NNS, since it may make more sense to screen a large number of people for a rare but serious outcome, while a less serious outcome might call for a smaller NNS.
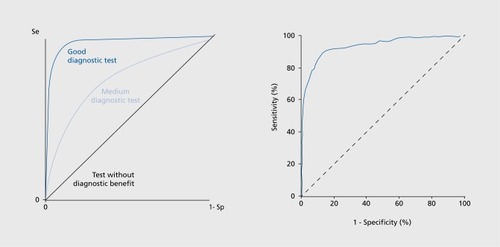
Typical end points
Treatment outcomes can cover a range of end points, including symptom reduction, recurrence, relapse, and adverse events. Symptom reduction is a key goal, but as an end point in psychiatry, it is often complicated by spontaneous remissions, placebo effects, and other factors beyond simple response to treatment. Major depression is a good example, where placebo effects are often very prominent.Citation16 Recurrence and relapse are usually distinguished by the duration (and completeness) of symptom reduction, both of which can be difficult to rate reliably, especially in retrospect. Adverse events are a less desirable end point, but can be quite important when they affect adherence—such as sexual dysfunction—or pose a serious health risk—such as agranulocytosis. Distinctive adverse events are often good targets for biomarker studies, although ascertainment of rare or delayed adverse events can be a challenge.
In designing a treatment outcomes study, it is important to consider the expected frequency of the outcome(s) of interest. Acute symptom reduction is best studied with a prospective, randomized, double-blind, repeated measures design.Citation17 Uncommon or rare adverse events cannot usually be studied in this way, since it would be impractical to prospectively follow the large group of patients needed to accumulate a sufficient sample of adverse outcomes. Such end points are better studied with retrospective case-control designs, where patients who have experienced the adverse outcome of interest are compared with those who did not.Citation18 The best case-control designs carefully match each case with one or more controls that address bias and potential confounders. Variables that cannot be matched in this way can often be handled through statistical tools such as regression, but the protection against confounding is not as secure.Citation19
Replication samples are always important, especially when a retrospective case-control design is employedCitation18 or when data-mining approaches are used to identify potential predictors. Failure to build in a sufficient replication sample can lead to biases such as overfilling, Winner's Curse, and false negative replication. Overfitting refers to the tendency to accumulate spurious predictors when multivariate models are fit to a single dataset.Citation20 The Winner's Curse, well-known in genetics studies, describes the overestimation of effect sizes in an initial sample, especially when many hypotheses are tested, as in a genome-wide association study.Citation21 Several good methods can correct for the Winner's Curse, but study of an independent sample is usually needed.Citation22,Citation23 False negative replication is the risk of failing to confirm a true finding when the replication sample was too small or too different from the initial sample to support a valid test of the null hypothesis.Citation24 To reduce this risk, replication samples should be larger than initial samples and should ideally be ascertained using identical methods.
Measurement of treatment outcomes
Treatment outcome research in psychiatry has a long history rooted in observational studies and randomized clinical trials. These kinds of studies have influenced the ways in which treatment outcomes are measured by developing widely used and highly reliable measures of symptoms and impairment.Citation25 Table I lists some examples of commonly used instruments. These instruments are often best-suited for acute treatment outcomes. Fewer well-validated instruments exist for the measurement of longer-term outcomes, especially for episodic disorders or those whose primary symptoms change over time. Rare adverse events that emerge after years of treatment are particularly difficult to detect and characterize.Citation26
Despite these limitations, it is now possible to measure treatment outcomes for most major psychiatric illnesses with a high degree of precision and reliability. There is a rich literature on measurement of mood symptoms,Citation27 psychotic symptoms,Citation28 anxiety,Citation29 and cognitive impairment.Citation30,Citation31 There are also good instruments for measuring symptom exacerbation,Citation25 episode recurrence, and relapse over time and their relationship to treatment interventions.Citation32-Citation34 Global measures of social or occupational impairment of have also been developed that offer a perspective on illness outcomes that crosses diagnostic boundaries.Citation35
Table I. Some commonly used measures of symptoms and impairment.
The best studied predictive factors
Diagnosis and clinical features
Considerable research, reaching back into the last century, has been focused on using diagnosis as a predictor of treatment outcome. Attempts have been made to delineate clinical forms of schizophrenia that are more responsive to antipsychotics,Citation36,Citation37 lithium-responsive forms of bipolar disorder,Citation38,Citation39 and treatment-resistant forms of depression.Citation40-Citation43
There has been some progress in this approach. For example, early age at onset is a consistent predictor of greater symptom severity in mood disorders, although not necessarily of treatment outcome.Citation43-Citation46 Symptom severity is another well-studied predictor, but its predictive value varies by diagnosis. Major depression is more responsive to medications when symptoms are severe.Citation47 In contrast, severe schizophrenia is less responsive to treatment.Citation48
The major limitation of predictors based on diagnosis and clinical features is dependence on clinical assessments that may be inaccurate, imprecise, or unstable over time. Diagnosis may also be too distal from underlying biological processes that presumably underlie various outcomes. New diagnostic systems such as the research domain criteria (RDoC) seek to address this problem by focussing on dimensions of observable behavior and neurobiological measures.Citation49
Based on this idea, much recent research has focused on cognitive symptoms in schizophrenia as prognostic indicators and as treatment targets in themselves.Citation50-Citation52 Widely used antipsychotics that control positive symptoms such as hallucinations do not treat—and may actually exacerbate—many of the deficits in working memory, social cognition, and executive functioning that contribute to the disability associated with schizophrenia.Citation53 Psychotherapeutic and pharmacologic interventions that effectively target these cognitive symptoms could thus contribute to better functional outcomes in patients suffering from schizophrenia and other psychotic disorders.
Treatment adherence
Treatment adherence is an obvious but sometimes overlooked factor that can play a major role in treatment outcome.Citation2 In research settings, treatment adherence can be monitored with blood levels, pill counts, or direct supervision. In clinical settings these measures may be difficult or impossible to implement. One consistent predictor of good treatment compliance is a subjective sense of positive regard for the treating clinician,Citation36,Citation54 which emphasizes the importance of the doctor-patient relationship in predicting treatment outcomes.
Treatment adherence is also related to adverse drug events. For example, in the STAR*D study of outpatients with major depression, reported adherence was lowest in those with the highest perceived side-effect burden.Citation54 A similar phenomenon was observed in the CATIE study of outpatients with schizophrenia.Citation28 These data suggest that efforts aimed at reducing perceived side effects could lead to better outcomes mediated by better treatment adherence.
Comorbidity
Among the general factors that affect treatment outcomes, comorbidity looms large, particularly in psychiatry. As a general rule, the more comorbidity, the poorer the outcome. This rule applies not only to psychiatric disorders, which are typically comorbid, but also to nonpsychiatric illnesses, especially chronic conditions such as heart disease,Citation55 kidney disease,Citation56 and diabetes.Citation57 Comorbid anxiety disorders are one well-known risk factor for poor treatment outcomes in major depression.Citation54,Citation58 Comorbid substance-use disorders (SUDs) are another major predictor of poor treatment outcomes. SUDs directly interfere with treatment response to antidepressants and anti-anxiety agents. SUDs are also associated with poorer treatment adherence, greater complaints of treatment-emergent adverse events, and more medical complications, all of which correlate with poorer outcomes.Citation59,Citation60
Genetic markers
All biomarkers represent correlations between an observation (test value) and an outcome. Correlation does not necessarily mean causation, since the observation may be the consequence of the outcome, rather than its cause (reverse causation), or may be correlated with some unobserved causal factor. Genetic markers can be especially useful as biomarkers since they are not subject to the reverse causation problem. The value of a genetic marker (ie, a basepair or set of basepairs in the inherited sequence of DNA) cannot reflect the result of a health outcome.
Genetic markers may still represent correlated, noncausal events, however. Genetic markers detected in a genome-wide association study (GWAS) are a good example. In most cases, the markers do not themselves represent a genetic variation with a direct biological impact, but are instead associated with one or more functional changes in the coding or regulatory sequence of a nearby gene. This fact also means that genetic markers emerging from GWAS are often difficult to follow up biologically, even when the markers themselves may reflect a valid association with a clinical outcome.
Occasionally, individual genetic markers may have predictive value for outcomes that reflect a major impact of a single gene, such as a Mendelian disease or a rare adverse event heavily influenced by only a few genes. Stevens-Johnson Syndrome in patients exposed to carbamazepine is a good example of this.Citation61 More often, the causal architecture of treatment outcomes is the result of a complex mixture of non-genetic factors and several different genes. Recent methods that take into account large sets of common genetic markers - hundreds to millions—show some promise for increased predictive value, but even in the best-case scenarios AUC values have tended to top out around 65%—too low for clinical utility.Citation62 More complex models that consider large sets of genetic markers along with clinical predictors could have greater predictive value,Citation63 but such models have so far been little studied.
There has been considerable interest recently in approaches that go beyond common genetic variation to encompass rare variants that may have a large impact on health in individuals. The personal genome movement represents a fresh opportunity to use genetic information in the prediction of individual treatment outcomes. Personal genome analysis uses extensive bioinformatic annotation of an individual's genome to generate probabilistic statements about disease risks and treatment outcomes.Citation64,Citation65 For this kind of analysis, sequence may focus on the portion of the genome transcribed into proteins—the “exome”—but whole genome sequence is best, since it represents noncoding and regulatory variation that may have an impact on disease.Citation66
From the “personal genomics” perspective, individual health outcomes are influenced uniquely by each person's total genetic endowment, most of which represents rare genetic variants that can only be discovered by high-throughput (whole exome or whole genome) sequencing.Citation64 While it is undeniable that each person is genetically unique, personal genomics needs to tackle some problems with inference before it can establish itself as a useful tool in medicine. If each person is unique, then how can we make predictions that are statistically valid?Citation67 How can we compare disease and treatment outcomes between groups? Can we practically amass the very large sample sizes needed to assess the impact of rare variation?Citation68 While there are still many methodological and ethical issues that will need to be addressed,Citation1 personal genome analysis will grow more and more powerful as the worldwide database of human genome sequence data and health outcomes grows.Citation69
Other biomarkers
Other biomarkers that are the subject of ongoing research include measures of gene expression,Citation70 neuroimaging measures,Citation71-Citation74 circulating inflammatory factors,Citation75 electroencephalographic measures,Citation76,Citation77 and metabolomics. Some interesting findings have also emerged in the field of proteomics, which go beyond measures of individual proteins to assess widespread patterns of protein expression.Citation78
Most of these approaches are severely limited by the inaccessibility of the brain in living patients. Even this barrier may fall in the future. New technologies such as induced pluripotent stem cells (iPSc) allow scientists to “reprogram” peripheral tissues (such as skin) to differentiate into neurons and glia.The cells express the same genome as the patient from whom they were collected, although epigenetic marks such as DNA methylation are lost. While challenging and expensive, iPSc methodology has already shown promise as a means of developing cellular models of mental illness that exhibit defects in neuronal development and synapse formation in cells derived from patients with schizophrenia.Citation79,Citation80 In the future, such models may be one way to design drug treatment regimens specifically tailored to an individual patient's genetics and cellular pathology.
Although promising, none of these biomarkers have been studied in sufficiently large samples to support firm conclusions. Integration of predictors across clinical and biological domains is another area that has so far been little explored,Citation40 but is of growing interest as the multifactorial nature of treatment outcome becomes increasingly clear.
Other predictive factors
Other factors that have been shown to influence treatment outcomes include socioeconomic status, race, and adverse life events.Citation81,Citation82 Since these variables tend to be correlated in many populations, it is difficult to disentangle causes from consequences. One approach takes advantage of genome-wide data to separate genetic ancestry from race, which can be especially useful in populations of mixed ancestry where genetics and race are not tightly correlated.Citation83 One big disadvantage of these kinds of variables is that they are often not considered modifiable risk factors for poor treatment outcomes, so will always have limited clinical utility.
Recent literature on response to a variety of psychotropic treatments has highlighted the importance of early symptomatic improvement. While the published studies vary in their definition of early response, several have suggested that a reduction in symptoms within the first week of drug exposure strongly predicts response after many weeks of therapy. In patients with unipolar major depression, sensitivities and specificities in the range of 80% have been reported.Citation84 For patients with bipolar depression, specificity is low, ie, lack of early improvement predicts poor response, but the presence of early improvement is unreliable.Citation85 Interestingly, this finding seems to hold both for pharmacological and psychotherapeutic (cognitive-behavioral) modalitiesCitation86 and has been linked to changes in brain-derived neurotropic factor (BDNF) in one study.Citation87 Similar results have been reported for mania, schizophrenia, and ADHD.Citation88-Citation90 These findings may contradict the established view that response to psychiatric treatments cannot be accurately gauged before several weeks. If supported in future studies, this observation could prove to be a valuable clinical predictor of response: patients who fail to show any response within the first week of treatment may benefit from a change.
The recent focus on genetics has also revived interest in the family history as a predictive tool.Citation91 Families can be uniquely informative since they provide a “natural laboratory” in which genetics and environment come together in a manner that is not seen in other groupings of people.Citation92 While everyone in a family is genetically distinct, relatives share substantial fractions of rare and common genetic variation in a context of shared environment and life experiences. Thus we should not be surprised that family history is still a much better predictor of common health and treatment outcomes than any available genetic tests.Citation93,Citation94 Just one example: family history is still the best predictor of response to lithium in bipolar disorder.Citation38,Citation95 This renewed interest in family history has also led to proposals for better, more efficient collection of family history data in research and clinical contexts.Citation96,Citation97
Summary and future directions
Prediction of treatment outcomes remains a significant challenge for psychiatry, as it does for the rest of medicine. While there has been considerable progress in recent years, major barriers to progress remain. Inadequate sample sizes are a significant problem, since psychiatric diagnostic categories remain quite heterogeneous, with little assurance that similarly diagnosed patients share similar etiological and pathophysiological factors. We still have a poor understanding of how events that can be described at the level of genes or neurons emerge as complex mental states characterized by disturbances in emotions, cognitions, and behaviors. And as in other fields of medicine, treatment outcomes will continue to be influenced by individual choices and disparities in social, economic, and educational opportunities that are largely beyond the control of clinicians.
We now have a solid foundation in prediction science, outcomes measurement, and genomics on which future research can build. The best predictions will need to take into account clinical as well as biomarker data. Genetic markers show special promise in this regard, but we need more research into the clinical utility of genetic information in individual treatment decisions. Integrative approaches that like the family history can jointly model genetic and environmental influences stand the best chance for generating clinically relevant predictions.
How far can we go in predicting treatment outcomes in psychiatry? As data accumulate, we should be able to move well beyond the largely trial-and-error approach most psychiatrists still rely on. Even the best predictions are ultimately limited by the great extent of human variation, without which, to paraphrase William Osier, medicine would be more of a science and less of an art.
REFERENCES
- McMahonFJ.InselTR.Pharmacogenomics and personalized medicine in neuropsychiatry.Neuron.20127477377622681682
- VelliganDl.WeidenPJ.SajatovicM.et alStrategies for addressing adherence problems in patintserious and persistent mental illness: recommendations from the expert consensus guidelines.J Psychiatr Pract.20101630632420859108
- AuffrayC.HoodL.Editorial: Systems biology and personalized medicine - the future is now.Biotechnol J.2012793893922887882
- DrozdaK.MullerDJ.BishopJR.Pharmacogenomic testing for neuropsychiatry drugs: current status of drug labeling, guidelines for using genetic information, and test options.Pharmacotherapy.2014341668424523097
- HoeheMR.KroslakT.Genetic variation and pharmacogenomics: concepts, facts, and challenges.Dialogues Clin Neurosci.2004652522033504
- WacholderS.Population risk measures.Epidemiol.200516 594
- HardyJ.Genetic analysis of pathways to Parkinson disease.Neuron.20106820120620955928
- GottesmanO.KuivaniemiH.TrompG.et alThe Electronic Medical Records and Genomics (eMERGE) Network: past, present, and future.Genet Med.20131576177123743551
- SiontisGCM.TzoulakiI.loannidisJPA.Predicting death: an empirical evaluation of predictive tools for mortality.Arch Intern Med.20111711721172621788535
- FreedmanRA.WinerEP.Adjuvant therapy for postmenopausal women with endocrine-sensitive breast cancer.Breast.201019697520034796
- Ferrantedi Ruffano L.HydeCJ.McCafferyKJ.BossuytPMM.DeeksJJ.Assessing the value of diagnostic tests: a framework for designing and evaluating trials.BMJ.2012344 e686
- Receiver Operating Characteristic Curve. Available at: http://www. adscience.eu/uploads/ckfiles/files/html_files/StatEL/statel_ROC_ curve, htm. Accessed October 2014
- AldousSJ.RichardsM.CullenL.TroughtonR.ThanM.Diagnostic and prognostic utility of early measurement with high-sensitivity troponin T assay in patients presenting with chest pain.Can Med Assoc J.2012184e260e26822291171
- WacholderS.Clinical utility in evaluation of risk models.Am J Epidemiol.201217649549622875758
- RemboldCM.Number needed to screen: development of a statistic for disease screening.BMJ.19983173073129685274
- WalshBT.SeidmanSN.SyskoR.GouldM.Placebo response in studies of major depression: variable, substantial, and growing.JAMA.20022871840184711939870
- Jane-witD.HorwitzRl.ConcatoJ.Variation in results from randomized, controlled trials: stochastic or systematic?J Clin Epidemiol.201063566319740624
- WacholderS.Design issues in case-control studies.Stat Methods Med Res.199542933098745128
- WacholderS.Bias in full cohort and nested case-control studies?Epidemiol.200920339340
- TzoulakiI.LiberopoulosG.loannidisJPA.Assessment of claims of improved prediction beyond the Framingham risk score.JAMA.20093022345235219952321
- KraftP.Curses-winner's and otherwise-in genetic epidemiology.Epidemiol.200819649651
- XiaoR.BoehnkeM.Quantifying and correcting for the winner's curse in genetic association studies.Genet Epidemiol.20093345346219140131
- ZollnerS.PritchardJK.Overcoming the winner's curse: estimating penetrance parameters from case-control data.Am J Hum Genet.20078060561517357068
- loannidisJPA.To replicate or not to replicate: the case of pharmacogenetic studies: have pharmacogenomics failed, or do they just need larger-scale evidence and more replication?Ore Cardiovasc Genet.20136413418
- CohenRM.GreenbergJM.IsHakWW.Incorporating multidimensional patient-reported outcomes of symptom severity, functioning, and quality of life in the Individual Burden of Illness Index for Depression to measure treatment impact and recovery in MDD.JAMA Psychiatry.20137034335023303512
- KatzAJ.DusetzinaSB.FarleyJF.et alDistressing adverse events after antidepressant switch in the Sequenced Treatment Alternatives to Relieve Depression (STAR*D) trial: influence of adverse events during initial treatment with citalopram on development of subsequent adverse events with an alternative antidepressant.Pharmacotherapy.20123223424322392456
- FavaM.RushAJ.TrivediMH.et alBackground and rationale for the sequenced treatment alternatives to relieve depression (STAR*D) study.Psychiatr Clin North Am.20032645749412778843
- StroupTS.McEvoyJP.SwartzMS.et alThe National Institute of Mental Health Clinical Antipsychotic Trials of Intervention Effectiveness (CATIE) project: schizophrenia trial design and protocol development.Schizophr Bull.200329153112908658
- DeckersbachT.PetersAT.SylviaL.et alDo comorbid anxiety disorders moderate the effects of psychotherapy for bipolar disorder? Results from STEP-BD.Am J Psychiatry.201417117818624077657
- FolsteinMF.FolsteinSE.McHughPR.“Mini-mental state”: A practical method for grading the cognitive state of patients for the clinician.J Psychiatr Res.1975121891981202204
- NortonJC.The Trail Making Test and Bender Background Interference Procedure as screening devices.J Clin Psychol.197834916922711883
- EisnerE.DrakeR.BarrowcloughC.Assessing early signs of relapse in psychosis: review and future directions.Clin Psychol Rev.20133363765323628908
- IshakWW.GreenbergJM.CohenRM.Predicting relapse in major depressive disorder using patient-reported outcomes of depressive symptom severity, functioning, and quality of life in the Individual Burden of Illness Index for Depression (IBI-D).J Affect Disord.2013151596523790554
- GrofP.DuffyA.CavazzoniP.et alIs response to prophylactic lithium a familial trait?J Clin Psychiatry.20026394294712416605
- McGrathLM.CornellsMC.LeePH.et alGenetic predictors of risk and resilience in psychiatric disorders: a cross-disorder genome-wide association study of functional impairment in major depressive disorder, bipolar disorder, and schizophrenia.Am J Med Genet Part B Neuropsychiatr Genet.2013162B779788
- SuzukiT.UchidaH.WatanabeK.KashimaH.Factors associated with response to clozapine in schizophrenia: a review.Psychopharmacol Bull.201144326022506438
- AwadAG.Drug therapy in schizophrenia-variability of outcome and prediction of response.Can J Psychiatry.1989347117202572318
- KleindienstN.EngelR.GreilW.Which clinical factors predict response to prophylactic lithium? A systematic review for bipolar disorders.Bipolar Disord.2005740441716176433
- PeturssonH.Prediction of lithium response.Compr Psychiatry.197920226241376222
- KempAH.GordonE.RushAJ.WilliamsLM.Improving the prediction of treatment response in depression: integration of clinical, cognitive, psychophysiological, neuroimaging, and genetic measures.CNS Spectr.2008131066108619179943
- BennabiD.VandelP.PapaxanthisC.PozzoT.HaffenE.Psychomotor retardation in depression: a systematic review of diagnostic, pathophysiologic, and therapeutic implications.BioMed Res Int.20132013158746
- PerlisRH.UherR.OstacherM.et alAssociation between bipolar spectrum features and treatment outcomes in outpatients with major depressive disorder.Arch Gen Psychiatry.20116835136021135313
- SungSC.WisniewskiSR.BalasubramaniGK.et alDoes early-onset chronic or recurrent major depression impact outcomes with antidepressant medications? A CO-MED trial report.Psychol Med.20134394596023228340
- KozelFA.TrivediMH.WisniewskiSR.et alTreatment outcomes for older depressed patients with earlier versus late onset of first depressive episode: a Sequenced Treatment Alternatives to Relieve Depression (STAR*D) report.Am J Geriatr Psychiatry.200816586418165462
- PerlisRH.DennehyEB.MiklowitzDJ.et alRetrospective age at onset of bipolar disorder and outcome during two-year follow-up: results from the STEP-BD study.Bipolar Disord.20091139140019500092
- ZisookS.LesserI.StewartJW.et alEffect of age at onset on the course of major depressive disorder.Am J Psychiatry.20071641539154617898345
- FournierJC.DeRubeisRJ.HollonSD.et alAntidepressant drug effects and depression severity: a patient-level meta-analysis.JAMA.2010303475320051569
- KaneJM.CorrellCU.Pharmacologic treatment of schizophrenia.Dialogues Clin Neurosci.20101234535720954430
- CuthbertBN.KozakMJ.Constructing constructs for psychopathology: the NIMH research domain criteria.J Abnorm Psychol.201312292893724016027
- HaganJJ.JonesDNC.Predicting drug efficacy for cognitive deficits in schizophrenia.Schizophr Bull.20053183085316177277
- HarveyPD.BowieCR.Cognitive enhancement in schizophrenia: pharmacological and cognitive remediation approaches.Psychiatr Clin North Am.20123568369822929873
- SarfatiY.Hardy-BayleM-C.Could cognitive vulnerability identify high-risk subjects for schizophrenia?Am J Med Genet.200211489389712457382
- MeltzerHY.McGurkSR.The effects of clozapine, risperidone, and olanzapine on cognitive function in schizophrenia.Schizophr Bull.19992523325510416729
- MurphyEJ.KassemL.ChemerinskiA.RushAJ.LajeG.McMahonFJ.Retention and attrition among African Americans in the STAR*D study: what causes research volunteers to stay or stray?Depress Anxiety.2013301137114423723044
- KerberKB.WisniewskiSR.LutherJF.et alEffects of heart disease on depression treatment: results from the COMED study.Gen Hosp Psychiatry.201234243422001552
- HedayatiSS.MinhajuddinAT.AfsharM.TotoRD.TrivediMH.RushAJ.Association between major depressive episodes in patients with chronic kidney disease and initiation of dialysis, hospitalization, or death.JAMA.20103031946195320483971
- BryanC.SongerT.BrooksMM.et alThe impact of diabetes on depression treatment outcomes.Gen Hosp Psychiatry.201032334120114126
- GollanJK.FavaM.KurianB.et alWhat are the clinical implications of new onset or worsening anxiety during the first two weeks of SSRI treatment for depression?Depress Anxiety.2012299410122147631
- DavisLL.PilkintonP.WisniewskiSR.et alEffect of concurrent substance use disorder on the effectiveness of single and combination antidepressant medications for the treatment of major depression: an exploratory analysis of a single-blind randomized trial.Depress Anxiety.20122911112222495941
- DavisLL.WisniewskiSR.HowlandRH.et alDoes comorbid substance use disorder impair recovery from major depression with SSRI treatment? An analysis of the STAR*D level one treatment outcomes.Drug Alcohol Depend.201010716117019945804
- LiXYuK.MeiSet alHLA-B*1502 increases the risk of phenytoin or lamotrigine induced Stevens-Johnson Syndrome/toxic epidermal necrolysis: Evidence from a meta-analysis of nine case-control studies.Drug Res Stuttg.2014 epub May 28 2014
- GrattenJ.WrayNR.KellerMC.VisscherPM.Large-scale genomics unveils the genetic architecture of psychiatric disorders.Nat Neurosci.20141778279024866044
- Le-NiculescuH.PatelSD.BhatM.et alConvergent functional genomics of genome-wide association data for bipolar disorder: comprehensive identification of candidate genes, pathways and mechanisms.Am J Med Genet Part B Neuropsychiatr Genet.2009150B155181
- Li-Pook-ThanJ.SnyderM.iPOP goes the world: integrated personalized Omics profiling and the road toward improved health care.Chem Biol.20132066066623706632
- HoodL.TianQ.Systems approaches to biology and disease enable translational systems medicine.Genomics Proteomics Bioinformatics.20121018118523084773
- BoyleAP.HongEL.HariharanM.et alAnnotation of functional variation in personal genomes using RegulomeDB.Genome Res.2012221790179722955989
- JanssensACJW.loannidisJPA.van DuijnCM.LittleJ.KhouryMJ.Strengthening the reporting of genetic risk prediction studies: the GRIPS statement.Genet Med.20111345345621502867
- loannidisJPA.Personalized genetic prediction: too limited, too expensive, or too soon?Ann Intern Med.200915013914119153414
- GreshakeB.BayerPE.RauschH.RedaJ.openSNP-a crowdsourced web resource for personal genomics.PLoS One.20149e8920424647222
- GaoY.GalanteM.El-MallakhJ.et alBDNF expression in lymphoblastoid cell lines carrying BDNF SNPs associated with bipolar disorder.Psychiatr Genet.20122225325522517381
- EvansKC.DoughertyDD.PollackMH.RauchSL.Using neuroimaging to predict treatment response in mood and anxiety disorders.Ann Clin Psychiatry.200618334216517451
- FuCHY.CostafredaSG.Neuroimaging-based biomarkers in psychiatry: clinical opportunities of a paradigm shift.Can J Psychiatry.20135849950824099497
- MacQueenGM.Magnetic resonance imaging and prediction of outcome in patients with major depressive disorder.J Psychiatry Neurosci.20093434334919721844
- KozelFA.RaoU.LuH.et alFunctional connectivity of brain structures correlates with treatment outcome in major depressive disorder.Front Psychiatry.20112721556277
- TomasikJ.RahmouneH.GuestPC.BahnS.Neuroimmune biomarkers in schizophrenia.Schizophr Res.2014 epub 12 August
- JaworskaN.ProtznerA.Electrocortical features of depression and their clinical utility in assessing antidepressant treatment outcome.Can J Psychiatry Rev Can Psychiatr.201358509514
- CookIA.HunterAM.GilmerWS.et alQuantitative electroencephalogram biomarkers for predicting likelihood and speed of achieving sustained remission in major depression: a report from the Biomarkers for Rapid Identification of Treatment Effectiveness in Major Depression (BRITE-MD) trial. JClin Psychiatry.2013745156
- GuestPC.Martins-de-SouzaD.SchwarzE.et alProteomic profiling in schizophrenia: enabling stratification for more effective treatment. Genome Med.2013525
- BrennandKJ.SimoneA.JouJ.et alModelling schizophrenia using human induced pluripotent stem cells.Nature.201147322122521490598
- WenZ.NguyenHN.GuoZ.et alSynaptic dysregulation in a human iPS cell model of mental disorders.Nature.201451541441825132547
- TrivediMH.Modeling predictors, moderators and mediators of treatment outcome and resistance in depression.Biol Psychiatry.2013742423787333
- LeuchterAF.CookIA.MarangellLB.et alComparative effectiveness of biomarkers and clinical indicators for predicting outcomes of SSRI treatment in major depressive disorder: results of the BRITE-MD study.Psychiatry Res.2009 16912413119712979
- MurphyE.HouL.MaherBS.et alRace, genetic ancestry and response to antidepressant treatment for major depression.Neuropsychopharmacol.20133825982606
- TomitaT.Yasui-FurukoriN.SatoY.et alSex differences in the prediction of the effectiveness of paroxetine for patients with major depressive disorder identified using a receiver operating characteristic curve analysis for early response.Neuropsychiatr Dis Treat.20141059960624748795
- KempDE.GanocySJ.BrecherM.et alClinical value of early partial symptomatic improvement in the prediction of response and remission during short-term treatment trials in 3369 subjects with bipolar I or II depression.J Affect Disord.201113017117921071096
- LewisCC.SimonsAD.KimHK.The role of early symptom trajectories and pretreatment variables in predicting treatment response to cognitive behavioral therapy.J Consult Clin Psychol.20128052553422730951
- DreimullerN.SchlichtKF.WagnerS.et alEarly reactions of brainderived neurotrophic factor in plasma (pBDNF) and outcome to acute antidepressant treatment in patients with Major Depression.Neuropharmacology.20126226426921803060
- CorrellCU.ZhaoJ.CarsonW.et alEarly antipsychotic response to aripiprazole in adolescents with schizophrenia: predictive value for clinicaloutcomes. J Am Acad Child Adolesc Psychiatry.201352689698
- Stentebjerg-OlesenM.JeppesenP.PagsbergAK.et alEarly nonresponse determined by the clinical global impressions scale predicts poorer outcomes in youth with schizophrenia spectrum disorders naturalistically treated with second-generation antipsychotics.J Child Adolesc Psychopharmacol.20132366567524266529
- BuitelaarJK.Van derGaag RJ.Swaab-BarneveldH.KuiperM.Prediction of clinical response to methylphenidate in children with attention-deficit hyperactivity disorder.J Am Acad Child Adolesc Psychiatry.199534102510327665441
- PyeritzRE.The family history: the first genetic test, and still useful after all those years?Genet Med.2012143922237427
- BergAO.BairdMA.BotkinJR.et alNational Institutes of Health State-of-the-Science Conference Statement: Family History and Improving Health.Ann Intern Med.200915187287719884615
- DoCB.HindsDA.FranckeU.ErikssonN.Comparison of family history and SNPs for predicting risk of complex disease.PLoS Genet.20128e100297323071447
- WilsonBJ.QureshiN.SantaguidaP.et alSystematic review: family history in risk assessment for common diseases.Ann Intern Med.200915187888519884616
- AnanthJ.PecknoldJC.Prediction of lithium response in affective disorders.J Clin Psychiatry.19783995100344305
- WattendorfDJ.HadleyDW.Family history: the three-generation pedigree.Am Fam Physician.20057244144816100858
- KendlerKS.Family history information in biomedical research.J Contin Educ Health Prof.20012121522311803766