Abstract
Background : There is a lack of reliable data in developing countries to inform policy and optimise resource allocation. Health and socio-demographic surveillance sites (HDSS) have the potential to address this gap. Mortality levels and trends have previously been documented in rural South Africa. However, complex space–time clustering of mortality, determinants, and their impact has not been fully examined.
Objectives : To integrate advanced methods enhance the understanding of the dynamics of mortality in space–time, to identify mortality risk factors and population attributable impact, to relate disparities in risk factor distributions to spatial mortality risk, and thus, to improve policy planning and resource allocation.
Methods : Agincourt HDSS supplied data for the period 1992–2008. Advanced spatial techniques were used to identify significant age-specific mortality ‘hotspots’ in space–time. Multivariable Bayesian models were used to assess the effects of the most significant covariates on mortality. Disparities in risk factor profiles in identified hotspots were assessed.
Results : Increasing HIV-related mortality and a subsequent decrease possibly attributable to antiretroviral therapy introduction are evident in this rural population. Distinct space–time clustering and variation (even in a small geographic area) of mortality were observed. Several known and novel risk factors were identified, and population impact was quantified. Significant differences in the risk factor profiles of the identified ‘hotspots’ included ethnicity; maternal, partner, and household deaths; household head demographics; migrancy; education; and poverty.
Conclusions : A complex interaction of highly attributable multilevel factors continues to demonstrate differential space–time influences on mortality risk (especially for HIV). High-risk households and villages displayed differential risk factor profiles. This integrated approach could prove valuable to decision makers. Tailored interventions for specific child and adult high-risk mortality areas are needed, such as preventing vertical transmission, ensuring maternal survival, and improving water and sanitation infrastructure. This framework can be applied in other settings within the region.
Appendices available online under Reading Tools.
Reliable mortality data are a prerequisite for planning health interventions, yet such data are often not available or reliable in developing countries, including those in sub-Saharan Africa Citation3. In many instances, health and socio-demographic surveillance systems (HDSS), though not representative at the national level, are often the only means to assess and more clearly understand population levels, trends, and determinants on a prospective basis Citation4 Citation5.
Recent advances in data availability and analytic methods have created new opportunities to improve the analysis and modelling of diseases on a local, national, or regional basis Citation6 Citation7. Spatial analysis and, for example, Bayesian geostatistical modelling are powerful and statistically robust tools for identifying high-mortality areas in a heterogeneous and imperfectly known environment and associated determinants Citation6 Citation8. An increasing body of literature on spatial analysis of health outcomes in developing countries has been motivated by the availability of geo-referenced data and by the recent advances in methods and software that can implement such complex models Citation7 Citation9. The identification of geographical clusters of high-risk mortality is an important policy issue that has received limited attention, especially the ability to identify individuals, households, and villages at elevated risk. This study contributes to other literature that investigates mortality and its risk factors that are important from a public health perspective Citation10. The study also provides guidance regarding the distribution of health services and other spatially-targeted interventions for disease control, mortality reduction, and resource allocation in rural South Africa and has application to broader sub-Saharan Africa.
Addressing health inequities in populations is a major challenge Citation11, and research that documents and quantifies inequities is needed to inform policies to close health gaps in the developing world. Evidence on reducing inequities within countries is growing. Successful approaches include those that improve geographic access to health interventions in poor communities, subsidize health care and health inputs for the poor, and empower poorer communities Citation12.
This study aims to describe and develop a framework that captures the space–time dynamics and determinants of age-specific mortality in rural South Africa.
Methods
Study area and population
The Agincourt HDSS is located in a sub-district in north-east South Africa (). There was a baseline census in 1992 that collected data on all individuals and households in the population Citation13. This has been followed by annual updates of births, deaths, and in- and out-migrations. It is a poor rural sub-district that includes former Mozambican refugees, temporary migrant workers, and a more stable permanent population Citation13. The site at present covers an area of about 400 km2 and contains 25 villages, 13,500 households, and 84,000 individuals. There is a full geographic information system (GIS), containing locations of all households within the site, which is updated annually. A household is defined as a group of people who reside and eat together, plus the linked temporary migrants who would eat with them on return. Verbal autopsies (VAs), a method of determining individuals’ causes of death in populations without a complete vital registration system, were introduced in 1993. A full VA is conducted on every death recorded during the annual census update and is administered to the closest caregiver of the deceased by a trained fieldworker Citation14. Three medical practitioners assess Vas to determine likely cause of death. Causes of death (main, immediate, and/or contributing) are coded to be consistent with the International Classification of Diseases (ICD-10). The main cause of death was used in these analyses.
Fig. 1. Maps showing the location of the Agincourt HDSS Citation41.
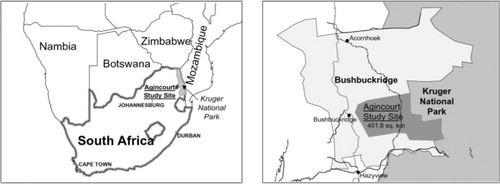
The study population comprised infants (<1 year), children (1–4 years), young adults (15–49 years), and older adults (50–64 years) in the original 21 villages for the period 1992–2008. Children aged 5–14 were not included in the more detailed analyses given their very low mortality rate (<1 death per 1,000 person years) and corresponding absolute number of deaths. Data from four new villages added to the site since 2007 were also not included in the analysis as they contributed minimal data to the study period.
Outcome and explanatory variables
The dichotomous age-specific mortality outcomes were defined as follows:
Infant – mortality within the first 365.25 days of life
Children – mortality between 1 and 4 years of age
Young to middle-age adults – mortality between 15 and 49 years of age
Older adults – mortality between 50 and 64 years of age
Risk factor analysis
A preliminary bivariate risk factor analysis was conducted to assess the relationship between mortality and each covariate. Covariates significant at the 10% level were then incorporated into the multivariable model. Given the inherent spatial and temporal correlation of longitudinal HDSS data, problems arise when using standard statistical methods as they assume independence of outcome measures (e.g. mortality). Objects in close proximity are often more alike, and common exposures (measured or unmeasured) may influence adult mortality similarly in households of the same geographical area, introducing spatial correlation in mortality outcomes. Including the spatial effect of proximity is important for an efficient estimation of parameters and prediction Citation16. Ignoring this correlation introduces bias in the risk factor analysis as the standard error of the covariates is underestimated, thereby overestimating the significance of the risk factors. Geostatistical models relax the assumption of independence and assume that spatial correlation is a function of distance between locations. They are highly parameterised models, and their full estimation has only become possible in the last decade by formulating them within a Bayesian framework Citation17 and estimating the parameters via Markov chain Monte Carlo (MCMC) simulation. With the development of MCMC methods and software such as WinBUGS Citation18, Bayesian approaches are being applied to the analysis of many social and health problems in addition to disease mapping and modelling or kriging Citation19. Thus, Bayesian geostatistical multivariable models are needed to analyse longitudinal data in order to address these problems.
Different analytical dataset structures were used for the various age groups. This dictated the corresponding modelling approach to examine the multivariable association between the significant covariates and age-specific mortality. For infants, a negative binomial model (selected due to over dispersion) was used with an offset of time in days contributed in the first year given their higher risk earlier on. For children (1–4 years), a monthly discrete time logistic or event history approach was used to track any changes of selected covariates in the given intervals. A monthly time interval was used as it was a better approximation of the risk than using a yearly interval. For the adult models, a continuous time to event or survival approach (Weibull parametric model) was adopted that split episodes of time for any relevant changes in selected covariates, for example, change of location or household. For a detailed comparison of the strengths and weaknesses of each analytical approach, please see Appendix 1. Details of the infant and child statistical models and selected results have been published previously Citation20 Citation21. However, the infant results in this paper include important additional variables (mother death due to HIV or non-HIV, breastfeeding) not used previously. A spatial random effect at the village level was included to take account of spatial correlation and was modelled using a multivariate Gaussian distribution with a covariance matrix expressed as a parametric function of the distance between pairs of village centroids points Citation17. Furthermore, an unstructured household-level random effect was included to take into account repeated household observations where time episodes were split to incorporate any time varying issues such as change of household physical location. MCMC simulation Citation22 was employed to estimate the model parameters. Detailed formulation of the models as well as the WinBUGS codes to implement each can be found in Appendices 2 and 3, respectively.
Model assessment
Model comparison in STATA was based on the Akaike information criterion (AIC). The deviance information criterion (DIC) was used to assess the various Bayesian multivariable models Citation23. Both the AIC/DIC are a measure of the relative goodness of fit of a statistical model. Generally, the smaller the AIC/DIC, the better the model fit.
Spatial analysis
The Kulldorff spatial scan statistic Citation24 was used to identify significant spatial clustering of mortality. Simulation-based Bayesian Poisson kriging Citation25 was also used to produce smoothed maps of all-cause mortality risk within the whole HDSS area. All-cause and cause-specific baseline models used included a constant and spatial random effect only. All identifying features (such as village centroids, boundaries) have been removed from the maps to ensure confidentiality and avoid stigmatisation of potentially high-risk villages. The HIV and tuberculosis mortality risk map is also not shown for the abovementioned reason. Model spatial estimates were exponentiated to give relative risk (RR). Risk maps were developed using a heat scale of the location specific RR prediction. Darker areas reflect increasingly higher RR, while increasingly lighter areas indicate lowering RR. A simple map showing potentially high-risk areas as a function of straight-line distance to nearest health facility was constructed using a circular buffer zone around health facilities based on significant cut-off found in the risk factor analysis.
Software
Data were extracted from the Agincourt database using Microsoft SQL Server. The analysis was carried out in STATA version 10.0 SE Citation26 and WinBUGS Citation18. Risk maps were constructed in MapInfo Professional version 9.5.
Results
Demographic and mortality profile
The demographic and mortality profile of the study samples are provided in . Overall 9,035 deaths occurred during 1992–2008, based on 1,110,166 person-year time contributed, at an overall crude mortality rate of 8.1 per 1,000 person-years. The highest mortality rates occurred among infants followed by the older adult (50–64 years) age group (29 and 19 per 1,000 person-years, respectively). The mortality rate among children and younger adults (15–49 years) was similar at 5.7 and 6.9 per 1,000 person-years, respectively. Among infants 216 deaths occurred during the perinatalFootnote1 period and 251 in the neonatalFootnote2 period, that is, the majority occurred in the perinatal or early neonatal phase. The overall perinatal and neonatal mortality rates were 7.6 and 8.8 per 1,000 person-years, respectively. The lowest mortality rates were observed in the 5–14 year age group. Among adults (15–64 years), mortality rates showed a steady increase by 5-year grouping with a non-linear excess in the 30–34 and 35–39 age groups due to HIV/AIDS.
Table 1. Demographic profile of study sample by age group, 1992–2008
Leading causes of death by age group, 1992–2008
The leading cause-of-death in all age groups () was HIV/TB. Among children, the second most prominent cause-of-death was diarrhoea or malnutrition. Among younger adults (15–49 years), external cases of death, namely assault and transport accidents, featured as the second and third top causes-of-death, with lifestyle-related diseases following. In the older adult age group (50–64 years) following HIV/TB, chronic non-communicable diseases featured prominently.
Table 2. Top five causes-of-death by age group, 1992–2008
Temporal trends in age-specific mortality
A significant increase in all age group mortality rates was observed over the study period (), especially due to the impact of the HIV epidemic from the late 1990s to mid-2000s. All-cause mortality began to plateau around 2004 following rollout of the antiretroviral therapy (ART) programme in 2003 and reduction of HIV-related mortality. The temporal trend terms included in each multivariable model confirmed the significant increase in mortality across all age-groups over the study period both linearly and by period ().
Table 3. All-cause multivariable risk factor analyses for age-specific mortality using Bayesian modelling
Major risk factors for age-specific mortality
Infants: maternal death in the infant's first year (especially due to HIV/TB), higher number of cumulative household deaths, no breastfeeding, and previous birth interval less than 1 year emerged as highly significant risk factors for all-cause infant mortality (). Mother being a migrant remained significantly protective. Male gender, increasing parity, and death of previous child were no longer significant risk factors following multivariable adjustment. No significant association was observed between infant mortality and household SES, increasing distance to nearest health facility and climate (using elevation as a proxy which corresponds to the rainfall gradient in the sub-district).
Children: maternal death between the child's first and fifth birthdays, particularly due to HIV/TB, was the most prominent risk factor from the multivariable analysis (), followed by father death due HIV/TB, four or more children aged less than 5 years living within the household, Mozambican origin of the mother, and winter season. Increasing age of the child remained highly protective. No significant association was observed between mortality risk and increased distance to nearest health facility. In contrast to infants, however, a significant and increasing trend of protective association was observed with increasing household SES based on the bivariate analysis.
Adults: The most prominent risks for 15–49 year mortality following multivariable adjustment were male gender, being a migrant, increasing number of other household deaths, household head death, and distance to nearest health facility (>6 km) (). Increasing wealth of household, household head being male, and older than 40 years were significant and prominent protective factors. Villages with a mortality proportion of HIV/TB above the median value remained at a significantly higher risk. Mozambican ethnicity and education were no longer significant after multivariable adjustment.
The most prominent risks for 50–64 year mortality following multivariable adjustment were male gender, being a migrant, and death of household head (). Households headed by older males again reduced older adult mortality risk. Mozambicans appeared to have significantly lower risk in this age group when compared to South Africans. In contrast to the findings for younger adults, following multivariable adjustment in the 50–64 year model, distance to nearest health facility (>6 km) and household SES were no longer significant risk factors.
Based on the risk prediction for straight-line distance to health facility, we can see that two villages in particular, one in the upper and the other in the lower south-east region, appear to have a higher mortality risk as a function of increased distance to the nearest local clinic in the Agincourt sub-district (). We also observe that there are other villages that appear to be far from the nearest health facility.
Spatial distribution of age-specific mortality
Spatial risk estimates based on a Bayesian kriging model suggest a higher risk of infant mortality on the eastern border of the site while child mortality was concentred in two distinct foci: upper central and south-east corner of the sub-district. Five distinct foci of higher mortality in the 15–49 year age-group were observed using Bayesian kriging (). Three are in the central to upper central region of the site and two in the south east. These correlate to areas with higher risk of infectious disease mortality in this age group, largely HIV/TB. A very similar pattern was seen in the 50–64 year age-group when compared to 15–49 years though with one minor difference in that one village in the south-east was no longer at higher risk and one additional village in the upper central region emerged as high risk. Similarly, this distribution is largely driven by HIV/TB mortality. Higher non-communicable disease mortality risk was observed in one particular village in the upper central region of the site.
Potential proximate reasons for the observed high-risk clusters
The high age-specific mortality clusters (Kulldorff spatial cluster scan statistic p<0.05), when compared to the lower mortality clusters, had significantly (p<0.05):
more deaths due to HIV/TB and diarrhoea or malnutrition
lower duration of breastfeeding
higher number of deaths of previous children
higher number of mothers dying of HIV/TB
lower mean maternal education years
higher number of cumulative household deaths
younger household heads
higher incidence of household heads dying
more Mozambican household heads
lower SES
higher temporary migration rates
lower proportion of individuals with secondary or higher level education.
Discussion
This study has demonstrated the usefulness of advanced epidemiological modelling in assessing risk factors and producing smooth maps of mortality risk in a population.
Earlier work in South Africa and Agincourt has shown the profound impact of HIV/TB on mortality across most age groups Citation27–Citation29, with higher than normal mortality rates evident. The results show that infectious diseases (particularly HIV/TB) were the most prominent cause-of-death over the study period and have largely contributed to the observed mortality trends. The levelling out of mortality round 2005 is possibly linked to the ART rollout, which began in South Africa round 2004. Current studies in this area are assessing the impact of ART rollout on mortality, as well as specific villages or areas where equity of access may be an issue. Mortality from non-communicable disease has also increased significantly in adults 30 years and older in the rural Agincourt sub-district Citation29 and has implications with regard to the epidemiological transition.
Results suggest that strong and significant space–time mortality disparities exist, even within a small geographic area. This distribution is being driven by a complex web of multilevel interacting factors that have likely increased communicable disease mortality (HIV) and non-communicable disease mortality (in the older age-group) in specific risk areas. According to the spatial analyses, the south-east and upper central regions of the site were consistently identified as high-risk areas for most age groups, thus indicating a definite non-random element to the mortality distribution in this rural sub-district. A strong geographical pattern of higher infectious disease mortality risk (particularly HIV/TB and diarrhoea/malnutrition) and former Mozambican settlements lying to the east of the site was also generally observed. Mozambican settlements in the south-east have generally been shown to have poorer access to water, sanitation, and waste disposal; in addition, they had fewer schools and poor quality of housing and were particularly isolated from public transport Citation30 Citation31.
Key individual and household level determinants have been confirmed and certain novel determinants have emerged. The results confirmed the importance of infant and child mortality risk factors such as maternal age, birth spacing, season, village and ethnic group Citation32 Citation33. Lack of breastfeeding in infancy and maternal death during infancy or childhood Citation1–Citation4 were major risk factors as were a higher number of cumulative household deaths. Other reports indicate that infants who survive the death of the mother have a 10% chance or less of living past the age of 1 year Citation34. A complex interaction of higher HIV prevalence, large distance from nearest health facility, low SES, low education, high household mortality burden, and high migrancy rates (increased risk behaviour) appear to be driving communicable disease mortality, particularly HIV. Low SES and few employment opportunities locally have led to adults migrating externally for work. Migration patterns have been shown to influence HIV risk Citation35 Citation36, and temporary or labour migrants are more vulnerable to HIV than more settled populations. This has been shown in other African and southern African countries Citation37. One village in the upper central region appears to be at consistently higher risk across all age groups and has a significantly younger and highly mobile population, potentially engaging in higher risk behaviour with more time spent away as described by Collinson Citation31. Health education messages are also needed that focus on high-risk sexual behaviour that increases risk for HIV infection, and its consequences. Death of household members also appeared to be a significant risk in all age-groups. Previous research indicates that HIV has arguably had the greatest impact at the household level in terms of dissolution and reduced economic status Citation38.
There are several studies relating geographical access to use of health facilities. As one would expect, members of communities that are more distant use the facilities less than those that live nearer, but this does not necessarily translate into increased mortality risk Citation39. In this study, we did not observe significant excess risk associated with increased distance to nearest health facility among infants and children, and this has been demonstrated previously in this setting Citation20 Citation21. Conversely, larger distance from the nearest health facility had a significantly higher risk associated with adult (15–49 years) mortality. This has been shown in a previous study on adult mortality in China Citation40.
A limitation of the study is the potential to miss infant deaths, particularly neonatal deaths, which would underestimate the overall infant mortality burden. However, infant death ascertainment has improved in the study site, especially towards the end of the study period Citation41. Determination of cause-of-death through VA is more problematic for diseases that have less specific symptoms such as HIV/AIDS Citation42. Thus it is likely that the HIV burden may have been underestimated. However, previous validation studies of the VA in Agincourt HDSS have shown that it performs well in this high HIV prevalence setting Citation43. Levels of stigma associated with HIV are high in South Africa particularly prior to the introduction of HAART and may have also contributed to this underestimation.
Conclusions
This work has contributed through the testing, refinement, and application of various advanced spatial–temporal analyses and statistical modelling of risk factors to large longitudinal cohorts such as an HDSS. The novel application of methodologies in public health contributes to our understanding of factors related to mortality and how to quantify them accurately for correlated geostatistical and longitudinal data. This study also contributes to the development of public health interventions by targeting clusters of adverse health outcomes that appear to aggregate geographically and in time as well as the tracking and targeting of other emerging (or re-emerging) communicable diseases that are compromising achievements made in developing countries Citation44. In particular, space–time modelling and mapping can be an effective tool in public health by showing and monitoring diffusion patterns of communicable diseases and in searching for infectious agents. The identification of disparities in the distribution of mortality and related risk factors in space and time, can guide effective policy interventions and programmes. The methods developed, assessed, and used in this thesis contribute to our understanding of risk factor modelling of large correlated longitudinal data.
This study should be regarded as a first step in prioritising specific areas for follow-up public health efforts and evaluating their impact in this rural setting. Targeting prevention of HIV/TB and antiretroviral rollout in significant child and adult mortality clusters and ensuring maternal survival appear key to improving infant and child mortality rates. Further spatial assessment of antiretroviral therapy (ART) rollout that started in this area in 2007 as well as identifying any villages or areas not accessing ART equitably is also critical. The provision of adequate water and sanitation is needed in the mortality clusters particularly in the south-east where diarrhoeal mortality appears high.
Conflict of interest and funding
The author has not received any funding or benefits from industry or elsewhere to conduct this study.
Appendix I
Download MS Word (38 KB)Appendix II
Download MS Word (43.5 KB)Appendix III
Download MS Word (38 KB)Acknowledgements
This work was supported by a PhD fellowship from the South African Centre for Epidemiological Modelling and Analysis (SACEMA), a National Research Foundation (NRF) Centre of Excellence, as well as research fellowships from the MRC/Wits Rural Public Health and Health Transitions Research Unit (Agincourt) through the Wellcome Trust (grant no. 069683/Z/02/Z), UK, and the Swiss–South Africa Joint Research Programme (project no. JRP IZLSZ3_122926). Additional funding was provided by a Wits Faculty of Health Sciences Medical Research Endowment Fund (MREF) (grant no. SARB000). The Agincourt HDSS was funded by the Wellcome Trust, UK (grant no. 058893/Z/99/A, 069683/Z/02/Z, 069683/Z/08/Z), the University of the Witwatersrand, the South African Medical Research Council, and the Andrew W. Mellon Foundation, USA. P. Vounatsou was supported by the Swiss National Science Foundation (project no. 325200_118379).
Notes
1Perinatal period: last period of gestation up to first 7 days of life.
2Neonatal period: within the first 28 days of life.
References
- Cohen M. Changing patterns of infectious disease. Nature. 2000; 406: 726–67.10.3402/gha.v6i0.19239.
- Mathers C, Lopez A, Murray C. The burden of disease and mortality by condition: data, methods and results for 2001. Global burden of disease and risk factors. Lopez AD, Mathers CD, Ezzati M, Jamison D, Murray CJLOxford University Press. New York NY, 2006; 45–240.
- Mathers C, Fat D, Inoue M, Rao C, Lopez A. Counting the dead and what they died from: an assessment of the global status of cause of death data. Bull World Health Organ. 2005; 83: 171–7.
- Kaufman J, Asuzu M, Rotimi C, Johnson O, Owoaje E, Cooper R. The absence of adult mortality data for sub-Saharan Africa: a practical solution. Bull World Health Organ. 1997; 75: 389–95.
- Kuh D, Shlomo Y. A life course approach to chronic disease epidemiology. OxfordUK:Oxford University Press. 2004.
- Best N, Richardson S, Thomson A. A comparison of Bayesian spatial models for disease mapping. Stat Meth Med Res. 2005; 14: 35–9.10.3402/gha.v6i0.19239.
- Walter S. Disease mapping: a historical perspective. Spatial epidemiology – methods and applications. Elliott PWJ, Best N, Briggs DJOxford University Press. OxfordUK, 2000; 223–39.
- Clements AC, Lwambo NJ, Blair L, Nyandindi U, Kaatano G, Kinung'hi S, et al.. Bayesian spatial analysis and disease mapping: tools to enhance planning and implementation of a schistosomiasis control programme in Tanzania. Trop Med Int Health. 2006; 11: 490–503.10.3402/gha.v6i0.19239.
- Rezaeian M, Dunn G, St Leger S, Appleby L. Geographical epidemiology, spatial analysis and geographical information systems: a multidisciplinary glossary. J Epidemiol Community Health. 2007; 61: 98–102.10.3402/gha.v6i0.19239.
- Gobalet J, Thomas R. Demographic data and geographic information systems for decision making: the case of public health. Popul Res Pol Rev. 1996; 15: 537–48.
- Feachem RG. Poverty and inequity: a proper focus for the new century. Bull World Health Organ. 2000; 78: 1–2.
- Marmot M. Social determinants of health inequalities. Lancet. 2005; 365: 1099–104.
- Tollman S, Herbst K, Garenne M, Gear J, Kahn K. The Agincourt demographic and health study: site description, baseline findings and implications. S Afr Med J. 1999; 89: 858–64.
- Kahn K, Tollman S, Collinson M, Clark S, Twine R, Clarke B, et al.. Research into health, population and social transitions in rural South Africa: data and methods of the Agincourt Health and Demographic Surveillance System. Scand J Public Health. 2007; 69: 8–20.10.3402/gha.v6i0.19239.
- Sartorius K, Sartorius B, Tollman S, Schatz E, Kirsten J, Collinson M. Rural poverty ynamics and refugee communities in South Africa: a spatial–temporal model. Popul Space Place. 2011. DOI: 10.3402/gha.v6i0.19239.
- Wikle C, Royle J. Spatial statistical modelling in biology. Mississauga. EOLSS Publishers Co. Ltd. ON, 2002
- Diggle P, Moyeed R, Tawn J. Model-based geostatistics (with discussion). Appl Stat. 1998; 48: 299–350.
- Spiegelhalter D, Thomas A, Best N. WinBUGS version 1.2 user manual Cambridge. MRC Biostatistics Unit. UK, 1999
- Langford I, Leyland A, Rashbas J, Goldstein H. Multilevel modeling of the geographic distribution of diseases. J R Stat Soc Ser C Appl Stat. 1999; 48: 253–68.10.3402/gha.v6i0.19239.
- Sartorius B, Kahn K, Vounatsou P, Collinson M, Tollman S. Young and vulnerable: spatial-temporal trends and risk factors for infant mortality in rural South Africa (Agincourt), 1992–2007. BMC Public Health. 2010; 10: 645.10.3402/gha.v6i0.19239.
- Sartorius B, Kahn K, Collinson MA, Vounatsou P, Tollman SM. Survived infancy but still vulnerable: spatial-temporal trends and risk factors for child mortality in the Agincourt rural sub-district, South Africa, 2011–2007. Geospat Health. 1992; 5: 285–95.
- Gelfand A, Smith A. Sampling-based approaches to calculating marginal densities. J Am Stat Assoc. 1990; 85: 398–409.10.3402/gha.v6i0.19239.
- Spiegelhalter D, Best N, Carlin B, van der Linde A. Bayesian measures of model complexity and fit. J R Stat Soc B. 2002; 64: 583–639.10.3402/gha.v6i0.19239.
- Kulldorff M, Nagarwalla N. Spatial disease clusters: detection and inference. Stat Med. 1995; 14: 799–810.10.3402/gha.v6i0.19239.
- Gelfand A, Ravishanker N, Ecker M. Modeling and inference for point-referenced binary spatial data. Generalized linear models: a Bayesian perspective. Dey D, Ghosh S, Mallick BMarcel Dekker Inc. New York NY, 2000; 373–86.
- StataCorp. Stata Statistical Software: Release 12. College Station. , TX: StataCorp LP. 2011.
- Bradshaw D, Timaeus I, Dorrington R, Nannan N. Reversal in adult mortality trends in South Africa. International Conference on AIDS 2000. Durban, South Africa, 8–13July2000.
- Kahn K, Garenne M, Collinson M, Tollman S. Mortality trends in a new South Africa: hard to make a fresh start. Scand J Public Health Suppl. 2007; 69: 26–34.10.3402/gha.v6i0.19239.
- Tollman SM, Kahn K, Sartorius B, Collinson MA, Clark SJ, Garenne ML. Implications of mortality transition for primary health care in rural South Africa: a population-based surveillance study. Lancet. 2008; 372: 893–901.10.3402/gha.v6i0.19239.
- Dolan CG, Tollman SM, Nkuna VG, Gear JS. The Links between legal status and environmental health: a case study of Mozambican refugees and their hosts in the Mpumalanga (Eastern Transvaal) Lowveld, South Africa. Health Hum Rights. 1997; 2: 62–84.10.3402/gha.v6i0.19239.
- Collinson MA. Striving against adversity: the dynamics of migration, health and poverty in rural South Africa. Glob Health Action. 2010; 3. DOI: 10.3402/gha.v6i0.19239.
- Becher H, Muller O, Jahn A, Gbangou A, Kynast-Wolf G, Kouyate B. Risk factors of infant and child mortality in rural Burkina Faso. Bull World Health Organ. 2004; 82: 265–73.
- Hammer GP, Some F, Muller O, Kynast-Wolf G, Kouyate B, Becher H. Pattern of cause-specific childhood mortality in a malaria endemic area of Burkina Faso. Malar J. 2006; 5: 47.10.3402/gha.v6i0.19239.
- Ronsmans C, Chowdhury ME, Dasgupta SK, Ahmed A, Koblinsky M. Effect of parent's death on child survival in rural Bangladesh: a cohort study. Lancet. 2010; 375: 2024–31.10.3402/gha.v6i0.19239.
- Lurie M. Migration and AIDS in Southern Africa: a review. S Afr J Sci. 2000; 96: 343–7.
- Collinson M, Tollman S, Kahn K, Clark S. Highly prevalent circular migration: households, mobility and economic status in rural South Africa. African Migration in Comparative Perspective Johannesburg. Tienda MFS, Tollman S, Preston-Whyte EWits University Press. South Africa, 2006; 194–216.
- Lurie MN, Williams BG, Zuma K, Mkaya-Mwamburi D, Garnett GP, Sweat MD, et al.. Who infects whom? HIV-1 concordance and discordance among migrant and non-migrant couples in South Africa. AIDS. 2003; 17: 2245–52.10.3402/gha.v6i0.19239.
- Hosegood V, McGrath N, Herbst K, Timaeus I. The impact of adult mortality on household dissolution and migration in rural South Africa. AIDS. 2004; 18: 1585–90.10.3402/gha.v6i0.19239.
- Stock R. Distance and the utilization of health facilities in rural Nigeria. Soc Sci Med. 1983; 17: 563–70.10.3402/gha.v6i0.19239.
- Ali M, Jin Y, Kim D, De Z, Park J, Ochiai R, et al.. Spatial risk for gender-specific adult mortality in an area of southern China. Int J Health Geogr. 2007; 6: 31.10.3402/gha.v6i0.19239.
- Kahn K, Tollman SM, Collinson MA, Clark SJ, Twine R, Clark BD, et al.. Research into health, population and social transitions in rural South Africa: data and methods of the Agincourt Health and Demographic Surveillance System. Scand J Public Health Suppl. 2007; 69: 8–20.10.3402/gha.v6i0.19239.
- Garenne M, Fauveau V. Potential and limits of verbal autopsies. Bull World Health Organ. 2006; 84: 164.10.3402/gha.v6i0.19239.
- Kahn K, Tollman S, Garenne M, Gear J. Validation and application of verbal autopsies in a rural area of South Africa. Trop Med Int Health. 2000; 5: 824–31.10.3402/gha.v6i0.19239.
- Sen K, Bonita R. Global health status: two steps forward, one step back. Lancet. 2000; 356: 577–82.10.3402/gha.v6i0.19239.
- Sartorius B, Kahn K, Vounatsou P, Collinson M, Tollman S. Survived infancy but still vulnerable: spatial-temporal trends and risk factors for child (1–4) mortality in rural South Africa (Agincourt), 1992–2007. Geospat Health. 2011; 5: 285–95.