Abstract
Background : South Africa accounts for more than a sixth of the global population of people infected with HIV and TB, ranking her highest in HIV/TB co-infection worldwide. Remote areas often bear the greatest burden of morbidity and mortality, yet there are spatial differences within rural settings.
Objectives : The primary aim was to investigate HIV/TB mortality determinants and their spatial distribution in the rural Agincourt sub-district for children aged 1–5 years in 2004. Our secondary aim was to model how the associated factors were interrelated as either underlying or proximate factors of child mortality using pathway analysis based on a Mosley-Chen conceptual framework.
Methods : We conducted a secondary data analysis based on cross-sectional data collected in 2004 from the Agincourt sub-district in rural northeast South Africa. Child HIV/TB death was the outcome measure derived from physician assessed verbal autopsy. Modelling used multiple logit regression models with and without spatial household random effects. Structural equation models were used in modelling the complex relationships between multiple exposures and the outcome (child HIV/TB mortality) as relayed on a conceptual framework.
Results : Fifty-four of 6,692 children aged 1–5 years died of HIV/TB, from a total of 5,084 households. Maternal death had the greatest effect on child HIV/TB mortality (adjusted odds ratio=4.00; 95% confidence interval=1.01–15.80). A protective effect was found in households with better socio-economic status and when the child was older. Spatial models disclosed that the areas which experienced the greatest child HIV/TB mortality were those without any health facility.
Conclusion : Low socio-economic status and maternal deaths impacted indirectly and directly on child mortality, respectively. These factors are major concerns locally and should be used in formulating interventions to reduce child mortality. Spatial prediction maps can guide policy makers to target interventions where they are most needed.
Appendices available online under Reading Tools.
Tuberculosis (TB) and the HIV epidemic are among the top public health priorities especially in sub-Saharan Africa. The sub-Saharan African region, which constitutes 10% of the world's population, is home to more than 67% (22.9 million) of people living with HIV Citation1. TB, which is preventable and curable, was responsible for 25% of HIV-related deaths in 2009 and infected 33% of those living with HIV. As a result, the World Health Organization (WHO) aims to reduce TB-related deaths by 1 million by 2015 Citation2. South Africa's population is about 7% [50.6 million Citation3] of the sub-Saharan African population, yet accounts for 24.6% (5.63 million of the 22.9 million) of people living with HIV and an estimated 67% of these have TB. South Africa ranks as the country with the highest co-infection ratesCitation1.
Children are affected in two ways: a very high proportion of child deaths (60% in the year 2000) are attributed to the HIV epidemic Citation4, and approximately 1.9 million children have been orphaned by the HIV epidemic in South Africa Citation1. The nation at the onset of the 21st century had an estimated 70,000 children [about 25% rate of mother-to-child-transmissions (MTCT)] newly infected, whereas 10 years later only 10,000 [3.5% rate of MTCT Citation5], which is indicative of an improved and more effective mother-to-child prevention programme Citation6. In public health, determining patterns of disease occurrence provides the first steps toward increased understanding of determinants and potentially greater control of the disease Citation7. Understanding the associated factors of child HIV/TB mortality and how these are geographically distributed is key for effective interventions.
Several determinants of child mortality have been reported over the past three decades. In the 1980s, researchers explored medical, socio-economic, and demographic causes of child mortality Citation8. In the 1990s, fertility behaviour, nutritional status, breastfeeding and infant feeding, the use of health services by mothers and children, ecological variables, and socio-economic status were studied Citation9. In the 21st century, the focus has been on HIV exposure, poor maternal health, inadequate infant care, increased exposure to infections, deaths of parents or caregivers, immune system abnormalities, poor nutrition, reduced breastfeeding and antiretroviral treatment exposure Citation10. These determinants are interrelated and can be combined into a conceptual framework. This combined with spatial aspects forms social ecological models, which are a component of the fast developing systems science that examines multiple effects and interrelatedness of social elements in an environment Citation11. Analysis of such frameworks can now be handled with the aid of structural equation models also known as pathway analysis.
The motivation for spatial analysis in epidemiological modelling is based on the notion that people living in a household and those in close proximity share similar exposures, which impact on the observed outcomes. In HIV/TB epidemiological research, the focus has largely been on drug development, societal integration, personal nutrition, physical health, and care giving Citation8–Citation10 Citation12. Country-wide or region-specific pooled results are often reported, disregarding geographical confounding that commonly exists. Little has been done to interlink factors associated with child HIV/TB mortality or model their impact on areas of residence (households) Citation13. The few studies implementing spatial analysis have looked across different infectious diseases Citation14–Citation16, used different study designs (cross-sectional and longitudinal) Citation17 Citation18, and considered varying levels of analysis (village, household, and individual) Citation16 Citation18 Citation19. As this is an emerging field, few studies have focused on HIV/TB cause-specific mortality assessing geographical differentials in South Africa over time Citation19.
The primary aim of this paper was to investigate the cause-specific (HIV/TB) mortality determinants and their spatial (geographical) distribution in the Agincourt sub-district of rural northeast South Africa for children aged 1–5 years in 2004. Our secondary aim was to model how the associated factors were interrelated as either direct (underlying) or indirect (proximal) factors of child HIV/TB mortality using pathway analysis based on the Mosley-Chen conceptual framework that incorporates social, biological, and ecological determinants of child mortality Citation8.
Methods
Agincourt sub-district data and study design
The Agincourt health and socio-demographic surveillance system was established in 1992 in a remote rural sub-district in the Bushbuckridge Municipality of Mpumalanga Province, northeast South Africa near the Mozambique border Citation20. There are annual census updates that collect socio-demographic information including in-migration and out-migration, mortality, fertility, health, and behavioural data Citation20.
In this study, we extracted data for 2004 describing a cross-sectional study of households in the study site. In that year, the Agincourt health and socio-demographic surveillance system covered a sub-district population of over 70,000 persons living in approximately 12,000 households scattered throughout 21 neighbouring villages. There were several health facilities in the area as shown in .
Fig. 1. Agincourt sub-district in 2004 showing villages (grey shading) schools (flags), and health facilities (circled grey crosses).
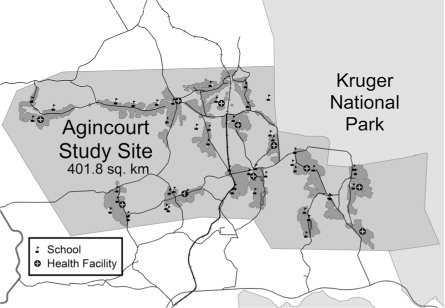
Cause of death data were obtained through verbal autopsies conducted on every recorded death during the year Citation21. Trained lay field workers conducted interviews within 1–11 months after a death, with the closest caregiver of the deceased in his/her mother tongue. Two medical practitioners independently determined cause of death; a third diagnosis was sought to resolve discrepancies. Their consensus cause of death was classified according to the WHO International Classification of Diseases 10 (ICD10) Citation22. The reported signs and symptoms ascertained HIV/TB mortality in children, and in some instances this was substantiated by the mother's cause of death Citation22. Over 90% of the health and socio-demographic surveillance system (HDSS) households were geo-coded, thus enabling spatial analyses at household level.
Dependent and independent variables
The study sample included all children aged between 1 and less than 5 years who were registered in the Agincourt HDSS between January and December 2004. The independent variables used were child's gender, nationality and age; mother's age, parity and death; gender of household head, cumulative deaths in household, number of household dwellers, minimum distance to health facility, and household socio-economic status. The latitude and longitude of each household were used to construct the variables for the spatial correlation (random effects), based on the straight line (Euclidean) distances between all pairs of households. The dependent variable was death due to HIV and TB determined by the ICD10 codes A16–A19Footnote1 for TB and B20–B24Footnote2 for HIV. The verbal autopsy cannot reliably distinguish HIV and TB due to low sensitivity and specificity; thus, these were combined in our analysis Citation23.
Conceptual framework: determinants of child mortality
As a way of interrelating child mortality determinants, we formulated a hybrid conceptual framework for child mortality determinants and disease ecology as proposed by Mosley-Chen and Meade Citation8 Citation24. This approach integrates ecological, social, and biological variables in the analysis, with the idea that these determinants operate through a common set of either ‘proximate’ (intermediate or indirect) or ‘underlying’ (direct) variables that impact on HIV/TB child mortality Citation8 Citation25–Citation27. Variables are either endogenous (outcome variables) or exogenous (explanatory) or both, and these are modelled using structural equation models. Details of the structural equation model are given in Appendix 1.
We used the observed variables: child specific, mother specific, household related; and unobserved (latent) variables: socio-economic quintiles and spatial (ecological) variables. We tested the pathways shown in , to measure the direct and indirect determinants of child mortality for the Agincourt sub-district in 2004. Two approaches that complemented each other were used in our modelling. Firstly, we modelled the HIV/TB mortality determinants for children aged 1–5 years in 2004 and adjusted for their spatial random effects. In relation to our , we estimated the direct effects pathways (red arrows) from all categories of the explanatory (exogenous) variables controlling for confounders amongst them and their spatial or geographical effects (orange arrows). Results for these are presented in and . Lastly, using the significant variables as outcomes (endogenous), we determined the indirect effects (proximal denoted by purple arrows) within and between categories to establish those factors impacting on child HIV/TB mortality.
Fig. 2. Conceptual framework for modelling childhood HIV/TB-related mortality [adapted from Meade Citation24]. The red arrows reflect a Fig. 2. Conceptual framework for modelling childhood HIV/TB-related mortality [adapted from Meade (24)]. The red arrows reflect a direct effect on mortality and the purple (explanatory variables) and orange (spatial random effects) arrows depict an indirect effect.
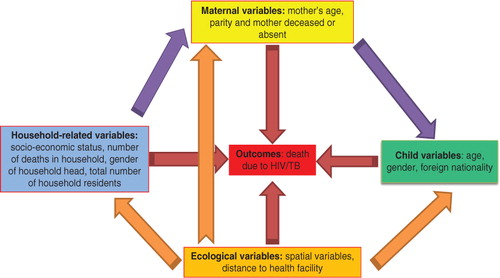
Table 1. Child HIV/TB mortality: descriptive statistics and results of two multiple logit regression analyses, Agincourt sub-district 2004
Statistical methodology
We modelled the child HIV/TB mortality data to find associated factors and determine geographic disparities. Statistical techniques take into account spatial correlations by introducing spatially structured random effects into the model. These are usually based on independent observations. However, with multilevel correlated structures commonly used, maximum likelihood estimation (MLE) methods often underestimate the standard error; thus, the statistical significance is overestimated Citation28. Analysis was carried out using a geospatial logit regression model, which catered for individual- and household-level random effects Citation29. Inference was done using classical MLE and Bayesian inference techniques to fit the non-spatial and spatial models, respectively. Bayesian estimation of the many parameters was done using Markov Chain Monte Carlo (MCMC) simulations with the aid of Metropolis Hastings (MH) steps Citation29. Geospatial prediction maps showing the geographical coordinates, adjusting for child HIV/TB mortality determinants, were used in the analysis.
Data extraction was carried out using Structured Query Language (SQL), data management was done in STATA 10.0 Citation30, and structural equation modelling was performed in STATA 12.1 (Stata Corporation, College Station, Texas, USA). The Bayesian models were fit using software available in the public domain: BayesX version 2.1 for the spatial modelling and R-cran version 2.12.2 for geospatial mapping using the analysis and mapping packages, maptools, maps, spBayes, and Multilevel B-spline Approximation (MBA) Citation31 Citation32.
Ethical clearance and informed consent
While this study used secondary data, the original study on which it was based was careful to consider ethical issues. Verbal informed consent was obtained when the Agincourt health and socio-demographic update rounds were conducted and also when verbal autopsy data were collected from a close relative of the deceased. To avoid emotionally stressing the interviewee, a culturally accepted period of 1 month was observed before the interview was conducted. Risks of stigmatisation were minimised through special training of fieldworkers on the importance of confidentiality. This work was granted ethical clearance by the University of the Witwatersrand's Committee for Research on Human Subjects (No. 960720 and M081145).
Results
Of the 6,692 children between 1 and less than 5 years, with a mean age of 2.8 years, 54 HIV/TB-related deaths were recorded in the HDSS in 2004. There were 5,084 fully geo-coded households that recorded children either living or dead. Just over half the children were female (51%), and more than a third (36%) were born to former Mozambican refugees. The mean age of mothers was 29 years, and the mean distance to the nearest health facility was 2.33 km. shows the results for the descriptive, univariate, and multivariable logit regression analyses. Maternal death had the greatest effect on child HIV/TB mortality [logit=1.386 (0.707)], equivalent to an adjusted odds ratio (AOR) of 4.00 [95% Bayesian credible interval (BCI)=1.01–15.80] controlling for spatial correlation and keeping all other variables constant.
Multivariable analysis showed three further significant predictors of child mortality in 2004, which were child, maternal, and household specific. Adjusting for spatial correlation and keeping all other variables constant, there were several significant determinants of child HIV/TB mortality. Child-specific differentials showed that boys were almost twice as likely to die compared to girls [logit=0.641 (0.291); AOR=1.89; 95% BCI=1.07–3.36]. As the children got older, there was a protective effect against death; for every year the child aged, the likelihood of death decreased by about 50% [logit=−0.630 (0.137); AOR=0.53; 95% BCI 0.40–0.70]. Male-headed households had a 60% lower risk of child HIV/TB mortality compared to female-headed households [logit=−0.895 (0.30); AOR=0.41; 95% BCI 0.23–0.74].
Spatial disparities can be viewed on maps, which can show unexpected relationships that may have been overlooked in standard regression analysis. We obtained household-specific posterior odds ratios, which were adjusted for child-, maternal-, and household-related variables in the multivariable models. One main hotspot emerged with high mortality in the north, and there were two southerly areas with low mortality. The odds ratio posterior map in shows the hotspot area between longitude 20° and 25° and latitude −2,750° and −2,745°. This area is characterised by the following: higher rate of maternal deaths, more male child mortality, lack of a health facility, and being furthest from any health facility as shown in .
The results from the conceptual framework analysis of child HIV/TB mortality are shown in and . All of the connected arrows show pathways that were statistically significant whether directly or indirectly. Being male (logit=5.2×10−3) and older child age (logit=−4.6×10−3) both impacted directly on HIV/TB mortality controlling for potential confounders related to the maternal and households variables. Upon treating male household headship as an exogenous variable, this directly impacted negatively on child HIV/TB deaths (logit=−7.6×10−3), adjusting for household variables. Moreover, when male headship was an endogenous variable, the following household variables indirectly impacted mortality: better socio-economic status and larger household size had a protective effect (logit=7.7×10−3) and increase in household deaths resulted in greater child HIV/TB mortality (logit=−8.3×10−2).
Fig. 4. Conceptual framework pathways results for Agincourt sub-district child mortality determinants in 2004. Key for variable names: very poor versus most poor (vpoor_mostpoor), moderately poor versus most poor (modpoor_mostpoor), least poor versus most poor (leastpoor_mostpoor), poor versus most poor (poor_mostpoor), age of child in years (age_ch), household size (hh_size), cumulative household deaths (cum_hh_deaths), gender of household head (hh_gender), mother age in years (age_mum), mother deceased by 2004 (mother_deceased), child born to former refugee parents (refuge), gender (gender), minimum distance to health facility (min_dist) parity (parity), child deceased in 2004 (dead). Other: the arrows pointing from the exogenous (explanatory) to endogenous (dependent) variables and the error terms ( ɛ ) placed on all five endogenous variables.
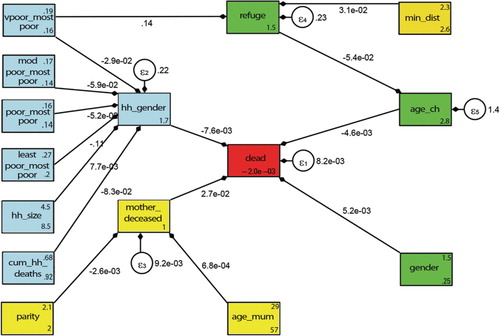
Table 2. Direct, indirect and total effects of child HIV/TB mortality in 2004, Agincourt sub-district
details the direct pathways for child HIV/TB deaths (arrows directly linked to the red square) and the indirect pathways showing all possible routes for child HIV/TB deaths as shown in . In our analysis, poverty indirectly impacted on HIV/TB child mortality as conferred by two pathways: first, male headship of households (protective), and second, refugee status (a risk) controlling for child's age (as shown on foot note). The greatest direct impact on child HIV/TB mortality was maternal death (logit=2.7×10 −2), adjusting for the indirect effects of parity (logit=−2.6×10 −3) and mother's age (logit=6.8×10 −4).
Discussion
In this study, we showed that HIV/TB child mortality is influenced by a combination of child, maternal, household, and spatial determinants. Over the years 2002–2005, HIV/TB alone accounted for 34% of all-cause mortality among children living in the area Citation23. While being male was a risk factor for HIV/TB mortality as children got older, irrespective of sex they were less likely to die. A major finding in our study was that maternal death had the greatest direct impact on HIV/TB child deaths. A comparable study in the same area, adjusting for spatial random effects at village level, showed that infants whose mothers had died were at greater risk of death during the first 12 months of life Citation16.
The main source of TB transmission among children are adults, as shown by a study on a high-risk spatial cluster in South Africa with an incidence three times higher than the WHO levels Citation17. The most likely cause of HIV infection was mother-to-child transmission. Prevention of mother-to-child transmission (PMTCT), using a single-dose of nevirapine (sdNVP) administered to the child and mother, was found to reduce child mortality by about 9% in South Africa Citation33. Between 2004 and 2012 the guidelines for PMTCT and ART eligibility have changed. There is also a seven-fold lower (from 25 to 3.5%) rate of infection from mother to child over the period 2000–2010 Citation5. Kimani-Murage et al. (2009) found that knowledge of child HIV status influenced the manner of care-giving as well as acceptance and up-take of community-wide interventions Citation31. The mother's survival significantly increases the likelihood of the child's survival, a finding that strengthens the current policy focus on maternal health. In South Africa, a comprehensive three stage process has been set up to improve maternal, foetal, infant, and ultimately child survival through antenatal care, labour and delivery, and postnatal care Citation34. Reduction of TB morbidity and mortality is the focus of the WHO 2015 goal of zero new TB infections and zero TB-related deaths Citation2. The positive impact of the PMTCT programme and ART access has also been demonstrated in another rural South Africa site Citation35.
In Agincourt, female-headed households had a higher child mortality compared to those headed by males. Studies in Tanzania, Zambia, and Limpopo province South Africa showed similar results Citation36–Citation38. The pathway analysis () demonstrates that male-headship does not independently lead to lower mortality but is a proxy for better socio-economic status of households. Main breadwinners are usually male with females having fewer opportunities in the labour market and consequently earning less. The absence from the household of a male due to death or permanent out-migration tended to impact negatively on household socio-economic status, leading to 90% greater child mortality odds Citation39. In other African countries, similar patterns were observed as men temporarily migrate within a country or to neighbouring countries due to either pull or push factors Citation40–Citation42. HIV/TB deaths have lowered the life expectancy of working men from the area who often “return home to die” from their jobs Citation21. A recent qualitative study found that a father's unemployment, inability to work, and reluctance to provide support were common factors in HIV-infected households Citation31. From our pathway analysis ( and ), we observed an indirect impact on child HIV/TB mortality: as the number of household deaths increased, the risk of child deaths was higher. This suggests that households with a greater burden of mortality are more vulnerable socio-economically through loss of breadwinners and high health care and funeral costs. This compromises a family's ability to feed and care for all children, including those who are HIV positive. We also observed that former Mozambicans are at greater risk of child HIV/TB deaths, indicating the need for health care interventions that target these marginalised and vulnerable households.
Strengths and limitations of the study
The strengths and limitations of this paper are discussed in four categories: classification of exposures and outcomes; local or global relevance of results; confounding and effect modification; and analysis deviance.
A major strength in our observed variables is that the data are consistently updated, corrected, and checked for inconsistencies. Nevertheless, there are potentially two variable-related biases, namely selection and recall bias. Selection bias may occur if permanent out-migrants differ from residents or temporary migrants, which results in incomparable groups. Recall bias on the outcome variable mortality is unlikely as death is a major household event. However, it may affect the quality of the verbal autopsy interview, conducted at least 1 month but up to 11 months after death, and hence the ability of medical experts to assign a likely cause of death.
Our study focused on one sub-district only, but demonstrated geographical differentials in child HIV/TB mortality. There was one main hotspot with highest mortality and a visible downward trend in mortality moving southwards. These patterns are comparable to those found in other studies carried out in the area Citation16 Citation19. While many rural areas are not geo-coded to enable direct comparison of trends and patterns, our findings reflect similar patterns in other rural settlements at least in northeast South Africa.
To deal with confounding variables, we used multiple regression and added the spatial random effects in our modelling and also performed pathway analysis. Mediation was tested for in the pathway analysis as we determined factors directly and indirectly related to mortality and malnutrition. However, we were unable to model time varying covariates as this was a cross-sectional study design.
The robustness of our analysis procedures was demonstrated in that after catering for the spatial household random effects, we obtained narrower confidence bands compared to the non-spatial analogue. Therefore, catering for the spatial random effects further improves the model and parameter estimation compared to classical statistical models. Bayesian and pathway analysis combined were able to handle the complex structure of hierarchical data from the Agincourt health and socio-demographic surveillance system. Other advantages of Bayesian modelling include the ability to adjust for spatial confounding, and the ability to handle many parameters and inclusion of prior-known information on the distribution of data.
Conclusion
The growing field of spatio-temporal epidemiology brings in person, place, and time aspects in the risk factor analysis. Spatial Bayesian models provide better-fitting models compared to non-spatial models. This procedure also enables the drawing of risk maps, which can be used by policy makers to target and develop community-based interventions.
Going forward, further studies investigating trends over time using spatio-temporal Bayesian analysis will strengthen the evidence on associated factors and trends found in this study and demonstrate the value of this analytic approach. Studies comparing deaths due to other causes with those for HIV/TB in children would add policy value. Similarly, adopting a secondary analysis of a cohort study design would have enabled temporality to be investigated in greater detail and survival analysis to be undertaken. Moreover, a combination of qualitative and quantitative analysis methods is needed to understand the proximal and distal factors that influence child HIV/TB mortality. Findings that can be used for national policy would require sentinel sites located strategically across the country.
Conflict of interest and funding
The authors declare that they have no competing interests.
Appendix
Download PDF (134.4 KB)Acknowledgements
This work was made possible by doctoral fellowship support from the World Health Organization, Tropical Disease Research (WHO/TDR), and Swiss–South Africa Joint Research Programme. The MRC/Wits Rural Public Health and Health Transitions Research Unit (Agincourt) is funded by the Wellcome Trust, UK, (grants 058893/Z/99/A, 069683/Z/02/Z and 085477/Z/08/Z), the University of the Witwatersrand and Medical Research Council, South Africa, and the Andrew W Mellon and William and Flora Hewlett Foundations, USA. Special thanks goes to Benn Sartorius for data extraction, David Rees for his valuable review of an early draft, community leaders, field workers, supervisors, and the Agincourt community.
Notes
1A16=Respiratory tuberculosis; not confirmed bacteriologically or histologically; A17=Tuberculosis of nervous system; A18=Tuberculosis of other organs; A19=Miliary tuberculosis.
2B20=Human immunodeficiency virus (HIV) disease resulting in infectious and parasitic diseases; B21=Human immunodeficiency virus (HIV) disease resulting in malignant neoplasms; B22=Human immunodeficiency virus (HIV) disease resulting in other specified diseases; B23=Human immunodeficiency virus (HIV) disease resulting in other conditions; B24=Unspecified human immunodeficiency virus (HIV) disease.
References
- UNAIDS. Report on the global AIDS epidemic 2011. Available from: http://www.avert.org/africa-hiv-aids-statistics.htm [cited 23 October 2012].
- WHO. One million lives can be saved between now and the end of 2015 by preventing and treating tuberculosis among people living with HIV.. Available from: http://www.who.int/tb/challenges/hiv/fastfactsbrochure.pdf [cited 23 October 2012]..
- Statistics South Africa (StatsSA). Statistical release P0302 2011. Available from: http://www.statssa.gov.za/publications/P0302/P03022011.pdf [cited 23 October 2012].
- Bradshaw D, Bourne D, Nannan N. What are the leading causes of death among South African children. MRC Policy Brief & Unicef. 2003; 3: 1–4.
- Doherty T, Sanders D, Goga A, Jackson D. Implications of the new WHO guidelines on HIV and infant feeding for child survival in South Africa. Bull World Health Organ. 2011; 89: 62–7.10.3402/gha.v6i0.19266.
- UNICEF. State of the World's Children year 2009. Available from: http://www.unicef.org/publications/index_47127.html [cited 23 October 2012].
- Waller LA, Gotway CA. Applied spatial statistics for public health data. Wiley. Atlanta GA, 2004
- Mosley WH, Chen LC. An analytical framework for the study of child survival in developing countries. Popul Dev Rev. 1984; 10: 25–45.10.3402/gha.v6i0.19266.
- Rutstein SO. Factors associated with trends in infant and child mortality in developing countries in the 1990s. Bull World Health Organ. 2000; 78: 1256–70.
- Filteau S. The HIV-exposed, uninfected African child. Trop Med Int Health. 2009; 14: 276–87.10.3402/gha.v6i0.19266.
- Guerrero L, La Valley A. Conflict, emotion, and communication. The SAGE handbook of conflict communication: integrating theory, research, and practice. Oetzel JG, Ting-Toomey SSAGE. Thousand Oaks CA, 2006; 69–96.
- Hargreaves J, Collinson M, Kahn K, Clark S, Tollman S. Childhood mortality among former Mozambican refugees and their hosts in rural South Africa. Int J Epidemiol. 2004; 33: 1271–8.10.3402/gha.v6i0.19266.
- Marshall RJ. A review of methods for the statistical analysis of spatial patterns of disease. J Roy Stat Soc Series A (Statistics in Society). 1991; 154: 421–41.10.3402/gha.v6i0.19266.
- Gosoniu L, Vounatsou P, Tami A, Nathan R, Grundmann H, Lengeler C. Spatial effects of mosquito bednets on child mortality. BMC Publ Health. 2008; 8: 356.10.3402/gha.v6i0.19266.
- Moonan PK, Bayona M, Quitugua TN, Oppong J, Dunbar D, Jost KC, et al.. Using GIS technology to identify areas of tuberculosis transmission and incidence. Int J Health Geogr. 2004; 3: 23.10.3402/gha.v6i0.19266.
- Sartorius B, Kahn K, Vounatsou P, Collinson M, Tollman S. Young and vulnerable: spatial-temporal trends and risk factors for infant mortality in rural South Africa (Agincourt), 1992–2007. BMC Publ Health. 2010; 10: 645.10.3402/gha.v6i0.19266.
- Middelkoop K, Bekker LG, Morrow C, Zwane E, Wood R. Childhood tuberculosis infection and disease: a spatial and temporal transmission analysis in a South African township. S Afr Med J. 2009; 99: 738–43.
- Tanser F, LeSueur D, Solarsh G, Wilkinson D. HIV heterogeneity and proximity of homestead to roads in rural South Africa: an exploration using a geographical information system. Trop Med Int Health. 2000; 5: 40–6.10.3402/gha.v6i0.19266.
- Musenge E, Vounatsou P, Kahn K. Space-time confounding adjusted determinants of child HIV/TB mortality for large zero-inflated data in rural South Africa. Spat Spatio-temporal Epidemiol. 2011; 2: 205–17.10.3402/gha.v6i0.19266.
- Kahn K, Tollman SM, Collinson MA, Clark SJ, Twine R, Clark BD, et al.. Research into health, population and social transitions in rural South Africa: data and methods of the Agincourt Health and Demographic Surveillance System. Scand J Publ Health. 2007; 35: 8–20.10.3402/gha.v6i0.19266.
- Clark SJ, Collinson MA, Kahn K, Drullinger K, Tollman SM. Returning home to die: circular labour migration and mortality in South Africa. Scand J Public Health Suppl. 2007; 69: 35–44.10.3402/gha.v6i0.19266.
- Kahn K, Tollman SM, Garenne M, Gear JS. Validation and application of verbal autopsies in a rural area of South Africa. Trop Med Int Health. 2000; 5: 824–31.10.3402/gha.v6i0.19266.
- Tollman SM, Kahn K, Sartorius B, Collinson MA, Clark SJ, Garenne ML. Implications of mortality transition for primary health care in rural South Africa: a population-based surveillance study. Lancet. 2008; 372: 893–901.10.3402/gha.v6i0.19266.
- Meade MS. Medical geography as human ecology: the dimension of population movement. Geogr Rev. 1977; 67: 379–93.10.3402/gha.v6i0.19266.
- Lewis JJC, Donnelly CA, Mare P, Mupambireyi Z, Garnett GP, Gregson S. Evaluating the proximate determinants framework for HIV infection in rural Zimbabwe. Sex Transm Infect. 2007; 83: i61–9.10.3402/gha.v6i0.19266.
- Boerma J, Weir SS. Integrating demographic and epidemiological approaches to research on HIV/AIDS: the proximate-determinants framework. J Infec Dis. 2005; 191: S61.10.3402/gha.v6i0.19266.
- Lopman B, Nyamukapa C, Mushati P, Mupambireyi Z, Mason P, Garnett GP, et al.. HIV incidence in 3 years of follow-up of a Zimbabwe cohort—1998–2000 to 2001–03: contributions of proximate and underlying determinants to transmission. Int J Epidemiol. 2008; 37: 88–105.10.3402/gha.v6i0.19266.
- Cressie NAC. Statistics for spatial data. Wiley. New York, 1993
- Banerjee S, Carlin BP, Gelfand AE. Hierarchical modeling and analysis for spatial data. Chapman & Hall/CRC. Boca Raton FL, 2004
- Stata Corporation. Stata statistical software release 10. College StationTX: Stata Corporation LP. 2007.
- Kimani-Murage EW, Manderson L, Norris SA, Kahn K. ‘You opened our eyes’: care-giving after learning a child's positive HIV status in rural South Africa. Health Soc Care Community. 2010; 18: 264–71.
- Belitz C, Brezger A, Kneib T, Lang S, Fronk E, Heinzl F, et al.Bayesx-software for bayesian inference in structured additive regression models. 2011. Version 2.01. Available from: http://www.stat.uni-muenchen.de/∼bayesx [cited 23 October 2012]..
- Coetzee D, Hilderbrand K, Boulle A, Draper B, Abdullah F, Goemaere E. Effectiveness of the first district-wide programme for the prevention of mother-to-child transmission of HIV in South Africa. Bull World Health Organ. 2005; 83: 489–94.
- National Department of Health. Clinical guidelines: prevention of mother-to-child transmission (PMTCT) 2010. Available from: http://wwwfidssacoza/images/PMTCT_Guidelinespdf [cited 23 October 2012]..
- Ndirangu J, Newell M-L, Tanser F, Herbst AJ, Bland R. Decline in early life mortality in a high HIV prevalence rural area of South Africa: evidence of HIV prevention or treatment impact?. AIDS. 2010; 24: 593–602.10.3402/gha.v6i0.19266.
- Katapa R. A comparison of female-and male-headed households in Tanzania and poverty implications. J Biosoc Sci. 2006; 38: 327.10.3402/gha.v6i0.19266.
- Wiegers E, Curry J, Garbero A, Hourihan J. Patterns of vulnerability to AIDS impacts in Zambian households. Dev Change. 2006; 37: 1073–92.10.3402/gha.v6i0.19266.
- Oni S, Obi C, Okorie A, Thabede D, Jordan A. The economic impact of HIV/AIDS on rural households in Limpopo Province. S Afr J Econ. 2002; 70: 551–62.10.3402/gha.v6i0.19266.
- Collinson MA. Striving against adversity: the dynamics of migration, health and poverty in rural South Africa. Medical Dissertations, Umeå University, Umeå. 2009
- Posel D, Casale D. What has been happening to internal labour migration in South Africa, 1993–1999?. S Afr J Econ. 2003; 71: 455–79.10.3402/gha.v6i0.19266.
- Hahn MB, Riederer AM, Foster SO. The livelihood vulnerability index: a pragmatic approach to assessing risks from climate variability and change--a case study in Mozambique. Global Environ Change. 2009; 19: 74–88.10.3402/gha.v6i0.19266.
- Beguy D, Bocquier P, Zulu EM. Circular migration patterns and determinants in Nairobi slum settlements. Demographic Res. 2010; 23: 549–86.10.3402/gha.v6i0.19266.
- Stata Corporation. Stata statistical software release 12. College StationTX: Stata Corporation LP. 2011.
- Kline RB. Principles and practice of structural equation modeling. 3rd ed. Guilford Press. New York, 2011
- Bollen KA. Structural equations with latent variables. Wiley. New York, 1989
- Bollen KA, Curran PJ. Latent curve models: a structural equation perspective. Wiley-Interscience. New York, 2006