Abstract
Lakes influence the structure of the atmospheric boundary layer and, consequently, the local weather and local climate. Their influence should be taken into account in the numerical weather prediction (NWP) and climate models through parameterisation. For parameterisation, data on lake characteristics external to the model are also needed. The most important parameter is the lake depth. Global database of lake depth GLDB (Global Lake Database) is developed to parameterise lakes in NWP and climate modelling. The main purpose of the study is to upgrade GLDB by use of indirect estimates of the mean depth for lakes in boreal zone, depending on their geological origin. For this, Tectonic Plates Map, geological, geomorphologic maps and the map of Quaternary deposits were used. Data from maps were processed by an innovative algorithm, resulting in 141 geological regions where lakes were considered to be of kindred origin. To obtain a typical mean lake depth for each of the selected regions, statistics from GLDB were gained and analysed. The main result of the study is a new version of GLDB with estimations of the typical mean lake depth included. Potential users of the product are NWP and climate models.
1. Introduction
A lake is a considerable volume of water within a land depression without inlet from a sea. Lakes occupy only about 1.8% of the land surface but are distributed very irregularly. Turbulent and radiation fluxes from lakes and from land surface differ a lot, thus lakes influence local weather conditions and local climate (Eerola et al., Citation2010; Samuelsson et al., Citation2010). In regions with a large number of lakes such as Canada, the Scandinavian Peninsula, Finland, Northern Russia, their influence is of particularly importance. For regions with a small number of lakes, their influence is less pronounced, but still not negligible. Lakes are also involved in a carbon cycle (Tranvik et al., Citation2009), and thermokarst lakes are among the sources of methane (Walter et al., Citation2007), which means that lakes may noticeably influence global climate. The effect of lakes should be parameterised in numerical weather prediction (NWP) and climate modelling. Sometimes a proper description of a lake state in a model may help prevent serious errors in a forecast (K. Eerola, personal communication 2014). A lake parameterisation becomes more influential with an increase of a model horizontal resolution and when a model grid box is divided into several different surface types. Therefore, even small-size lakes become visible on a model grid.
NWP and climate models need a computationally cheap lake model for the parameterisation of lakes. Currently, for this purpose many atmospheric models use the bulk (zero-dimensional) lake model FLake (Mironov, Citation2008). Information about characteristics of lakes (external model parameters) is also necessary. Atmospheric models need gridded data. Therefore, lake data should be provided in a gridded form. Lake fields should be global and contain information about all existing lakes. Lake depth is the most important parameter used by all lake models. We may use the bathymetry when it is available, or for middle and small size lakes we may use the mean lake depth, which is recognised to be a reasonable approximation in atmospheric applications, when we are mainly interested in surface lake processes on large scales. Moreover, for atmospheric modelling (in contrast with hydrological), an accuracy and even a reliability of depth data are not critical, but a global coverage is essential. In the absence of direct measurements, indirect estimates, though less precise, can be used.
For the parameterisation of lakes in NWP and climate modelling, Global Lake Database (GLDB) (Kourzeneva, Citation2009; CitationKourzeneva et al., 2012a) was developed. It contains gridded data on the lake depth and includes slightly more than 13000 lakes in its first version (GLDBv.1). However, the recently assumed number of lakes in the world exceeds 8 million. Most lakes, especially of small size, were never inspected to measure the depth. To estimate the depth of the uninspected lakes, it is natural to assume (Doganovsky, Citation2006; Doganovsky, Citation2012) that water bodies of the same origin and the same age should have a similar size. For example, it is widely known that tectonic lakes are deeper than glacial, karst lakes usually have small surface area, but are rather deep, and eolian lakes are usually shallow. So, different information, for example, about the geological origin of lakes, could be used to estimate the lake depth indirectly (Kondratiev, Citation2010).
There are many studies devoted to estimations of the lake depth and the water transparency characteristics on the basis of richness in nutrients and/or mineralisation and soil type of a surrounding landscape (Canfield et al., Citation1985; Lee and Rast, Citation1997). However, richness in nutrients is hard to determine precisely without any direct observations. Besides, different studies propose different methods for different parameters based on statistics from different territories, and these results need to be generalised. Hence, resulting maps would have low accuracy. We should also mention an attempt described in Balsamo et al. (Citation2010) to estimate the lake depth by variational methods, using the annual lake water surface temperature measurements from remote sensing and a lake model. The main problem with this approach is errors in remote sensing data.
As for geological origin of lakes, in literature we found only two studies devoted to its relation to the lake depth and size. In Doganovsky (Citation2012) and Doganovsky (Citation2006), lake volume is estimated as a function of the lake area with the regional coefficients dependent on the regional geology mostly of the Quaternary period. From the lake volume and the lake area, the mean lake depth may be easily calculated. Hereafter, we refer to this method as ‘improved geomorphologic’. Unfortunately this method is developed only for the Baltic region and is not applicable for very small lakes. The method described in Kitaev (Citation1984) is based on geographical zones (we refer to it as ‘geographical’). Different lake characteristics are considered to be dependent on the geographical zone, where the lake is located. Only geographical zones belonging to the boreal zone are considered. This method has low accuracy. A detailed description of both methods can be found in Sections 4.3 and 4.4. Since each of them has their advantages and disadvantages, in our study they were used only in addition.
The main purpose of our study is to upgrade the GLDB for parameterisation of lakes in atmospheric modelling (CitationKourzeneva et al., 2012a) by indirect estimates of the mean depth for lakes in a boreal zone on the basis of their geological origin. Estimates are obtained from statistical and expert evaluation. First, we outlined boundaries of regions with kindred geological origin of lakes. Second, we proposed a typical lake depth for these regions. Finally, the new version of GLDB was developed. It includes typical mean depth estimations from the geological origin of boreal lakes. Section 2 describes data sources of the study, namely GLDBv.1 and different maps which were used. Section 3 describes methodology of allocating regions with kindred origin of lakes. Section 4 is devoted to expert evaluation to propose the typical lake depth for allocated regions from statistical analysis, method of analogies, improved geomorphologic and geographical methods. Section 5 presents the new product. Section 6 demonstrates a sensitivity of modelling results to the upgrades in GLDB. Section 7 presents our conclusions of the study.
The study is interdisciplinary. The problem was formulated by the meteorological community, which is the main user of the product and defines main requirements to it. However to solve it, knowledge from hydrology and hydrogeology was necessary. To outline regions with typical geological origin of lakes, we used an approach which we refer to as ‘the bottom-up approach’ in the rest of the paper. We started from information about the deepest layers of the Earth crust from Tectonic Plates Map, then we applied data about middle layers from the geological map, then about upper layers from the map of Quaternary deposits and finally about the top layer from the geomorphologic map. We considered first the boreal zone, making the first step forward to global estimates. Even nowadays, this climate zone remains the most explored, as it was in the mid-80s, when the first study generalising morphological characteristics of lakes appeared (Kitaev, Citation1984). Northern boundary of the boreal climate zone was considered to be the North Pole. Southern boundary was drawn in the Northern hemisphere at 30° latitude for the North America continent, 50° latitude for the Europe continent (westward from the 40° longitude) and 40° latitude for the Asia continent (eastward from the 40° longitude).
2. Data sources
2.1. GLDBv.1 and the mapping method
GLDB (Kourzeneva, Citation2009; CitationKourzeneva et al., 2012a) was developed especially for the purpose of parameterisation of lakes in NWP and climate modelling. It contains mapped information on the lake depth. This information is presented on the fine grid with the resolution of 30 arc sec (approximately 1 km). Basically, it contains the mean lake depth data, with bathymetry for several large lakes. Information in gridded form gives an opportunity to use it for global atmospheric models.
GLDB applies the following data sources: (i) data on the mean depth for individual lakes from different regional databases (ca. 13000 lakes), (ii) the global map – ecosystem dataset ECOCLIMAP2 (Champeaux et al., Citation2004), and (iii) bathymetry data for 36 large lakes, from ETOPO1 (Amante and Eakins, Citation2009) and digitised navigation and topographic maps.
The dataset for individual lakes contains the following information for each lake: the name, the location country name, geographical coordinates, the mean depth, the maximum depth, and the surface area. At the moment, the dataset for individual lakes consists of over 13000 freshwater lakes (and in addition 220 saline lakes and endorheic basins). Natural and artificial lakes both are presented and not distinguished. In this study, we updated this list by fixing errors and adding ca. 500 lakes.
To map the data for individual lakes, the automatic method described in CitationKourzeneva et al. (2012a) is used. The method is probabilistic: it is assumed that all data have random errors. Data on freshwater lakes are processed and data on saline lakes are skipped. When there is a lake on the map, but its depth is unknown (no data in the dataset for individual lakes), a ‘default’ depth of 10 m is used. The result of data processing is the global gridded lake depth data set with a resolution of 30 arc sec. In areas with missing data, the map shows the ‘default’ depth. An additional map containing information about sources of data is also provided. This information is coded according to the following legend:
no lake or river (sea or land), depth=0 m
no information about this lake in the list of individual lakes, depth=10 m
missing lake depth in the list of individual lakes, depth=10 m
information about lake depth is taken from the list of individual lakes or bathymetry
river, depth=3 m.
2.2. Tectonic Plates Map and geological maps
To allocate regions with kindred geological origin of lakes, the bottom-up approach was used. The main idea of this approach is to start from a description of the deepest layers of the Earth's crust, gradually elevating to the surface. We started from tectonic plates and cratons, then distinguished different types of rocks, and finally outlined deposits in the last geological period and boundaries of the permafrost. Information was obtained from Tectonic Plates Map and different geological maps of the world. In this study, we used maps from Physical Geography Atlas of the World published by ‘Academy of Science of the USSR and Head Office of Geodesy and Cartography of the USSR’ (PGAW, Citation1964).
Tectonic Plates Map shows forms of bedding, time and conditions of formation of structural elements of the Earth's crust. From this map, we outlined boundaries of tectonic plates, cratons, orogenies, volcanic plateaus, intercontinental rifts and fault areas. Fault areas were considered as the 1° zones around fault lines. We also outlined boundaries of the oceanic crust segments, uplifted above the sea level. Geological maps depending on their content and purpose include: stratigraphic maps, maps of Quaternary deposits, and geomorphologic maps. Stratigraphic maps show age, composition, origin and type of rocks bedding. From them, we outlined the boundaries of igneous, metamorphic and sedimentary rocks. Quaternary maps show location of the deposits of different origin in the last geological period. From these maps we outlined the boundaries of glacier activity areas, as well as areas of the marine and fluvioglacial Quaternary deposits. Other types of deposits were considered not to be relevant to origin of present lakes and these territories were assumed to be covered with terrigenous rocks. From a geomorphological map, which shows the main types of the land relief and its individual elements according to their origin and age, we outlined only the southern boundary of permafrost.
We digitised contours of regions by tools of geographic information systems. A form of digitised information was a vector, namely each contour was approximated by a polygon specified by coordinates of its vertices.
3. Methodology of allocating regions of similar geological origin of lakes
To allocate regions with the similar geological conditions, it was necessary to find intersections of the digitised contours. Hypothetically (mathematically), all intersections may exist and all combinations should be checked. With using of some a priory geographical knowledge, we could exclude many combinations. For example, there are no terrigenous Quaternary deposits in Iceland; hence, there are also no appropriate intersections in this area. For even existing intersections, sometimes there is no lake depth information in GLDBv.1. Hence, there is no practical reason to outline them. To find combinations, which for sure exist geographically and are useful in practice, we also engaged lake information. We developed and applied an innovative algorithm, which combines together both information about lake location and geological information.
Input data were: (i) digitised contours from Tectonic Plates Map and geological maps in ‘bottom-up’ order, and (ii) coordinates of individual lakes and/or gridded map of lakes from GLDBv.1. To specify if the lake point with the certain geographical coordinates belongs to the contour in question, we used a polygon test with a crossing number algorithm (Hormann and Agathos, Citation2001). If the point belongs to several contours, it means that these contours intersect. Coordinates of lake points may be known either from the dataset of individual lakes or from the pixel coordinates of the gridded lake map. For better statistical analysis, we processed data twice: both for the list of individual lakes and for the gridded lake map.
For every lake from the list of individual lakes with known mean depth and coordinates with the polygon test, we determined: (i) the tectonic plate and (ii) the craton which the lake in question belongs to, (iii) presence/absence of the permafrost, (iv) Quaternary deposits type and (v) type of rocks on the territory where the lake in question is located. Different combinations of information from (i) to (v) formed an individual code. These codes corresponded to intersections of contours, which we assume to be the regions with similar geological conditions of lake origin. A logical scheme to process data from the list of individual lakes is presented in . For pixels of the gridded lake map with known lake depth, we applied a similar algorithm. To process data automatically, we developed software with the Fortran90 programming language.
Fig. 1 Logical scheme to find existing intersections of contours from geological maps engaging data from the list of individual lakes.
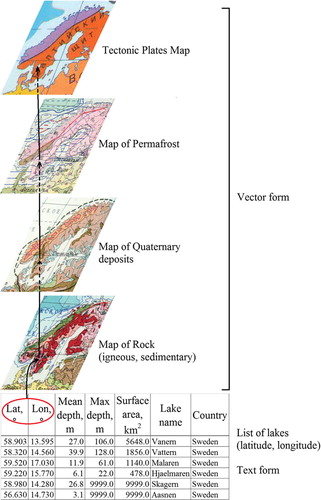
Altogether, we allocated 141 regions. The list of allocated regions is presented in the Appendix. Polygons corresponding to different tectonic plates, cratons, permafrost, Quaternary deposits and rock type, were overlapped. Contours of the allocated regions were obtained by tools of geographic information systems and used further for visual analysis. gives an example of the allocated region: Region No. 2 from the list in the Appendix (black on the map) belongs to the group of the North American Plate, the Cadomian and Caledonian Orogenies, with igneous rocks covered by glacial Quaternary deposits, and in the region there is no permafrost.
Fig. 2 Geographical location of Region No. 2 from the list in the Appendix. Region in question is marked with black on the map.
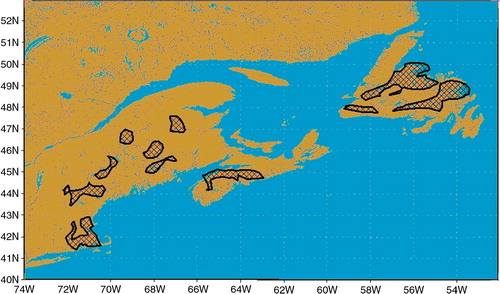
4. Evaluation of the typical lake depth
4.1. Expert evaluation based on statistical analysis
To find the typical lake depth for allocated regions, statistics from the GLDBv.1 was gained and analysed. The core of statistical analysis was building histograms of the lake depth for each region. Maximum of the histogram (the most probable value) was considered to be a guess for the typical lake depth for the region in question. Later on, starting from this guess, an expert decision about the typical lake depth was reached. Statistics were collected both from the list of individual lakes and from pixels of the gridded lake map, bearing in mind that statistics from lake pixels indirectly takes into account lake areas. Lists of lakes for each region were also compiled, as they were helpful in arriving at an expert decision.
The assumption about common geological origin of lakes in allocated regions is of course rather rigid. There could be some lakes in a region which had been formed differently from the majority of neighbouring ones. We did our best to exclude them from our analysis. For that, we performed our analysis in several steps. First, we analysed full statistics collected from the list of individual lakes and from the pixels of the gridded lake map. If there were enough data in GLDBv.1 and maximums of histograms for individual lakes and for lake pixels agreed well, the expert decision about the typical lake depth could be arrived at quite easily. Very different maximums of histograms indicated situations of non-typical large lakes located in the region. To cope with these situations, we also collected filtered statistics, with excluded large lakes, and built the appropriate histograms. For filtering, we used a criterion of the lake surface area to be more than 200 km2. This threshold value was based on practical reasons. First, the mean depth of most lakes like this is known, while measurements for smaller lakes are rather rare. Second, large lakes are often of more complex origin. They may occupy a particular geological structure that is not typical for the region. Usually they are older and have passed through the numerous cycles in their evolution. It makes them non-representative for the given statistical subset of lakes. For each of the 141 regions, four histograms were built and then analysed: for individual lakes and for lake pixels, with full data and with filtered data, making up 564 histograms in total. Statistics was collected using specially developed Fortran90 software. The expert decision about typical lake depth was reached individually for each region on the basis of the obtained statistics. Here we give some examples of expert evaluation of the typical lake depth.
The first example is Region No. 2 from the list in the Appendix displayed in (the North American Plate, the Cadomian and Caledonian Orogenies, with glacial Quaternary deposits on igneous rocks, no permafrost). First, we specify how many lakes and lake pixels are in the region: here, 17 lakes and 1139 lake pixels. Second, we give a short geographical description of the region: it consists of several rather small fragments located in different parts of the Island of Newfoundland and on the seashore between the Gulf of Maine and the City of Providence. Third, we describe lakes in the region qualitatively, using the list of individual lakes for this region: here, five lakes are deeper than 10 m, four lakes are larger than 10 km2, and one lake has missing data on the lake area; small lakes (less than 3 km2) are generally dominant. The fourth step is statistical analysis, with full and filtered statistics for individual lakes and pixels of the gridded lake map (). A maximum of the histogram for individual lakes corresponds to the depth of 3 m, while that for lake pixels – to 50 m. This is because of a large lake which dominates in pixel statistics and which is, in fact, partly located in the neighbouring region. Since the maximums of the histograms are too far from each other, a sure decision cannot be reached on the basis of full statistics only. So we consider filtered statistics, only for lakes with the surface area less than 200 km2. There are 17 such lakes, the number of corresponding lake pixels is 417. The maximum of the histogram for individual lakes still corresponds to 3 m, but for lake pixels it changes to 7 m. Finally, the expert decision about the typical lake depth in Region No. 2 is 7 m. This is the secondary maximum of the full data histograms and the primary maximum of the filtered data lake pixel histogram.
Fig. 3 Lake depth histograms for Region No. 2 from the list in the Appendix, for individual lakes (upper row), and for lake pixels on the gridded map (lower row), with full statistics (left column), and with filtered statistics, for lakes with the surface area <200 km2 (right column).
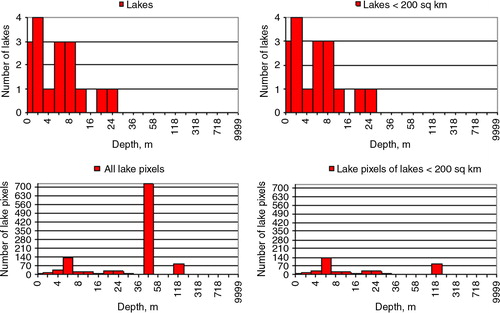
The second example is Region No. 130 from the list in the Appendix displayed in . It is located on the Eurasian Plate, on the Precambrian Shield, there are fluvioglacial deposits on sedimentary rocks and no permafrost. Four histograms, with full and filtered statistics, for individual lakes and lake pixels are displayed in . From full statistics, the maximum of the histogram for individual lakes corresponds to the depth of 7 m, but for lake pixels – of 18 m. This is probably due to a large lake, not typical for the region. Filtered statistics helped us to come to an expert decision about typical lake depth in this region of 7 m.
Fig. 4 Geographical location of Region No. 130 from the list in the Appendix. Region in question is marked with black on the map.
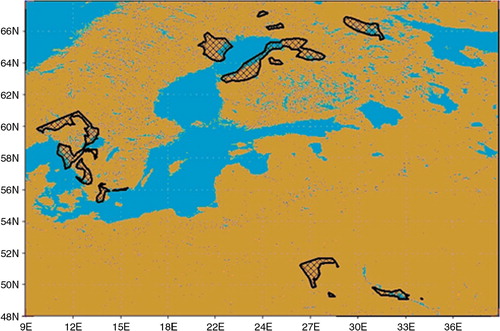
Fig. 5 Lake depth histograms for Region No. 130 from the list in the Appendix, for individual lakes (upper row), and for lake pixels on the gridded map (lower row), with full statistics (left column), and with filtered statistics, for lakes with the surface area<200 km2 (right column).
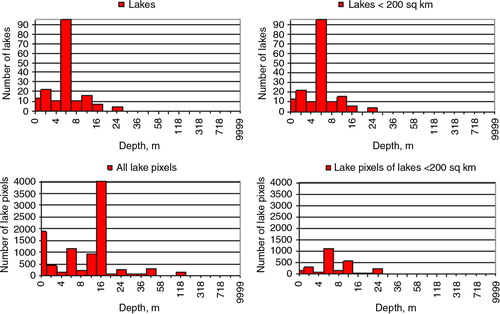
In general, statistical analysis was enough to reach a decision about the typical lake depth in a region. However, in some cases it was difficult to obtain a confident estimation on the basis of statistical analysis only; in this situation, we used the method of analogies. Also, geographical and improved geomorphologic methods were used where it was necessary and possible.
4.2. Method of analogies
We used this approach when there were no sufficient statistics about the lake depth for one region, but enough for other regions with similar geological and geomorphologic structure. The main idea of the method is extrapolation of statistics using certain geological knowledge. From this knowledge, we specified regions–analogues as follows: (i) regions with glacial, marine and fluvioglacial Quaternary deposits of one tectonic plate, (ii) cratons of one tectonic plate, (iii) Precambrian shields of different tectonic plates, (iv) orogenies of one tectonic plate. We did not combine the orogenies of one tectonic plate, but used this information to assess reliability of depth estimates.
In addition, in expert evaluation sometimes we used morphological and geographical information to consider properties of the regions (shape of lakes, elevation, surrounding vegetation, etc.). For two regions with very shallow lakes (Region No. 110 located in Southern Urals and in the north-eastern part of China and Region No. 115 located at the British and the Netherlands’ coast of the Northern Sea), we modified the typical lake depth of 1 m, given by statistical evaluation. Usually, when a lake is 1 m deep, either its bottom is visible on images or its surface in summer is covered by macrophytes. Very often, shallow lakes dry up during the summer period. From careful visual analysis of satellite images provided by Google Earth and based on Landsat and other high-resolution sensors, this was not the case for these regions. So, the typical lake depth there was estimated to be slightly larger, that is, 2 m.
4.3. Improved geomorphologic method for North East of Europe
This method (Doganovsky, Citation2006, Citation2012) was developed only for Northwest Russia and was applied here for the middle size lakes (>10 km2). This method is also based on information about geological origin of lakes. The lake volume (from which the mean lake depth may be derived) is estimated from a statistical dependency on the lake area, and this dependency is different for regions with different geological situation. To draw contours of these regions, characteristics of many known lakes with different geographical locations were analysed. Contours were drawn on the basis of knowledge about lake origin well documented for this area. Four regions were distinguished: I) region with glacial–tectonic lakes, II) lowland region with glacial–accumulative lakes, III) region with hilly–moraine glacial–accumulative lakes, IV) region with ancient glacial–accumulative lakes formed after the second glacial epoch (170–125 ka). Using the large dataset of in situ measurements of morphological parameters of lakes in Doganovsky (Citation2012) and Doganovsky (Citation2006), the relation between the water volume (W) and the surface area (F) was established:1
where y=log10 (W+1), x=log10 (F+1), a is a parameter, dependent on the typical lake size on the territory, and m is a parameter, dependent on geological characteristics of the territory. Values of a and m for each region are given in . Therefore, from a given lake area, we can estimate the lake volume and the mean lake depth h by inverting eq. (1):2
Table 1. Parameters a and m in formula (1)
where h is given in m, and F is in km2.
We considered this method to have high reliability and gave it the highest priority on the appropriate territory. The development of this method is being continued for other territories.
4.4. Geographical approach
This method was developed by Kitaev (Citation1984) for the boreal zone. It implies that the mutual distribution of lake parameters is specific for each geographical zone. Geographical zones of tundra, northern taiga, middle taiga and mixed forest were considered. This approach is not related to lake origin, although it may implicitly account for morphology of the territory through the dependency of vegetation on lithology of a rock or permafrost conditions. The distributions of the mean lake depth for different zones depending on the lake area are generalised and represented in . This method has very low reliability and we gave it the lowest priority. It was used for the territories where neither our statistical expert evaluations, nor improved geomorphologic method were possible. A specific case was Region No. 110 located in Southern Urals and in the north-eastern part of China (it belongs to the Eurasian Plate, sediments on the Paleozoic craton, with terrigenous deposits on sedimentary rocks without permafrost). In Southern Urals (to the north from 50° latitude), the priority was given for the geographical method: here post-permafrost lakes prevail, and the geographical method was developed especially for them.
Table 2. Lake mean depth, m, depending on lake area for different geographical zones
The geographical zones were defined using the landscape map of SAW (Citation1990). As legends used there and in Kitaev (Citation1984) were different, in some cases we combined or split different landscape types to obtain better coherence. We merged together six different types of tundra landscapes, five different taiga landscape types and five mixed forest landscape types. We split taiga into northern and middle parts along a polar circle. Mountain zones were excluded.
To improve the accuracy and reliability of this method, we verified it and improved it using data from GLDBv.1. We generated analytical equations approximating statistical dependencies and used them instead of tables. For some geographical zones, we used equations based on statistics from GLDBv.1, while for others, where data in GLDBv.1 were scarce, we used data based on information from Kitaev (Citation1984). Equations for different zones with references for data sources are given in .
Table 3. Equations for different geographical zones used in geographical method (F is the lake surface area, km2; h is the mean lake depth, m)
For both geomorphological and geographical methods, it was necessary to know the surface area of each lake F. To obtain it, we summarised pixels of GLDBv.1 raster map for each lake, bearing in mind that a 30 arc sec. pixel area S in longitude–latitude projection is:3
where 0.86 km2 is the pixel area on the Earth's equator, and ϕ is the latitude.
5. New product and discussion
Indirect estimates of the mean lake depth from their geological origin where appended to GLDBv.1 and the new raster map of the world GLDBv.2 was produced. Technically, the new map was combined from GLDBv.1 and three auxiliary maps of the world, containing the lake depth estimates from our expert evaluation, improved geomorphologic and geographical methods (see Section 4) accordingly. The additional map from GLDBv.1 containing coded information about sources of data was used for flagging and then updated. Values for pixels with code 3 (see Section 2) were just copied from the old map to a new one. For them the lake depth was measured and no indirect estimates were needed. The same was done for code 4 (river). For codes 1 and 2, we applied the following procedure: (i) if a geomorphologic method was possible, we specified the mean lake depth from it (this situation was coded by digit 7); if not, then (ii) if estimations from our expert evaluation were possible, we used them (this was coded by digit 5); if not, then (iii) if a geographical method was possible, we specified the mean lake depth from it (digit 6); if not, then (iv) we used the default depth=10 m and codes 1 or 2 accordingly. Exception from this rule was Region No. 110, for a section of it, the priority was given to the geographical method (see Section 4.4 for an explanation).
As a result, two new global raster maps were produced. The first map contains gridded information about lake depth including indirect estimates (or predictions). The second map contains information about sources of data coded with the following legend:
no lake or river (sea or land), depth=0 m
no information about this lake in the list of individual lakes, depth=10 m
missing lake depth in the list of individual lakes, depth=10 m
information about lake depth is from the list of individual lakes or bathymetry
river, depth=3 m
lake depth is estimated by our expert evaluations
lake depth is estimated by the geographical method
lake depth is estimated by the geomorphologic method.
and give an example of the new product in comparison with the old one, for the territory close to Hudson Bay in North America. In GLDBv.1, the lake depth for unknown lakes is 10 m (code 1 on A and 7A). In GLDBv.2, the lake depth is smaller in the north-western part and larger in the south-western part of the region. In the south-western part (along the Gulf of St. Laurence), lakes have mostly tectonic origin, so they are supposed to be deep. This is in accordance with our estimations and modifications of the lake depth here from 10 m in GLDBv.1 to 22 m in GLDBv.2.
Fig. 7 Fragments of the global gridded map with coded information about origin of lake data (see legend in text, Section 5). (a) GLDBv.1; (b) GLDBv.2.
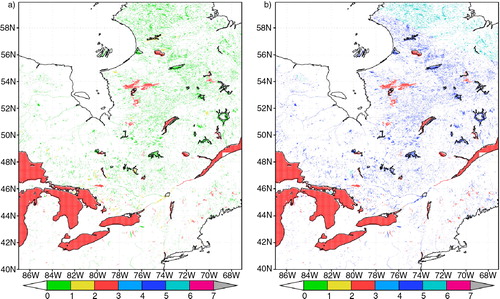
Currently, we have no additional dataset to verify or validate our product. Cross-validation is also impossible, because of data scarcity for many regions. Our estimates are noticeably subjective and basically difficult to verify. Nevertheless, we evaluated our product qualitatively, as in the example presented above. We suggest that the new version of the GLBD is more realistic as it provides physically-based estimations without contradiction to the seldom real lake depth measurements. In future, when the new data is available, validation will be performed as well as improvements being made. Another way to validate the new product is indirect. We may compare modelling results of the lake surface temperature with remote sensing observations. The main problem with this approach is errors in remote sensing data, which on the global scale may be large and should be carefully filtered out. This validation is also planned.
6. Sensitivity of the modelling results
To demonstrate the sensitivity of the modelling results to the corrections of the lake depth field, the lake model climatology (CitationKourzeneva et al., 2012b) was used. This climatology was developed to initialise the lake model variables in operational NWP model runs of so-called ‘cold’ start. The climatology is obtained by the 20-yr off-line runs of the two-layer bulk lake model FLake (Mironov, Citation2008). This model uses a self-similar representation (an assumed shape) of the temperatures profile in a lake including the mixed layer and the thermocline. The model also contains the ice module, the snow module and the bottom sediments module. The bottom sediments module was switched off in climatologic runs to save computational time and because of low sensitivity of the lake surface temperature to it. The snow module was also switched off, because it had not been yet enough validated at that moment. For atmospheric forcing, the global dataset described in Sheffield et al. (Citation2006) was used. The product is presented on the grid in geographical coordinates (longitude and latitude) with 1° resolution. Simulations were performed for 12 hypothetic lakes with depths varying from 1 to 50 m in each grid box. Modelling results were averaged in time for 10-d intervals. The grid of a certain NWP model may have different resolution and map projection than the grid of the lake climatology product. Thus, lake information must be projected from one grid to another with the closest neighbour interpolation method. During this step, data about real lake depth on the target NWP model grid are applied, see CitationKourzeneva et al. (2012b) for details.
The sensitivity of the lake modelling results to modifications in GLDB is demonstrated with the climate fields of the mean water temperature, the surface water temperature and the thickness of ice for different seasons. The target grid in this example is in geographical coordinates (longitude and latitude) with a horizontal resolution of 0.02°. The domain contains part of North America with Hudson Bay where many modifications in GLDB have been done (see and ). A and A represent fields of the lake mean water and surface temperature for the second decade of August calculated using the new lake depth dataset. B and 9B represent the difference, if the lake depth from GLDBv.2 and GLDBv.1 is used. Negative difference means colder temperatures with GLDBv.2; it mainly corresponds to deeper lakes. The difference both in terms of the mean water and surface temperature may reach 5°C. Ice conditions are sensitive to the modifications in GLDB mainly in autumn, since the lake depth controls an onset of the ice season. The difference between two simulations in terms of the ice thickness for the first decade of December reaches 50 sm (). Negative difference means less ice with GLDBv.2: many lakes, which were ice covered with the old version of GLDB, are ice-free with the new version. These lakes are deeper in GLDBv.2.
Fig. 8 Lake mean water temperature, °C, for the second decade of August, if the lake depth is extracted from GLDBv.2 (a), and the difference, if the lake depth from GLDBv.1 is used (b).
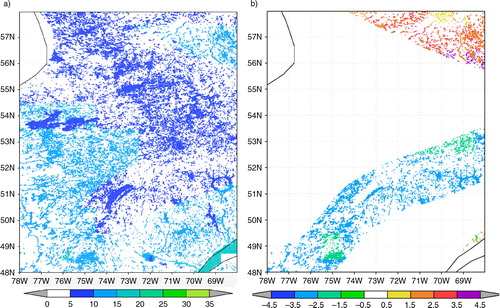
7. Conclusion
Lakes influence local weather and the regional climate through the surface turbulent fluxes and fluxes of radiation. To parameterise them in NWP and climate modelling, external parameters are needed. The main objective of this study was to upgrade the existing GLDBv.1 (CitationKourzeneva et al., 2012a) by estimations (predictions) of the mean lake depth depending on their geological origin and to produce the new version of the database.
For that, we outlined the regions with similar geological and geomorphologic history suggesting similar origin of lakes there, and proposed the typical lake depths for them using expert evaluations on the basis of the statistics from the first version of the database GLDBv.1 and involving other sources of information. In addition, geomorphologic (Doganovsky, Citation2006) and geographical (Kitaev, Citation1984) methods were used where necessary and possible. We produced the new version of the database GLDBv.2, which includes estimates of the typical mean depth for the boreal lakes. Final products include the global gridded map of lake depth with the resolution of 30 arc sec. containing also indirect estimates (predictions) of the mean lake depth, and the updated list of individual lakes now containing ca. 13500 lakes.
Apart from continuous maintenance of GLDB by updating the list of individual lakes, the further developments could be proposed: (i) Extend this study and obtain the typical mean depth estimates for lakes outside boreal climate zone. (ii) Add bathymetry for more large lakes. (iii) Increase the map resolution by using different global raster maps – different ecosystem datasets.
8. Acknowledgements
The authors thank Yurii Batrak and Suleiman Mostamandi (Russian State Hydrometeorological University), as well as Pavel Andreev (North-West Interregional Territorial Department of the Federal Service for Hydrometeorology and Environmental Monitoring) for useful tips and discussions. Two anonymous reviewers made many useful comments. The project was made possible due to the support from ECMWF.
References
- Amante C. , Eakins B. W . ETOPO1 1 Arc-minute global relief model: procedures, data sources and analysis. NOAA Tech. Memorand. NESDIS NGDC-24. 2009; 3: 1–19.
- Balsamo G. , Dutra E. , Stepanenko V. M. , Viterbo P. , Miranda P. M. A. , co-authors . Deriving an effective lake depth from satellite lake surface temperature data: a feasibility study with MODIS data. Boreal Environ. Res. 2010; 15: 178–190.
- Canfield Jr, D. E. , Langeland K. A. , Linda S. B. , Haller W. T . Relations between water transparency and maximum depth of macrophyte colonization in lakes. J. Aquat. Plant Manage. 1985; 23: 25–28.
- Champeaux J.-L. , Han K.-S. , Arcos D. , Habets F. , Masson V . Ecoclimap2: a new approach at global and European scale for ecosystems mapping and associated surface parameters database using SPOT/VEGETATION data – First Results. Int. Geosci. Remote Sens. Symp. 2004; 3: 2046–2049.
- Doganovsky A . Spatial patterns of lake depressions. Proceedings of the Conference “LIX Herzen Readings”, Geography and Allied Sciences, Saint-Petersburg . 2006; 15–23. (in Russian).
- Doganovsky A . External water exchange of lakes as the integral indicator of water body types. Limnol. Rev(2012). 2012; 12(1): 11–17. 10.2478/v10194-011-0040-2.
- Eerola K. , Rontu L. , Kourzeneva E. , Shcherbak E . A study on effects of lake temperature and ice cover in HIRLAM. Boreal Environ. Res. 2010; 15: 130–142.
- Hormann K. , Agathos A . The point in polygon problem for arbitrary polygons. Comput. Geom. Theory Appl. 2001; 20: 131–144.
- Kitaev S . Ecological Basis of Lakes’ Bioproduction in Different Landscapes. 1984; 208 Moscow: Nauka. 16–19. (in Russian).
- Kondratiev S . External Parameters for the Parameterization of Lakes in NWP and Climate Modeling. 2010. Diploma Thesis, monography, Saint-Petersburg, Russia, 51 (in Russian).
- Kourzeneva E . Bouttier F. , Fischer C . Global dataset for the parameterization of lakes in numerical weather prediction and climate modelling. ALADIN Newsletter. 2009; Toulouse, France: Meteo-France. 46–53. July–December.
- Kourzeneva E. , Asensio H. , Martin E. , Faroux S . Global gridded dataset of lake coverage and lake depth for use in numerical weather prediction and climate modelling. Tellus A. 2012a; 64: 15640. 10.3402/tellusa.v64i0.15640.
- Kourzeneva E. , Martin E. , Batrak Y. , Le Moigne P . Climate data for parameterisation of lakes in numerical weather prediction models. Tellus A. 2012b; 64: 17226. 10.3402/tellusa.v64i0.17226.
- Lee R. W. , Rast W . Light attenuation in a shallow, turbid reservoir, lake Houston, Texas. U. S. Geological Survey: Water-Resources Investigation Report, Austin, Texas, . 1997; 97–4064.
- Mironov D . Parameterization of Lakes in Numerical Weather Prediction. Description of a Lake Model. 2008; 11 Offenbach am Main, Germany: Deutscher Wetterdienst. 41. COSMO Technical Report.
- PGAW (Physical Geography Atlas of the World). Academy of Science of the USSR and Head Office of Geodesy and Cartography of the USSR. 1964; Moscow: Academy of Science of the USSR and Head Office of Geodesy and Cartography of the USSR. (in Russian).
- Samuelsson P. , Kourzeneva E. , Mironov D . The impact of lakes on the European climate as simulated by a regional climate model. Boreal Environ. Res. 2010; 15: 113–129.
- Sheffield J. , Goteti G. , Wood E. F . Development of a 50-year high-resolution global dataset of meteorological forcings for land surface modelling. J. Clim. 2006; 19(13): 3088–3111.
- SAW (Small Atlas of the World). Head Office of Geodesy and Cartography Under the Council of Ministers of the USSR. 1990; Moscow: Head Office of Geodesy and Cartography under the Council of Ministers of the USSR. (in Russian).
- Tranvik L. J. , Downing J. A. , Cotner J. B. , Loiselle S. A. , Streigl R. G. , co-authors . Lakes and reservoirs as regulators of carbon cycling and climate. Limnol. Oceanogr. 2009; 54(6, part 2): 2298–2314.
- Walter K. , Smith L. , Chapin III F. . Methane bubbling from northern lakes: present and future contributions to the global methane budget. Philos. Trans. R. Soc. A. 2007; 365: 1657–1676.
9. Appendix
List of allocated regions with kindred geological origin of lakes and the typical mean depth h, m.