Abstract
Fungal taxonomy and ecology have been revolutionized by the application of molecular methods and both have increasing connections to genomics and functional biology. However, data streams from traditional specimen- and culture-based systematics are not yet fully integrated with those from metagenomic and metatranscriptomic studies, which limits understanding of the taxonomic diversity and metabolic properties of fungal communities. This article reviews current resources, needs, and opportunities for sequence-based classification and identification (SBCI) in fungi as well as related efforts in prokaryotes. To realize the full potential of fungal SBCI it will be necessary to make advances in multiple areas. Improvements in sequencing methods, including long-read and single-cell technologies, will empower fungal molecular ecologists to look beyond ITS and current shotgun metagenomics approaches. Data quality and accessibility will be enhanced by attention to data and metadata standards and rigorous enforcement of policies for deposition of data and workflows. Taxonomic communities will need to develop best practices for molecular characterization in their focal clades, while also contributing to globally useful datasets including ITS. Changes to nomenclatural rules are needed to enable validPUBLICation of sequence-based taxon descriptions. Finally, cultural shifts are necessary to promote adoption of SBCI and to accord professional credit to individuals who contribute to community resources.
Introduction
Fungi make up an underdescribed, poorly documented clade of eukaryotes that have immense ecological and economic impacts. Many fungi are microscopic or have cryptic life cycles that make detection difficult. Approximately 135 000 species of fungi have been described, but the actual diversity of the group is likely to be in the millions of species (CitationAmend et al. 2010, CitationDavison et al. 2015).
Analyses of environmental DNA and RNA sequences may involve two complementary but distinct activities: Sequence-based classification (SBC) and sequence-based identification (SBI) (CitationHerr et al. 2015). The goals of SBC are to discover, name, and classify fungal species according to their phylogenetic relationships. In contrast, SBI uses the products of taxonomy to identify individuals and determine the composition of fungal communities with reference to existing classifications. SBI is a central element of ecological studies, including metatranscriptomic studies of community-level metabolic processes. Collectively, sequence-based classification and identification (SBCI) denotes the full range of activities required to detect and characterize fungi in the environment based on nucleic acid sequences ().
Table I. Terms and concepts for sequence-based classification and identification
New resources for SBCI are required to fully exploit the staggering volume of data flowing from fungal molecular ecology studies using high-throughput sequencing technologies. Huge numbers of undescribed taxa known only from environmental sequences need to be classified and linked to phenotypic, ecological, and functional traits. This article aims to: (i) envision the potential of SBCI and identify its conceptual challenges; (ii) survey current resources for SBCI in fungi and assess their strengths, limitations, and opportunities for enhancement; and (iii) consider options for integrating sequence-based fungal species into taxonomic systems based on specimens and cultures.
Goals and conceptual challenges of SBCI
In the ideal model of SBCI it would be possible to submit sequences of any nucleic acids from specimens or environmental samples to appropriate databases and retrieve lists of taxa with information on their relative abundance, phylogenetic relationships, ecological roles, and metabolic properties. The reference databases themselves would become richer as the results from each new study were integrated, creating new knowledge about fungal diversity, biogeography, population structure, and functional biology (). However, current methods of SBCI are based almost entirely on analyses of PCR-amplified nuclear rRNA genes, particularly the internal transcribed spacer (ITS) region, and they draw on incomplete taxonomic and functional databases. New environmental data are not systematically integrated with existing resources. Here, we list six general challenges to achieving the model of SBCI described above; subsequent sections describe these challenges and the actions required to overcome them: (i) develop community standards for taxon recognition based on sequence data; (ii) create and curate sequence databases and analytical tools for SBCI; (iii) link sequence data to phenotypic data, including data from type specimens; (iv) achieve reproducibility in studies utilizing SBCI; (v) encourage the scientific and lay communities to adopt SBCI; and (vi) accord professional credit for contributing resources for SBCI.
Fig. 1. Conceptual diagram of Sequence-Based Classification and Identification (SBCI) in fungi. The upper part of the figure shows traditional mycological data streams originating with cultures and collections (green boxes), whereas the lower part indicates environmental molecular sampling (blue boxes). SBCI is included in the integrating middle layer (red arrows), with outputs including synthetic understanding of community composition and functional biology and contributions to taxonomic and functional databases. Metadata not indicated in the diagram include temporal and geographic information or host information, which could contribute to fields such as ecological niche modeling, biogeography, and epidemiology. Metaproteomics and non-molecular aspects of functional biology (e.g. morphology and development) are also not shown, but could be integrated into SBCI and used to predict phenotypic properties of species detected with environmental sequences.
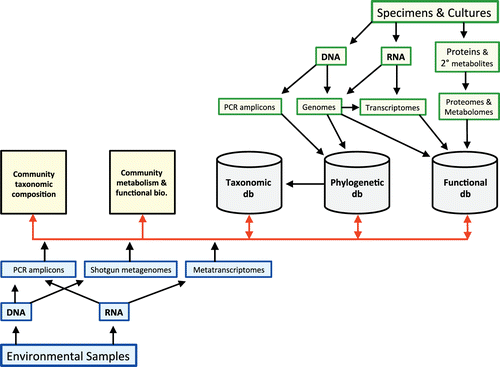
Surveying the landscape: current resources and needs for SBCI in Fungi
Some of the earliest applications of comparative molecular data in fungi were to identify environmental samples that lacked the morphological characters necessary for traditional taxonomic identification (CitationBidartondo 2008) and insufficiently identified sequences (CitationParr et al. 2012, p. 2013) that reside in the International Nucleotide Sequence Database Collaboration (INSDC), with its three partners: GenBank at the National Center for Biotechnology Information (NCBI), the European Nucleotide Sequence Archive of the European Molecular Biology Laboratory (EMBL), and the DNA Data Bank of Japan (DDBJ) (CitationCochrane et al. 2016).
Table II. Databases and tools for sequence-based classification and identification
Specialized databases and general resources for SBCI
A number of databases and tools have been developed to enable high-throughput analyses and address the problems caused by misidentified or insufficiently identified sequences. One of the first such efforts was the Ribosomal Database Project (RDP) (CitationLarsen et al. 1993, CitationMaidak et al. 1994), which initially consisted of nuclear small subunit (SSU) rRNA gene sequences from Archaea, Bacteria, and Eukarya. The RDP has evolved since its inception, providing alignments, trees, and similarity assessment tools, based on its core database of curated sequences. Recent additions to RDP include new databases for fungal ITS and large subunit (LSU) rRNA genes (CitationCole et al. 2014).
An innovation of the RDP is the naïve Bayesian classifier, which has been implemented for both the bacterial and archaeal small subunit (16S) rRNA genes (CitationLiu et al. 2012) and ITS (CitationCaporaso et al. 2010) and Mothur (CitationCole et al. 2014). In addition, RDP has made available versions of the Classifier trained on the UNITE database (described below) including an updated training set named to honor the Australasian mycologist J.H. Warcup (CitationParberry and Robertson 1999). The Warcup training set (2.0) is available from the RDP website and contains 8551 species and 17 878 ITS sequences organized into taxonomic classifications. Both sets performed well in testing (CitationDeshpande et al. 2015).
The UNITE database was initially developed for ITS sequences of ectomycorrhizal fungi (CitationAbarenkov et al. 2010, CitationKõljalg et al. 2013). UNITE is the product of a consortium of fungal ecologists, taxonomists, and bioinformaticians. Queries can be performed with reference to the custom-curated UNITE database, which includes many sequences from specimens that were collected and deposited by taxonomic specialists specifically for the purpose of building the database or all INSDC sequences. Only about 0.5% of the 657813 sequences in UNITE have yet to be submitted to the INSDC databases but all can be searched online.
UNITE groups sequences into “Species Hypotheses” (SH), which are generated by a two-tier clustering process, first at the subgeneric/generic level then at the species level (CitationKõljalg et al. 2013). Sequences are grouped into SHs based on similarity to a reference sequence at a particular similarity cutoff (e.g. 97%, 98%, etc.). All SHs have a unique digital object identifier (DOI) to promote unambiguous communication. The UNITE database has also been adapted for use in QIIME and Mothur and a range of other applications (https://unite.ut.ee/repository.php).
The Barcode of Life Database (BOLD) and its European mirror, EUBOLD, provides sequence based identification tools (CitationRatnasingham and Hebert 2007). However, BOLD retains a strong focus on animal identification. Although it contains more than 117 000 ITS sequences with just under 100 000 sequences tied to voucher data, more than 84% of these were obtained from thePUBLIC INSDC databases. Coverage for “Fungi and other organisms” is just over 16 000 species, compared to approximately 154 000 species for Animals and 58 000 for Plants. Currently, NCBI will only assign a BARCODE keyword to sequences that meet all BOLD criteria, including the deposition of original sequence trace files. Direct submissions to BOLD allow for trace submission and although it is technically feasible at NCBI as well, no fungal sequences have yet been tagged with the official BARCODE keyword in the INSDC. At the same time, the term “DNA barcode” has been widely applied as a generic descriptor in mycology for an ITS sequence tied to voucher data.
The RefSeq Targeted Loci Project is a relatively new resource at NCBI. This is part of the curated microbial resources in the Reference Sequences databases (CitationO’Leary et al. 2015, CitationTatusova et al. 2015). RefSeq ITS highlights significant sequences that do not meet all the strict DNA barcode requirements but that could act as references for SBCI. RefSeq ITS accessions are selected from GenBank records that pass a rigorous set of standards and can be curated by third parties (CitationSchoch et al. 2014). Some entries have already been shared between RefSeq and UNITE and a formal pipeline to facilitate this process is in development.
RefSeq Targeted Loci will remain focused on sequences from type material, with a few exceptions for sequences from verified voucher material for economically important species or poorly represented groups. It currently contains data from the three most commonly used ribosomal regions. The most comprehensive RefSeq dataset contains 4779 ITS accessions. It is intended that this resource will increase representation and expand into other commonly used marker sequences with continued involvement of other databases and the research community. Whole genome sequence data from type material is one area of expansion and is already being applied for bacteria at NCBI. This is incorporated in a broader effort to add type material identifiers to the NCBI Taxonomy database, which allows use of BLAST and other tools to search multiple databases for reliable entries that are specifically tied to type material (CitationFederhen 2015).
The projects described above provide diverse tools for analyzing environmental sequences, and new resources are being produced regularly. For example, a database of ITS sequences for medically important species was recently released by the International Society for Human and Animal Mycology (ISHAM) (CitationIrinyi et al. 2015) and is now linked in NCBI and UNITE.
SBCI using alternative markers
ITS has emerged as the primary marker for fungal barcoding (CitationBruns et al. 1998). A current resource using alternative markers is the MaarjAM database (CitationÖpik et al. 2010) of the arbuscular mycorrhizal (AM) Glomeromycota, which emphasizes SSU rRNA gene sequences. MaarjAM applies “virtual taxon” (VT) identifiers connected to curated “type sequences” and it currently contains ca. 22 000 accessions of SSU rRNA sequences linked to ca. 400 VT, 2000 ITS, and 2000 LSU sequences (CitationÖpik et al. 2014). MaarjAM also includes curated information on sequence origin (ecological metadata), which is increasingly used to study AM fungal distribution patterns (CitationOhsowski et al. 2014). The inclusion of SSU rRNA gene sequences in MaarjAM reflects a historical preference for this region by AM fungal systematists (CitationKoren et al. 2013), it will become routine to obtain contiguous sequences containing ITS and its flanking LSU and SSU regions, which will facilitate integration of current datasets based on single markers.
Intragenomic heterogeneity among ITS copies poses challenges for SBCI, although an analysis using 454 sequencing of ITS amplicons in 99 diverse fungal isolates suggested that it may not be a major source of error in estimates of species richness (CitationLindner et al. 2013). Nevertheless, it is necessary to look beyond ITS in groups that are known to suffer from high levels of intragenomic heterogeneity, such as the polypore genus Laetiporus (CitationLindner and Banik 2011, CitationLindner et al. 2013). Another group in which ITS is a poor choice for SBCI is Fusarium, which possesses highly divergent non-orthologous copies of the ITS2 (CitationO’Donnell et al. 1998). ITS generally fails to resolve species boundaries in Fusarium that are discernible using other markers (CitationO’Donnell et al. 2015). This difficulty has led to the recommended use of multiple protein-coding genes for species-level classification in Fusarium, with the intron-rich 5′ end of translation elongation factor 1-alpha gene (tef1) being favored as the primary locus for species identification, followed by partial nucleotide sequences of DNA-directed RNA polymerase II largest (RPB1) and second largest subunit (RPB2). Accordingly, the FUSARIUM-ID and Fusarium MLST databases were created to facilitate identification in Fusarium via BLAST (CitationGeiser et al. 2004, CitationO’Donnell et al. 2010). The current versions of FUSARIUM-ID and Fusarium MLST contain multilocus data from 1366 isolates, with data from ten different protein-coding and ribosomal gene regions (CitationPark et al. 2010). Stand-alone multilocus databases have been developed for several other fungal groups, including TrichoBLAST for Trichoderma (CitationKopchinskiy et al. 2005) and multiple databases maintained at the CBS Biodiversity Centre focusing on Aspergillus, Morchella, Russula, dermatophytes, and indoor fungi.
Markers such as tef1 are superior to ITS for SBCI in some groups (CitationLutzoni et al. 2004). Conversely, ecologists using single-locus approaches should understand that ITS will enable only coarse identification in some clades, and they should be prepared to follow up with targeted analyses of other regions when species-level identification is critical.
Increasingly, data for SBCI are coming from whole genome sequencing studies, including those performed under the auspices of the 1000 Fungal Genomes Project (1KFG). Unfortunately, because of their repetitive nature, rRNA gene sequences are usually left unassembled in the sequence read archives (CitationBengtsson-Palme et al. 2013). Where marker sequences can be extracted from whole genome sequences of sufficient quality and provenance, they can also be incorporated into the RefSeq system. The Fungal Genomes Program of the DOE Joint Genome Institute (CitationGrigoriev et al. 2014) is now requiring ITS sequences as part of the metadata associated with samples for genome and transcriptome analyses. This information is requested to avoid sequencing of contaminants, but it could also be made available to link genomes with metagenomic data.
Barriers, inefficiencies, and unexploited opportunities
The resources described above have evolved independently, resulting in a diversity of useful but largely unintegrated tools, with sequences and their metadata imported from a variety of sources. Greater attention to data standards and formats will facilitate data integration and SBCI (CitationTedersoo et al. 2015). At present, many individual databases require unique input formats, different data fields, and a great deal of manual curation. There is also inconsistency in the format and quality of metadata provided for sequence-based diversity studies, whether the sequences are derived from specimens or directly from the environment.
Ambitious attempts are underway to integrate and standardize phenotype descriptions across all biology (CitationDeans et al. 2015). Fortunately, standards for formatting and minimum information have been developed and are available for adoption in SBCI. For example, the BIOM format (CitationMcDonald et al. 2012a) is widely used for transferring metadata from one source to another, while the MIMARKS (minimum information about a marker gene sequence) standard (CitationYilmaz et al. 2011) establishes core annotation items for metagenomics/microbiome studies. These core metadata are key for SBCI, because they provide information regarding the origin and quality of sequences. RDP has produced a MIMARKS-compliant Google Sheet for metadata management that can be exported into WebIN and Sequin accessible formats, facilitating submission to EMBL and GenBank. With a few minimal adjustments, these tools should suffice for the development of a recommended format and standards for sequence data and metadata for SBCI that would also be compatible with formats for metagenomic analysis pipelines, submission to sequence repositories, and many other uses.
Linkages between environmental sequences and metabolic and phenotypic traits are needed to make predictions about the biological properties of organisms that have not been observed directly. Functional predictions for entire communities and individual species could be made by comparing environmental sequences to whole genomes. However, phenotypic data pose special challenges because they are diverse and are not archived in centralized databases. Apart from a few pioneering efforts, including MycoBank and the MycoPortal of the Macrofungi Collection Consortium, phenotypic information is generally scattered. An example of the sort of tools that are needed is the recently developed FUNGuild database and its associated bioinformatics resources (CitationNguyen et al. 2016), which make it possible to parse large numbers of environmental sequences into broad trophic guilds (e.g. saprotrophs vs. mycorrhizal fungi). Resources like FUNGuild have the potential to integrate SBCI with phenotypic and ecological traits, such as enzymatic activity (CitationTalbot et al. 2015).
Building phenotypic databases will require an increased focus on specimen and culture annotations using a standardized format that can be traced across multiple databases and electronically availablePUBLICations. To take advantage of SBCI, these databases must be extended to include information on geographic distribution and habitat gained from environmental sequencing. Where possible, databases should use the Darwin Core data standards, which promote interoperability among biodiversity information resources (CitationWieczorek et al. 2012).
The lack of linkages among sequences, alignments, and phylogenies is also a limiting factor for SBCI. Phylogenetic inference is laborious and requires expert decisions about data inclusion and analytical settings. Delimitation of taxa by systematists is based on tree topologies and often involves consideration of morphological characters and other evidence such as genealogical exclusivity (CitationTaylor et al. 2000), not merely sequence similarity. In contrast, SBCI as it is practiced now, is usually based only on pairwise sequence comparisons, with uniform (but adjustable) clustering criteria applied across all taxa. Phylogeny-based approaches to OTU delimitation have promise, such as the EPA-PTP (evolutionary placement algorithm-poisson tree process) method, which incorporates tree inference using RAxML (CitationZhang et al. 2013).
SBCI could be enhanced if phylogenetic trees and tree-based taxon concepts were incorporated into the pipelines used to analyze environmental sequences. The basic tools for tree-based SBCI already exist, such as lowest common ancestor (LCA) algorithms that can determine clade contents, as implemented in the (now defunct) mor pipeline for automated phylogenetic taxonomy of Agaricomycetes (CitationHibbett et al. 2005). Unfortunately, the input data for such analyses, alignments, and phylogenies are largely unavailable. An analysis by the Open Tree of Life Project found that only about 17% of published fungal phylogenies are available in electronic format (CitationDrew et al. 2013), even though many mycological journals have stated requirements that datasets and trees be submitted to TreeBASE or Dryad. One reason that submissions have lagged is that data submission is tedious, but lack of editorial oversight is also to blame. To facilitate the future development of tree-based SBCI that can take advantage of “gold standard” taxon delimitations by expert systematists, it will be necessary to create user-friendly tools for uploading phylogenetic trees to databases and for journals to enforce existing policies regarding data deposition. Development of informatics resources for tree storage is underway as part of the Open Tree of Life Project (CitationHinchliff et al. 2015).
Lessons from prokaryotes
Microbiologists have developed rich databases and sophisticated informatics tools for SBCI, as well as broadly accepted standards for molecular taxon description. This section describes some of the major initiatives in SBCI of prokaryotes, including successful efforts that mycologists would be wise to emulate and pitfalls to avoid.
Prokaryotic taxonomy, informatics, and nomenclature
Under the International Code of Nomenclature of Prokaryotes (ICNP) (CitationParker et al. 2015) 16S rRNA gene sequences are required for the description of new species, with a defined cutoff of <97% similarity between species (CitationMende et al. 2013). Deposition of a type culture is also required.
The adoption of 16S rRNA genes as the primary taxonomic marker for Bacteria and Archaea greatly facilitated the discovery and documentation of prokaryotic diversity and resulted in a dramatic increase in the number of newly described species. Molecular documentation of described taxa is nearly complete, with about 99% of culturable prokaryotic species (including about 11 900 type strains) represented by 16S rRNA gene sequences (CitationChun and Rainey 2014). Projects such as the sequencing orphan species (SOS) initiative have filled in the gaps for prokaryotes that had valid published names but lacked 16S rRNA genes sequences (CitationYarza et al. 2013). A similar effort to target orphan fungal species is needed, taking advantage of biodiversity collections networks such as the Global Biodiversity Information Facilty (GBIF), iDigBio and the World Federation for Culture Collections to identify sources of material.
Microbiologists have created comprehensive resources for analyses of rRNA gene sequences. For example, the All-Species Living Tree Project provides updated databases, alignments, and phylogenetic trees for about 11 900 species with sequenced type strains (CitationMunoz et al. 2011). Other curated databases contain sequences derived from both cultures and environmental samples. The latest release of the RDP includes 3.2 million aligned and annotated bacterial and archaeal sequences, of which about 85% come from environmental samples (CitationCole et al. 2014). RDP and other projects, such as SILVA (CitationDeSantis et al. 2006, CitationMcDonald et al. 2012b), Mothur (CitationCaporaso et al. 2010) provide additional tools and reference datasets to facilitate high throughput analyses of prokaryotic sequence data, and several now include reference datasets and tools for fungi from UNITE and other sources. The fungal community stands to benefit from continued collaboration with the microbial informatics community, with the aim of developing tools for fungal SBCI comparable to those already available for prokaryotes.
Despite the advances described above, there remains a huge gap between the number of described prokaryotes and the number of uncultured species that have already been discovered (CitationHedlund et al. 2015). Eighty-eight per cent of cultures belong to only four phyla, and more than half of the approximately 60 major prokaryotic lineages (phyla and divisions) are currently represented only by sequence data (CitationRinke et al. 2013). Even when cultures are available, the minimal standards for descriptions of new taxa (http://www.bacterio.net/-minimalstandards.html) adopted by prokaryotic researchers, requiring phenotypic, chemotaxonomic, and genotypic data, too often represent insurmountable barriers to formal classification of much of the diversity of prokaryotes (CitationSchleifer 2009).
Given the restrictions on naming uncultured species, microbiologists have made wide use of informal names for new species, as well as phyla and divisions (CitationBrown et al. 2015, CitationMurray and Schleifer 1994, CitationMurray and Stackebrandt 1995). However, sequence data alone are not sufficient to propose a Candidatus taxon; other information, such as phenotypic and ecological characters or in situ visualization, must also be provided (CitationParte 2014), which represents a minuscule fraction of uncultured prokaryotic diversity. Several proposals have now been made to replace DNA–DNA hybridization for circumscription of species with genome sequences (CitationChun and Rainey 2014) and even use it as type material (CitationWhitman 2015, Citation2016).
Prokaryotic genomics and large-scale environmental sampling
The number of prokaryotic genomes has increased exponentially in recent years (CitationLand et al. 2015). In 2015 the GOLD database (http://www.genomesonline.org) reported 36 824 bacterial and 851 archaeal whole genome sequencing projects (CitationFederhen 2015). The use of genome wide comparisons has already made it possible to consider the streamlining of taxonomic identifications using metrics such as average nucleotide identity (ANI) and k-mer scores (frequencies of sequences of length k) (CitationFederhen et al. 2016). Currently, RefSeq contains 18 454 16S rRNA gene sequences from type strains from 13 314 prokaryotic species (CitationO’Leary et al. 2015).
The mycological counterpart to GEBA is the 1000 Fungal Genomes (1KFG) project (CitationGrigoriev et al. 2014), which is providing a platform for sharing protocols and for community networking and contributing to the transformation of fungal biology into a genome-enabled discipline (CitationHibbett et al. 2013). Genomic data from 1KFG and other projects may not have a major impact on species-level identification, but they will be invaluable for predicting community function. Anyone can nominate a species for the 1KFG project, which aims to sequence two species per family. The emerging resources arePUBLICly accessible and phylogenetically balanced, which will enhance their utility for SBCI. The 1KFG project is not complete, but mycologists should already be looking ahead to the collaborations that will develop the next generation of genomic resources, perhaps modeling their efforts on collaborative ventures associated with GEBA, such as the GEBA Type Strain (CitationKyrpides et al. 2014) and GEBA Microbial Dark Matter projects (http://microbialdarkmatter.org, http://standardsingenomics.org/index.php/sigen/article/view/sigs.5068949).
Prokaryotic genomics is set to expand further through the application of new technologies such as single-cell genomics (CitationAlbertsen et al. 2013), while improved methods for isolating and growing previously uncultured microbes within their natural soil environments (CitationNichols et al. 2010) are opening possibilities to important new discoveries (CitationLing et al. 2015).
New analytical approaches are also facilitating comparisons of genomes for SBCI in prokaryotes. For example, new methods for multilocus sequence analysis have been developed (CitationMende et al. 2013) that could increase accuracy and ameliorate the impact of horizontal gene transfer, which is of particular concern in prokaryotes but also occurs in fungi (CitationKämpfer and Rosselló-Mora 2004, CitationChun and Rainey 2014). Nevertheless, even with whole genomes, prokaryotic SBCI is still challenged to define appropriate cut-off levels for species discrimination (CitationFox et al. 1992, CitationKämpfer and Rosselló-Mora 2004, CitationFraser et al. 2009).
Prokaryotes are focal taxa in global and local culture-based and environmental mega sequencing projects such as NEON (http://www.neoninc.org), the Earth Microbiome (http://www.earthmicrobiome.org/), Terragenome (http://www.terragenome.org/), the Global Microbial Identifier (http://www.globalmicrobialidentifier.org), and Human Microbiome Project (http://www.hmpdacc.org). Mycologists need to be engaged in these efforts, both to promote technology transfer and to ensure that the projects generate data that are useful for fungal SBCI.
Integrating SBCI and fungal taxonomy and nomenclature
In contrast to the prokaryotic ICNB, the International Code of Nomenclature for algae, fungi and plants (the Code) does not require sequence data to describe taxa, but it does mandate that a type specimen be indicated (an illustration or a culture may also be used for fungi under some conditions; Arts. 8.1, 8.4, 40.5) (CitationMcNeill et al. 2012). Consequently, there are many validly named species that lack sequences (), as well as species inferred only from environmental sequences that lack names (CitationHibbett et al. 2011, CitationÖpik et al. 2014). This disconnect presents a barrier to SBCI and it limits understanding of fungal diversity among nonspecialists. To integrate fungal taxonomy and molecular ecology, it will be necessary to expand reference sequence databases based on specimens and consider formally naming species based only on sequences. Sequence data should be obtained not only from new collections but from existing specimens and isolates in fungaria and culture collections, which have been shown to house substantial unrecognized biodiversity (CitationBrock et al. 2009, CitationNagy et al. 2011).
Fig. 2. New fungal species published from 2010 to 2015 based on Index Fungorum records, with and without sequence data of any locus in GenBank as of Jun 2016, as total (A) and proportional (B) values. The complete list of taxa with their higher-level classifications,PUBLICation information, and GenBank accession numbers (where these exist) are included (Supplementary information).
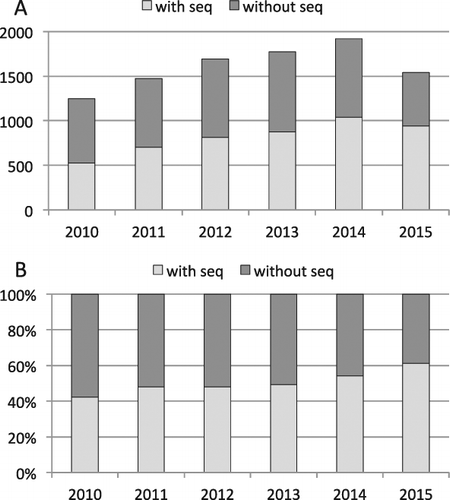
Growing sequence databases for described species
From 1999 to 2009, only about 26% of newly described species of fungi had sequences of any locus deposited in GenBank (CitationHibbett et al. 2011), but from 2010 to 2015 the proportion of new species with sequences increased to 50% overall (60% in 2015, , listed in Supplementary information). One way to grow the number of sequenced species even further would be to modify the Code to require sequence data as part of taxon descriptions. However, this would prevent the naming of fossils and other challenging materials, such as obligate biotrophs and other unculturable fungi that lack macroscopic structures, and could result in the needless destruction of specimens. For example, 64 species of Laboulbeniales (minute obligate insect symbionts) were described in 2010–2016 (so far), but only two have sequence data (see Supplementary information). Even in cases where organisms grow well in culture or produce large reproductive structures, some scientists lack resources for molecular work, particularly in the developing world, where much of the undescribed fungal diversity resides. Increased collaboration between fungal molecular systematists and field mycologists could reduce the number of species that are described without sequence data.
Sequence data should not be required for taxon description under the Code, but there should be general standards, enforced by journal policies and reviewers, that molecular data and metadata accompany all new taxon descriptions when it is reasonable to expect them. Communities of taxonomic specialists will need to determine the appropriate loci to be sequenced, which should include ITS and other markers that are commonly used for SBCI in each group (e.g. tef1 in Fusarium and SSU in Glomeromycota). Organizations such as the International Commission on the Taxonomy of Fungi (ICTF; http://www.fungaltaxonomy.org/) could play a role in promoting best practices. Collaborative efforts to sequence representatives of described species, as in the prokaryotic SOS initiative, should be encouraged. Whenever possible, type materials should be sequenced, but this is often difficult. In such cases, systematists should consider epitypification of names with sequence data from a recent collection, from which a culture and/or nucleic acid sample can be derived (CitationAriyawansa et al. 2014). This approach is particularly important because many important fungal names are not associated with a physical type specimen.
Toward sequence-based species description
The steps outlined above will increase the representation of described species in sequence databases, but they will not solve the problem posed by species known only from environmental sequences. For example, at present UNITE contains 3412 SHs identified only to phylum (CitationNilsson et al. 2016). Similarly, MaarjAM currently contains 292 Glomeromycota VTs composed of only unidentified sequences and 60 named VTs. In natural communities, only 30–50% of AM fungal VTs are named (CitationOhsowski et al. 2014). Groups such as Archaeorhizomycetes and Cryptomycota are known almost entirely from environmental sequences (CitationHibbett 2016). Sequence-based taxonomic platforms such as UNITE and MaarjAM make it possible to group sequences into species hypotheses (SH), virtual taxa (VT), and other MOTUs (molecular operational taxonomic units) (CitationBlaxter et al. 2005). These resources permit fungal ecologists and evolutionary biologists to communicate about taxa known only from sequences and to conduct repeatable analyses (because MOTUs are delimited based on explicit algorithms). However, MOTUs of any sort are obscure concepts to nonspecialists, and they are not included in names-based taxonomic databases such as the Catalogue of Life or Global Biodiversity Information Facility. To facilitate communication and maximize awareness of fungal biodiversity among scientists and the generalPUBLIC, it would be helpful to assign Linnaean binomials to species based solely on sequences (CitationHibbett et al. 2011).
Mycologists were among the first to advocate for the use of DNA sequences in species diagnoses (CitationNagy et al. 2011). However, if estimates of the extant diversity of fungi are anywhere near correct (CitationBlackwell 2011, CitationHibbett et al. 2011). In any case, it is not the purpose of the Code to certify taxonomic hypotheses; its rules do not address the scientific evidence required to justify taxonomic decisions, such as species delimitation. Nonetheless, the Code plays an important gatekeeper role in taxonomy, because only valid names are considered to be “correct” and have the protection of priority.
The next opportunity to change the Code will come in 2017, at the International Botanical Congress XIX in Shenzhen, China, and any changes will become effective on 1 Jan 2018. A proposal to modify the Code to allow sequence-based species description in fungi has recently been published and will be voted on at IBC XIX (CitationHawksworth et al. 2016). The proposal would apply to species “where data were obtained from voucherless environmental sequencing techniques and no individual material is available to serve as the type of a name of a new taxon.” Proposed recommendations would suggest that: (i) new taxa based on sequences should be described with reference to a published phylogenetic analysis; (ii) sequences representing the new taxon should have been detected in multiple independent studies; and (iii) sequences used for taxon description should be drawn from regions deemed appropriate by the relevant taxonomic communities (CitationHawksworth et al. 2016). Other measures that would strengthen sequenced-based species description would include requiring that all appropriate reference databases have been searched and closely related sequences have been included in analyses and that appropriate tests for sequence quality, including tests for chimeric sequences, have been performed (CitationNilsson et al. 2012, CitationHyde et al. 2013, CitationLindahl et al. 2013, CitationHart et al. 2015, CitationSelosse et al. 2016). Again, organizations such as the ICTF could play an important role in developing and disseminating best practices, and journals could help by adopting and enforcing these criteria.
It remains to be seen if the proposal to allow sequences to serve as types (CitationHawksworth et al. 2016) will be approved. However, mycologists do not need to wait until 2018 to begin describing species based on environmental sequences. In the interim, demonstration papers could be produced that present worked examples of sequence-based species descriptions. To some extent, this is already happening. For example, a new species of Neocallimastigomycota, Piromyces cryptodigmaticus, was described based only on sequence data, although a technical voucher sample was obtained (CitationKirk 2012). Similarly, 48 species of Archaeorhizomyces that have been detected with environmental sequences in at least two independent studies were identified, although they were not given Linnaean binomials (CitationMenkis et al. 2014).
A fine example of SBC in action is provided by Hawksworthiomyces sequentia ENAS, which was described by Citationde Beer et al. (2016) based on two independent sequences, one from Canada and the other from Sweden. The ENAS suffix indicates that this taxon was described based on environmental sequences (Table I; CitationTaylor 2011). In describing H. sequentia, de Beer et al. made a serious effort to obtain all relevant cultures, and they performed thorough phylogenetic analyses of sequences from GenBank and UNITE. The study was peer-reviewed and a diagnostic alignment was provided as supplementary material. Thus, the description of H. sequentia provides a model for other mycologists who wish to describe ENAS.
Until the Code is changed sequence-based species names will not have the protection of priority. If taxonomists working with physical specimens rediscover a species that was described based on sequence data, they should consider validating the original sequence-based name, ideally in collaboration with the author of the sequence-based name. To do otherwise would cause confusion and create redundant names, as well as deprive individuals of credit for their discoveries. To promote recognition of sequence-based species, databases of names (“nomenclators”) such as Index Fungorum, MycoBank, and NCBI should be encouraged to take up sequence-based species names, perhaps labeled as “candidate species” (CitationHibbett et al. 2011), or with a suffix to indicate their provisional status (nom. prov.) or their nature as sequence-based taxa (MOTU, ENAS), even if they are not compliant with the Code.
Enabling the community to participate in SBCI
Mycologists were early adopters of SBCI (CitationGardes et al. 1991, CitationBruns et al. 1998), molecular phylogenetics (CitationBruns et al. 1991) and comparative genomics (CitationBirren et al. 2002), but there is still a long way to go before fungal molecular ecology, taxonomy, and functional biology are fully integrated (). Among the most important challenges facing fungal biology are to encourage and enable more mycologists to adopt SBCI, and to contribute molecular and phenotypic information toPUBLICly accessible databases.
Promoting SBCI
Probably the most effective means of promoting SBCI is to publish illustrative examples of research using community resources (Table II). Excellent models already exist for some areas, such as ecological studies of indoor air (CitationAdams et al. 2013), human gut and skin microbes (CitationFindley et al. 2013, CitationHoffmann et al. 2013), AM fungi (CitationDavison et al. 2015), or forest soils (CitationTalbot et al. 2014, CitationTedersoo et al. 2014). InnovativePUBLICations can suggest the way forward but without efforts to define and disseminate best practices, broad adoption will be slow. Critical analyses of published examples and focused meetings held in conjunction with regional and international mycology and microbiology meetings could help to identify the optimal approaches for each group of fungi and research problem. To teach the best practices, tutorials can be developed for workflows and posted on sites such as Wikipedia or YouTube, with links to mycological sites such as MycoBank and UNITE. Mycological sites should catalog these resources, with Joseph Felsenstein’s compilation of phylogeny programs as a model (http://evolution.genetics.washington.edu/phylip/software.html). Practical workshops providing intensive training in both wet-bench and computational methods would also promote adoption of best practices in SBCI.
Academic mycologists have been the leaders in developing SBCI, but to reach the full potential of these methods they must reach out to other groups, including scientists in industry and government who are focused on agriculture, biotechnology, and medicine. These professionals may be the best equipped to develop workflows and teach workshops that reach large numbers of practitioners (e.g. pathologists, quarantine agents, and industrial microbiologists). Liaison with professional societies, such as the American Phytopathological Society and others, will be key to involving these professionals in teaching SBCI.
Another group that will be important to the general acceptance of SBCI is non-professional mycologists, who often have a better knowledge of fungi in the environment than many professional mycologists. Outreach to non-professional groups, such as the North American Mycological Association (NAMA) and regional mycological clubs will help broaden awareness of SBCI. A good example is the North American Mycoflora Project (CitationAmend et al. 2010, CitationBarberan et al. 2015).
Recruiting citizen scientists calls for a multipronged approach, targeting K–12 schools, universities, and the generalPUBLIC, with social media playing a major role. Reaching students will require inclusion of SBCI in curricula and textbooks and direct outreach to organizations such as the National Association of Biology Teachers. Organizations involved in conservation and restoration biology can also help to promote SBCI. For this to occur, fungi must be explicitly included among the target organisms for conservation efforts, and there must be enhanced understanding of the role of SBCI for monitoring fungal populations and assessing their risk of extinction (Veresoglou et al. 2015).
Maintenance and sustainability of databases and collections
The value of databases increases as they grow, and that growth depends on scientists depositing data and analytical workflows inPUBLICly accessible repositories. To simplify this process, it would be helpful if researchers would document their workflows in formats that are ready to upload, e.g. an IPython Notebook. As noted previously, journals and granting agencies have an important role to play in enforcing good practices in research workflows and archiving.
The growth of SBCI also highlights the importance of fungaria and culture collections. Specimens can provide clues to the morphology of organisms known only from sequences, and cultures may provide sufficient DNA for genomic analysis and enable experimental studies that complement environmental observations. Direct sampling of nucleic acids in the environment facilitates large-scale discovery of taxa and complements studies relying on culturing or direct observation of individual organisms. A brilliant example of this complementarity is provided by the aforementioned Archaeorhizomycetes, which was an unnamed and widely distributed clade of phenotypically mysterious fungi known only from environmental LSU sequences (CitationBell et al. 2010, CitationReiser et al. 2016).
According credit for tending the commons
SBCI becomes more powerful as new data are added toPUBLIC repositories. However, career advancement is traditionally based onPUBLICations, not unattributed contributions to databases and collections. An argument can be made that the most concrete legacy of any scientist is the data and materials that they have made available to the field (CitationMcNutt 2014). In the case of biology based on “big data,” this argument is clear and to foster the science we need to move from a reward system that strongly emphasizesPUBLICations to one that also values “quantum contributions” (CitationMaddison et al. 2012), such as DNA sequences and other data, specimens, and cultures, as well as curation and identification services, software, databases, and websites.
Technological as well as cultural shifts will be needed to assess and accord credit for contributions to community resources (CitationMcDade et al. 2011). Automated systems to track usage of data resources could provide quantitative measures of their impact, but they need to include unique identifiers for the workers who provided the data. New onlinePUBLICations such as Biodiversity Data Journal (http://biodiversitydatajournal.com/) and Research Ideas and Outcomes (http://riojournal.com/) allow researchers to publish datasets and workflows, allowing them to be cited by other researchers who use those resources.
Behavioral changes are also needed. For example, studies using data from GenBank/INSDC should not only include accession numbers but should also consider citing the articles in which the sequences were first reported, which will accord merit to the data providers and give them an incentive to update accessions withPUBLICation information (CitationSeifert et al. 2008). In general, increased communication between data providers and data users, possibly leading to co-authorships, can only improve the quality of analyses. Researchers should include sections in their curricula vitae describing contributions to community resources, as is traditionally done by systematists in sections for “taxonomic novelties”. Senior scientists and reviewers have a particular responsibility to train administrators and tenure-and-promotion committees to appreciate the value of contributions to resources for SBCI and their impact on understanding of fungal diversity.
Acknowledgments
Preparation of this article was initiated in a 2 d workshop on SBCI in Fungi that was held at the Kellogg Center of Michigan State University, East Lansing, Michigan, 12–13 Jun 2014 (CitationHerr et al. 2015), which was supported by US National Science Foundation award No. DEB 1424740 to DG, AP-A, DSH, and JWT. A related symposium on “Sequence-based identification in Fungi” was held on 11 Jun 2014 at the annual meeting of the Mycological Society of America, and a Special Interest Group session on “Classifying, naming and communicating sequence based species” took place at the International Mycological Congress, 5 Aug 2014, Bangkok, Thailand. The authors thank Sam Kovaka, who performed the query of GenBank for species names (, Supplementary information), P. Brandon Matheny, and two anonymous reviewers for helpful comments. Mention of trade names or commercial products in thisPUBLICation is solely for the purpose of providing specific information and does not imply recommendation or endorsement by the Victorian government or the US Department of Agriculture. USDA is an equal opportunity provider and employer. CLS acknowledges support from the Intramural Research Program of the National Institutes of Health, National Library of Medicine. Mö acknowledges support from the Estonian Research Council (grant No. IUT20-28) and European Regional Development Fund (Centre of Excellence EcolChange). DSH acknowledges support of NSF award No. DEB-1208719.
Literature cited
- Abarenkov KHenrik Nilsson RLarsson KHAlexander IJEberhardt UErland SHoiland KKjoller RLarsson EPennanen TSen RTaylor AFTedersoo LUrsing BMVralstad TLiimatainen KPeintner UKoljalg U.2010. The UNITE database for molecular identification of fungi—recent updates and future perspectives. New Phytol 186:281–285 doi:10.1111/j.1469-8137.2009.03160.x
- Adams RIMiletto MTaylor JWBruns TD.2013. The diversity and distribution of fungi on residential surfaces. PLoS One 8:e78866 doi:10.1371/journal.pone.0078866
- Albertsen MHugenholtz PSkarshewski ANielsen KLTyson GWNielsen PH.2013. Genome sequences of rare, uncultured bacteria obtained by differential coverage binning of multiple metagenomes. Nat Biotechnol 31:533–538 doi:10.1038/nbt.2579
- Amend ASSeifert KASamson RBruns TD.2010. Indoor fungal composition is geographically patterned and more diverse in temperate zones than in the tropics. Proc Natl Acad Sci U S A 107:13748–13753 doi:10.1073/pnas.1000454107
- Ariyawansa HAHawksworth DLHyde KDJones EBGMaharachchikumbura SSNManamgoda DSThambugala KMUdayanga DCamporesi EDaranagama AJayawardena RLiu J-KMcKenzie EHCPhookamsak RSenanayake ICShivas RGTian QXu J-C.2014. Epitypification and neotypification: guidelines with appropriate and inappropriate examples. Fungal Divers 69:57–91 doi:10.1007/s13225-014-0315-4
- Barberan ALadau JLeff JWPollard KSMenninger HLDunn RRFierer N.2015. Continental-scale distributions of dust-associated bacteria and fungi. Proc Natl Acad Sci U S A 112:5756–5761 doi:10.1073/pnas.1420815112
- Bell JMasaoka JZimmerman S.2010. Nonprofit sustainability: making strategic decisions for financial viability. San Francisco, California: Wiley. 208 p.
- Bengtsson-Palme JRyberg MHartmann MBranco SWang ZGodhe ADe Wit PSánchez-García MEbersberger Ide Sousa FAmend AJumpponen AUnterseher MKristiansson EAbarenkov KBertrand YJKSanli KEriksson KMVik UVeldre VNilsson RH.2013. Improved software detection and extraction of ITS1 and ITS2 from ribosomal ITS sequences of fungi and other eukaryotes for analysis of environmental sequencing data. Methods Ecol Evol 4:914–919 doi:10.1111/2041-210x.12073
- Bidartondo MI.2008. Preserving accuracy in GenBank. Science 319:1616 doi:10.1126/science.319.5870.1616a
- Birren BFink GLander E.2002. Fungal genome initiative. Cambridge, Massachusetts: Whitehead Institute Center for Genome Research. 26 p.
- Blackwell M.2011. The fungi: 1, 2, 3 … 5.1 million species? Am J Bot 98:426–438 doi:10.3732/ajb.1000298
- Blaxter MMann JChapman TThomas FWhitton CFloyd RAbebe E.2005. Defining operational taxonomic units using DNA barcode data. Philos Trans R Soc B 360:1935–1943 doi:10.1098/rstb.2005.1725
- Bridge PDRoberts PJSpooner BMPanchal G.2003. On the unreliability of published DNA sequences. New Phytol 160:43–48 doi:10.1046/j.1469-8137.2003.00861.x
- Brock PMDöring HBidartondo MI.2009. How to know unknown fungi: the role of a herbarium. New Phytol 181:719–724 doi:10.1111/j.1469-8137.2008.02703.x
- Brown CTHug LAThomas BCSharon ICastelle CJSingh AWilkins MJWrighton KCWilliams KHBanfield JF.2015. Unusual biology across a group comprising more than 15% of domain Bacteria. Nature 523:208–211 doi:10.1038/nature14486
- Bruns TD.2012. The North American Mycoflora Project—the first steps on a long journey. New Phytol 192:972–974 doi:10.1111/nph.12027
- Bruns TDSzaro TMGardes MCullings KWPan JJTaylor DLHorton TRKrentzer AGarbelotto MLi Y.1998. A sequence database for the identification of ectomycorrhizal basidiomycetes by phylogenetic analysis. Molec Ecol Resour 7:257–272 doi:10.1046/j.1365-294X.1998.00337.x
- Bruns TDWhite TTaylor J.1991. Fungal molecular systematics. Ann Rev Ecol Syst 22:525–564 doi:10.1146/annurev.es.22.110191.002521
- Caporaso JGKuczynski JStombaugh JBittinger KBushman FDCostello EKFierer NPeña AGGoodrich JKGordon JIHuttley GAKelley STKnights DKoenig JELey RELozupone CAMcDonald DMuegge BDPirrung MReeder JSevinsky JRTurnbaugh PJWalters WAWidmann JYatsunenko TZaneveld JKnight R.2010. QIIME allows analysis of high-throughput community sequencing data. Nat Methods 7:335–336 doi:10.1038/nmeth.f.303
- Chun JRainey FA.2014. Integrating genomics into the taxonomy and systematics of the Bacteria and Archaea. Int J Syst Evol Micr 64:316–324 doi:10.1099/ijs.0.054171-0
- Cochrane GKarsch-Mizrachi ITakagi T Sequence Database Collaboration IN.2016. The International Nucleotide Sequence Database Collaboration. Nucleic Acids Res 44:D48–D50 doi:10.1093/nar/gkv1323
- Cole JRWang QFish JAChai BMcGarrell DMSun YBrown CTPorras-Alfaro AKuske CRTiedje JM.2014. Ribosomal Database Project: data and tools for high throughput rRNA analysis. Nucleic Acids Res 42:D633–D642 doi:10.1093/nar/gkt1244
- Corsaro DWalochnik JVenditti DSteinmann JMuller KDMichel R.2014. Microsporidia-like parasites of amoebae belong to the early fungal lineage Rozellomycota. Parasitol Res 113:1909–1918 doi:10.1007/s00436-014-3838-4
- Davison JMoora MÖpik MAdholeya AAinsaar LBâ ABurla SDiedhiou AGHiiesalu IJairus TJohnson NCKane AKoorem KKochar MNdiaye CPärtel MReier ÜSaks ÜSingh RVasar MZobel M.2015. Global assessment of arbuscular mycorrhizal fungus diversity reveals very low endemism. Science 349:970–973 doi:10.1007/s00436-014-3838-4
- Deans ARLewis SEHuala EAnzaldo SSAshburner MBalhoff JPBlackburn DCBlake JABurleigh JGChanet BCooper LDCourtot MCsösz SCui HDahdul WDas SDececchi TADettai ADiogo RDruzinsky REDumontier MFranz NMFriedrich FGkoutos GVHaendel MHarmon LJHayamizu TFHe YHines HMIbrahim NJackson LMJaiswal PJames-Zorn CKöhler SLecointre GLapp HLawrence CJLe Novère NLundberg JGMacklin JMast ARMidford PEMikó IMungall CJOellrich AOsumi-Sutherland DParkinson HRamírez MJRichter SRobinson PNRuttenberg ASchulz KSSegerdell ESeltmann KCSharkey MJSmith ADSmith BSpecht CDSquires RBThacker RWThessen AFernandez-Triana JVihinen MVize PDVogt LWall CEWalls RLWesterfeld MWharton RAWirkner CSWoolley JBYoder MJZorn AMMabee P.2015. Finding our way through phenotypes. PLoS Biol 13:e1002033 doi:10.1371/journal.pbio.1002033
- de Beer ZWMarincowitz SDuong TAKim J-JRodrigues AWingfield MW.2016. Hawksworthiomyces gen. nov. (Ophiostomatales), illustrates the urgency for a decision on how to name novel taxa known only from environmental nucleic acid sequences (ENAS). Fungal Biol doi:10.1016/j.funbio.2016.07.004
- DeSantis TZHugenholtz PLarsen NRojas MBrodie ELKeller KHuber TDalevi DHu PAndersen GL.2006. Greengenes, a chimera-checked 16S rRNA gene database and workbench compatible with ARB. Appl Environ Microbiol 72:5069–5072 doi:10.1128/AEM.03006-05
- Deshpande VWang QGreenfield PCharleston MPorras-Alfaro AKuske CRCole JRMidgley DJTran-Dinh N.2015. Fungal identification using a Bayesian classifier and the Warcup training set of internal transcribed spacer sequences. Mycologia 108:1–5 doi:10.3852/14-293
- Drew BTGazis RCabezas PSwithers KSDeng JRodriguez RKatz LACrandall KAHibbett DSSoltis DE.2013. Lost branches on the tree of life. PLoS Biol 11:e1001636 doi:10.1371/journal.pbio.1001636
- Federhen S.2015. Type material in the NCBI taxonomy database. Nucleic Acids Res 43:D1086–D1098 doi:10.1093/nar/gku1127
- Federhen SRossello-Mora RKlenk H-PTindall BJKonstantinidis KTWhitman WBBrown DLabeda DUssery DGarrity GMColwell RRHasan NGraf JParte AYarza PGoldberg BSichtig HKarsch-Mizrachi IClark KMcVeigh RPruitt KDTatusova TFalk RTurner SMadden TKitts PKimchi AKlimke WAgarwala RDiCuccio MOstell J.2016. Meeting report: GenBank microbial genomic taxonomy workshop (12–13 May 2015). Stand Genomic Sci 11:1–8.
- Findley KOh JYang JConlan SDeming CMeyer JASchoenfeld DNomicos EPark MKong HHSegre JAComp NIHISC.2013. Topographic diversity of fungal and bacterial communities in human skin. Nature 498:367–370 doi:10.1038/nature12171
- Fox GEWisotzkey JDJurtshuk P.1992. How close is close: 16S rRNA sequence identity may not be sufficient to guarantee species identity. Int J Syst Evol Micr 42:166–170 doi:10.1099/00207713-42-1-166
- Fraser CAlm EJPolz MFSpratt BGHanage WP.2009. The bacterial species challenge: making sense of genetic and ecological diversity. Science 323:741–746 doi:10.1126/science.1159388
- Gardes MMueller GMFortin JAKropp BR.1991. Mitochondrial DNA polymorphisms in Laccaria bicolor, L. laccata, L. proxima and L. amethystina. Mycol Res 95:206–216 doi:10.1016/S0953-7562(09)81013-7
- Geiser DMdel Mar Jiménez-Gasco MKang SMakalowska IVeeraraghavan NWard TJZhang NKuldau GAO’Donnell K.2004. FUSARIUM-ID 1.0: a DNA sequence database for identifying Fusarium. In: Mulè GBailey JACooke BMLogrieco A eds. Molecular diversity and PCR-detection of toxigenic Fusarium species and ochratoxigenic fungi: under the aegis of COST Action 835 ‘Agriculturally Important Toxigenic Fungi 1998–2003’, EU project (QLK1-CT-1998-01380) and the ISPP ‘Fusarium Committee’. Dordrecht, the Netherlands: Springer. p 473–479.
- Glass DJTakebayashi NOlson LETaylor DL.2013. Evaluation of the authenticity of a highly novel environmental sequence from boreal forest soil using ribosomal RNA secondary structure modeling. Mol Phylognet Evol 67:234–245 doi:10.1016/j.ympev.2013.01.018
- Grigoriev IVNikitin RHaridas SKuo AOhm ROtillar RRiley RSalamov AZhao XKorzeniewski FSmirnova TNordberg HDubchak IShabalov I.2014. MycoCosm portal: gearing up for 1000 fungal genomes. Nucleic Acids Res 42:D699–D704 doi:10.1093/nar/gkt1183
- Hart MMAleklett KChagnon P-LEgan CGhignone SHelgason TLekberg YÖpik MPickles BJWaller L.2015. Navigating the labyrinth: a guide to sequence-based, community ecology of arbuscular mycorrhizal fungi. New Phytol 207:235–247 doi:10.1111/nph.13340
- Hawksworth DLHibbett DKirk PMLücking R.2016. (308–310) Proposals to amend Art. 8 to permit the use of DNA sequences as types of names of fungi. Taxon 65:899–900 doi:10.12705/654.31
- Hedlund BPDodsworth JAStaley JT.2015. The changing landscape of microbial biodiversity exploration and its implications for systematics. Syst Appl Microbiol 38:231–236 doi:10.1016/j.syapm.2015.03.003
- Herr JRÖpik MHibbett D.2015. Towards the unification of sequenced-based classification and sequenced-based identification of host-associated microorganisms. New Phytol 205:27–31 doi:10.1111/nph.13180
- Hibbett D.2016. The invisible dimension of fungal diversity. Science 351:1150–1151 doi:10.1126/science.aae0380
- Hibbett DNilsson RSnyder MFonseca MCostanzo JShonfeld M.2005. Automated phylogenetic taxonomy: an example in the Homobasidiomycetes (mushroom-forming Fungi). Syst Biol 54:660–668 doi:10.1080/10635150590947104
- Hibbett DOhman AGlotzer DNuhn MKirk PNilsson RH.2011. Progress in molecular and morphological taxon discovery in Fungi and options for formal classification of environmental sequences. Fungal Biol Rev 25:38–47 doi:10.1016/j.fbr.2011.01.001
- Hibbett DStajich JESpatafora JW.2013. Toward genome-enabled mycology. Mycologia 105:1339–1349 doi:10.3852/13-196
- Hinchliff CESmith SAAllman JFBurleigh JGChaudhary RCoghill LMCrandall KADeng JDrew BTGazis RGude KHibbett DSKatz LALaughinghouse HDIMcTavish EJMidford PEOwen CLRee RHRees JASoltis DEWilliams TCranston KA.2015. Synthesis of phylogeny and taxonomy into a comprehensive tree of life. Proc Natl Acad Sci U S A 112:12764–12769 doi:10.1073/pnas.1423041112
- Hofer U.2013. Environmental microbiology: exploring diversity with single-cell genomics. Nat Rev Microbiol 11:598–599 doi:10.1038/nrmicro3095
- Hoffmann CDollive SGrunberg SChen JLi HZWu GDLewis JDBushman FD.2013. Archaea and fungi of the human gut microbiome: correlations with diet and bacterial residents. PLoS One 8:e66019 doi:10.1371/journal.pone.0066019
- Hyde KDUdayanga DManamgoda DSTedersoo LLarsson EAbarenkov KBertrand YJKOxelman BHartmann MKauserud HRyberg MKristiansson ENilsson RH.2013. Incorporating molecular data in fungal systematics: a guide for aspiring researchers. Curr Res Environ Appl Mycol J Fungal Biol 3:32.
- Irinyi LSerena CGarcia-Hermoso DArabatzis MDesnos-Ollivier MVu DCardinali GArthur INormand A-CGiraldo Ada Cunha KCSandoval-Denis MHendrickx MNishikaku ASde Azevedo Melo ASMerseguel KBKhan AParente Rocha JASampaio Pda Silva Briones MRe Ferreira RCde Medeiros Muniz MCastañón-Olivares LREstrada-Barcenas DCassagne CMary CDuan SYKong FSun AYZeng XZhao ZGantois NBotterel FRobbertse BSchoch CGams WEllis DHalliday CChen SSorrell TCPiarroux RColombo ALPais Cde Hoog SZancopé-Oliveira RMTaylor MLToriello Cde Almeida Soares CMDelhaes LStubbe DDromer FRanque SGuarro JCano-Lira JFRobert VVelegraki AMeyer W.2015. International Society of Human and Animal Mycology (ISHAM)-ITS reference DNA barcoding database—the quality controlled standard tool for routine identification of human and animal pathogenic fungi. Med Mycol 53:313–337 doi:10.1093/mmy/myv008
- James TYBerbee ML.2012. No jacket required—new fungal lineage defies dress code: recently described zoosporic fungi lack a cell wall during trophic phase. Bioessays 34:94–102 doi:10.1002/bies.201100110
- Jones MDMForn IGadelha CEgan MJBass DMassana RRichards TA.2011. Discovery of novel intermediate forms redefines the fungal tree of life. Nature 474:200–203 doi:10.1038/nature09984
- Kämpfer PRosselló-Mora R.2004. The species concept for prokaryotic microorganisms—an obstacle for describing diversity? Poiesis Prax 3:62–72 doi:10.1007/s10202-004-0068-3
- Kirk PM.2012. Nomenclatural novelties. Index Fungorum 1:1–1.
- Kõljalg ULarsson K-HAbarenkov KNilsson RHAlexander IJEberhardt UErland SHøiland KKjøller RLarsson EPennanen TSen RTaylor AFSTedersoo LVrålstad T.2005. UNITE: a database providing web-based methods for the molecular identification of ectomycorrhizal fungi. New Phytol 166:1063–1068 doi:10.1111/j.1469-8137.2005.01376.x
- Kõljalg UNilsson RHAbarenkov KTedersoo LTaylor AFSBahram MBates STBruns TDBengtsson-Palme JCallaghan TMDouglas BDrenkhan TEberhardt UDueñas MGrebenc TGriffith GWHartmann MKirk PMKohout PLarsson ELindahl BDLücking RMartín MPMatheny PBNguyen NHNiskanen TOja JPeay KGPeintner UPeterson MPõldmaa KSaag LSaar ISchüßler AScott JASenés CSmith MESuija ATaylor DLTelleria MTWeiss MLarsson K-H.2013. Towards a unified paradigm for sequence-based identification of fungi. Mol Ecol 22:5271–5277 doi:10.1111/mec.12481
- Kopchinskiy AKomon MKubicek CPDruzhinina IS.2005. Trichoblast: a multilocus database for Trichoderma and Hypocrea identifications. Mycol Res 109:658–660 doi:10.1017/S0953756205233397
- Koren SHarhay GPSmith TPBono JLHarhay DMMcvey SDRadune DBergman NHPhillippy AM.2013. Reducing assembly complexity of microbial genomes with single-molecule sequencing. Genome Biol 14:1 doi:10.1186/gb-2013-14-9-r101
- Kyrpides NCHugenholtz PEisen JAWoyke TGöker MParker CTAmann RBeck BJChain PSGChun JColwell RRDanchin ADawyndt PDedeurwaerdere TDeLong EFDetter JCDe Vos PDonohue TJDong X-ZEhrlich DSFraser CGibbs RGilbert JGilna PGöker FOJansson JKKeasling JDKnight RLabeda DLapidus ALee J-SLi W-JMa JMarkowitz VMoore ERBMorrison MMeyer FNelson KEOhkuma MOuzounis CAPace NParkhill JQin NRossello-Mora RSikorski JSmith DSogin MStevens RStingl USuzuki K-iTaylor DTiedje JMTindall BWagner MWeinstock GWeissenbach JWhite OWang JZhang LZhou Y-GField DWhitman WBGarrity GMKlenk H-P.2014. Genomic Encyclopedia of Bacteria and Archaea: sequencing a myriad of type strains. PLoS Biol 12:e1001920 doi:10.1371/journal.pbio.1001920
- Land MHauser LJun SRNookaew ILeuze MRAhn THKarpinets TLund OKora GWassenaar TPoudel SUssery DW.2015. Insights from 20 years of bacterial genome sequencing. Funct Integr Genomics 15:141–161 doi:10.1007/s10142-015-0433-4
- Lara EMoreira DLópez-García P.2010. The environmental clade LKM11 and Rozella form the deepest branching clade of Fungi. Protist 161:116–121 doi:10.1016/j.protis.2009.06.005
- Larsen NOlsen GJMaidak BLMcCaughey MJOverbeek RMacke TJMarsh TLWoese CR.1993. The ribosomal database project. Nucleic Acids Res 21:3021–3023 doi:10.1093/nar/21.13.3021
- Lindahl BDNilsson RHTedersoo LAbarenkov KCarlsen TKjøller RKõljalg UPennanen TRosendahl SStenlid JKauserud H.2013. Fungal community analysis by high-throughput sequencing of amplified markers—a user’s guide. New Phytol 199:288–299 doi:10.1111/nph.12243
- Lindner DLBanik MT.2011. Intra-genomic variation in the ITS rDNA region obscures phylogenetic relationships and inflates estimates of operational taxonomic units in genus Laetiporus. Mycologia 103:731–740 doi:10.3852/10-331
- Lindner DLCarlsen THenrik Nilsson RDavey MSchumacher TKauserud H.2013. Employing 454 amplicon pyrosequencing to reveal intragenomic divergence in the internal transcribed spacer rDNA region in fungi. Ecol Evol 3:1751–1764 doi:10.1002/ece3.586
- Ling LLSchneider TPeoples AJSpoering ALEngels IConlon BPMueller ASchaberle TFHughes DEEpstein SJones MLazarides LSteadman VACohen DRFelix CRFetterman KAMillett WPNitti AGZullo AMChen CLewis K.2015. A new antibiotic kills pathogens without detectable resistance. Nature 517:455–459 doi:10.1038/nature14098
- Liu K-LPorras-Alfaro AKuske CREichorst SAXie G.2012. Accurate, rapid taxonomic classification of fungal large-subunit rRNA genes. Appl Environ Microbiol 78:1523–1533 doi:10.1128/AEM.06826-11
- Lutzoni FKauff FCox CJMcLaughlin DCelio GDentinger BPadamsee MHibbett DJames TYBaloch EGrube MReeb VHofstetter VSchoch CArnold AEMiadlikowska JSpatafora JJohnson DHambleton SCrockett MShoemaker RSung G-HLücking RLumbsch TO’Donnell KBinder MDiederich PErtz DGueidan CHansen KHarris RCHosaka KLim Y-WMatheny BNishida HPfister DRogers JRossman ASchmitt ISipman HStone JSugiyama JYahr RVilgalys R.2004. Assembling the fungal tree of life: progress, classification, and evolution of subcellular traits. Am J Bot 91:1446–1480 doi:10.3732/ajb.91.10.1446
- Maddison DRGuralnick RHill AReysenbach ALMcDade LA.2012. Ramping up biodiversity discovery via online quantum contributions. Trends Ecol Evol 27:72–77 doi:10.1016/j.tree.2011.10.010
- Maidak BLLarsen NMcCaughey MJOverbeek ROlsen GJFogel KBlandy JWoese CR.1994. The ribosomal database project. Nucleic Acids Res 22:3485–3487 doi:10.1093/nar/22.17.3485
- McDade LAMaddison DRGuralnick RPiwowar HAJameson MLHelgen KMHerendeen PSHill AVis ML.2011. Biology needs a modern assessment system for professional productivity. Bioscience 61:619–625 doi:10.1525/bio.2011.61.8.8
- McDonald DClemente JCKuczynski JRideout JRStombaugh JWendel DWilke AHuse SHufnagle JMeyer FKnight RCaporaso JG.2012a. The Biological Observation Matrix (BIOM) format or: how I learned to stop worrying and love the ome-ome. Gigascience 1:1–6 doi:10.1186/2047-217X-1-7
- McDonald DPrice MNGoodrich JNawrocki EPDeSantis TZProbst AAndersen GLKnight RHugenholtz P.2012b. An improved Greengenes taxonomy with explicit ranks for ecological and evolutionary analyses of bacteria and archaea. ISME J 6:610–618 doi:10.1038/ismej.2011.139
- McNeill JBarrie FRBuck WRDemoulin VGreuter WHawksworth DLHerendeen PSKnapp SMarhold KPrado JPrud’homme van Reine WFSmith GFWiersema JHTurland NJ.2012. International Code of Nomenclature for algae, fungi, and plants (Melbourne Code), Adopted by the Eighteenth International Botanical Congress Melbourne, Australia, Jul 2011. Köenigstein, Germany: Koeltz Scientific Books. 240 p.
- McNutt M.2014. The measure of research merit. Science 346:1155–1155 doi:10.1126/science.aaa3796
- Mende DRSunagawa SZeller GBork P.2013. Accurate and universal delineation of prokaryotic species. Nat Methods 10:881–884 doi:10.1038/nmeth.2575
- Menkis AUrbina HJames TYRosling A.2014. Archaeorhizomyces borealis sp. nov. and a sequence-based classification of related soil fungal species. Fungal Biol 118:943–955 doi:10.1016/j.funbio.2014.08.005
- Munoz RYarza PLudwig WEuzéby JAmann RSchleifer K-HOliver Glöckner FRosselló-Móra R.2011. Release LTPs104 of the all-species living tree. Syst Appl Microbiol 34:169–170 doi:10.1016/j.syapm.2011.03.001
- Murray RSchleifer K.1994. Taxonomic notes: a proposal for recording the properties of putative taxa of procaryotes. Int J Syst Bacteriol 44:174–176 doi:10.1099/00207713-44-1-174
- Murray RStackebrandt E.1995. Taxonomic note: implementation of the provisional status Candidatus for incompletely described procaryotes. Int J Syst Bacteriol 45:186–187 doi:10.1099/00207713-45-1-186
- Nagy LPetkovits TKovács GVoigt KVágvölgyi CPapp T.2011. Where is the unseen fungal diversity hidden? A study of Mortierella reveals a large contribution of reference collections to the identification of fungal environmental sequences. New Phytol 191:789–794 doi:10.1111/j.1469-8137.2011.03707.x
- Nguyen NHSong ZBates STBranco STedersoo LMenke JSchilling JSKennedy PG.2016. FUNGuild: an open annotation tool for parsing fungal community datasets by ecological guild. Fungal Ecol 20:241–248 doi:10.1016/j.funeco.2015.06.006
- Nichols DCahoon NTrakhtenberg EMPham LMehta ABelanger AKanigan TLewis KEpstein SS.2010. Use of Ichip for high-throughput in situ cultivation of “uncultivable” microbial species. Appl Environ Microbiol 76:2445–2450 doi:10.1128/AEM.01754-09
- Nilsson RHTedersoo LAbarenkov KRyberg MKristiansson EHartmann MSchoch CLNylander JAABergsten JPorter TMJumpponen AVaishampayan POvaskainen OHallenberg NBengtsson-Palme JEriksson KMLarsson K-HLarsson EKõljalg U.2012. Five simple guidelines for establishing basic authenticity and reliability of newly generated fungal ITS sequences. MycoKeys 4:37–63 doi:10.3897/mycokeys.4.3606
- Nilsson RHWurzbacher CBahram MCoimbra VRMLarsson ETedersoo LEriksson JDuarte CSvantesson SSánchez-García MRyberg MKKristiansson EAbarenkov K.2016. Top 50 most wanted fungi. MycoKeys 12:29–40 doi:10.3897/mycokeys.12.7553
- O’Donnell KCigelnik ENirenberg HI.1998. Molecular systematics and phylogeography of the Gibberella fujikuroi species complex. Mycologia 90:465–493 doi:10.2307/3761407
- O’Donnell KSutton DARinaldi MGSarver BAJBalajee SASchroers H-JSummerbell RCRobert VARGCrous PWZhang NAoki TJung KPark JLee Y-HKang SPark BGeiser DM.2010. Internet-accessible DNA sequence database for identifying Fusaria from human and animal infections. J Clin Microbiol 48:3708–3718 doi:10.1128/JCM.00989-10
- O’Donnell KWard TJRobert VARGCrous PWGeiser DMKang S.2015. DNA sequence-based identification of Fusarium: current status and future directions. Phytoparasitica 43:583–595 doi:10.1007/s12600-015-0484-z
- Ohsowski BMZaitsoff PDÖpik MHart MM.2014. Where the wild things are: looking for uncultured Glomeromycota. New Phytol 204:171–179 doi:10.1111/nph.12894
- O’Leary NAWright MWBrister JRCiufo SHaddad DMcVeigh RRajput BRobbertse BSmith-White BAko-Adjei DAstashyn ABadretdin ABao YBlinkova OBrover VChetvernin VChoi JCox EErmolaeva OFarrell CMGoldfarb TGupta THaft DHatcher EHlavina WJoardar VSKodali VKLi WMaglott DMasterson PMcGarvey KMMurphy MRO’Neill KPujar SRangwala SHRausch DRiddick LDSchoch CShkeda AStorz SSSun HThibaud-Nissen FTolstoy ITully REVatsan ARWallin CWebb DWu WLandrum MJKimchi ATatusova TDiCuccio MKitts PMurphy TDPruitt KD.2015. Reference sequence (RefSeq) database at NCBI: current status, taxonomic expansion, and functional annotation. Nucleic Acids Res gkv1189v1.
- Öpik MDavison JMoora MZobel M.2014. DNA-based detection and identification of Glomeromycota: the virtual taxonomy of environmental sequences1. Botany 92:135–147 doi:10.1139/cjb-2013-0110
- Öpik MVanatoa AVanatoa EMoora MDavison JKalwij JMReier UZobel M.2010. The online database MaarjAM reveals global and ecosystemic distribution patterns in arbuscular mycorrhizal fungi (Glomeromycota). New Phytol 188:223–241 doi:10.1111/j.1469-8137.2010.03334.x
- Page RD.2013. BioNames: linking taxonomy, texts, and trees. Peer J 1:e190 doi:10.7717/peerj.190
- Parberry DGRobertson NF.1999. Obituary: John Henry (Jack) Warcup: 29 October 1921–15 May 1998. Mycologist 13:48–49 doi:10.1016/S0269-915X(99)80092-5
- Park BPark JCheong K-CChoi JJung KKim DLee Y-HWard TJO’Donnell KGeiser DMKang S.2010. Cyber infrastructure for Fusarium: three integrated platforms supporting strain identification, phylogenetics, comparative genomics and knowledge sharing. Nucleic Acids Res 2010:1–7.
- Parker CTTindall BJGarrity GM.2015. International Code of Nomenclature of Prokaryotes. Int J Syst Evol Microbiol (In press), doi:10.1099/ijsem.0.000778
- Parr CSGuralnick RCellinese NPage RDM.2012. Evolutionary informatics: unifying knowledge about the diversity of life. Trends Ecol Evol 27:94–103 doi:10.1016/j.tree.2011.11.001
- Parte AC.2014. LPSN—list of prokaryotic names with standing in nomenclature. Nucleic Acids Res 42:D613–D616 doi:10.1093/nar/gkt1111
- Porras-Alfaro ALiu K-LKuske CRXie G.2014. From genus to phylum: large-subunit and internal transcribed spacer rRNA operon regions show similar classification accuracies influenced by database composition. Appl Environ Microbiol 80:829–840 doi:10.1128/AEM.02894-13
- Quast CPruesse EYilmaz PGerken JSchweer TYarza PPeplies JGlöckner FO.2013. The SILVA ribosomal RNA gene database project: improved data processing and web-based tools. Nucleic Acids Res 41:D590–D596. doi:10.1093/nar/gks1219
- Ratnasingham SHebert PDN.2007. BOLD: The Barcode of Life Data System (http://www.barcodinglife.org). Mol Ecol Notes 7:355–364 doi:10.1111/j.1471-8286.2007.01678.x
- Reddy TBThomas ADStamatis DBertsch JIsbandi MJansson JMallajosyula JPagani ILobos EAKyrpides NC.2015. The genomes online database (GOLD) 5: a metadata management system based on a four level (meta)genome project classification. Nucleic Acids Res 43:D1099–1106 doi:10.1093/nar/gku950
- Reiser LBerardini TZLi DMuller RStrait EMLi QMezheritsky YVetushko AHuala E.2016. Sustainable funding for biocuration: the Arabidopsis Information Resource (TAIR) as a case study of a subscription-based funding model. Database bav018 doi:10.1093/database/baw018
- Renner SS.2016. A return to Linnaeus’s focus on diagnosis, not description: the use of DNA characters in the formal naming of species. Syst Biol (In press), doi:10.1093/sysbio/syw032
- Reynolds DTaylor J.1991. DNA specimens and the ‘International code of botanical nomenclature’. Taxon 40:311–351 doi:10.2307/1222985
- Richter MRosselló-Móra R.2009. Shifting the genomic gold standard for the prokaryotic species definition. Proc Natl Acad Sci USA 106:19126–19131 doi:10.1073/pnas.0906412106
- Rinke CSchwientek PSczyrba AIvanova NNAnderson IJCheng J-FDarling AMalfatti SSwan BKGies EADodsworth JAHedlund BPTsiamis GSievert SMLiu W-TEisen JAHallam SJKyrpides NCStepanauskas RRubin EMHugenholtz PWoyke T.2013. Insights into the phylogeny and coding potential of microbial dark matter. Nature 499:431–437 doi:10.1038/nature12352
- Rosling ACox FCruz-Martinez KIhrmark KGrelet G-ALindahl BDMenkis AJames TY.2011. Archaeorhizomycetes: unearthing an ancient class of ubiquitous soil fungi. Science 333:876–879 doi:10.1126/science.1206958
- Ryberg MKristiansson ESjökvist ENilsson RH.2009. An outlook on the fungal internal transcribed spacer sequences in GenBank and the introduction of a web-based tool for the exploration of fungal diversity. New Phytol 181:471–477 doi:10.1111/j.1469-8137.2008.02667.x
- Schadt CWMartin APLipson DASchmidt SK.2003. Seasonal dynamics of previously unknown fungal lineages in tundra soils. Science 301:1359–1361 doi:10.1126/science.1086940
- Schleifer KH.2009. Classification of Bacteria and Archaea: past, present and future. Syst Appl Microbiol 32:533–542 doi:10.1016/j.syapm.2009.09.002
- Schloss PDWestcott SLRyabin THall JRHartmann MHollister EBLesniewski RAOakley BBParks DHRobinson CJSahl JWStres BThallinger GGVan Horn DJWeber CF.2009. Introducing mothur: open-source, platform-independent, community-supported software for describing and comparing microbial communities. Appl Environ Microbiol 75:7537–7541 doi:10.1128/AEM.01541-09
- Schoch CLRobbertse BRobert VVu DCardinali GIrinyi LMeyer WNilsson RHHughes KMiller ANKirk PMAbarenkov KAime MCAriyawansa HABidartondo MBoekhout TBuyck BCai QChen JCrespo ACrous PWDamm UDe Beer ZWDentinger BTMDivakar PKDueñas MFeau NFliegerova KGarcía MAGe Z-WGriffith GWGroenewald JZGroenewald MGrube MGryzenhout MGueidan CGuo LHambleton SHamelin RHansen KHofstetter VHong S-BHoubraken JHyde KDInderbitzin PJohnston PRKarunarathna SCKõljalg UKovács GMKraichak EKrizsan KKurtzman CPLarsson K-HLeavitt SLetcher PMLiimatainen KLiu J-KLodge DJJennifer Luangsaard JLumbsch HTMaharachchikumbura SSNManamgoda DMartín MPMinnis AMMoncalvo J-MMulè GNakasone KKNiskanen TOlariaga IPapp TPetkovits TPino-Bodas RPowell MJRaja HARedecker DSarmiento-Ramirez JMSeifert KAShrestha BStenroos SStielow BSuh S-OTanaka KTedersoo LTelleria MTUdayanga DUntereiner WADiéguez Uribeondo JSubbarao KVVágvölgyi CVisagie CVoigt KWalker DMWeir BSWeiß MWijayawardene NNWingfield MJXu JPYang ZLZhang NZhuang W-YFederhen S.2014. Finding needles in haystacks: linking scientific names, reference specimens and molecular data for Fungi. Database 2014.
- Schoch CLSeifert KAHuhndorf SRobert VSpouge JLLevesque CAChen WBolchacova EVoigt KCrous PWMiller ANWingfield MJAime MCAn KDBai FYBarreto RWBegerow DBergeron MJBlackwell MBoekhout TBogale MBoonyuen NBurgaz ARBuyck BCai LCai QCardinali GChaverri PCoppins BJCrespo ACubas PCummings CDamm Ude Beer ZWde Hoog GSDel-Prado RDentinger BDieguez-Uribeondo JDivakar PKDouglas BDueñas MDuong TAEberhardt UEdwards JEElshahed MSFliegerova KFurtado MGarcía MAGe ZWGriffith GWGriffiths KGroenewald JZGroenewald MGrube MGryzenhout MGuo LDHagen FHambleton SHamelin RCHansen KHarrold PHeller GHerrera GHirayama KHirooka YHo HMHoffmann KHofstetter VHognabba FHollingsworth PMHong SBHosaka KHoubraken JHughes KHuhtinen SHyde KDJames TJohnson EMJohnson JEJohnston PRJones EBKelly LJKirk PMKnapp DGKõljalg UKovács GMKurtzman CPLandvik SLeavitt SDLiggenstoffer ASLiimatainen KLombard LLuangsa-Ard JJLumbsch HTMaganti HMaharachchikumbura SSMartín MPMay TWMcTaggart ARMethven ASMeyer WMoncalvo JMMongkolsamrit SNagy LGNilsson RHNiskanen TNyilasi IOkada GOkane IOlariaga IOtte JPapp TPark DPetkovits TPino-Bodas RQuaedvlieg WRaja HARedecker DRintoul TRuibal CSarmiento-Ramirez JMSchmitt ISchussler AShearer CSotome KStefani FOStenroos SStielow BStockinger HSuetrong SSuh SOSung GHSuzuki MTanaka KTedersoo LTelleria MTTretter EUntereiner WAUrbina HVágvölgyi CVialle AVu TDWalther GWang QMWang YWeir BSWeiß MWhite MMXu JYahr RYang ZLYurkov AZamora JCZhang NZhuang WYSchindel D.2012. Nuclear ribosomal internal transcribed spacer (ITS) region as a universal DNA barcode marker for Fungi. Proc Natl Acad Sci U S A 109:6241–6246 doi:10.1073/pnas.1117018109
- Schussler A.2001. A new fungal phylum, the Glomeromycota: phylogeny and evolution. Mycologia 105:1413–1421.
- Seifert KACrous PWFrisvad JC.2008. Correcting the impact factors of taxonomic journals by appropriate citation of taxonomy. Persoonia 20:105–105.
- Selosse MAVincenot LÖpik M.2016. Data processing can mask biology: towards better reporting of fungal barcoding data? New Phytol 210:1159–1164 doi:10.1111/nph.13851
- Spang ASaw JHJorgensen SLZaremba-Niedzwiedzka KMartijn JLind AEvan Eijk RSchleper CGuy LEttema TJG.2015. Complex archaea that bridge the gap between prokaryotes and eukaryotes. Nature 521:173–179 doi:10.1038/nature14447
- Stackebrandt EFrederiksen WGarrity GMGrimont PADKampfer PMaiden MCJNesme XRossello-Mora RSwings JTruper HGVauterin LWard ACWhitman WB.2002. Report of the ad hoc committee for the re-evaluation of the species definition in bacteriology. Int J Syst Evol Micr 52:1043–1047 doi:10.1099/00207713-52-3-1043
- Stielow JBLevesque CASeifert KAMeyer WIriny LSmits DRenfurm RVerkley GJGroenewald MChaduli DLomascolo AWelti SLesage-Meessen LFavel AAl-Hatmi AMDamm UYilmaz NHoubraken JLombard LQuaedvlieg WBinder MVaas LAVu DYurkov ABegerow DRoehl OGuerreiro MFonseca ASamerpitak Kvan Diepeningen ADDolatabadi SMoreno LFCasaregola SMallet SJacques NRoscini LEgidi EBizet CGarcia-Hermoso DMartin MPDeng SGroenewald JZBoekhout Tde Beer ZWBarnes IDuong TAWingfield MJde Hoog GSCrous PWLewis CTHambleton SMoussa TAAl-Zahrani HSAlmaghrabi OALouis-Seize GAssabgui RMcCormick WOmer GDukik KCardinali GEberhardt Ude Vries MRobert V.2015. One fungus, which genes? Development and assessment of universal primers for potential secondary fungal DNA barcodes. Persoonia 35:242–263 doi:10.3767/003158515X689135
- Talbot JMBruns TDTaylor JWSmith DPBranco SGlassman SIErlandson SVilgalys RLiao H-LSmith MEPeay KG.2014. Endemism and functional convergence across the North American soil mycobiome. Proc Natl Acad Sci U S A 111:6341–6346 doi:10.1073/pnas.1402584111
- Talbot JMMartin FKohler AHenrissat BPeay KG.2015. Functional guild classification predicts the enzymatic role of fungi in litter and soil biogeochemistry. Soil Biol Biochem 88:441–456 doi:10.1016/j.soilbio.2015.05.006
- Tatusova TCiufo SFederhen SFedorov BMcVeigh RO'Neill KTolstoy IZaslavsky L.2015. Update on RefSeq microbial genomes resources. Nucleic Acids Res 43:D599–D605 doi:10.1093/nar/gku1062
- Taylor DLHollingsworth TNMcFarland JWLennon NJNusbaum CRuess RW.2014. A first comprehensive census of fungi in soil reveals both hyperdiversity and fine-scale niche partitioning. Ecol Monogr 84:3–20 doi:10.1890/12-1693.1
- Taylor JW. 2011. One fungus = one name: DNA and fungal nomenclature twenty years after PCR. IMA Fungus 2:113–120 doi:10.5598/imafungus.2011.02.02.01
- Taylor JWJacobson DJKroken SKasuga TGeiser DMHibbett DSFisher MC.2000. Phylogenetic species recognition and species concepts in fungi. Fungal Genet Biol 31:21–32 doi:10.1006/fgbi.2000.1228
- Tedersoo LBahram MPõlme SKõljalg UYorou NSWijesundera RRuiz LVVasco-Palacios AMThu PQSuija ASmith MESharp CSaluveer ESaitta ARosas MRiit TRatkowsky DPritsch KPõldmaa KPiepenbring MPhosri CPeterson MParts KPärtel KOtsing ENouhra ENjouonkou ALNilsson RHMorgado LNMayor JMay TWMajuakim LLodge DJLee SSLarsson K-HKohout PHosaka KHiiesalu IHenkel TWHarend HGuo L-dGreslebin AGrelet GGeml JGates GDunstan WDunk CDrenkhan RDearnaley JDe Kesel ADang TChen XBuegger FBrearley FQBonito GAnslan SAbell SAbarenkov K.2014. Global diversity and geography of soil fungi. Science 346:1256688 doi:10.1126/science.1256688
- Tedersoo LRamirez KSNilsson RHKaljuvee AKõljalg UAbarenkov K.2015. Standardizing metadata and taxonomic identification in metabarcoding studies. GigaScience 4:1–4.
- Thiéry OVasar MJairus TDavison JRoux CKivistik P-AMetspalu AMilani LSaks ÜMoora MZobel MÖpik M.2016. Sequence variation in nuclear ribosomal small subunit, internal transcribed spacer and large subunit regions of Rhizophagus irregularis and Gigaspora margarita is high and isolate-dependent. Mol Ecol 25:2816–2832 doi:10.1111/mec.13655
- Tindall BJRossello-Mora RBusse HJLudwig WKampfer P.2010. Notes on the characterization of prokaryote strains for taxonomic purposes. Int J Syst Evol Micr 60:249–266 doi:10.1099/ijs.0.016949-0
- Veresoglou SDHalley JMRillig MC.2015. Extinction risk of soil biota. Nat Commun 6:8862 doi:10.1038/ncomms9862
- Wang QGarrity GMTiedje JMCole JR.2007. Naive Bayesian classifier for rapid assignment of rRNA sequences into the new bacterial taxonomy. Appl Environ Microbiol 73:5261–5267 doi:10.1128/AEM.00062-07
- Wayne LGBrenner DJColwell RRGrimont PADKandler OKrichevsky MIMoore LHMoore WECMurray RGEStackebrandt EStarr MPTruper HG.1987. Report of the ad hoc committee on reconciliation of approaches to bacterial systematics. Int J Syst Evol Micr 37:463–464 doi:10.1099/00207713-37-4-463
- White TJBruns TLee STaylor J.1990 Amplification and firect sequencing of fungal ribosomal RNA genes for phylogenies. In: Innis MAGelfand DHSninsky JJWhite TJ eds. PCR protocols. San Diego, California: Academic Press. p 315–322.
- Whitman WB.2015. Genome sequences as the type material for taxonomic descriptions of prokaryotes. Syst Appl Microbiol 38:217–222 doi:10.1016/j.syapm.2015.02.003
- Whitman WB.2016. Modest proposals to expand the type material for naming of prokaryotes. Int J Syst Evol Micr 66:2108–2112 doi:10.1099/ijsem.0.000980
- Wieczorek JBloom DGuralnick RBlum SDöring MGiovanni RRobertson TVieglais D.2012. Darwin Core: an evolving community-developed biodiversity data standard. PLoS One 7:e29715 doi:10.1371/journal.pone.0029715
- Yarza PLudwig WEuzéby JAmann RSchleifer K-HGlöckner FORosselló-Móra R.2010. Update of the all-species living tree project based on 16S and 23S rRNA sequence analyses. Syst Appl Microbiol 33:291–299 doi:10.1016/j.syapm.2010.08.001
- Yarza PRichter MPeplies JEuzeby JAmann RSchleifer K-HLudwig WGlöckner FORosselló-Móra R.2008. The All-Species Living Tree project: a 16S rRNA-based phylogenetic tree of all sequenced type strains. Syst Appl Microbiol 31:241–250 doi:10.1016/j.syapm.2008.07.001
- Yarza PSpröer CSwiderski JMrotzek NSpring STindall BJGronow SPukall RKlenk H-PLang EVerbarg SCrouch ALilburn TBeck BUnosson CCardew SMoore ERBGomila MNakagawa YJanssens DDe Vos PPeiren JSuttels TClermont DBizet CSakamoto MIida TKudo TKosako YOshida YOhkuma MArahal DRSpieck EPommerening Roeser AFigge MPark DBuchanan PCifuentes AMunoz REuzéby JPSchleifer K-HLudwig WAmann RGlöckner FORosselló-Móra R.2013. Sequencing orphan species initiative (SOS): filling the gaps in the 16S rRNA gene sequence database for all species with validly published names. Syst Appl Microbiol 36:69–73 doi:10.1016/j.syapm.2012.12.006
- Yilmaz PKottmann RField DKnight RCole JRAmaral-Zettler LGilbert JAKarsch-Mizrachi IJohnston ACochrane GVaughan RHunter CPark JMorrison NRocca-Serra PSterk PArumugam MBailey MBaumgartner LBirren BWBlaser MJBonazzi VBooth TBork PBushman FDButtigieg PLChain PSGCharlson ECostello EKHuot-Creasy HDawyndt PDeSantis TFierer NFuhrman JAGallery REGevers DGibbs RAGil ISGonzalez AGordon JIGuralnick RHankeln WHighlander SHugenholtz PJansson JKau ALKelley STKennedy JKnights DKoren OKuczynski JKyrpides NLarsen RLauber CLLegg TLey RELozupone CALudwig WLyons DMaguire EMethe BAMeyer FMuegge BNakielny SNelson KENemergut DNeufeld JDNewbold LKOliver AEPace NRPalanisamy GPeplies JPetrosino JProctor LPruesse EQuast CRaes JRatnasingham SRavel JRelman DAAssunta-Sansone SSchloss PDSchriml LSinha RSmith MISodergren ESpor AStombaugh JTiedje JMWard DVWeinstock GMWendel DWhite OWhiteley AWilke AWortman JRYatsunenko TGlockner FO.2011. Minimum information about a marker gene sequence (MIMARKS) and minimum information about any (x) sequence (MIxS) specifications. Nat Biotechnol 29:415–420 doi:10.1038/nbt.1823
- Zhang JKapli PPavlidis PStamatakis A.2013. A general species delimitation method with applications to phylogenetic placements. Bioinformatics 29:2869–2876 doi:10.1093/bioinformatics/btt499