Abstract
The ideal method to estimate direct genomic values (DGV) would calculate the conditional mean of the breeding value given the genotype of individuals at each quantitative traits locus (QTL). In this study we compare accuracies of DGV obtained using three different prior distributions of the single-nucleotide polymorphism (SNP) effects (normal, Student’s t and double-exponential) in simulated data, to understand the extent of reduction in DGV accuracy when the prior distribution does not match the true distribution of QTL effects. We then apply the methods in a real dataset of 1149 Australian Holstein-Friesian bulls, both to find the prior distribution that is most robust across traits and to make interpretations about the true distribution of QTL effects. Methods using normal and Student’s t prior distributions had fixed hyper-parameters, whereas hyper-parameters for double-exponential prior distribution were conditional to the data. Using the Student’s t distribution for the prior distribution of SNP effects gave the largest estimates of SNP effects in the presence of QTL with large effects in both simulated and real data, and achieved the best accuracies of DGV in both datasets. The double-exponential distribution resulted in higher shrinkage of SNP effect estimates, even when a large true effect was present. The normal distribution resulted in the greatest degree of shrinkage of estimated effects, and gave the lowest accuracies. The amount of information of the data analyzed might still be inadequate to estimate these hyper-parameters accurately. A Student’s t distribution with fixed hyper-parameters was the best approximation of the QTL distribution for the two dairy traits analyzed.
Introduction
The recent availability of dense genome-wide single-nucleotide polymorphism (SNP) panels has allowed implementation of genomic selection (GS) in a number of livestock breeding programs worldwide (Harris et al., Citation2008; Legarra et al., Citation2008; Van Raden et al., Citation2009; Hayes et al., Citation2009; van der Werf, Citation2009; Gonzalez-Recio et al., Citation2009). The best statistical method for the estimation of direct genomic values (DGV) for selection candidates is still under discussion. Goddard and Hayes (Citation2007) argued that the ideal method to estimate breeding values from genomic data would calculate the conditional mean of the breeding value given the genotype of individuals at each quantitative traits locus (QTL). This conditional mean requires a prior distribution of QTL effects. However, in practice, both genotypes at QTLs and the distribution of QTL effects are unknown, with SNP markers being used to track the QTL through linkage disequilibrium. Meuwissen et al. (Citation2001) evaluated Bayesian methods either with a normal (BLUP), a Student’s t (BayesA), or a 0-t mixture distribution of marker effects (e.g., assigning a 0 variance to a percentage of markers assumed not linked to any QTL and a Student’s t distribution for the others; BayesB) in simulated data. Their results indicated that BayesA and BayesB performed better than BLUP. However, these results could reflect the simulated distribution of QTL effects they used, which was a small number of QTL of moderate to large effect. On the other hand, results reported from real data show in general small differences in the accuracy of DGV from non-linear Bayesian models and BLUP models, depending on the trait analyzed (VanRaden et al., Citation2009; Hayes et al., Citation2009; Luan et al., Citation2009; Gredler et al., Citation2009; Macciotta et al., Citation2010; Habier et al., Citation2010; Pintus et al., 2012, Citation2013; Gaspa et al., Citation2013). VanRaden et al. (Citation2009) tested both a BLUP and a non-linear model (similar to BayesA) over 27 traits in dairy cattle. They showed that predictions using non-linear model were more accurate for some traits highly affected by QTL of large effects (i.e. fat and protein percentage). However, considering the results obtained across all 27 traits, only 1% average difference was observed between BLUP and non-linear models. Also in data from dairy cattle, Hayes et al. (Citation2009) obtained higher accuracies with BayesA in Australian Selection Index (ASI), Australian Profit Ranking (APR), protein yield and protein percentage indexes. Accuracy differences between BayesA and BLUP models ranged from a minimum of 2% in APR to a maximum of 7% in protein percentage. Opposite results were obtained for female fertility, where BLUP obtained a 4% higher accuracy than BayesA. These results indicate that, when dealing with real data, more flexible (or different) assumptions are needed to account for the different QTL distributions (and heritability) of complex traits. One potential solution is a two or three level hierarchical model, called the Bayesian LASSO (Park and Casella, Citation2008), a Bayesian counterpart of the original least absolute shrinkage and selection operator (LASSO; Tibshirani, Citation1996). The Bayesian LASSO assumes a double exponential prior distribution for SNP effects. Park and Casella (Citation2008) describe a computationally efficient approach to implement the Bayesian LASSO using a hierarchical approach, whereby SNP effects are sampled from a normal distribution with a SNP specific variance, and these prior variances of marker effects are in turn sampled from an exponential distribution. The exponential distribution has a regularization hyper-parameter λ, which is sampled conditional on the data. Whereas in BLUP, BayesA and BayesB hyper-parameters for SNP marker variances are fixed (e.g., degrees of freedom and scale of the scaled inverted chi-squared distribution are assumed known), in the Bayesian Lasso the hyper-parameter of the marker variance exponential distribution is considered unknown and sampled from a distribution. In fact, the regularization hyper-parameter plays a key role in the model, as the degree of shrinkage of the estimates will be determined by the information in the data itself. In simulated data, de los Campos et al. (Citation2009) tested five sets of parameters and two different distributions (gamma and beta distributions) for the prior of the regularization hyper-parameter, observing only small differences in terms of SNP effect estimates. They also analyzed the effect of the inclusion of a polygenic term in the Bayesian LASSO model, on wheat and mice real datasets. Their results (using only beta prior distribution for the regularization parameter) indicated that the inclusion of a polygenic effect increased the predictive ability of the model.
In this paper we compare accuracies of DGV and estimates of SNP effects in both simulated and real scenarios using three widely used models for GS: Bayesian Gaussian Regression (BGR, with assumptions similar to BLUP; Verbyla et al., Citation2010), BayesA (Meuwissen et al., Citation2001) and two Bayesian LASSO (de los Campos et al., Citation2009). These models have different hyper-parameter prior distributions and different definition of parameters indexing the hyper-parameters distribution, as discussed in de los Campos et al. (Citation2009). Since we are comparing accuracies with three different prior distributions of SNP effects (i.e., Normal distribution in BGR, Student’s t in BayesA and double-exponential in Bayesian LASSO), an additional objective is to gain some insight into the distribution of QTL effects for the traits analyzed in the real dataset.
Materials and methods
Methods were tested on both simulated and real data. First, a simulated dataset was used to study the properties of the methods in a scenario with known QTL positions and effects. Then, genotypes, phenotypes and pedigree information of Australian Holstein-Friesian bulls were analyzed for two production traits to test the performance of all methods using real data.
Simulated dataset
The simulated dataset, provided by the XII QTL-MAS workshop (QTL-MAS, Citation2008), comprised 5865 individuals structured in 7 generations. A total of 46 QTL with additive effect and 2 QTL with epistatic effect were distributed along the simulated genome. QTL effects were drawn from a Gamma distribution using Hayes and Goddard (Citation2001) parameters. Pedigree relationships and genotypes at 6000 SNP evenly distributed across six chromosomes were available for all individuals, whereas phenotypic information was provided for the first 4 generations only. All 4665 individuals from the first 4 generations were considered as training animals and individuals from the last 3 generations as prediction young animals.
Phenotypes were pre-corrected with estimates of fixed effects derived from the following fixed- effects model:
where y is the original phenotype for the ith animal; SEX is the fixed effect of sex (p=1,2); GEN is the fixed effect of generation (j=0-6); and e is the random residual. The corrected phenotype for each animal was considered as:
True breeding values (TBV), were available for all animals. QTL effects considered in this study where those reported by Crooks et al. (2010). Accuracies were obtained by calculating the correlation between DGV and TBV (r(DGV,TBV)). Prediction bias was assessed by calculating the regression coefficient of TBV on DGV.
Real dataset
A total of 1250 progeny tested Australian Holstein-Friesian bulls born between 1950 and 2005 were genotyped with the Illumina Bovine SNP50TM chip (54K). SNP were eliminated from the dataset if they had more than 10% of missing genotypes, less than 1% of MAF and extreme values for χ2-test for Hardy-Weinberg equilibrium. Mendelian inheritance of SNP was investigated and bulls with genotype incompatible with pedigree were eliminated. A total of 1149 animals and 39,048 SNP were retained for the analysis. The 763 older bulls (born between 1950 and 2002) where considered as reference population and the rest as validation population. Dependent variables (e.g., phenotypes) were de-regressed proofs of Australian Breeding Values (ABV), with the de-regression removing contribution from relatives other than daughters (see Hayes et al. Citation2009, for details). Accuracies were obtained as correlation between DGV and ABV. Regression coefficients of ABV on DGV were calculated to investigate bias of predictions. Traits analyzed were protein yield and fat percentage. These two traits were chosen as there is some information about a different distribution of QTL effects for these traits (Grisart et al., Citation2002; Viitala et al., Citation2006; Thaller et al., Citation2003; Cole et al., Citation2009), thus making them ideal for our goals.
Description of models
The following model was fitted:
where:
y is a vector of phenotypes (ABV) in the reference population;
1n is a vector of ones;
µ is the general mean;
g is a vector of (random) SNP effects;
X is the corresponding design matrix with elements of Xij=0,1,2 for genotypes 11, 12 and 22, respectively for the ith animal and jth SNP;
u is a vector of polygenic breeding values assumed to be normally distributed, with ui ˜N(0,Aσ2a), where A is the average relationship matrix and σ2a is the additive genetic variance; Z is the corresponding design matrix linking polygenic breeding values to the data; and e is a vector of random residuals, with ei ˜ N(0,σ2e), where σ2e is the residual variance. Direct genomic values were calculated as:
The BGR method assumed a normal prior distribution of SNP effects (maintaining BLUP infinitesimal assumptions). The variance of this normal distribution was sampled in each iteration of the Gibbs Sampler (Verbyla et al., Citation2010). BayesA prior structure and (fixed) hyper-parameters followed Meuwissen et al. (Citation2001), thus, degrees of freedom were set to 4.012.
The Bayesian LASSO (B-LASSOgamma) was defined as follows (after de los Campos et al. Citation2009):
where N(yi| m+x’ig+ui,s2 e) is a normal distribution with mean m+x’ig+ui and variance s2 e;N( m| 0,s2 u), N(gj| 0,s2 e t2 j) and N(u| 0, A,s2 u) are normal distributions for general mean, SNP effects and polygenic breeding values, with null mean and variances s2 u,o2 e t2 j and As2 u, respectively; X–2( s2 e|dofe,Se) and X–2( s2 u|dofu,Su) are scaled inverted chisquared distributions with degrees of freedom dof and scale parameter S, for random residual and polygenic variances, respectively; Exp ( t2 j| l) is an exponential distribution for marker variances, controlled by a single parameter l (the regularization parameter); and G ( l2| a1 a2) is a Gamma distribution with a1 and a2 as shape and rate parameters, respectively. The above conditional distributions have a closed form, thus, a Gibbs Sampler can be used to solve the equations. Shape and scale parameters for the regularization hyperparameter hyperparameter λ were defined as p(λ2|P,S)μ G(λ2 | P=0.1, S=1¥10–04).
A modification to B-LASSOgamma tested in de los Campos et al. (Citation2009), was a more flexible Beta prior distribution for the hyper-parameter λ. We tested this method as well (BLASSObeta). This distribution allows setting a relative flat prior in a wider range of values than Gamma distribution. In this case, parameters used for l hyper-prior distribution were p(l|max,a1,a2) μ Beta((l|max=400) | a1=1.4, a2=1.4). A Metropolis-Hastings step was required because the Beta distribution is not a conjugate prior. Further details on both Bayesian LASSO methods applied in this study are available in de los Campos et al. (Citation2009).
A total of 20,000 runs of iteration were performed for each method under study. The first 10,000 iterations were discarded as burn-in, and no thinning interval was considered.
Results
Simulated dataset
The Student’s t prior distribution of SNP effects assumed in BayesA allowed to obtain the largest magnitude of effects in the presence of QTL with true moderate to large effects (). BayesA also estimated QTL with smaller effects most accurately (), obtained the highest accuracies of DGV (0.87) and the lowest bias ().
Accuracy of DGV obtained with the two Bayesian LASSO methods were lower than those obtained with BayesA. The degree of shrinkage of marker estimates with the Bayesian LASSO was greater than BayesA in the presence of QTL with large effects, with the largest QTL effects being severely underestimated (,). In fact, marker effects in these regions were on average 75% lower than those obtained with BayesA, and substantially lower than the true effects of the QTL (). This led to accuracies 10% lower (and higher bias) than those obtained with BayesA (). The two distributions of the λ hyper-parameter did not influence the results: negligible differences were observed in posterior estimates of λ from B-LASSOgamma and B-LASSObeta.
The BGR method gave accuracies 3% lower than the Bayesian LASSO methods. Marker effect estimates in QTL regions with large effects were always lower from BayesA, but in general they were similar to the estimates obtained in both Bayesian LASSO methods (). The correlation between DGV from BGR and Bayesian LASSO methods was 0.96, whereas the correlation between DGV from BGR and BayesA was lower at 0.87.
Real dataset
For fat percentage, BayesA identified three SNPs with large to moderate effects: one on BTA14 (i.e. in the diacylglycerol-acyltrans-ferase 1 region; DGAT1), one on BTA20 (i.e. in the growth hormone receptor region, GHR) and one on BTA5. A mutation with moderate to large effect on fat percentage in the DGAT1 gene has been previously reported (Grisart et al., Citation2002). Marker effects of flanking SNP in these regions were close to zero (). On the other hand, both Bayesian LASSO methods identified 32 SNP in the DGAT1 region (with the highest effect obtaining one third of the effect observed in BayesA) and one SNP in the GHR region (with much lower estimated effect than in BayesA), however failing to identify SNP with relative high effects on BTA5 (,). The distribution of effects in BGR were similar to those from Bayesian LASSO methods, with a total of 35 SNP with relative high effect in the DGAT1 and GHR regions, and no SNP with relative high effect in BTA5 (). However, the magnitude of the highest SNP effects was more than ten-fold lower.
The influence of the DGAT1 mutation was also evident on protein yield (). Again, BayesA identified only a single SNP with a negative effect in this region, whereas Bayesian LASSO and BGR methods showed a similar pattern grouping a large number of SNPs with relatively large, but smaller than BayesA, effects on BTA14. In general, for protein yield, SNP estimates of both Bayesian LASSO and BGR showed only a slight difference in terms of magnitude of SNP effects.
A difference in posterior estimates of the regularization hyper-parameter λ was observed between B-LASSOgamma and B-LASSObeta for both fat percentage and protein yield (, ). Higher penalization was observed in protein yield (128.72 and 108.47, in B-LASSOgamma and B-LASSObeta, respectively) rather than in fat percentage (100.48 and 70.04 in the aforementioned methods). Furthermore, posterior estimates of λ were more variable (i.e. higher standard deviation of posterior estimates after burn-in) in protein yield than in fat percentage. These results could reflect the distribution of QTL structure for the traits. The conditional regularization hyper-parameter obtained for fat percentage, is likely to be driven by the few QTL of large effect that affect the trait, resulting in a reduced (and more stable) penalization parameter.
BGR obtained the lowest accuracies of DGV (-16% respect to BayesA and -12% respect to both Bayesian LASSO) and the highest bias for fat percentage. For protein yield, however, differences in terms of accuracy of DGV were greatly reduced (-5% with BayesA and -1% with B-LASSOgamma).
Discussion
Bayesian LASSO methods have been successfully tested in real data for QTL mapping studies (Yi and Xu, Citation2008), to test genomic selection in wheat, maize, mice and cows (de los Campos et al., Citation2009; Weigel et al., Citation2009; Crossa et al., Citation2010; Weigel et al., Citation2010; Cleveland et al., Citation2010), for GWA studies and to choose sub-sets of SNP for genomic predictions in livestock (Li et al., Citation2011; Weigel et al., Citation2009; Vazquez et al., Citation2010). Cleveland et al. (Citation2010) observed a tendency of the Bayesian LASSO methods to obtain SNP effects of lower magnitude than BayesA where the true QTL effect were moderate to large. The same trend was observed in our study. However, DGV accuracy results in both studies do not agree, most probably because of differences in the model and the simulated dataset used. In any case, both simulated datasets are likely to be far from the reality for complex traits, as the number of SNP and QTL was very limited compared to real data. In our case, for example, the XII QTL-MAS dataset included four QTL explained more than 50% of the genetic variability of the trait. However, using the simulated data did allow us to study and compare the different performances of all methods in a simple dataset with a known distribution of QTL effects. In this scenario, BayesA more accurately estimated the effects of QTL, in fact obtaining best DGV accuracies and the lowest bias. On the other hand, both Bayesian LASSO methods and BGR resulted in very similar SNP estimates and DGV accuracy results. These results were markedly lower than those obtained with BayesA method, as expected in a trait highly affected by few QTL, as BayesA resulted in much less shrinkage of estimates of large to moderate QTL effects than either BGR or Bayesian LASSO.
In this study we also analyzed milk fat percentage and protein yield in Australian Holstein Friesian dairy cattle, as there is some information about the distribution of QTL effects for these traits. For fat percentage, a mutation in DGAT1 explains up to 30% of the variation of the trait (Grisart et al., Citation2002). Another interesting QTL for fat percentage is located on BTA20, in the region of GHR (Viitala et al., Citation2006). However, no such large or moderate-effect QTL are present for protein yield, although there is evidence that DGAT1 contributes to the negative correlation between these two traits (Thaller et al., Citation2003; Cole et al., Citation2009). Thus, known QTL regions with large effects in the traits analyzed, accuracies and bias results can be used to compare the performance of all methods tested in this study. All methods identified the DGAT1 and GHR regions, although with large differences in the number of SNPs involved and magnitude of the effects. In addition, BayesA identified a third region with a high effect on BTA5 for fat percentage. This SNP is positioned at 101,042,396bp, which is within a region where two QTL with large confidence intervals have been reported for milk and fat yield (CattleQTLdb, Citation2013; Olsen et al., Citation2002; Viitala et al., Citation2003).
There are two possible explanations for why BayesA gives a large effect to only one SNP in the DGAT1 region while the Bayesian LASSO methods identify many more SNP. One is that the prior in BayesA is flexible enough to allow all of the mutation effect to be captured by one SNP in high LD, while the prior for the Bayesian LASSO methods shrink the effects so hard that even when this SNP effect is removed, there is some effect of the mutation remaining, and thus this effect is then distributed over other SNP which are in LD with the mutation. An alternative explanation would be that the prior used in BayesA results in poor mixing during Gibbs sampling, such that once the effect of the mutation is allocated to one SNP, the following SNP in the chain never receive an effect (ter Braak et al., Citation2005). In this case the Bayesian LASSO results would indicate better mixing. If the mutation itself were genotyped and included in the data, we could determine which of the explanations is correct.
Using the double exponential prior distribution in Bayesian LASSO resulted in a degree of shrinkage of SNP effect estimates that was much higher than BayesA. However, this method gave only 4% lower accuracies than BayesA for both fat percentage and protein yield. Regularization hyper-parameter differences in both Beta and Gamma hyper-prior structures did not influence accuracy results. In simulated data, de los Campos et al. (Citation2009) noted that inferences on SNP effects were robust over a large range of values of the regularization hyper-parameters. For example, their results in simulated data showed nearly twofold differences of λ posterior estimates in models using Gamma and Beta prior distributions similar to those reported in this study, although obtaining similar accuracies. Our results in the dairy data are in agreement with their observations.
As previously reported, the normal prior distribution used in the BGR method gave the lowest DGV accuracy for fat percentage, compared to methods that allowed SNP effects to assume non-normal distributions (VanRaden et al., Citation2009; Hayes et al., Citation2009). Interestingly, estimates of SNP effects from the Bayesian LASSO for protein yield were very similar to BGR for protein yield, a trait not controlled by QTL with large effects (in fact, the accuracies of DGV were only 1% higher, for both B-LASSOgamma and B-LASSObeta).
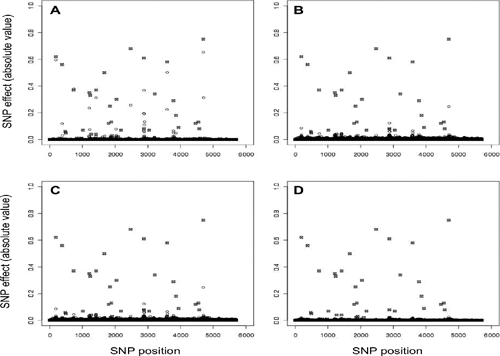
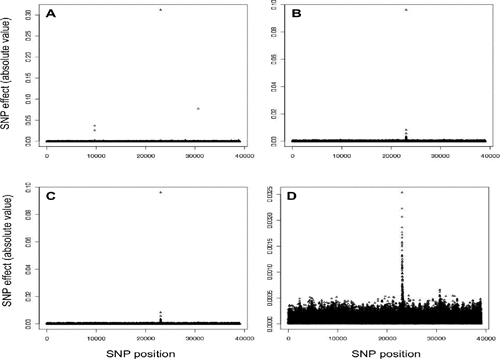
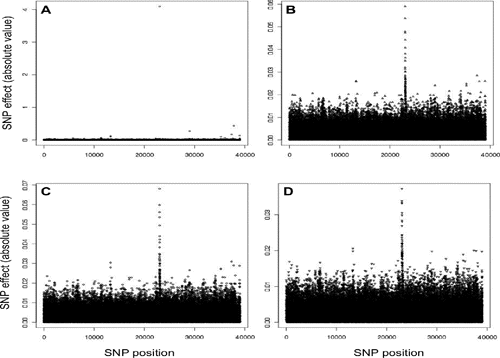
Table 1. Absolute SNP effects in simulated QTL regions with high effect.
Table 2. Accuracies, regression coefficients and regularization parameters obtained in the simulated dataset.
Table 3. Accuracies, regression coefficients and regularization parameters obtained in the real dataset for fat percentage.
Table 4. Accuracies, regression coefficients and regularization parameters obtained in the real dataset for protein yield.
Conclusions
An appealing feature of Bayesian LASSO methods as described by Park and Casella (Citation2008) is that hyper parameters of the double exponential prior distribution of SNP effects are conditional to the data. On the contrary, in methods such as BayesA, the leptokurtosis of the Student t prior must be specified a priori. However, with the limited amount of data in our study, the Bayesian LASSO methods resulted in strong shrinkage of SNP effect estimates, which in some cases was similar to what observed in BGR. With large datasets there would be more information from the data to condition the hyper-parameters, which may result in more optimal shrinkage. The Bayesian LASSO methods may be particularly useful with next generation SNP-chips (i.e. with many more SNP than analyzed here in much greater linkage disequilibrium with QTL) and larger datasets.
Acknowledgments
The authors wish to thank Phil Bowman for his help in coding the scripts. Gustavo de los Campos and Christian Maltecca are acknowledged for their help in setting up the Bayesian Lasso methods. ELN was funded by AGRISYSTEM PhD fellowship (13th Cycle) and by grants SELMOL and INNOVAGEN of the Ministry of Agricultural, Food and Forestry Policies (MIPAAF, Italy).
References
- CattleQTLdb, 2013. Animal Quantitative Trait Locus database (Bovine), Release 13. Available from: http://www.animalgenome.org/cgi-bin/gbrowse/cattle/
- ClevelandM. ForniS. DeebN. MalteccaC. 2010. Genomic breeding value prediction using three Bayesian methods and application to reduced density marker panels. BMC Proceedings 4(Suppl.1):S6.
- ColeJ.B. VanRadenP.M. O’ConnellJ.R. Van TasselC.P. SonstegardT.S. SchnabelR.D. TaylorJ.F. WiggansG.R. 2009. Distribution and location of genetic effects for dairy traits. J. Dairy Sci. 92:2931-2946.
- CrooksL. SahanaG. de KoningD. LundM.S. CarlborgÖ. 2009. Comparison of analyses of the QTL-MAS XII common dataset. II: genome-wide association and fine mapping. BMC Proceedings 3(Suppl.1):S2.
- CrossaJ. de los CamposG. PerezP. GianolaD. BurguenoJ. ArausJ.L. MakumbiD. SinghR. DreisigackerS. YanJ. AriefV. BanzigerM. BraunH.J. 2010. Prediction of genetic values of quantitative traits in plant breeding using pedigree and molecular markers. Genetics 186:713-724.
- de los CamposG. NayaH. GianolaD. CrossaJ. LegarraA. ManfrediE. WeigelK. CotesJ.M. 2009. Predicting quantitative traits with regression models for dense molecular markers and pedigree. Genetics 182:375-385.
- GaspaG. PintusM.A. NicolazziE.L. VicarioD. ValentiniC. MacciottaN.P.P. 2013. Use of principal component approach to predict direct genomic breeding values for beef traits in Italian Simmental cattle. J. Anim. Sci. 91:29-37.
- GoddardM.E. HayesB.J. 2007. Genomic selection. J. Anim. Breed. Genet. 124:323-330.
- Gonzàles-RecioO. GianolaD. RosaG.J. WeigelK.A. KranisA. 2009. Genome-assisted prediction of a quantitative trait measured in parents and progeny: application to food conversion rate in chickens. Genet. Sel. Evol. 41:3.
- GredlerB. NireaK.G. SolbergT.R. Egger-DannerC. MeuwissenT.H.E. SölknerJ.A. 2009. Comparison of methods for genomic selection in Austrian dual purpose Simmental cattle. Proc. 18th Nat. Conf. of Ass. Advanc. Anim. Breed. Genet., Barossa Valley, South Australia, 18:568-571.
- GrisartB. CoppietersW. FarnirF. KarimL. FordC. BerziP. CambisanoN. MniM. ReidS. SimonP. SpelmanR. GeorgesM. SnellR. 2002. Positional candidate cloning of a QTL in dairy cattle: identification of a missense mutation in the bovine DGAT1 gene with major effect on milk yield and composition. Genome Res. 12:222-231.
- HabierD. TetensJ. SeefriedF. LichtnerP. ThallerG. 2010. The impact of genetic relationship information on genomic breeding values in German Holstein cattle. Genet. Sel. Evol. 42:5.
- HarrisB.L. JohnsonD.L. SpelmanR.J. 2008. Genomic selection in New Zealand and the implications for national genetic evaluation. Proc. 36th ICAR Biennial Session, Niagara Falls, Canada, 38:325-330.
- HayesB.J. GoddardM.E. 2001. The distribution of the effects of genes affecting quantitative traits in livestock. Genet. Sel. Evol. 33:209-229.
- HayesB.J. BowmanP.J. ChamberlainA.J. GoddardM.E. 2009. Invited review: Genomic selection in dairy cattle: progress and challenges. J. Dairy Sci. 92:433-445.
- LegarraA. Robert-GranieC. ManfrediE. ElsenJ.M. 2008. Performance of genomic selection in mice. Genetics 180:611-618.
- LiJ. DasK. FuG. LiR. WuR. 2011. The Bayesian lasso for genome-wide association studies. Bioinformatics 27:516-523.
- LuanT. WooliamsJ. LienS. KentM. SvendsenM. MeuwissenT. 2009. The accuracy of genomic selection in Norwegian red cattle assessed by cross-validation. Genetics 183:1119-1126.
- MacciottaN.P.P. GaspaG. SteriR. NicolazziE.L. DimauroC. PieramatiC. Cappio-BorlinoA. 2010. Using eigenvalues as variance priors in the prediction of genomic breeding values by principal component analysis. J. Dairy Sci. 93:2765-2774.
- MeuwissenT.H.E. HayesB.J. GoddardM.E. 2001. Prediction of total genetic values using genome-wide dense marker maps. Genetics 157:1819-1829.
- OlsenH.G. Gomez-RayaL. VageD.I. OlsakerI. KlunglandH. SvendsenM. AdnoyT. SabryA. KlemetsdalG. SchulmanN. KramerW. ThallerG. RonningenK. LienS. 2002. A genome scan for quantitative trait loci affecting milk production in Norwegian dairy cattle. J. Dairy Sci. 85:3124-3130.
- ParkT. CasellaG. 2008. The Bayesian Lasso. J. Am. Stat. Assoc. 103:681-686.
- PérezP. de los CamposG. CrossaJ. GianolaD. 2010. Genomic-enabled prediction based on molecular markers and pedigree using the Bayesian linear regression package in R. Plant Gen. 2:106-116.
- PintusM.A. NicolazziE.L. Van KaamJ.B. BiffaniS. StellaA. GaspaG. DimauroC. MacciottaN.P. 2013. Use of different statistical models to predict direct genomic values for productive and functional traits in Italian Holstein. J. Anim. Breed Genet. 130:32-40.
- ter BraakC.J.F. BoerM.P. BinkM.C.A.M. 2005. Extending Xu’s Bayesian model for estimating polygenic effects using markers of the entire genome. Genetics 170:1435-1438.
- ThallerG. KrämerW. WinterA. KaupeB. ErhardtG. FriesR. 2003. Effects of DGAT1 variants on milk production traits in German cattle breeds. J. Anim Sci. 81:1911-1918.
- TibshiraniR. 1996. Regression shrinkage and selection via the Lasso. J. R. Stat. Soc. 58:267-288.
- van der WerfJ.H.J. 2009. Potential benefit of genomic selection in sheep. Proc. 18th Nat. Conf. of Ass. Advanc. Anim. Breed. Genet., Barossa Valley, South Australia, 18:38-41.
- VanRadenP.M. Van TassellC.P. WiggansG.R. SonstegardT.S. SchnabelR.D. TaylorJ.F. SchenkelF. 2009. Invited review: Reliability of genomic predictions for North American Holstein bulls. J. Dairy Sci. 92:16-24.
- VazquezA.I. RosaG.J.M. WeigelK.A. de los CamposG. GianolaD. AllisonD.B. 2010. Predictive Ability of Subsets of SNP with and without Parent Average in US Holsteins. J. Dairy Sci. 93:5942-5949.
- VerbylaK. BowmanP.J. HayesB.J. GoddardM.E. 2010. Sensitivity of genomic selection to using different prior distributions. BMC Proceedings 4(Suppl.1):S5.
- ViitalaS. SzydaJ. BlottS. SchulmanN. LidauerM. Mäki-TanilaA. GeorgesM. VilkkiJ. 2006. The role of the bovine growth hormone receptor and prolactin receptor genes in milk, fat and protein production in Finnish Ayrshire dairy cattle. Genetics 173:2151-2164.
- ViitalaS.M. SchulmanN.F. de KoningD.J. EloK. KinosR. VirtaA. VirtaJ. Maki-TanilaA. VilkkiJ.H. 2003. Quantitative trait loci affecting milk production traits in Finnish Ayrshire dairy cattle. J. Dairy Sci. 86:1828-1836.
- WeigelK.A. de los CamposG. Gonzàles-RecioO. NayaH. WuX.L. LongN. RosaG.J.M. GianolaD. 2009. Predictive ability of direct genomic values for lifetime net merit of Holstein sires using selected subsets of single nucleotide polymorphism markers. J. Dairy Sci. 92:5248-5257.
- WeigelK.A. de los CamposG. VazquezA.I. RosaG.J.M. GianolaD. Van TassellC.P. 2010. Accuracy of direct genomic values derived from imputed single nucleotide polymorphism genotypes in Jersey cattle. J. Dairy Sci. 93:5423-5435.
- QTL-MAS, 2008. Proc. 12th Eur. Workshop, Uppsala, Sweden. Available from:http://www.computationalgenetics.se/QTLMAS 08/QTLMAS/DATA.html
- YiN. XuS. 2008. Bayesian LASSO for quantitative trait loci mapping. Genetics 179:1045-1055.