Abstract
This article covers the very recent developments undertaken for estimating tropical deforestation from Earth observation data. For the United Nations Framework Convention on Climate Change process it is important to tackle the technical issues surrounding the ability to produce accurate and consistent estimates of GHG emissions from deforestation in developing countries. Remotely-sensed data are crucial to such efforts. Recent developments in regional to global monitoring of tropical forests from Earth observation can contribute to reducing the uncertainties in estimates of carbon emissions from deforestation. Data sources at approximately 30 m × 30 m spatial resolution already exist to determine reference historical rates of change from the early 1990s. Key requirements for implementing future monitoring programs, both at regional and pan-tropical regional scales, include international commitment of resources to ensure regular (at least yearly) pan-tropical coverage by satellite remote sensing imagery at a sufficient level of detail; access to such data at low-cost; and consensus protocols for satellite imagery analysis.
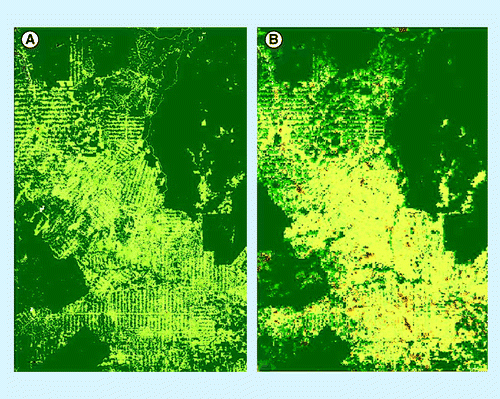
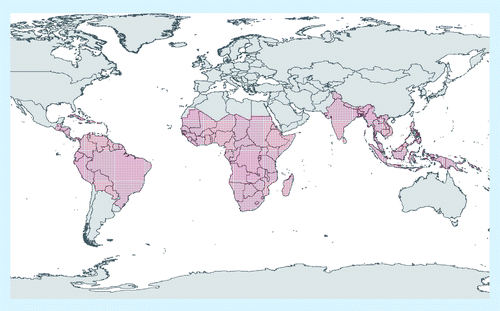
ETM+ Enhanced thermic mapper; TM: Thermic mapper.
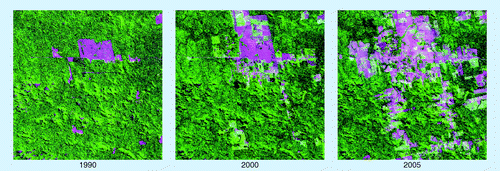
(A) 1990 and (B) 2000 (thermic mapping and enhanced thermic mapping plus) and (C) Advanced Land Observing Satellite Phased Array L-band Synthetic Aperture Radar Mosaic at approximately 50 m × 50 m resolution of 2007 over a 20 km × 20 km size sample unit in Congo Basin.
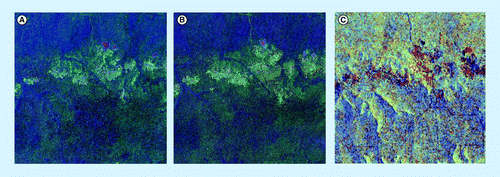
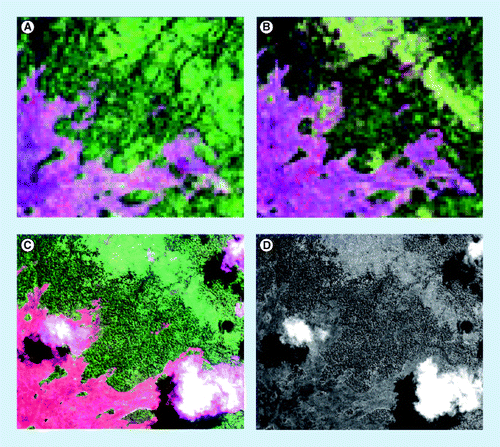
Role of tropical deforestation in global carbon emissions
Tropical deforestation is estimated to total approximately 13 million ha per year in the period 2000–2010 compared with 16 million ha per year in the period 1990–2000 Citation[1]. The net loss of forest area globally has been significantly reduced from -8.3 million ha per year between 1990 and 2000 to -5.2 million ha between 2000 and 2010. This reduction of net loss is largely due to an increase in afforestation, natural forest regrowth, reforestation and forest plantations. However, the global rate of gross deforestation, mainly the conversion of tropical forests to agricultural land, is still alarmingly high and has significant impacts on global climatic change and biodiversity issues.
Tropical deforestation, resulting from different causes Citation[2–4], leads to emissions of CO2 and, if the biomass is burned during the clearing process, additional non-CO2 gases are emitted Citation[5,6]. Deforestation causes a large loss of carbon stock per unit area relative to other practices in forests (e.g., logging) that may only result in forest degradation and lower loss of carbon stock Citation[7]. Deforestation in the tropics is thought to be a major contributor to GHG emissions. Emissions from tropical deforestation, forest and peat degradation are currently estimated to be 15% of the world’s anthropogenic GHG emissions, mainly through CO2 emissions (in the range of 8–20%) Citation[8]. For the period 1997–2006, global net carbon emissions resulting from land-use changes, predominantly deforestation in the tropics and peat degradation, have been estimated at 1.5 GtC yr–1 (in the range of 1.1–1.9 GtC yr–1); 1.22 GtC yr–1 from deforestation and forest degradation and 0.3 GtC yr–1 from peat degradation, when the total global anthropogenic emissions (i.e., fossil fuel and cement emissions + flux resulting from land use changes) were 10.1 GtC yr–1 in 2008.
Old-growth tropical forests store carbon at an estimated rate of 0.5 tC ha–1 yr–1 (confidence interval [CI]: 0.29–0.66) Citation[9], which leads to a carbon sink of -1.3 GtC yr–1 (CI: 0.8–1.6) across all tropical forests during recent decades. Such estimates are consistent with a study combining analysis of atmospheric CO2 concentration data and atmospheric transport models, which report a net tropical emission of +0.1 GtC yr–1Citation[10], which then can be related as the sum of emissions from land-use changes, predominantly in the tropics (+1.5 GtC yr–1) and uptake from remaining tropical forests (-1.3 GtC yr–1). In complement to carbon emissions, tropical deforestation also weakens this natural sink capacity.
However, uncertainties in the land-use change flux of the global carbon budget are high. As reported in the Intergovernmental Panel on Climate Change (IPCC) 4th Assessment report Citation[11]: “The land use carbon source has the largest uncertainties in the global carbon budget.” To obtain accurate estimates of emissions from land-use changes in the tropics, several components must be estimated accurately, in particular: area of forest cover changes; initial carbon stocks (above- and below-ground biomass and soil organic matter) in forests before deforestation or forest degradation; and processes of changes in the carbon stocks within forests caused by deforestation, gains from growth and losses from degradation and other processes Citation[12–14]. Accurate figures of forest cover changes are needed to reconcile estimates of land-use change emissions from different sources, which are presently calculated from different databases: Houghton Citation[10] used deforestation figures from the Food and Agriculture Organization (FAO) Global Forest Resources Assessment 2000 Citation[15], while Achard et al.Citation[16] and DeFries Citation[17] used their own remote sensing-derived deforestation estimates.
Reference data on biomass and accepted procedures to estimate the fluxes are equally essential Citation[18], although it is not the focus of this article. The full potential of satellite remote sensing imagery for biomass monitoring is yet to be realized Citation[19]. A new challenge lies in estimating change in carbon stocks directly from remote sensing and then linking the information on forest carbon stocks and satellite-derived maps of changes in forest cover to the estimation of emissions Citation[14]. Data on forest carbon stocks at both continental and global scales is improving Citation[20–22]. It has been suggested that lack of funding limits efforts to reduce uncertainties of estimates of biomass Citation[23].
Reducing emissions from tropical deforestation: a recent mechanism of the United Nations Framework Convention on Climate Change
Although uncertainties in emissions from tropical deforestation are high, the contribution of such emissions to the global carbon budget is recognized by the international climate change community as being very significant. As a consequence, official international discussions were initiated at the United Nations Framework Convention on Climate Change (UNFCCC) 11th Conference of Parties (COP) on the issue of reducing emissions from deforestation and degradation (REDD) in developing countries. At COP-11, the UNFCCC launched a process for investigating the technical issues surrounding the feasibility of reducing such emissions.
At the UNFCCC COP-15 held in Copenhagen in December 2009, the need to provide incentives for REDD was, for the first time, mentioned in the final declaration of the Heads of State and governments, referred as Decision 2 of the COP-15 (2/CP.15). This decision follows on from Decision 2 of the COP-13 (2/CP.13) on “approaches to stimulate action” for “reducing emissions from deforestation in developing countries”. The Copenhagen Accord recognizes “the crucial role of reducing emission from deforestation and forest degradation and the need to enhance removals of GHG emission by forests” and encourages the “immediate establishment of a mechanism including REDD-plus to enable the mobilization of financial resources from developed countries”.
Beyond the recognition of the crucial role of tropical forest and the agreement on the need to provide incentives to such actions of Decision 2/CP.15, Decision 4/CP.15 provides methodological guidance for activities relating to REDD and the role of conservation, sustainable management of forests and enhancement of forest carbon stocks in developing countries, the so-called ‘REDD-plus’ extended activities Citation[24]. Decision 2/CP.13 invited Parties “to further strengthen and support ongoing efforts to reduce emissions from deforestation and forest degradation on a voluntary basis” and has been completed in Decision 4/CP.15 by requesting “developing country Parties, […] (a) to identify drivers of deforestation and forest degradation resulting in emissions and also the means to address these; (b) to identify activities within the country that result in reduced emissions and increased removals, and stabilization of forest carbon stocks […]”. Decision 4/CP.15 further recognizes that forest reference emission and forest reference levels should be established transparently, taking into account historic data and national circumstances. The core of Decision 4/CP.15 on methodological guidance deals with the establishment of “robust and transparent national forest monitoring systems and, if appropriate, sub-national systems” with the following characteristics:
▪ Combination of remote sensing and ground-based forest carbon inventory approaches | |||||
▪ Transparent, consistent and, as far as possible, accurate estimates taking into account national capabilities and capacities; | |||||
▪ Results available and suitable for review as agreed by the COP. |
Decision 4/CP.15 also invites “Parties in a position to do so and relevant international organizations” to enhance the capacities of developing countries to collect and access, analyze and interpret data, in order to develop estimates and to enhance coordination of the activities of the different stakeholders. Parties are requested “to use the most recent IPCC guidance and guidelines, as adopted or encouraged by the COPs, as appropriate, as a basis for estimating anthropogenic forest-related GHG emissions by sources and removals by sinks, forest carbon stocks and forest area changes”. The definitions of forest and deforestation agreed under the UNFCCC will serve as a reference for the future mechanism Citation[25]. There is no official definition of forest degradation but, in a REDD-plus context, it corresponds to a loss of carbon stocks in forests remaining forests due to human activities.
A monitoring activity in support of a potential REDD-plus mechanism necessitates a capacity to estimate changes throughout all forests within a country’s boundaries. Nationwide monitoring is needed, in particular to avoid displacement of deforestation within a country where reduced deforestation could occur in one area of the country but increase in another area Citation[26]. Appropriate methods need be used by developing countries to demonstrate that they are fulfilling requirements in the framework of the REDD-plus mechanism. Monitoring systems at national levels in tropical countries can benefit from pan-tropical and regional observations and monitoring systems, by allowing countries to access standard methods across regions and to ensure consistency between different national monitoring systems. The monitoring of forest resources at a pan-tropical scale also contributes to the UNFCCC REDD-plus activities by monitoring potential international leakage effects (displacement of deforestation between countries) and by providing global historical references Citation[27].
Scope of the review: use of remote sensing technology to estimate deforestation at pan-tropical to regional scales
Estimating deforestation would be a major challenge without the use of satellite imagery, in particular for large and remote regions Citation[28]. Satellite remote sensing combined with ground measurements plays a key role in determining loss of forest cover. Technical capabilities and statistical tools have advanced since the early 1990s Citation[29] and operational forest monitoring systems at the national level are now a feasible goal for most developing countries in the tropics Citation[30,31]. However, reducing uncertainties in the land-use change flux of the global carbon budget requires the capability to estimate changes throughout all forests of the tropical belt.
Improved pan-tropical observations can support the activities that are starting in the framework of the REDD-plus readiness mechanism. While primary reporting would occur at national or sub-national levels, pan-tropical monitoring could contribute through identifying critical areas of change, helping to establish areas within countries that require detailed monitoring, and ensuring consistency among national efforts. The main requirements of pan-tropical monitoring systems are that they measure changes throughout all forested areas, use consistent methodologies at repeated intervals to obtain accurate results, and verify results with fine resolution observations Citation[32].
This article is intended to complement the existing IPCC guidance and guidelines for land use, land-use change and forestry (LULUCF) and the Global Observation of Forest and Land Cover Dynamics (GOFC-GOLD) REDD Sourcebook, as written from a different perspective. The Good Practice Guidance for LULUCF Citation[33] has been approved by developed countries (‘Annex-I’ countries) for Kyoto Protocol reporting and has been recommended to be used by developing countries (‘non Annex-I’ countries) for REDD-plus. Based on UNFCCC-approved methodologies, the GOFC-GOLD REDD sourcebook aims to provide additional explanation and methodologies to support readiness mechanisms for building national REDD-plus monitoring systems Citation[32]. This article is not intended to provide technical guidance or to be a sourcebook of methods, but is aimed at providing a scientific review on a related topic (i.e., on how to estimate tropical deforestation from Earth observation data).
This article develops from a previous review Citation[31] and considers a range of issues for monitoring forest cover at the pan-tropical level. Many methods of satellite imagery analysis can produce adequate results from pan-tropical to national scales. Four general remote sensing-based approaches are currently used for capturing deforestation trends at the pan-tropical level:
▪ Global land cover mapping and identification of areas of rapid forest cover changes from coarse spatial resolution imagery using optical sensors; | |||||
▪ Nested approach with coarse and moderate spatial resolution imagery from optical sensors (i.e., analysis of wall-to-wall coverage from coarse resolution data to identify locations of large deforestation fronts for further analysis with a sample of moderate spatial resolution data); | |||||
▪ Statistical sampling designed to estimate deforestation from moderate spatial resolution imagery from optical sensors; | |||||
▪ Analysis of wall-to-wall coverage from moderate spatial resolution imagery from optical or radar sensors. |
The article describes the latest developments and projects related to these four general remote-sensing approaches applied at the pan-tropical scale or over large-sized regions or countries. The article will not dicuss the technical details of the range of possible change detection methods for forest ecosystem monitoring, which are available from other sources Citation[34]. From a methodological point of view, we only mention that one of the key issues is that satellite data needs to be interpreted (digitally or visually) for forest cover change; in other words, focusing on the interdependent interpretation of multitemporal imagery to detect and characterize changes. The use of moderate resolution satellite imagery for historical assessment of deforestation has been boosted by the recent free availability of the Landsat Global Land Survey Database, which is described hereafter before the three general remote sensing-based approaches using such imagery. Finally the article will discuss the need for accuracy assessment with ground data or fine resolution imagery and the perspectives on future satellite remote sensing imagery and technology.
Global land cover mapping and identification of hot spots of deforestation from coarse resolution satellite imagery
Global land cover maps provide a static depiction of land cover and cannot be used to map changes in forest area, owing to uncertainty levels, which are higher than levels of area changes Citation[35]. However, land cover maps can serve as a stratification against which future change can be assessed and can help to establish forest areas that need to be monitored for change.
Multiple methods are appropriate and reliable for forest cover mapping at pan-tropical scales using remote sensing data acquired by space-based platforms supported by ground-based observations. Coarse spatial resolution (from 250 m × 250 m to 1 km × 1 km) satellite imagery is currently used for global land or forest cover mapping.
In the 1990s, global or pan-continental maps were produced at approximately 1 km × 1 km resolution from a single data source: the advanced very high resolution radiometer (AVHRR) sensor onboard the satellites of the United States National Oceanographic and Atmospheric Administration (NOAA) . In the early 2000s, new global land cover datasets were produced at similar resolution – 1 km × 1 km – from advanced Earth observation sensors (VEGETATION [sensor] on board SPOT-4 and SPOT-5, and the moderate resolution imaging spectro-radiometer [MODIS], on board the Terra and Aqua platforms). These products, GLC-2000 Citation[36] and MODIS Global Land Cover product Citation[37], allowed for a spatial and thematic refinement of the previous global maps owing to the greater stability of the platforms and the spectral characteristics of the sensors. An international initiative was also carried out to harmonize existing and future land cover datasets at the 1 km resolution to support operational Earth observation of the Earth’s land surface Citation[38].
More recently, new global land cover datasets at finer spatial resolution (from 250 m × 250 m to 500 m × 500 m) were generated from TERRA-MODIS or ENVISAT-MERIS sensors. The two key products at this scale are the Vegetation Continuous Fields (VCF) product and the GlobCover map. The MODIS-derived VCF product depicting sub-pixel vegetation cover traits at a spatial resolution of 500 m × 500 m Citation[39]. The systematic geometric and radiometric processing of MODIS data has enabled the implementation of operational land cover characterization algorithms. Currently, 10 years (2000–2010) of global VCF tree cover data are now available to researchers and are being incorporated into various forest cover and change analyses. A more recent (2005) 500 m × 500 m resolution version of the MODIS Global Land Cover product has also been generated with substantial differences arising from increased spatial resolution and changes in the input data and classification algorithm Citation[40]. The ‘GlobCover’ initiative produced a global land cover map using the 300 m resolution mode from the MERIS sensor onboard the ENVISAT satellite Citation[41]. Data have been acquired from 1 December 2004 to 30 June 2006. A global land cover map was generated from these data from automatic classification tools using equal-reasoning areas. This product has complemented previous global products and other existing comparable continental products Citation[42–44], with improvement in terms of spatial resolution .These global products can also be used as complementary forest maps when they do not already exist at the national level Citation[45], in particular for ecosystem stratification to help in the estimation of forest biomass through spatial extrapolation methods Citation[22]. Static forest cover maps used in concert with change detection studies provide the basis for establishing rates of change, and are particularly useful as a stratification tool in developing sampling approaches for forest change estimation. Since up to date information on global land cover can play an important role in land cover monitoring, reporting the accuracy of these products is essential through the use of agreed protocols Citation[46] and new potentially valuable avenues, such as web-based land cover and land-use validation tools Citation[35]. The overall accuracies of the GLC-2000, MODIS Global Land Cover (through cross-validation analysis) and GlobCover products have been reported at 68, 75 and 73% respectively Citation[36,40,41].
The principal monitoring requirement to support REDD-plus activities falls at the national level. Analyses that span the tropics, using coarser resolution data than would be used at the national level, can supplement these efforts by providing consistency and ensuring that major areas of deforestation are detected. A first global map of the main deforestation fronts in the 1980s and 1990s was produced in the early 2000s Citation[47]. This map combines knowledge of deforestation fronts in the humid tropics, a time-series analysis of tree cover based on NOAA AVHRR 8 km resolution data and, for the Amazon basin, deforestation maps derived from time-series of Landsat thermic mapper (TM) data. In this exercise, the use of expert knowledge ensured that areas of major change not detected with the satellite-based approaches were not overlooked. More recently, a more detailed identification of deforestation areas at the pan-tropical level has been produced for the period 2000–2005 from MODIS imagery Citation[48].
The Brazilian PRODES monitoring system for the Brazilian Amazon uses also a hotspot approach to identify ‘critical areas’ based on the previous year’s monitoring Citation[49]. These critical areas are priorities for analysis in the following year. Other databases such as transportation networks, population changes in rural areas, and locations of government resettlement programs can be used to help identify areas where more detailed analysis needs to be performed. Since May 2005, the Brazilian government has also had the Detecção de Desmatamento em Tempo Real (DETER) system in operation to serve as an alert in almost real-time (every 15 days) for deforestation events larger than 25 ha. The system uses MODIS data (at 250 m × 250 m resolution) and wide field imager data on board CBERS-2 satellite (260 m × 260 m resolution) and a combination of linear mixture modeling and visual analysis. The results are publicly available Citation[101].
Use of moderate spatial resolution imagery for historical references: the Landsat Global Land Survey Database
The use of moderate resolution satellite imagery for historical assessment of deforestation rates has been boosted by the recent free availability of the Landsat Global Land Survey Database through the US Geological Survey (USGS).
By the late 1960s, NASA had designed a satellite mid-resolution sensor that was able to collect land information at a landscape scale. Earth Resources Technology Satellite (ERTS-1) was launched on 23 July 1972. This satellite, renamed ‘Landsat’, was the first in a series (seven to date) of Earth-observing satellites that have permitted continuous coverage since 1972. Subsequent satellites have been launched every 2–3 years. Landsats 4, 5 and 7 cover the same ground track again every 16 days.
Nearly complete pan-tropical coverage from the Landsat satellites is now available at no cost from the Earth Resources Observation Systems (EROS) Data Center (EDC) of the USGS Citation[102]. A recent product, called the Global Land Survey (GLS), was derived by reprocessing GeoCover data, a selection of good quality, orthorectified and geodetically accurate global land dataset of Landsat multispectral scanner (68 m × 83 m resolution), Landsat TM (30 m × 30 m) and Landsat enhanced thermic mapper plus (ETM+; 30 m × 30 m) satellite images with a global coverage, which was created from the following epochs: mid-1970s at 60 m × 60 m resolution; and circa 1990, circa 2000 and mid-2000s at 28.5 m × 28.5 m resolution by NASA Citation[50]. USGS has undertaken the effort of improving the geolocation for the best quality scenes of their extensive Landsat data archive. For the terrain correction of the GLS process, digital elevation data was available at a resolution of 90 m × 90 m from the Shuttle Radar Topography Mission (SRTM). GLS data uses the collection of geodetic control points, provided by American National Imagery and Mapping Agency with approximately 500 Landsat ETM+ definitive ephemeris scenes used in the process Citation[51].
Global Land Survey datasets were produced for the mid-1970s epoch (GLS-1975), the 1990 epoch (GLS-1990), the 2000 epoch (GLS-2000), and the 2005 epoch (GLS-2005). In this context, the GLS time indications stand, approximately, for the period of 1986–1993 for GLS-1990; 1999–2001 for GLS-2000; and 2004–2007 for GLS-2005. The selection criteria for the GLS data were based on parameters such as the least cloud cover (the most important factor), an approximate optimal seasonal date for the area, the year of acquisition as close as possible to the target years 1990, 2000 and 2005, the possible existence of ‘single data runs’, and the absence of image artifacts Citation[50].
The creation of the GLS-2005 dataset proved to be more difficult owing to the failure of the Landsat-7 ETM+ Scan Line Corrector (SLC) in May 2003, which creates along-scan data gaps and renders 23% of every acquisition unsuitable for interpretation; and the inability to secure a global coverage with Landsat-5 TM. To account for the Landsat-7 ETM+ problem, image mosaics in which data gaps due to SLC failure are filled were produced where possible. However, Landsat-7 gap-filling or scene replacement with Landsat-5 was only possible for areas without persistent cloud cover, leaving many areas over the tropics without good GLS imagery Citation[52].
These GLS datasets play a key role in establishing historical deforestation rates although in some parts of the tropics (e.g., Western Colombia, Central Africa and Borneo) persistent cloud cover is a major challenge to using these data. For these regions the GLS datasets can be complemented by remote sensing data from other satellite sensors with similar characteristics, in particular sensors in the optical domain with moderate spatial resolution (see also Table 2 in Citation[53]).
Nested approach with coarse & moderate resolution data from optical sensors
Analysis of coarse resolution data can identify locations of rapid and large deforestation fronts, although such data are unsuitable on their own for determining rates of deforestation based on changes in forest area Citation[54,55]. A nested approach in which wall-to-wall coarse resolution data are analyzed to identify locations requiring further analysis with more costly moderate resolution data (from 20 m × 20 m to 50 m × 50 m) can reduce the need to analyze the entire forested area within a country. The appropriateness of this approach depends on whether computational resources are available for analysis of coarse resolution data, as opposed to visual interpretation of moderate resolution data. Coarse resolution data have been available from the MODIS sensor for no cost since 2000. In some cases, it is possible to identify deforestation directly with coarse resolution data. Clearings for large-scale mechanized agriculture are detectable with coarse resolution data based on digital analysis Citation[54]. Small agricultural clearings or clearings for settlements require higher resolution data (<50 m × 50 m) to accurately detect clearings of 0.5–1 ha. Smaller clearings and more heterogeneous landscapes require data with finer spatial resolution (∼10 m × 10 m), more complex computer algorithms capable of detecting less pronounced differences in spectral reflectance and greater involvement of an interpreter for visual analysis and verification.
A novel nested approach that takes advantage of both coarse spatial resolution satellite data and the large Landsat data archive to estimate humid tropical forest cover change is demonstrated by South Dakota State University (SDSU) location within the NASA Land Cover and Land Use Change program Citation[48]. This method employs a fusion of coarse spatial resolution MODIS and moderate spatial resolution Landsat data to estimate and map forest cover change pan-tropically. Annual, 4- and 5-year MODIS forest cover change indicator maps are aggregated into 18.5 km square blocks and used to stratify the humid tropical forest biome into regions, or strata, of low, moderate and high amounts of forest cover clearing. Within each stratum, a random sample of moderate resolution Landsat image pairs of the same block size are carefully analyzed for forest cover clearing between 2000 and 2005 using a combination of automated algorithms and expert visual interpretation. A regression estimator, unique to each stratum, is created that relates the Landsat and MODIS-derived forest cover clearing. Estimates of forest clearing are generated from the relatively fine-scale resolution Landsat and, through the use of the regression models, can be extended to the continuous MODIS block data, thereby creating a calibrated, spatially explicit depiction of forest cover clearing per stratum.
Data fusion capitalizes on each data source’s best attributes and can overcome many of the limitations of single input approaches. Coarse spatial resolution sensor data, such as MODIS, are imaged daily over the globe, providing the best possibility for cloud-free observations from a polar-orbiting platform. However, coarse spatial resolution data do not directly allow for accurately estimating forest area changes given that most change occurs at sub-pixel scales. Conversely, moderate spatial resolution Landsat data, allows for more accurate measures of change areas, especially where changes are of a finer-scale. By using MODIS data as a stratification tool and Landsat data to estimate the forest area cleared, an integrated method is attained. The targeted sampling of change reduces the overall resources typically required in assessing biome-scale change and overcomes the problem encountered in previous studies of imprecise estimates of forest clearing attributable to the rarity of such clearing Citation[56,57].
Statistical sampling designed to estimate deforestation from moderate spatial resolution optical sensors
An analysis that covers the full spatial extent of the forested areas with moderate spatial resolution imagery, termed ‘wall-to-wall’ coverage, is ideal, but may not be practical over very large, heterogeneous areas, and places commensurate constraints on resources for analysis. For digital analysis with moderate-resolution satellite images at pan-tropical or continental levels, several approaches have been successfully applied by sampling within the total forest area so as to reduce costs and time for analysis.
A sampling procedure that adequately represents deforestation events can capture deforestation trends. Since deforestation events are not randomly distributed in space, particular attention is needed to ensure that the statistical design is adequately sampled within areas of potential deforestation (e.g., in proximity to roads or other access networks); for example, through a high density systematic sampling when resources are available Citation[58,59].
For its present global assessment, the Forest Resources Assessment 2010 programme (FRA 2010), the FAO is continuing to develop its monitoring of forest cover changes at global to continental scales to complement national reporting. Technological improvements and better access to remote sensing data make it possible to expand the scope of the survey compared with both FRA 1990 and FRA 2000 Citation[60]. The remote sensing survey (RSS) of FRA 2010 has been extended to all lands (not just the pan-tropical zone). The survey aims to estimate forest change for the two periods 1990–2000 and 2000–2005 based on a sample of moderate resolution satellite imagery. Estimates of forest areas and change rates will be statistically valid (i.e., accurate and precise) at global, continental and sub-regional levels. The survey will be based on a much higher number of smaller sample units than the previous FRA exercises, with a systematic grid – sample units are located at each intersection of the 1° × 1° lines of latitude and longitude that falls over land. Each sample unit has a core size of 10 km × 10 km with an external 5-km buffer for forest cover contextual information (i.e., the full size of sample units is 20 km × 20 km for land cover information). These dimensions were chosen to allow spatially explicit monitoring at a scale relevant to land management. This sampling scheme leads to approximately 13,500 sample units for the terrestrial part of the globe or approximately 9000 sample units when excluding desert areas, and represents approximately 1% of the land surface (0.8% along the equator) with the geographical grid . This approach is expected to deliver regionally accurate estimates of forest cover change, as well as national estimates for those countries where sampling intensity is sufficient. Testing of the systematic sampling design within the Brazilian Amazon resulted in a low standard error of less than 5% of forest cover change rate Citation[61,62].
Time-series of moderate resolution remote-sensing data are attached to each sampling location through a quality-controlled, standardized and decentralized process. For the FAO’s FRA2010 RSS exercise, SDSU produced a global database of multitemporal 20 km × 20 km sample tiles extracted from the USGS GLS archives Citation[63,103]. For the proportion of the sample tiles that are not available from the GLS database or have persistent cloud contamination, other Landsat imagery or alternative remote sensing data have been used [Beuchle R, Eva HD, Stibig H-J, Unpublished data]. This global systematic sampling scheme has been developed jointly by FAO and the Joint Research Center (JRC) to estimate rates of deforestation at global or continental levels at intervals of 5–10 years Citation[58, 64].
Being a main partner of FAO’s FRA 2010 RSS Citation[65], JRC scientists collaborate with remote sensing and forestry experts from tropical countries in order to estimate forest cover changes at the pan-tropical level. In this context, the JRC organized several workshops to train forestry experts on the remote sensing methods developed at JRC for the validation of the initial tree cover maps Citation[66]. JRC and FAO scientists collaborated with more than 100 remote sensing and forestry experts from tropical countries, including largely forested countries such as Brazil, India, Indonesia and the Democratic Republic of Congo. The land-cover legend includes a few land-cover classes: tree-cover, mosaics of trees and other land-cover, shrub-cover, other land-cover, water, clouds and no data. A minimum mapping unit (MMU) of 5 ha (or 50 pixels at 30 m × 30 m resolution) is considered for the interpretation of the satellite imagery to identify the forest cover changes. A finer “detection unit level” at approximately 1 ha is used in a first automated segmentation and labeling step before aggregation to 5 ha objects for the interpretation phase. These criteria are based on initial tests performed on datasets for Papua New Guinea.
Forest cover change estimation at a regional scale
The systematic sampling approach has been applied earlier in Central Africa to derive area estimates of forest cover change for the period 1990–2000 using Landsat imagery Citation[67]. The approach was operationally applied to the entire Congo River basin to estimate deforestation regionally. The survey was composed of 10 km × 10 km sampling sites systematically distributed every 0.5° × 0.5° longitude over the whole forest domain of Central Africa, corresponding to a sampling rate of 3.3%. For each of the 571 sites, subsets were extracted from both Landsat TM and ETM+ imagery acquired in 1990 and 2000, respectively. The satellite imagery was analyzed with object-based (multidate segmentation) unsupervised classification techniques Citation[68]. Approximately 60% of the 390 cloud-free images do not show any forest cover change. For the other 165 sites, a change matrix is derived for every sample site describing four land-cover change processes; for example, deforestation, reforestation, forest degradation and forest recovery. The exercise illustrates that the statistical precision depends on the sampling intensity. For a region such as Central Africa (with 180 million ha), using 400 samples, the exercise estimates the deforestation rate at 2.1% over a 10-year period with a statistical CI of ± 0.5%. The overall accuracy of the land cover classifications used for deriving forest change dynamics was assessed by an independent interpreter to be 91%.
The global systematic sampling scheme above can be intensified to produce results at the national level. Deforestation estimates derived from two levels of sampling intensities have been compared with estimates derived from the official inventories for the Brazilian Amazon and for French Guiana Citation[62]. By extracting nine sample data sets from the official wall-to-wall deforestation map derived from satellite interpretations produced for the Brazilian Amazon for the year-long period from 2002 to 2003 Citation[49], the global systematic sampling scheme estimate gives 2.8 million ha of deforestation with a standard error of 0.1 million ha. This compares with the full population estimate from the wall-to-wall interpretations of 2.7 million ha deforested. The relative difference between the mean estimate from the sampling approach and the full population estimate is 3.1% and the standard error represents 4.0% of the full population estimate. To intensify this global sampling to French Guiana, Landsat-5 TM data were used for the historical reference period, 1990, and a coverage of SPOT-high resolution visible sensor imagery at 20 m × 20 m resolution was used for 2006. The estimates of deforestation between 1990 and 2006 from the intensified global sampling scheme over French Guiana are compared with those produced by the national authority to report on deforestation rates under the Kyoto protocol rules for its overseas department Citation[69]. The latter estimates come from a sampling scheme of nearly 17,000 plots derived from the traditional forest inventory methods carried out by Inventaire Forestier National (IFN) and analyzed from same spatial imagery acquired between 1990 and 2006. The intensified global sampling scheme leads to an estimate with a relative difference from the IFN of 5.4%. These results, as well as other studies Citation[70], demonstrate that the intensification of the global sampling scheme can provide forest area change estimates close to those achieved by official forest inventories with precisions of less than 10%, although only estimated errors from sampling have been considered and not errors from the use of surrogate data.
Analysis of wall-to-wall coverage from moderate spatial resolution imagery from optical or radar sensors
A few very large countries, in particular the well-known cases of Brazil Citation[49] and India Citation[71], have already demonstrated for many years that operational wall-to-wall systems over very large regions, or countries, can be established based on moderate resolution satellite imagery.
Brazil has been measuring deforestation rates in the Brazilian Amazonia since the 1980s. The Brazilian National Space Agency (INPE) produces annual estimates of deforestation in the ‘legal Amazon’ from a comprehensive annual national monitoring program called PRODES. The Brazilian Amazon covers an area of approximately 5 million km2, including approximately 4 million km2 that is covered by forests. The Government of Brazil decided to generate periodic estimates of the extent and rate of gross deforestation in the Amazon, “a task which could never be conducted without the use of space technology”. The first complete assessment by INPE was undertaken in 1978. Annual assessments have been conducted by INPE since 1988. For each assessment, 229 Landsat satellite images are acquired around August and analyzed. Spatially-explicit results of the analysis of the satellite imagery are published every year Citation[104]. The PRODES project has been producing the annual rate of gross deforestation since 1988 using a minimum mapping (change detection) unit of 6.25 ha with the release of estimates foreseen around the end of each year. PRODES uses imagery from Landsat TM and ETM+ sensors, complemented by imagery from sensors of Disaster Monitoring Constellation (DMC) and CBERS satellites with similar spatial resolutions .
The use of satellite remote sensing technology to assess the forest cover of the whole of India began in the early 1980s. The first forest map of the country was produced in 1984 at 1:1 million scale by visual interpretation of Landsat data. The Forest Survey of India (FSI) has since been assessing the forest cover of the country on a 2-year cycle. Over the years, there have been improvements both in the remote sensing data and the interpretation techniques. The latest assessment (11th biennial cycle) has been completed using satellite data from end of 2006 from the Indian satellite IRS P6 (Sensor LISS III at 23.5 m × 23.5 m resolution). Satellite imagery was interpreted digitally with a minimum mapping unit of 1 ha. The entire assessment from the procurement of satellite data to the reporting, including image rectification, interpretation, ground truthing and validation of the changes by the State/Province Forest Department, takes almost 2 years. The interpretation involves a hybrid approach combining unsupervised classification in raster format and on-screen visual interpretation of classes. Accuracy assessment is an independent exercise using randomly selected sample points verified on the ground (field inventory data) or with satellite data at 5.8 m × 5.8 m resolution and compared with interpretation results. In the last assessment, 4291 validation points randomly led to an overall accuracy level of the assessment of 92% Citation[71].
Data fusion approaches are also being employed to produce spatially exhaustive, or wall-to-wall, estimates and maps of forest cover clearing within the humid tropics. In the Congo Basin, MODIS and Landsat data are used to create best-pixel, multispectral composites, forest area, and forest cover change maps of the entire basin at the Landsat scale for the years 1990, 2000 and 2005 Citation[72], [Lindquist EJ, Hansen MC, Adusei B et al., Unpublished data]. MODIS-derived forest cover from the VCF product helps radiometrically normalize Landsat data and provide forest cover training inputs into supervised classification algorithms. Compositing viable, temporally proximate, normalized pixels from multiple Landsat acquisitions overcomes limitations of wall-to-wall mapping caused by poor quality data, owing to cloud or missing data, due to the Scan Line Corrector failure of the Landsat 7 sensor Citation[73]. This approach, which makes use of a large proportion of the Landsat data archive collected over the Congo Basin, is made possible by the decision of the USGS to make all Landsat data freely available Citation[105]. The method is currently being applied pan-tropically.
Another method, the CLASlite satellite mapping system, has been applied for the regional mapping of vegetation type and condition (e.g., forest cover, deforestation, degradation and regrowth) using Landsat imagery in annual time steps in the Amazon basin Citation[74].
Optical mid-resolution data have been the primary tool for deforestation monitoring. Other types of sensors (e.g., Synthetic Aperture Radar [SAR] or Radar), are potentially useful and appropriate (a full list of current and future SAR sensors is available in the GOFC-GOLD REDD Sourcebook Citation[32]). Radar remote sensors are active systems: it transmits short pulses of energy toward the surface below, which interact with surface features such as forest vegetation. Radar sensors operate in the microwave region (∼3–70 cm), which generally penetrate through atmospheric particulates (e.g., haze, smoke and clouds), thus alleviating limitations of optical data in persistently cloudy parts of the tropics. Microwaves interact with forest canopies, with the amount of backscattered energy dependant in part on the 3D structure and moisture content of the vegetation and underlying soils. SAR backscatter is correlated to forest biomass for low values of biomass with saturation for high values Citation[75]. Longer wavelengths (e.g., P-/L-band) penetrate deeper into forest canopies than shorter wavelengths (e.g., C-/X-band). As a consequence, the saturation in the relationship between backscattered signal and biomass occurs at higher values for longer wavelengths, (i.e., ∼30 T/ha for C-band, 50 T/ha for L-band and 150–200 T/ha for P-band).
In addition to wavelength, the polarization of the emitted and received microwave energy (horizontal transmit and horizontal receive [HH], vertical transmit and vertical receive [VV] or horizontal transmit and vertical receive [HV]) and the incidence angle of the emitted wave can also be considered for the characterization of the forest structure. While the first space-borne SAR sensors operated with only one polarization (HH or VV) and at moderate resolutions (from 30 m × 30 m to 100 m × 100 m), a number of recent SAR sensors now have full-polarimetric and fine spatial resolution capabilities (e.g., TerraSAR-X at 5 m × 5 m resolution). However, these more advanced features imply a reduction of the sensor swath width; for example, 15 km swath width for the experimental polarimetric mode of TerraSAR-X, and, consequently, a reduction of the coverage. Such limitations do not allow for obtaining wall-to-wall coverage for large areas in a reasonable time (i.e., within a few years) but can be considered for sample coverage where more details of the vegetation cover are available.
Imagery from radar sensors have been demonstrated to be usable for tropical forest cover monitoring at local scales Citation[76,77], although no operational tropical forest monitoring has yet been developed for large areas (region or continent). This is mainly owing to three reasons: lack of technical expertise and resources to process and analyze radar imagery; lack of consistent regional and pan-tropical coverage of SAR data until recently; and technical limitations of SAR data for the assessment of deforestation. Indeed, in some cases, deforested areas are confused with forest areas in SAR imagery, in particular when the deforestation event is very recent with presence of tree debris on the ground, or when dense secondary vegetation has already regrown. This highlights the need for time-series of satellite radar imagery with high temporal frequency (at least once a year) to increase the efficiency of detection of deforestation events. Strategies are therefore needed to ensure regular and systematic acquisition of SAR data coverages over large regions. So far, only the Japanese Aerospace Exploration Agency (JAXA) has designed such a dedicated observation strategy for the Phased Array L-band Synthetic Aperture Radar (PALSAR) sensor on board the Advanced Land Observing Satellite (ALOS), launched in January 2006. The JAXA acquisition strategy for this sensor consists of a systematic imagery acquisition over all of Earth’s land masses in a wall-to-wall manner at 10 m × 10 m to 100 m × 100 m resolution at least twice per year. Owing to this observation strategy, consistent datasets are being used for the early detection of deforestation Citation[78] or for regional tropical forest mapping Citation[79,80]. Wall-to-wall mosaics of pan-tropical regions are being produced in the frame of the ALOS Kyoto and Carbon Initiative [De Grandi GF, Bouvet A, Lucas R et al., Unpublished data], and will be compared with the tropical mosaics generated by the SAR sensor of the Japanese Earth Resources Satellite (JERS-1) in the mid-1990s Citation[81,82]. The ALOS mosaics are also expected to complement or replace optical-based remote sensing surveys for regions where little or no cloud-free data is available, as illustrated in .
Accuracy assessment: use of reference data or fine spatial resolution imagery
Whether through wall-to-wall or sample-based approaches, the key requirements of ensuring consistency of results across continents or countries lies in verification that the methods are reproducible, provide consistent results when applied at different times and meet standards for assessment of accuracy Citation[83,84]. Ground reference data (or information derived from very fine spatial resolution imagery that can be considered to be surrogate to ground reference data) are generally recommended as the most appropriate data to assess the accuracy of land-cover change estimation, although their imperfections (e.g., related to methods used to assess tree crown over from the ground) may introduce biases into estimators of change Citation[85]. Reporting the overall accuracy (i.e., not only the statistical accuracy, but also the interpretation accuracy) and verification of results are essential components of a monitoring system. Interpretation accuracies of 80–95% are achievable for monitoring changes in forest cover with mid-resolution imagery when using only two classes: forest and nonforest Citation[68]. Interpretation accuracies can be assessed through in situ observations or analysis of very high resolution aircraft or satellite data. While it is difficult to verify change from one time to another on the ground unless the same location is visited at two different time periods, a time series of fine (to very fine) resolution data can be used to assess accuracy of identifying deforestation.
A new challenge is to provide a consistent coverage of fine resolution satellite imagery for global forest cover monitoring; in other words, at least a statistical sample or, more challenging, a wall-to-wall coverage. The finer resolution (from 1 m × 1 m up to 10 m × 10 m) will allow more precise forest area estimates and canopy cover assessment to be derived, and therefore more reliable statistical information on forest area changes, in particular for estimating forest degradation and forest regrowth, and their related carbon emissions or removals.
To continue to complement the JRC’s TREES forest cover assessment covering the period 1990–2005, the European Space Agency (ESA) launched a joint action with the JRC to produce better estimates of forests cover changes in the tropical forests of Latin America and South East Asia up to the year 2010 from fine spatial resolution satellite imagery. Assessments for year 2010 are essential for building updated deforestation reference rates at global to regional levels. ESA will create a harmonized remote sensing ortho-rectified imagery geo-database that will cover sample units of 20 km × 20 km size located at the degree confluence for 2010. This imagery geo-database will be based on satellite data acquisitions performed during the period mid 2009 to the beginning of 2011 from a series of satellite sensors with fine spatial resolution: AVNIR-2 sensor (10 m × 10 m resolution) onboard the ALOS platform complemented by sensor onboard DEIMOS-1 platform (22 m × 22 m resolution) for each 1° × 1° geographical latitude/longitude intersection; and multispectral camera sensor (4 m × 4 m resolution) onboard the KOMPSAT-2 satellite for each 2° × 2° geographical latitude/longitude intersection. This dataset will be used to assess the state of the tropical forest cover changes for the period 1990–2010 and is expected to constitute a benchmark dataset for accurate assessment of forest degradation and forest regrowth at continental level. shows a comparison of Landsat TM and Kompsat-2 imagery for 1990, 2000 and 2010 over one sample site in Papua New Guinea.
In the perspective of forest monitoring with higher level of details, it is important to note that the first satellite of the Sentinel-2 system, funded under a joint programme of the ESA and the European Commission, is planned to be launched in 2013 Citation[86]. The Sentinel-2 satellite will carry onboard an optical Earth observation multispectral optical sensor with 10 m × 10 m spatial resolution and swath of 290 km. With an orbiting revisit time of 10 days for one satellite and 5 days when a pair of satellites are operational, the Sentinel-2 system will include a systematic acquisition plan of satellite imagery over all terrestrial land areas of the world. The envisaged data policy will allow full and open access to Sentinel-2 data, aiming for maximum availability of Earth observation data in support of the implementation of environmental and climate change policies.
In the near future, the utility of Radar is also expected to be enhanced from better data access, processing and scientific developments. In particular, two future space missions should provide complementary SAR imagery systems for the monitoring of tropical forest areas and biomass. First, the Sentinel-1 mission is a pair of two C-band SAR sensors to be launched by ESA around the time of 2012. This system is designed to provide a biweekly global coverage of fine spatial resolution (5 m × 20 m) Radar imagery with a revisit time of 6 days Citation[87]. Second, the BIOMASS mission is one of the three candidates currently undergoing a feasibility study for the next Earth Explorer Core Mission of ESA’s Living Planet Programme to be launched around 2016 Citation[88]. The BIOMASS mission would carry a SAR P-band sensor aimed at acquiring regular spatial measurements of forest biomass over the globe with the provision of imagery with higher contrast between forest and nonforest areas and a continuous acquisition strategy.
It is expected that the future availability of Earth observation data at finer spatial resolution (e.g., 0.01 ha vs 0.1 ha) and higher temporal frequency (e.g., yearly vs 5-year interval), and combined with recent (e.g., using Landsat, ALOS PALSAR) and future full open data access strategy will allow for more accurate assessments of forest area changes in the tropics.
Future perspective
There are strong incentives to reduce uncertainty in the estimation of carbon fluxes arising from deforestation by using better data on forest above-ground biomass or carbon stocks in combination with more accurate satellite-derived estimates of deforestation Citation[89,90].
In the framework of the UNFCCC REDD-plus activities, the extension of the analysis of tropical deforestation to degradation and forest regrowth will be a crucial requirement Citation[91].
Dedicated tools for monitoring forest cover need to be improved through the use of the most recent satellite image technology, including radar and optical imagery at finer spatial resolution (10 m × 10 m or finer), with a higher temporal frequency (at least yearly) Citation[92]. The on-going methodological efforts are expected to narrow the gaps between the demand of more accurate estimation of global carbon budget and the limitations of currently available remote sensing data.
International coordination needs to be further developed between space agencies and implementing institutions (e.g., through the Committee on Earth Observation Satellites or the Group on Earth Observations) to ensure repeated coverage of the world’s forests with different types of data and easy access to adequate quality data at reasonable to no cost Citation[93].
Effective technology transfer on forest monitoring using remote sensing to local institutions throughout the tropics needs to be developed and sustained in the very near future.
Table 1. Main global land-cover maps derived from remote sensing data from 1 km × 1 km–300 m × 300 m spatial resolution.
Table 2. Availability of moderate resolution (20 m × 20 m to 50 m × 50 m) optical sensors.
As adopted by the United Nations Framework Convention on Climate Change at the 7th Conference of the Parties (COP-7) under the ‘Marrakesh accords’, “For land use, land-use change and forestry activities under Article 3, paragraphs 3 and 4 of the Kyoto Protocol, the following definitions shall apply: (a) “Forest” is a minimum area of land of 0.05–1.0 ha with tree crown cover (or equivalent stocking level) of more than 10–30 percent with trees with the potential to reach a minimum height of 2–5 m at maturity in situ. A forest may consist either of closed forest formations where trees of various stories, and undergrowth cover a high proportion of the ground or open forest […].” COP-7 further noted that parties recognize that there should be certain flexibility. To date, most countries are defining forests with a minimum crown cover of 30%.
As further adopted by the United Nations Framework Convention on Climate Change at COP-7, “Deforestation is the direct human-induced conversion of forested land to nonforested land”.
The gathering of information about planet Earth’s physical, chemical and biological systems. It is used to monitor and assess the status of, and changes in, the natural environment and the built environment. In recent years, Earth observation has become technologically more and more sophisticated. It has also become more important owing to the significant impact that modern human civilization is having on the Earth.
▪ The ability to monitor changes in forest area at the pan-tropical level with high accuracy is one crucial component for reducing scientific uncertainties in the estimates of greenhouse gas emissions from tropical deforestation. | |||||
▪ The monitoring of forest resources at a pan-tropical scale also contributes to the United Nations Framework Convention on Climate Change Reducing Emissions from Deforestation and Forest Degradation Plus activities by developing standard methods across regions, by monitoring potential leakage effects (displacement of deforestation between countries) and by providing global historical references. | |||||
▪ Analysis of remotely sensed data from satellites is the only practical approach to measure changes in forest area at pan-tropical to regional scales. With remote sensing technology, one can produce independent and up-to-date maps and estimates of forest cover changes. | |||||
▪ Various methods are available to appropriately to analyze satellite data for estimating forest cover and forest cover changes. At the pan-tropical scale, these methods range from wall-to-wall mapping with coarse to moderate resolution imagery to statistical sampling with moderate to fine resolution imagery. | |||||
▪ Since the early 1990s, changes in forest area at pan-tropical level can be measured from space with confidence, although uncertainties in estimates of deforestation need to be reduced for better understanding of the global climate budget and for policy implementation. | |||||
▪ Key constraints in implementing large scale systems for monitoring changes in forest cover are presently related to the availability and access to moderate resolution data (i.e., 20 m × 20 m to 50 m × 50 m), and in the near future, availability and access to finer resolution data (i.e., 10 m × 10 m resolution or finer after 2012). |
Acknowledgements
The authors thank the five anonymous referees for their helpful and constructive comments.
Financial & competing interests disclosure
The authors have no relevant affiliations or financial involvement with any organization or entity with a financial interest in or financial conflict with the subject matter or materials discussed in the manuscript. This includes employment, consultancies, honoraria, stock ownership or options, expert testimony, grants or patents received or pending, or royalties.
No writing assistance was utilized in the production of this manuscript.
Bibliography
- Global Forest Assessment 2010: Main Report. Food and Agriculture Organization of the UN, Rome, Italy (2010).
- DeFries RS, Rudel T, Uriarte M, Hansen M. Deforestation driven by urban population growth and agricultural trade in the twenty-first century. Nature Geosci3,178–181 (2010).
- Gibbs K, Ruesch AS, Achard F et al. Tropical forests were the primary sources of new agricultural land in the 1980s and 1990s. Proc. Natl Acad. Sci. USA107(38),16732–16737 (2010).
- Rudel TK, Defries R, Asner GP, Laurance WF. Changing drivers of deforestation and new opportunities for conservation. Conserv. Biol.23(6),1396–1406 (2009).
- Penman J, Gytarsky M, Hiraishi T et al.Good Practice Guidance for Land Use, Land-Use Change and Forestry. IPCC National Greenhouse Gas Inventories Programme and Institute for Global Environmental Strategies. Kanagawa, Japan (2003).
- Denman KL, Brasseur G, Chidthaisong A et al. Couplings between changes in the climate system and biogeochemistry. In: Climate Change 2007: The Physical Science Basis. Contribution of Working Group I to the Fourth Assessment Report of the Intergovernmental Panel on Climate Change. Solomon S, Qin D, Manning M et al. (Eds). Cambridge University Press, Cambridge, UK (2007).
- Asner GP, Powell GVN, Mascaro J et al. High-resolution forest carbon stocks and emissions in the Amazon. Proc. Natl Acad. Sci. USA107(38),16738–16742 (2010).
- van der Werf GR, Morton DC, DeFries RS et al. CO2 emissions from forest loss. Nature Geosci.2,737–738 (2009).
- Lewis SL, Lopez-Gonzalez G, Sonké B et al. Increasing carbon storage in intact African tropical forests. Nature457,1003–1006 (2009).
- Stephens BS, Gurney KR, Tans PP et al. Weak northern and strong tropical land carbon uptake from vertical profiles of atmospheric CO2. Science316,1732–1735 (2007).
- Solomon S, Qin D, Manning M et al. Technical summary. In: Climate Change 2007: The Physical Science Basis. Contribution of Working Group I to the Fourth Assessment Report of the Intergovernmental Panel on Climate Change. Solomon S, Qin D, Manning M et al. (Eds). Cambridge University Press, Cambridge, UK (2007).
- Houghton RA. Aboveground forest biomass and the global carbon balance. Glob. Chang. Biol.11,945–958 (2005).
- Ramankutty N, Gibbs H, Achard F, DeFries RS, Foley J, Houghton RA. Challenges to estimating carbon emissions from tropical deforestation. Glob. Chang. Biol.13,51–66 (2007).
- Baker DJ, Richards G, Grainger A et al. Achieving forest carbon information with higher certainty: a five-part plan. Environ. Sci. Policy.13,249–260 (2010).
- Global Forest Resources Assessment 2000. Food and Agriculture Organization of the UN. Rome, Italy,140 (2001).
- Achard F, Eva HD, Mayaux P, Stibig H-J, Belward A. Improved estimates of net carbon emissions from land cover change in the tropics for the 1990s. Global Biogeochem. Cycles18(GB2008) (2004).
- DeFries RS, Houghton RA, Hansen M, Field C, Skole DL, Townshend J. Carbon emissions from tropical deforestation and regrowth based on satellite observations for the 1980s and 90s. Proc. Natl Acad. Sci. USA99(22),14256–14261 (2002).
- Keith H, Mackey BG, Lindenmayer DB. Re-evaluation of forest biomass carbon stocks and lessons from the world’s most carbon-dense forests. Proc. Natl Acad. Sci. USA106(28),11635–11640 (2009).
- McRoberts RE, Cohen WB, Næsset E, Stehman SV, Tomppo EO. Using remotely sensed data to construct and assess forest attribute maps and related spatial products. Scand. J. For. Res.25(4),340–367 (2010).
- Baccini A, Laporte N, Goetz SJ, Sun M, Dong H. A first map of tropical Africa’s above-ground biomass derived from satellite imagery. Environ. Res. Lett.3,045011 (2008).
- Gibbs HK, Brown S, Niles JO, Foley JA. Monitoring and estimating tropical forest carbon stocks: making REDD a reality. Environ. Res. Lett.2(4),045023 (2007).
- Saatchi SS, Houghton RA, Dos Santos Alvala RC, Soares JV, Yu Y. Distribution of aboveground live biomass in the Amazon basin. Glob. Chang. Biol.13,816–837 (2007).
- Houghton RA, Hall FG, Goetz SJ. The importance of biomass in the global carbon cycle. J. Geophys. Res. Biogeosci.114,G00E03 (2009).
- Methodological Guidance for Activities Relating to Reducing Emissions from Deforestation and Forest Degradation and the Role of Conservation, Sustainable Management of Forests and Enhancement of Forest Carbon Stocks in Developing Countries. UNFCCC Secretariat, Bonn, Germany (2009).
- Seventh Conference of Parties: The Marrakech Accords. UNFCCC Secretariat, Bonn, Germany (2002).
- Mollicone D, Achard F, Federici et al. An incentive mechanism for reducing emissions from conversion of intact and non-intact forests. Clim. Change83,477–493 (2007).
- Moving Ahead with REDD: Issues, Options and Implications.Angelsen A (Ed.). Center for International Forestry Research, Bogor, Indonesia (2008).
- Grainger A. Difficulties in tracking the long-term trend in tropical forest area. Proc. Natl Acad. Sci. USA105(2),818–823 (2008).
- McRoberts RE, Tomppo EO. Remote sensing support for national forest inventories. Remote Sens. Environ.110(4),412–419 (2007).
- DeFries RS, Achard F, Brown S et al. Earth observations for estimating greenhouse gas emissions from deforestation in developing countries. Environ. Sci. Policy10,385–394 (2007).
- Achard F, DeFries R, Eva HD, Hansen M, Mayaux P, Stibig H-J. Pan-tropical monitoring of deforestation. Environ. Res. Lett.2,045022 (2007).
- A Sourcebook of Methods and Procedures for Monitoring and Reporting Anthropogenic Greenhouse Gas Emissions and Removals Caused by Deforestation, Gains and Losses of Carbon Stocks in Forests Remaining Forests, and Forestation. Achard F, Brown S, De Fries R et al. (Eds). Global Observation of Forest and Land Cover Dynamics, Alberta, Canada (2009).
- Good Practice Guidance for Land Use, Land-Use Change and Forestry (GPG-LULUCF). Intergovernmental Panel on Climate Change. Institute for Global Environmental Strategies, Kanagawa, Japan (2003).
- Coppin P, Jonckheere I, Nackaerts K, Muys B. Digital change detection methods in ecosystem monitoring: a review. Int. J. Remote Sens.25(9),1565–1596 (2004).
- Fritz S, McCallum I, Schill C et al. Geo-wiki.org: the use of crowdsourcing to improve global land cover. Remote Sens.1,345–354 (2009).
- Bartholomé E, Belward AS. GLC2000: a new approach to global land cover mapping from Earth observation data. Int. J. Remote Sens.26(9),1959–1197 (2005).
- Friedl MA, McIver DK, Hodges JCF et al. Global land cover mapping from MODIS: algorithms and early results. Remote Sens. Environ.83(1–2),287–302 (2002).
- Herold M, Woodcock C, Di Gregorio A et al. A joint initiative for harmonization and validation of land cover datasets. IEEE Trans. Geosci. Remote Sens.44,1719–1727 (2006).
- Hansen MC, Townshend J, DeFries RS, Carroll M. Estimation of tree cover using MODIS data at global, continental and regional/local scales. Int. J. Remote Sens.26(19),4359–4380 (2005).
- Friedl MA, Sulla-Menashe D, Tan B et al. MODIS Collection 5 global land cover: algorithm refinements and characterization of new datasets. Remote Sens. Environ.114(1),168–182 (2010).
- Arino O, Bicheron P, Achard F, Latham J, Witt R, Weber JL. The most detailed portrait of Earth. European Space Agency Bulletin136,24–31 (2008).
- Eva HD, Belward AS, De Miranda E et al. A land cover map of South America. Global Change Biol.10,732–745 (2004).
- Mayaux P, Bartholomé E, Fritz S, Belward AS. A new landcover map of Africa for the year 2000. J. Biogeogr.31,1–17 (2004).
- Stibig HJ, Belward AS, Roy PS et al. A land cover map for South and Southeast Asia derived from SPOT-VEGETATION data. J. Biogeogr.34,625–637 (2007).
- Vancutsem C, Pekel J-F, Evrard C, Malaisse F, Defourny P. Mapping and characterizing the vegetation types of the Democratic Republic of Congo using SPOT-VEGETATION time series. Int. J. Appl. Earth Obs. Geoinf.11,62–76 (2009).
- Strahler AH, Boschetti L, Foody GM et al.Global Land Cover Validation: Recommendations for Evaluation and Accuracy Assessment of Global Land Cover Maps. EUR 22156 EN. Office for Official Publications of the European Communities, Luxembourg (2006).
- Lepers E, Lambin EF, Janetos AC et al. A synthesis of information on rapid land-cover change for the period 1981–2000. Bioscience55,115–124 (2005).
- Hansen MC, Stehman SV, Potapov PV et al. Humid tropical forest clearing from 2000 to 2005 quantified by using multitemporal and multiresolution remotely sensed data. Proc. Natl Acad. Sci. USA105(27),9439–9444 (2008).
- Monitoramento da Floresta Amazônica Brasileira por Satelite, Projeto PRODES. Instituto de Pesquisas Espaciais, São Jose dos Campos, Brazil (2010).
- Tucker CJ, Grant DM, Dykstra JD. NASA’s Global Orthorectified Landsat Data Set. Photogramm. Eng. Remote Sens.70,313–322 (2004).
- Gutman G, Byrnes R, Masek J et al. Towards monitoring land-cover and land-use changes at a global scale: the Global Land Survey 2005. Photogramm. Eng. Remote Sens.74(1),6–10 (2008).
- Franks S, Masek J, Headley R, Gasch J, Arvidson T. Large area scene selection interface (LASSI) methodology of selecting landsat imagery for the Global Land Survey 2005. Photogramm. Eng. Remote Sens.75,1287–1296 (2009).
- Böttcher H, Eisbrenner K, Fritz S et al. An assessment of monitoring requirements and costs of ‘Reduced Emissions from Deforestation and Degradation’.Carbon Balance Manag.4(7) DOI: 10.1186/1750-0680-4-7 (2009) (Epub).
- Morton D, DeFries RS, Shimabukuro Y et al. Rapid assessment of annual deforestation in the Brazilian Amazon using MODIS data. Earth Interact.9,1–22 (2005).
- Bontemps S, Langner A, Defourny P. Monitoring forest changes in Borneo on a yearly basis by an object-based change detection algorithm using Spot-VEGETATION time series. Int. J. Remote Sens. (2010) (In Press).
- Czaplewski RL. Can a sample of Landsat sensor scenes reliably estimate the global extent of tropical deforestation? Int. J. Remote Sens.24(6),1409–1412 (2003).
- Stehman SV. Comparing estimators of gross change derived from complete coverage mapping versus statistical sampling of remotely sensed data. Remote Sens. Environ.96(3–4),466–474 (2005).
- Mayaux P, Holmgren P, Achard F, Eva HD, Stibig HJ, Branthomme A. Tropical forest cover change in the 1990s and options for future monitoring. Philos. Trans. R. Soc. B Biol. Sci.360,373–384 (2005).
- Gallego FJ. Stratified sampling of satellite images with a systematic grid of points. ISPRS J. Photogramm. Remote Sens.59,369–376 (2005).
- Drigo R, Lasserre B, Marchetti M. Patterns and trends in tropical forest cover. Plant Biosystems143(2),311–327 (2009).
- Broich M, Stehman SV, Hansen MC, Potapov P, Shimabukuro YE. A comparison of sampling designs for estimating deforestation from Landsat imagery: a case study of the Brazilian legal Amazon. Remote Sens. Environ.113(11),2448–2454 (2009).
- Eva HD, Carboni S, Achard F et al. Monitoring forest areas from continental to territorial levels using a sample of medium spatial resolution satellite imagery. ISPRS J. Photogramm. Remote Sens.65,191–197 (2010).
- Potapov P, Hansen MC, Gerrand AM et al. The global Landsat imagery database for the FAO FRA remote sensing survey. Int. J. Digital Earth1,1753–8955 (2010).
- Ridder RM. Options and Recommendations for a Global Remote Sensing Survey of Forests. Food and Agriculture Organization of the UN, Rome, Italy (2007).
- The 2010 Global Forest Resources Assessment Remote Sensing Survey: An Outline of the Objectives, Data, Methods and Approach. Food and Agriculture Organization of the UN, Rome, Italy (2009).
- Bodart C, Beuchle R, Raši R et al. Pre-processing and segmentation of a global sample of multi-scene Landsat imagery used to monitor forest cover changes over the tropics. ISPRS-J. Photogramm. Remote Sens. (2010) (In Press).
- Duveiller G, Defourny P, Desclée B, Mayaux P. Deforestation in Central Africa: estimates at regional, national and landscape levels by advanced processing of systematically-distributed Landsat extracts. Remote Sens. Environ.112(5),1969–1981 (2008).
- Desclée B, Bogaert P, Defourny P. Forest change detection by statistical object-based method. Remote Sens. Environ.102(1–2),1–11 (2006).
- Stach N, Salvado A, Petit M et al. Land use monitoring by remote sensing in tropical forest areas in support of the Kyoto Protocol: the case of French Guiana. Int. J. Remote Sens.30(19),5133–5149 (2009).
- Steininger MK, Godoy F, Harper G. Effects of systematic sampling on satellite estimates of deforestation rates. Environ. Res. Lett.4,034015 (2009).
- State of Forest Report 2009. Ministry of Environment and Forest, Dehra Dun, India (2009).
- Hansen MC, Roy DP, Lindquist E et al. A method for integrating MODIS and Landsat data for systematic monitoring of forest cover and change in the Congo Basin. Remote Sens. Environ.112(5),2495–2513 (2008).
- Lindquist EJ, Hansen MC, Roy DP, Justice CO. The suitability of decadal image data sets for mapping tropical forest cover change in the Democratic Republic of Congo: implications for the global land survey. Int. J. Remote Sens.29,7269–7275 (2008).
- Asner GP, Knapp DE, Balaji A, Paez-Acosta G. Automated mapping of tropical deforestation and forest degradation: CLASlite. J. Appl. Remote Sens.3,033543 (2009).
- Le Toan T, Quegan S, Woodward I, Lomas M, Delbart N, Picard G. Relating radar remote sensing of biomass to modelling of forest carbon budgets. Clim. Change67,379–402 (2004).
- Rosenqvist A, Finlayson CM, Lowry J, Taylor D. The potential of long-wavelength satellite-borne radar to support implementation of the Ramsar Wetlands Convention. Aquatic Conservation: Marine and Freshwater Ecosystems17,229–244 (2007).
- Almeida-Filho R, Shimabukuro YE, Rosenqvist A, Sanchez GA. Using dual-polarized ALOS PALSAR data for detecting new fronts of deforestation in the Brazilian Amazônia. Int. J. Remote Sens.30(14),3735–3743 (2009).
- Navarro de Mesquita H Jr, Crislei Silva M, Freitas de Moraes D et al. Law enforcement deforestation assessment. In: K&C Science report – Phase 1. Japanese Aerospace Exploration Agency, EORC report NDX-100003 (2009).
- Hoekman D, Vissers M, Wielaard N. PALSAR wide-area mapping of Borneo: methodology and map validation. IEEE JSTARS DOI: 10.1109/JSTARS.2010.2070059 (2010) (Epub ahead of print).
- Walker WS, Stickler CM, Kellndorfer JM, Kirsch KM, Nepstad DC. Land cover in the Brazilian Amazon: a comparative analysis of ALOS/PALSAR and Landsat data sources. IEEE JSTARS. DOI: 10.1109/JSTARS.2010.2076398 (2010) (Epub ahead of print).
- Mayaux P, De Grandi GF, Rauste Y, Simard M, Saatchi S. Large Scale vegetation maps derived from the combined L-band GRFM and C-band CAMP wide area radar mosaics of Central Africa. Int. J. Remote Sens.23(7),1261–1282 (2002).
- Sgrenzaroli M, De Grandi GF, Eva HD, Achard F. Tropical forest cover monitoring: estimates from the GRFM JERS-1 radar mosaics using wavelets zooming techniques and Validation. Int. J. Remote Sens.23(7),1329–1355 (2002).
- Stehman S. Sampling designs for accuracy assessment of land cover. Int. J. Remote Sens.30(20),5243–5272 (2009).
- Grassi G, Monni S, Federici S, Achard F, Mollicone D. Applying the conservativeness principle to REDD to deal with the uncertainties of the estimate. Environ. Res. Lett.3,035005 (2008)
- Foody GM. Assessing the accuracy of land cover change with imperfect ground reference data. Remote Sens. Environ.114(10),2271–2285 (2010).
- Martimort P, Berger M, Carnicero B et al. Sentinel-2 the optical high-resolution mission for GMES operational services. European Space Agency Bulletin131,19–23 (2007).
- Attema E, Bargellini P, Edwards P et al. Sentinel-1 the radar mission for GMES operational land and sea services. European Space Agency Bulletin131,11–17 (2007).
- Le Toan T, Quegan S, Davidson MWJ et al. The BIOMASS Mission: mapping global forest biomass to better understand the terrestrial carbon cycle. Remote Sens. Environ. (2010) (In Press).
- Goetz SJ, Baccini A, Laporte NT et al. Mapping and monitoring carbon stocks with satellite observations: a comparison of methods. Carbon Balance and Manag.4,2 (2009).
- Lefsky MA. A global forest canopy height map from the moderate resolution imaging spectroradiometer and the geoscience laser altimeter system. Geophys. Res. Lett.37,L15401 (2010).
- Asner GP, Rudel TK, Aide TM, Defries R, Emerson R. A contemporary assessment of change in humid tropical forests. Conserv. Biol.23(6),1386–1395 (2009).
- Kintish E. Improved monitoring of rainforests helps pierce haze of deforestation. Science316,536–537 (2007).
- Report on the Concept Phase for developing a Global Forest Observations Initiative. Planning Group under the GEO Forest Carbon Tracking Task (GEO Task CL-09–03b). The Group on Earth Observations, Geneva, Switzerland (2010).
- Loveland TR, Zhu Z, Ohlen DO, Brown JF, Reed C, Yang L. An analysis of the IGBP global land-cover characterization process. Photogramm. Eng. Remote Sens.1021–1032 (1999).
- Hansen MC, DeFries RS, Townshend JRG, Sohlberg R. Global land cover classification at 1 km spatial resolution using a classification tree approach. Int. J. Remote Sens.21,1331–1364 (2000).
- Achard F, Eva HD, Mayaux P. Tropical forest mapping from coarse spatial resolution satellite data: production and accuracy assessment issues. Int. J. Remote Sens.22(14),2741–2762 (2001).
▪ Websites
- Earth Observation General Coordination (OBT). Sistema Deter. www.obt.inpe.br/deter
- US Geological Survey. Global Visualisation Viewer. http://glovis.usgs.gov
- Global Forest Resources Assessment. Landsat Imagery Database. http://globalmonitoring.sdstate.edu/projects/fao/index.html
- Earth Observation General Coordination (OBT). Projecto Prodes. www.obt.inpe.br/prodes
- Imagery for everyone: timeline set to release entire USGS Landsat archive at no charge. USGS Technical Announcement (2010). http://landsat.usgs.gov/documents