Abstract
Background: The uncertainty of our understanding of the spatial variation in tropical forest biomass is still very high, due to lack of information on the effect of disturbances on carbon dynamics. Results: The spatial variation of aboveground living biomass (AGLB) in the tropical lowland forests of Mexico was high and show links with recorded human and natural disturbances. Areas with high biomass are limited to southeast Yucatan and east Chiapas. In plots with fire, land-use change and grazing, AGLB was approximately 20% lower than in undisturbed plots. Plots with forest management had higher AGLB. Conclusion: The high biomass outlier plots are an indication of the stock enhancement potential of the forests. The inventory design could be improved by collecting more quantitative information on disturbance intensity and history.
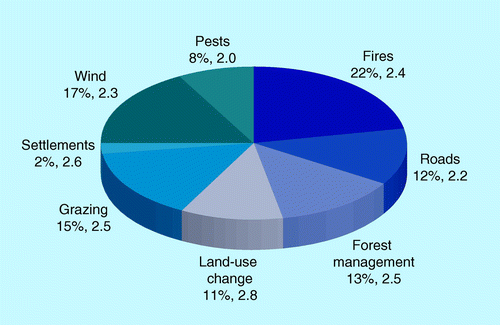
(A) Pests and diseases; (B) wind. Scale: 1 = very light to 4 = severe.EYP: East Yucatan Peninsula; GM-EC: The Gulf of Mexico and eastern Chiapas; SMF: Sierra Madre Foothills; SPC: South Pacific Coast; SYP: South Yucatan Peninsula; WPC: West Pacific Coast; WYP: West Yucatan Peninsula.
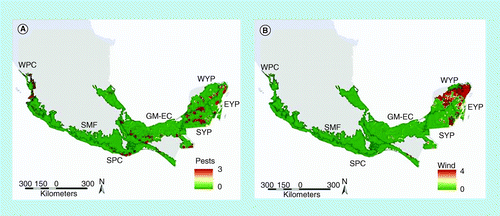
(A) Fire; (B) grazing; (C) land-use change; (D) forest management; (E) roads; and (F) settlements. Scale: 1 = very light to 4 = severe.
EYP: East Yucatan Peninsula; GM-EC: The Gulf of Mexico and eastern Chiapas; LUC: Land-use change; Mgmt: Forest management; SMF: Sierra Madre Foothills; SPC: South Pacific Coast; SYP: South Yucatan Peninsula; WPC: West Pacific Coast; WYP: West Yucatan Peninsula.
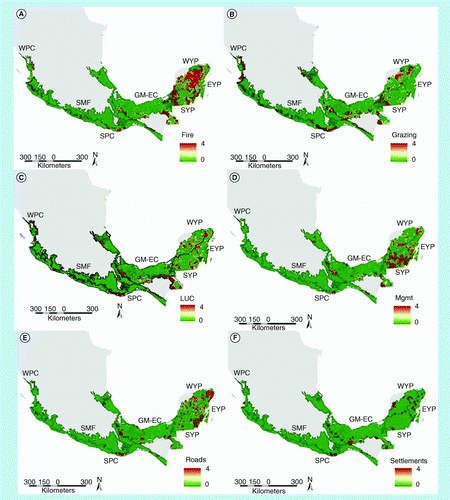
(A) Human disturbances; and (B) natural disturbances. Human disturbances scale: 0 = no disturbance to 16 = severe; natural disturbances scale: 0 = no disturbance to 6 = severe.
EYP: East Yucatan Peninsula; GM-EC: The Gulf of Mexico and eastern Chiapas; SMF: Sierra Madre Foothills; SPC: South Pacific Coast; SYP: South Yucatan Peninsula; WPC: West Pacific Coast; WYP: West Yucatan Peninsula.
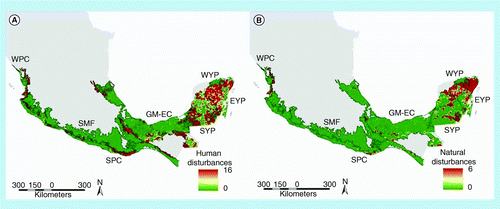
(A) Biomass clusters and outliers. (B) Aboveground living biomass, derived from the inverse weighted distance interpolation.
EYP: East Yucatan Peninsula; GM-EC: The Gulf of Mexico and eastern Chiapas; H-H: Clusters of high biomass plots; H-L: Outlier of a high biomass plot within a cluster of low biomass plots; L-H: Low biomass plot within a cluster of high biomass plots; L-L: Clusters of low biomass plots; SMF: Sierra Madre Foothills; SPC: South Pacific Coast; SYP: South Yucatan Peninsula; WPC: West Pacific Coast; WYP: West Yucatan Peninsula.
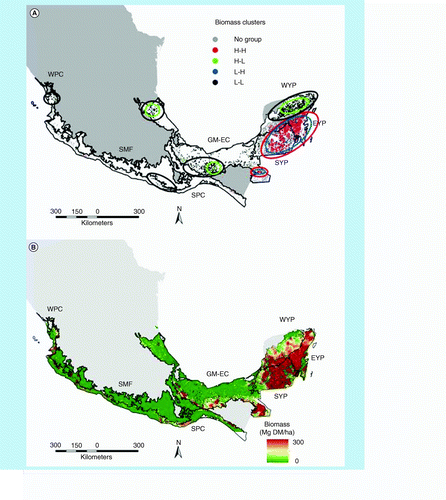
Forests are important components of the global carbon cycle Citation[1], either as sinks, by removing carbon from the atmosphere through the process of photosynthesis, or as sources due to biomass burning or decomposition. Literature is appearing at the global level that points out the inherent variation that exists in ecosystem carbon pools at multiple spatial scales and under different land uses Citation[2]. This is important because successful design and implementation of future strategies intended to reduce emissions through deforestation and forest degradation, or to manage and conserve stocks (REDD+), requires an understanding of carbon dynamics. This understanding includes both the size of carbon pools and the rates of carbon sequestration or loss in all pools by means of forest recovery and degradation Citation[3]. Despite the importance of neotropical forests to global carbon cycling and the growing literature on the variation in ecosystem carbon, the impacts of land management and human disturbance on carbon dynamics are still poorly understood in tropical forests Citation[4–6], considering a disturbance as a disruption of the state variables or fluxes of an ecosystem by any force external to the system (wind, fire, human disturbance, pests and diseases Citation[7]).
Forest conversion to agriculture or pasture land is readily monitored from space using satellite imagery, permitting the development of deforestation maps of large regions at a reasonable cost and speed Citation[8]. This approach to analyze the human effects on tropical forests neglects those forest alterations that reduce tree cover, such as logging and surface fires in standing forests Citation[9]. The current uncertainty of the spatial variation in tropical forest biomass is as important as the uncertainty in the rate of deforestation in the error of estimated net fluxes of carbon Citation[10]. In the tropics, an uncertainty of 50% in average biomass translates into an uncertainty of approximately 80% in estimates of flux. The number one uncertainty rests in the spatial variation of aboveground carbon stocks in primary forests, secondary forests and on fallow land Citation[10].
Conserving tropical forests by means of reducing forest disturbances is an important strategy for mitigating climate change Citation[8]. Other strategies include promoting sustainable forest management in suitable areas or stock enhancement in areas where carbon stock has been reduced due to human or natural disturbances. This requires an understanding of the role of the human and natural disturbances on forest biomass and forest structure and their spatial distribution. Factors that may influence the impact of disturbances are, among others, simultaneously occurring natural and human disturbance events and their variation in intensity. Knowing the spatial distribution of forest biomass is required for calculating the sources of carbon emissions that result from converting a forest to cleared land. While average biomass values have been used in most calculations of carbon flux to date, the possibility that forest degradation occurs in forests with biomass that is significantly different from the average suggests that linking specific locations of disturbance with geographically specific estimates of biomass would improve estimates of flux Citation[11].
There is a need to better document the location, date and, especially, the cause of disturbance (natural or human-induced). The carbon stocks in forests may change substantially without a change in forest area due to losses of biomass associated with human or natural disturbances Citation[8,12,13]. Human-induced, as well as natural disturbances, create patches with varying biomass with a spatial variability that may be as great between adjacent hectares as it is over thousands of kilometers Citation[10]. Loss of carbon from some reference point may be difficult to measure in countries where forests have been influenced by human beings for centuries Citation[8].
Disturbances have five components that are relevant to assessing their potential ecosystem effects Citation[7]: severity or intensity, frequency of occurrence, duration, spatial scale and point(s) of interaction with the ecosystem Citation[14–17]. If different disturbances occur at the same time in the same place, the cumulative effect of these on the ecosystem parameters is also of importance, particularly if a REDD+ mechanism is planned to be installed that reduces the impact of these disturbances.
In order to develop strategies within the framework of REDD+, it is important to analyze spatial distribution of biomass and the effect of specific disturbances on biomass that can be related to emissions of GHGs. To distinguish the spatial distribution of the disturbances, maps are required that indicate where specific disturbances are concentrated that can then be used to develop measures to control or avoid these disturbances. Tackling emission reductions of forest degradation needs to take into account to what extent certain disturbance events modify the biomass and other ecological services provided by the forests or create conditions that intensify the effect of subsequent disturbances Citation[18].
In Mexico, large tracts of tropical lowland forests are already considered as secondary forests due to the impact of human and natural disturbances Citation[19]. Land-use maps classified up to 70% of tropical lowland forests as secondary forests, without indicating if they were recovering from human or natural disturbances Citation[20]. It is not clear what are the specific events occurring in the forests that created the secondary forests and what are the links between these events and the biomass in these forests. Important disturbances known to occur in Mexican forests include human-induced fires, derived from out-of-control burning of land used for slash-and-burn agriculture, or burning of grassland to control shrubs and to induce rapid regrowth of grasses. Other important disturbances consist of land-use change (LUC) as part of the slash-and burn system, grazing, establishment of temporal and permanent roads, clearing of forests to establish settlements and forest management. Natural disturbances known to occur include damage due to windstorms, particularly in areas where hurricanes touch land, and forest pests and diseases. Some of these disturbances have a direct long-term effect on forest structure and biomass, such as intensive fires, LUC, settlements and roads, whereas others have a relative short-term and reduced effect, such as selective logging and grazing. However, the former may be followed by LUC and the latter take place mainly in woodlands, plantations and secondary forests that are part of the slash-and-burn system, and as such can be considered as an indicator of previous disturbances.
The objective of this paper is to analyze the spatial distribution of aboveground living biomass (AGLB) and reported disturbance events, to test if the presence of recorded disturbances can explain the spatial variation in biomass, and to estimate the relation between specific human disturbances and biomass in the tropical lowland forests of Mexico.
Methodology
The analysis is based on data of sampling plots of the Mexican National Forest and Soils Inventory of 2004–2008 (NFSI0408), corresponding to medium (canopy height 15–30 m) and high (canopy height >30 m) stature semi-deciduous (25–50% of trees evergreen) to evergreen tropical forests (medium to high tropical forest [MHTF]). These forest types occupy an area of approximately 135,570 km2, of which approximately 75% is considered secondary forest Citation[20]. These forest types cover most of the Yucatan Peninsula, and lowlands and foothills of the Gulf of Mexico and Pacific coast up to Tamaulipas and Nayarit, respectively.
Data from a total of 4136 sampling plots of the NFSI0408 are used in the analysis. The plots are systematically distributed over the forested area, with a plot distance of 5 by 5 km, with a plot density of one plot for each 3.3 km2 of MHTF. The selected plots correspond to seven ecoregions (ecoregions with less than 25 plots were excluded from the analysis) . The following is a short description of each of the seven ecoregions Citation[21].
▪ South Yucatan Peninsula (47,915 km2)
This is the most conserved region in the Yucatan Peninsula, covering the southern part of Campeche and Quintana Roo. The climate is warm and the mean annual precipitation varies from 1100 mm in the north to 1500 mm in the south. Vegetation is dominated by medium stature semi-evergreen (50–75% evergreen) and semi-deciduous forests with different levels of conservation, mixed with patches of low stature (canopy 4–15 m) semi-evergreen forest. There are a few small areas of high stature evergreen and semi-evergreen forest in the south. Forests corresponding to MHTF cover approximately 30,500 km2.
Parts of the area have been converted over time to livestock production, with small patches of subsistence agriculture. There has been some irrigation and nonirrigation agriculture, with crops including rice, sorghum, beans and chilies.
▪ West Yucatan Peninsula (42,341 km2)
This region is located on the northwestern part of the Yucatan peninsula, and includes parts of the states of Yucatan and Campeche. The climate is warm sub-humid to dry, with precipitation varying between 500 and 800 mm. Types of forests in this region include medium and low stature deciduous to semi-evergreen forests. Forests corresponding to MHTF cover approximately 30,500 km2. These forests are largely degraded and are in a continuing process of conversion to grasslands and agriculture.
▪ East Yucatan Peninsula (29,204 km2)
This region is located on the eastern part of the Yucatan peninsula and covers most of the state of Quintana Roo. Climate is warm subhumid with intermediate rains. Forest type is mostly medium stature tropical semi-evergreen forest. A large part of the land area is covered with dense forest that has been fairly well conserved. Forests corresponding to MHTF cover approximately 21,600 km2. There is very little agricultural activity, and the principal economic land-use based activity is forest management.
▪ South Pacific Coast (including Soconusco; 67,687 km2)
This region extends across the entire Southern Pacific coastal plains and adjoining hills from Michoacán to Chiapas. Climates are primarily warm, but there are also a few semi-warm and temperate climates in areas with higher elevation. Deciduous tropical forest predominates, pine and mixed pine–oak forests are found in higher elevation and tropical evergreen and semi-evergreen forests are found in the southern part, although these are gradually converted to secondary forests. Forests corresponding to MHTF cover approximately 12,100 km2. Agriculture and livestock are important land-use activities, as well as semi-permanent and permanent irrigated cropping systems.
▪ Gulf of Mexico & Eastern Chiapas (130,276 km2)
This region is located within the Veracruz and Tabasco Coastal Plains and part of Campeche, plus portions of San Luis Potosí, Hidalgo, Puebla, Oaxaca, and eastern and northern Chiapas. The climate is predominantly warm to semi-warm and humid to subhumid. Tropical evergreen forests in the coastal plains have been converted to grasslands and croplands. Eastern Chiapas still contains large areas of primary evergreen tropical forests, particularly in the Montes Azules biosphere reserve, although large tracts outside the reserve suffered some type of alteration. Forests corresponding to MHTF cover approximately 33,150 km2. This region has abundant water, in the form of rivers, lagoons and marshes. The most important economic activity is petroleum industry; livestock and agriculture are also very important economic activities.
▪ West Pacific Coast (11,660 km2)
This region is located within the coastal plains of Nayarit and Sinaloa and the coastal Sierras of Nayarit, Jalisco and Colima. Climate is generally warm and subhumid to dry. The dominant vegetation includes mangrove forests and medium stature tropical semi-evergreen and semi-deciduous forests, which is typical in protected canyons and sierras and in the interior of this region. Another important type of vegetation is low stature deciduous tropical forest. Forests corresponding to MHTF cover approximately 4300 km2. Irrigation agriculture is very important in the coastal plains, whereas in the sierras agriculture is mostly seasonal, with annual and permanent crops and cultivated grasslands.
▪ Sierra Madre Foothills (93,607 km2)
This region covers the sierras of Jalisco, Michoacán, Guerrero and Oaxaca. The climate of the region is varied, but is mostly warm and subhumid to humid in the areas with the tropical forests. Natural vegetation is maintained with varying degrees of alteration of cloud forests, pine and oak forests at the higher elevations and (semi)deciduous tropical forests at the lower elevations. Forests corresponding to MHTF cover approximately 3300 km2. Main land-use activities are wood exploitation, livestock and subsistence agriculture.
Data collected in each plot include measurements of all individual trees located in four 400 m2 subplots and the visible evidence of eight major disturbance events, recorded in five intensity classes. The subplots are distributed in a y-shape in such a way that the area surrounding the four subplots covers exactly one hectare. All living standing plants with a diameter at breast height (DBH; 1.30 m) larger than 7.5 cm were identified at the level of species; in addition, total height, bole height and crown diameter were recorded.
Biomass of living trees, lianas and palms were calculated based on the reported DBH, total height and species, applying published national allometric equations Citation[22–24]. For those species whose biomass was calculated with an ecosystem-type equation, the outcome was adjusted with published information on specific wood density Citation[24]. All biomass data were transformed to Mg dry matter per hectare (Mg DM/ha).
A total of eight disturbances were used for the analysis, subdivided into human disturbances (fire, roads, forest management, LUC, grazing and settlements) and natural disturbances (wind, pests and diseases). Each disturbance is reported in five intensity classes according to the visible evidence of the intensity of the specific disturbance (0: not present; 1: very light, hardly visible; 2: light, visible but small surface affected; 3: medium, visible and approximately half of the surface affected; and 4: severe; most of the surface affected by the disturbance). The following is a short description of each disturbance event .
▪ Spatial analysis
A cluster and outlier analysis was performed with the Anselin Local Moran’s I statistic to delimit areas with concentrations of high and low biomass plots and to identify plots with spatial outliers Citation[25]. The analysis distinguishes between statistically significant (p < 0.05) clusters of high biomass plots (H-H), clusters of low biomass plots (L-L), an outlier of a high biomass plot within a cluster of low biomass plots (H-L) or a low biomass plot within a cluster of high biomass plots (L-H). H-H clusters are ideal to implement action to avoid emissions from deforestation or implement sustainable forest management, L-L clusters require actions to increase biomass, while H-L outliers give an indication to what extent biomass can be increased in the L-L clusters, and finally the number of L-H outliers and their biomass indicate to what extent H-H clusters are fragmented.
The spatial distribution of the biomass and the reported intensity of each specific disturbance event, the sum of intensities of all human disturbances and the sum of all natural disturbances recorded in each plot, are visualized in maps, applying an inverse weighted distance interpolation of the georeferenced plot data. This procedure estimates values of points or areas without measurements, interpolating the information of the surrounding data points, with a linear decay function according to the distance to the data points applied in such a way that the information of distant points is less important than points close by for the estimation. The biomass and disturbances maps can be used to define the type of actions that need to be implemented in specific areas to define the type of REDD+ activity that needs to be developed and what type of disturbance event(s) need to be controlled.
▪ Statistical analysis
A square root conversion of the aboveground biomass data (Sqrt[AGLB]) was required to fit the data to ANOVA assumptions. All statistical analysis are thus performed with the transformed biomass data and, where required, back-transformed to total AGLB.
The average AGLB of the biomass clusters is calculated for the whole region and for those ecoregions where the clusters and outlier types occur. A multiple factor ANOVA was used to test if the total variance of Sqrt(AGLB) can be explained by the clustering of biomass plots and the presence–absence of human and natural disturbance events for all plots and for plots in those ecoregions where all cluster and outlier types occur.
A forward multiple generalized linear regression is used to select the specific human disturbance events that are statistically correlated with Sqrt(AGLB) for all plots where no natural disturbances were reported. This analysis is also applied to the plots in each ecoregion separately, to detect possible spatial variation of the importance of specific human disturbance events on biomass. The statistically significant linear regressions are applied to all plot data to estimate the effect of the specific disturbances on biomass for the whole area and for each ecoregion. The results can be used to identify the most important human disturbance events in terms of their relation to biomass.
Results
▪ Spatial distribution & intensity of reported disturbances events
Only 35% of the 4136 plots have no visible evidence of human or natural disturbances, in 26% of the plots at least one natural disturbance has been reported, and in 54% of the plots at least one human disturbance is observed. In 15% of the plots, at least one natural and one human disturbance event is reported simultaneously .
In approximately 36% of the plots visible evidence of one specific disturbance event is reported, 17% with two events, 8% with three events, and 4% with more than three events. The accumulated intensity of the reported events (0: specific event is not reported; 1: very light disturbance event; 2: light disturbance event; 3: medium disturbance event; and 4: severe disturbance event) range between 0 and 19; in approximately 79% of the plots the accumulated intensity ranges from 0 to 4, in 10% from 5 to 6, and in the remaining 11% of the plots the sum ranges from 7 to 19. It is noteworthy that approximately 45% of the plots where LUC is reported also show evidence of grazing, whereas 21% of the plots with wind damage are also affected by fire.
Fire evidence is the most reported disturbance event (22%), followed by wind (17%) and grazing (15%). Settlements is the least important disturbance events in terms of number of reported evidence (2%), but the average intensity (scale: 1 = very light to 4 = severe) is among the highest (2.6) together with LUC (2.8), as both imply complete removal or displacement of the natural forest. Pests has the lowest average intensity reported (2.0), with a maximum of 3 .
The reported disturbances are not evenly distributed. Evidence of wind damage is most frequently reported in west Yucatan Peninsula (WYP) and east Yucatan Peninsula (EYP) and to a lesser extent in south Yucatan Peninsula (SYP), whereas pests and diseases are apparent in all ecoregions with a relative low intensity . The human disturbances also show spatially heterogeneous patterns, with fire particularly evident in SYP, WYP and EYP, and less frequent in the Gulf of Mexico and eastern Chiapas (GM-EC) and Sierra Madre Foothills (SMF). Grazing is mostly observed in WYP and SMF, and to a lesser extent in SYP; LUC is notorious in GM-EC and SMF and sparsely distributed throughout SYP, EYP and WYP; forest management and roads are mainly observed in plots of EYP, whereas settlements are sparsely distributed throughout all ecoregions .
Particularly in the northern part of EYP and WYP the intensity of both human and natural disturbances are high, whereas the forests in the other regions are predominantly influenced by human disturbances .
▪ Spatial variation of biomass
The cluster and outlier analysis shows the existence of high biomass clusters (H-H) in large areas of SYP, WYP and EYP and a small area in GM-EC. Low biomass clusters (L-L) were located in WYP, various parts of GM-EC and small clusters in South Pacific Coast and West Pacific Coast (WPC). Both types of outliers (H-L and L-H) are present in SYP, WYP, EYP and GM-EC, whereas H-L outliers are mostly located in the low biomass clusters of WYP, EYP and GM-EC , . Almost 90% of the H-H and L-H plots are located in SYP and EYP, whereas 75% of the L-L and H-L plots are situated in WYP and GM-EC . Approximately 58% of all plots are clustered in any of the four groups, almost half of which in the two high biomass groups . The average biomass of the high biomass clusters is similar to the biomass in the H-L outlier groups for all plots, and for most of the ecoregions where both groups occur. Similarly, L-L plots had similar average biomass as the L-H outlier group . The highest biomass are found in H-H and H-L of GM-EC and H-H of WPC, and the lowest biomass in L-L of SYP, EYP and SMF. The plots outside the cluster and outlier groups have relatively low average biomass, varying between 70 in WYP and 86 Mg DM/ha in WPC , that is the lower part of the range of biomass between low and high biomass groups (22.2–33.5 for L-L and L-H plots to 140.9–283.4 for H-H and H-L plots, respectively; ). This is indicates that most of the forests outside the H-H clusters have relatively low biomass, as can be observed from the biomass map .
▪ Links between biomass & the presence/absence of human & natural disturbances
The multiple factor ANOVA analysis shows that the clustering of biomass in density groups and the presence/absence of human and natural disturbances together explain significantly the variation in biomass observed in all plots (p < 0.01; r2 = 0.8; ). However, analyzing the variation of biomass at the level of ecoregions, only in SYP, WYP, EYP and GM-EC the variation can be explained by the three independent variables, with presence/absence of natural disturbances considered and nonsignificant in the case of SYP and GM-EC .
▪ Links between biomass & the intensity of human disturbances
The ANOVA analysis, using the plots with only the specific disturbances recorded, shows that the variation in biomass observed can be explained by the varying intensity reported in the plots. Plots with reported evidence of fire, roads, LUC, grazing and settlements decrease in biomass with increasing intensity, whereas plots with forest management did not show this trend .
The multiple linear regression analysis between Sqrt(AGLB) as the dependent variable and all specific human disturbance events considered as independent variables indicate that all events are negatively correlated with biomass, except forest management that shows a positive correlation (p < 0.01). At the level of ecoregion, only for five ecoregions could an equation be developed with only some of the events significantly correlated . Fire, LUC and grazing are important in three ecoregions, whereas forest management is positively correlated in SYP and EYP, which coincides with the presence of H-H and L-H plots .
Applying the regression equations to all plots, the average Sqrt(AGLB) in plots with fire, LUC and grazing is around 20% lower than in plots without these disturbances, whereas Sqrt(AGLB) in plots with forest management is 12% higher than in plots without management . At the level of ecoregions it is noteworthy that in plots with grazing Sqrt(AGLB) is much lower in SYP (-45%), with LUC in South Pacific Coast (-40%) and with fire in WYP (-35%). Roads are only important using the data of all plots (-9%).
Discussion
▪ Spatial distribution of biomass
In general terms, the biomass in most of the plots are low, with 75% of the plots with less than 150 Mg DM/ha in AGLB. Furthermore, high biomass clusters are restricted to two major areas – southern Yucatan Peninsula and eastern Chiapas. The rest of the areas have much lower biomass with a number of low biomass clusters in various parts of southern Mexico, indicating the widespread, high degree of forest disturbance. However, within a number of these patches of high and low biomass clusters, we can observe outlier plots with low biomass in the high biomass clusters and low biomass in high biomass clusters. The high number of L-H plots (321 against 945 H-H plots) indicates the high level of fragmentation of the high biomass clusters, whereas the amount of biomass in high biomass outliers in low biomass clusters gives an indication of the original biomass before degradation, and can thus be used to estimate to what level biomass can be recovered with appropriate measures. Some authors suggest that part of the biomass pattern observed in the Yucatan Peninsula can be attributed to the rainfall gradient in the area. Becknell et al., for example, found a clear relation between annual rainfall and AGLB for the deciduous forests in Central America, including Mexico Citation[26]. This could partly explain the low biomass cluster in the northern part of the Yucatan Peninsula (WYP and part of EYP), where many plots are located in the dryer parts with rainfall around 800 mm/yr and the high biomass cluster in SYP and part of EYP, where annual rainfall increases to 1500 mm/yr. However, the relatively high number of high biomass outliers found in the northern part of the Peninsula show that high biomass can occur in these areas with relatively low rainfall, averaging up to 140 Mg DM/ha in H-L plots of WYP and 160 Mg DM/ha in EYP. This is also the case for the other ecoregions, such as the WPC, SMF and GM-EC, where H-L plots contained more than 200 Mg biomass opposed to around 25 Mg in the L-L clusters.
The relatively low biomass in the remaining plots that cannot be grouped in any of the clusters or outliers is also an indication that the MHTF forests can be considered to a large extent degraded. The biomass map, derived from the interpolation of the biomass data points, also shows that more than 70% of the area can be considered as degraded in terms of biomass. This is in agreement with the national LU map that considers around 70% of MHTF as secondary.
▪ Spatial variation in human & natural disturbances & their relation to biomass
The human and natural disturbances show a high spatial variation in presence and intensity. Of the human disturbances, fire was the most important reported disturbance variable in the number of plots reported with fire damage , with 24–35% less biomass in fire plots compared with nonfire plots in the three Peninsula ecoregions . Of the natural disturbances, wind damage was the most important variable, particularly in the eastern and northern part of the Yucatan Peninsula, since this area is frequently hit by hurricanes coming from the Caribbean. The combination of wind damage and subsequent sensitivity to intensive fires due to the accumulation of high amounts of woody debris is of particular concern for this region.
Other important disturbances, both in number of reported events and their relation to biomass, are LUC and grazing. In many cases these effects may be correlated, as grazing in MHTF forests is mostly limited to secondary vegetation, where more palatable species are available Citation[27]. The reported data confirm this, since in 45% of the plots where LUC is reported, evidence of grazing is shown. As such, the lower level of biomass in plots with grazing may at least partially attributed to LUC, in those regions where both are correlated to biomass, such as SYP and GM-EC.
Looking at the spatial distribution of the reported human and natural disturbances compared with the biomass map , the highest biomass seems to be located in those areas with few or no disturbances . Also, the linear correlation between the measured biomass and the human disturbances are significant for all cases taking into consideration all plots, but at the level of ecoregion, fewer variables are correlated with biomass. All variables are negatively correlated to biomass, indicating that biomass may have been affected by these events, although exceptions are found for those cases where activities may be focused on high biomass forests as, for example, in the case of forest management. The positive relationship between the biomass in plots and forest management in SYP and EYP coincides with the area where the H-H cluster is located. This is in accordance with studies in these regions that show that communities with forest management have lower rates of deforestation compared with areas without management Citation[28].
▪ REDD+ strategies
REDD+ activities include taking measures to decrease emissions by reducing deforestation or forest degradation, stock conservation, sustainable management of forests and stock enhancement. All of these require certain conditions of the forests, in order to be effective. Reducing deforestation, for example, is an activity particularly effective for countries with large areas of intact forests that are threatened to be removed in the short term. Stock conservation will be focused on countries with large areas of intact forests with little or no threats to be removed, at least in the short term. Both cases are not applicable to the MHTF forest of Mexico, as most of these forests are already in an advanced stage of degradation or fragmentation and the pressure on the remaining forests to continue the process of degradation are high.
Activities can be designed to decrease emissions due to forest degradation in the areas where forests still contain high levels of biomass, based on sustainable forest management that includes control of forest fires. But for most of the MHTF forests, the most promising strategy would be to develop activities directed towards increasing the current stocks of the highly degraded forest with the intention to direct these areas towards sustainably managed forest. The potential biomass increase is very high, taking into consideration the amount of biomass observed in the clusters, outlier groups and plots that are outside these groups. On average, at least 70% of the forest could enhance biomass with at least 100 Mg DM/ha. Good examples of interesting projects are being developed in various communities around the Calakmul Biosphere Reserve (Campeche) that are applying silvicultural treatments to improve and manage naturally regrowing secondary vegetation that can readily be converted into a promising REDD+ stock enhancement activity Citation[29].
Future perspective
Measuring forest degradation and related forest carbon stock changes is complicated and costly. Countries can measure current rates of degradation through field monitoring and/or remote sensing; however, most countries lack consistent historical field data on forest degradation Citation[11]. Therefore there is a need to incorporate historical information in the inventories that explain the current state of the forest plot. More precise data can be obtained in each plot in terms of historical dates of major disturbance events through interviews with the landowner that can then be tracked back with satellite imagery. In this way, a monitoring system can be developed that keeps track of the disturbance events that are corroborated with field data, through remote sensing and the dynamics of the vegetation. For each plot a disturbance trajectory can thus be obtained and connected to a biomass model, and together these can serve as a basis to develop bottom-up reference emission scenarios. Various explaining variables can be derived from forest inventory plots and related to data obtained from satellite imagery. Satellite imagery can estimate canopy cover and canopy height; thus, if correlations exist between these variables and AGLB, a good estimation of standing biomass can be derived for a whole area Citation[30]. These can then be incorporated into a time series monitoring system that keeps track of the disturbance events over time and combined with field plot information to relate these events to types of disturbances and subsequent biomass dynamics Citation[31–33]. A combination of free satellite monitoring systems, such as Landsat™, combined with infrequent aircraft-based LiDAR measurements, can provide a monitoring system of carbon stocks, losses and recovery in forests Citation[30], and related to ground-based data that register type and intensity of disturbance, disturbance history and other parameters that cannot be observed in satellite imagery or that are required to validate remotely sensed information. Focusing efforts to monitor carbon stock changes of those carbon pools that are subject to rapid changes due to disturbances (vulnerable carbon; Citation[34]), and on specific areas within the country where disturbances are concentrated, can make the monitoring more targeted and efficient Citation[11].
Table 1. Brief outline of human and natural disturbances.
Table 2. Number of plots used in the analysis with human disturbances, with natural disturbances, without disturbances and all plots in each ecoregion.
Table 3. Average biomass densities in high, low and outlier categories in all plots and in all ecoregions.
Table 4. Multiple variable ANOVA with biomass clusters, human and natural disturbance presence/absence as independent variables and a square root conversion of the aboveground biomass data as dependent variable.
Table 5. ANOVA and Tukey´s test for homogeneous groups of aboveground living biomass, according to the intensity of the specific disturbance.
Table 6. Statistically significant coefficients of the multiple linear regressions between a square root conversion of the aboveground biomass data and specific human disturbances for each ecoregion (p < 0.01).
Table 7. Level of biomass reduction in plots with human disturbances in percentage of a square root conversion of the aboveground biomass data of undisturbed plots, applying the regression equations of Table 6.
In the context of this paper, land-use change refers to converting forest to slash-and-burn agriculture.
Identifies statistically significant hotspots, cold spots and spatial outliers, calculating statistics of neighboring plots with similar or dissimilar high or low values.
Determines unmeasured cell values using a linearly weighted combination of a set of nearby measured sample points. The weight that is used in the interpolation is a decay function defined by the inverse distance. The method assumes that the variable being calculated decreases in influence from the sampled location.
Spatial distribution of biomass
▪ Biomass in tropical lowland forests of Mexico is generally low, with only a few clusters of high biomass forests in southeast Yucatan and east Chiapas. | |||||
▪ High biomass outliers can be used to estimate the stock enhancement potential of surrounding low biomass clusters. | |||||
▪ The number of low biomass outliers are an indication of the level of forest fragmentation in high biomass areas. |
Importance of human disturbances
▪ Fire, land-use change and grazing were the most important human disturbances. | |||||
▪ Land-use change and grazing are expected to be correlated disturbance events, as 45% of the land-use change plots show evidence of grazing. |
REDD+ strategies
▪ Stock enhancement is considered as the most important REDD+ option for the tropical lowland forests of Mexico, due to the current state of the forests. | |||||
▪ Sustainable forest management is an important option for southeast Yucatan Peninsula and east Chiapas, where high biomass forests are located. |
Future perspective
▪ A high priority is placed on developing monitoring strategies that combine remote sensing data with complementary field data collection to monitor impacts of human and natural disturbances on forest structure and biomass. |
Acknowledgements
The author would like to thank M Olguín-Alvarez for her help with quality control of the field data and transferring them to a GIS system. F Rojas-García helped compiling the allometric equation database, for which the author is grateful. Many thanks go to the Commisión Nacional Forestal (Jalisco, Mexico) for providing the author with access to the data of the National Forest and Soil Inventory, and to R Flores for explaining the structure of the data tables.
Financial & competing interests disclosure
The author received partial financial assistance from US Forest Service through the federal grant 12-IJ-11242306–054. The author has no other relevant affiliations or financial involvement with any organization or entity with a financial interest in or financial conflict with the subject matter or materials discussed in the manuscript apart from those disclosed.
No writing assistance was utilized in the production of this manuscript.
References
- Canadell JG, Raupach MR. Managing forests for climate change mitigation. Science320(5882),1456–1457 (2008).
- Kauffman JB, Hughes RF, Heider C. Carbon pool and biomass dynamics associated with deforestation, land use, and agricultural abandonment in the neotropics. Ecol. Appl.19(5),1211–1222 (2009).
- Kanninen M, Murdiyarso D, Seymour F, Angelsen A, Wunder S, German L. Do Trees Grow on Money? The Implications of Deforestation Research for Policies to Promote REDD. Center for International Forestry Research, Bogor, Indonesia, 61 (2007).
- Guild LS, Kauffman JB, Ellingson LJ et al. Dynamics associated with total aboveground biomass, C, nutrient pools and biomass burning of primary forest and pasture in Rondonia during SCAR-B. J. Geophys. Res.103(D24),32091–32100 (1998).
- Kauffman JB, Steele MD, Cummings DL, Jaramillo VJ. Biomass dynamics associated with deforestation, fire, and conversion to cattle pasture in a Mexican tropical dry forest. For. Ecol. Manage.176(1–3),1–12 (2003).
- Marin-Spiotta E, Ostertag R, Silver WL. Long-term patterns in tropical reforestation: plant community composition and aboveground biomass accumulation. Ecol. Appl.17(3),828–839 (2008).
- Lugo AE. Visible and invisible effects of hurricanes on forest ecosystems: an international review. Austral. Ecol.33(4),368–398 (2008).
- Asner GP. Painting the world REDD: addressing scientific barriers to monitoring emissions from tropical forests. Environ. Res. Lett.6,021002 (2011).
- Nepstad DC, Veríssimo A, Alencar A et al. Large-scale impoverishment of Amazonian forests by logging and fire. Nature398,505–508 (1999).
- Houghton RA. Aboveground forest biomass and the global carbon balance. Glob. Chang. Biol.11,945–958 (2005).
- Herold M, Román-Cuesta RM, Heymell V et al. A review of methods to measure and monitor historical carbon emissions from forest degradation. Unasylva238(62),16–24 (2011).
- Laurance WF, Delamonica P, Laurance SG, Vasconcelos H, Lovejoy TE. Rainforest fragmentation kills big trees. Nature404,836 (2000).
- Barlow J, Peres CA, Lagan BO, Haugaasen T. Large tree mortality and the decline of forest biomass following Amazonian wildfires. Ecol. Lett.6,6–8 (2003).
- Lugo AE. Stress and ecosystems. In: Energy and Environmental Stress in Aquatic Systems. Thorp JH, Gibbons JW (Eds). National Technical Information Services, Alexandria, VA, USA, 62–101 (1978).
- White PS. Pattern, process and natural disturbance in vegetation. Bot. Rev.45(3),229–299 (1979).
- Sousa WP. The role of disturbance in natural communities. Annu. Rev. Ecol. Syst.15,353–391 (1984).
- Pickett STA, White PS. The Ecology of Natural Disturbances and Patch Dynamics. Academic Press, Orlando, FL, USA, 472 (1985).
- Laurance WF, Curran TJ. Impacts of wind disturbance on fragmented tropical forests: a review and synthesis. Austral. Ecol.33(4),399–408 (2008).
- Orihuela-Belmonte DE, de Jong BHJ, Mendoza-Vega J et al. Carbon stocks and accumulation rates in tropical secondary forests at the scale of community, landscape and forest type. Agric. Ecosyst. Environ.171,72–84 (2013).
- Instituto de Estadística y Geografía. Carta de Uso de Suelo y Vegetación Series IV [Land Use and Vegetation Map, Series 4]. Direccción General de Geografía – Instituto Nacional de Estadística Geografía e Informática, Aguascalientes, Mexico (2012).
- Wiken E, Jiménez-Nava F, Griffith G. North American Terrestrial Ecoregions – Level III. Commission for Environmental Cooperation, Montreal, Canada (2011).
- Hughes RF, Kauffman JB, Jaramillo VJ. Biomass, carbon, and nutrient dynamics of secondary forests in a humid tropical region of Mexico. Ecology80(6),1892–1907 (1999).
- Cairns MA, Olmsted I, Granados J, Argaez J. Composition and aboveground tree biomass of a dry semi-evergreen forest on Mexico’s Yucatan Peninsula 2003. For. Ecol. Manage.186,125–132 (2003).
- Urquiza-Haas T, Dolman PM, Peres CA. Regional scale variation in forest structure and biomass in the Yucatan Peninsula, Mexico: effects of forest disturbance. For. Ecol. Manage.247,80–90 (2007).
- Anselin L. Local indicators of spatial association – LISA. Geogr. Anal.27(2),93–115 (1995).
- Becknell JM, Kissing Kucek L, Powers JS. Aboveground biomass in mature and secondary seasonally dry tropical forests: a literature review and global synthesis. For. Ecol. Manage.276,88–95 (2012).
- Farji-Brener AG. Why are leaf-cutting ants more common in early secondary forests than in old-growth tropical forests? An evaluation of the palatable forage hypothesis. Oikos92(1),169–177 (2002).
- Porter-Bolland L, Ellis EA, Guariguata MR, Ruiz-Mallén I, Negrete-Yankelevich S, Reyes-García V. Community managed forests and forest protected areas: an assessment of their conservation effectiveness across the tropics. For. Ecol. Manage.268,6–17 (2012).
- Venegas-Sandoval A. Efecto Del Manejo Forestal en el Contenido de Carbono en Vegetación y Suelo de Acahuales de Calakmul, México [Effect of Management on Carbon Content of Vegetation and Soil in Secondary Forests of Calakmul, Mexico] [MSc Thesis]. El Colegio de la frontera Sur, Campeche, Mexico (2013).
- Asner GP. Tropical forest carbon assessment: integrating satellite and airborne mapping approaches. Environ. Res. Lett.4(3),034009 (2009).
- Hayes DJ, Sader SA. Comparison of change detection techniques for monitoring tropical forest clearing and vegetation regrowth in a time series. Photogramm. Eng. Remote Sens.67(9),1067–1075 (2001).
- Yang X, Lo CP. Using a time series of satellite imagery to detect land use and land cover changes in the Atlanta, Georgia metropolitan area. Int. J. Remote Sens.23(9),1775–1798 (2002).
- Lu D, Mausel P, Brondízio E, Moran E. Change detection techniques. Int. J. Remote Sens.25(12),2365–2401 (2004).
- de Jong BHJ, Hellier A, Castillo-Santiago MA, Tipper R. Application of the ‘Climafor’ approach to estimate baseline carbon emissions of a forest conservation project in the Selva Lacandona, Chiapas, Mexico. Mitig. Adapt. Strat. Gl.10(2),265–278 (2005).