Abstract
Quantitating the copy number of demethylated CpG promoter sites of the CD3Z gene can be used to estimate the numbers and proportions of T cells in human blood and tissue. Quantitative methylation specific PCR (qPCR) is useful for studying T cells but requires extensive calibration and is imprecise at low copy numbers. Here we compared the performance of a new digital PCR platform (droplet digital PCR or ddPCR) to qPCR using bisulfite converted DNA from 157 blood specimens obtained from ambulatory care controls and patients with primary glioma. We compared both ddPCR and qPCR with conventional flow cytometry (FACS) evaluation of CD3 positive T cells. Repeated measures on the same blood sample revealed ddPCR to be less variable than qPCR. Both qPCR and ddPCR correlated significantly with FACS evaluation of peripheral blood CD3 counts and CD3/total leukocyte values. However, statistical measures of agreement showed that linear concordance was stronger for ddPCR than for qPCR and the absolute values were closer to FACS for ddPCR. Both qPCR and ddPCR could distinguish clinically significant differences in T cell proportions and performed similarly to FACS. Given the higher precision, greater accuracy, and technical simplicity of ddPCR, this approach appears to be a superior DNA methylation based method than conventional qPCR for the assessment of T cells.
Abbreviations:
ddPCR | = | droplet digital polymerase chain reaction |
DMR | = | differentially methylated regions |
qPCR | = | quantitative polymerase chain reaction |
FACS | = | fluorescence activated cell sorting |
MS | = | methylation specific |
CV | = | correlation coefficient |
Introduction
The DNA methylation status of specific CpG dinucleotides provide markers of cell differentiation in diverse types of mammalian cells,Citation1 including those of the haematopoietic system.Citation2,3 Our earlier experiments showed that cell lineage-specific differentially methylated regions (DMRs) distinguish normal human leukocyte subsets and can be used to detect and quantify these subsets in peripheral blood.Citation4-6 Methylation based detection of immune cells can be applied to many cohorts of archival blood samples that would otherwise be useless for immune cell enumeration by conventional FACS analyses. 2 types of assays characterize immune subsets using DNA methylation: one is based on single-gene DNA methylation events quantified by methylation specific (MS) real time PCR,Citation5,7 hereafter referred to as qPCR. A second assay uses off-the-shelf or custom DNA methylation microarrays and captures many cell type DMRs simultaneously.Citation6,Citation8-10 Both methods rely on the bisulfite conversion reaction that converts cytosine residues in DNA to thymidine in a subsequent PCR amplification step, except for those cytosines that are methylated. Methylated cytosine residues are resistant to the conversion, thus providing a biochemical distinction between methylated and non-methylated cytosine residues. In the most widely studied example of the single-gene approach, the copy number of demethylated FOXP3 gene estimates the frequency of human T regulatory cells in blood or tissues.Citation7,11 The advantage of single-gene based assays is their potential sensitivity compared with the current generation of array based methods. DMR targets in CpG sites within the promoter of the CD3Z gene estimate total T cells.Citation5 The CD3Z protein is a specific T cell lineage marker and associates with the T cell receptor and functions to generate activation signals in T lymphocytes. In T cells, the CD3Z gene promoter is demethylated, but this promoter is densely methylated in other immune cell subsets. Methylation specific primers and probes are then designed to hybridize only with the unmethylated version of the promoter and the copies of demethylated CD3Z are quantitated. Each cell contains 2 copies of the gene and, thus, 2 unmethylated copies of CD3Z correspond to a single T cell. We control for the total input of methylated and demethylated DNA by using a gene reference target that is insensitive to CpG methylation status (referred to as the C-less reaction).Citation37,38
An important application of this approach is in oncology patients who demonstrate T cell defects. It has also been recognized for many years that glioma patients, and particularly Stage IV glioblastoma (GBM) patients, show peripheral immune defects affecting T cells.Citation12,13 T cell counts in glioma patients are reduced and T-cell function is also suppressed, with impaired proliferation in response to IL2 and nonspecific mitogens.Citation14 A study of patients with high-grade gliomas (grades III and IV) treated with radiation and temozolomide showed that after 2 months of treatment about 40% of patients had CD4 T cell counts less than 200 cells/uL. Such low CD4 counts are associated with high risk for opportunistic infections; in contrast, levels of 500–1500 cells/uL are considered normal. These patients continued to have low T cell counts for the full year of follow-up and exhibited early mortality from tumor progression.Citation15 Other studies also have reported that low T cell counts were associated with poorer glioma survival times.Citation16,17 More generally, T cell lymphopenia has been shown to be a grave prognostic indicator in multiple types of cancers.Citation18-23
Recently, digital PCR platforms have been developed that offer advantages over real time qPCR including the capability to obtain absolute quantification without external references and to be highly robust to variations in PCR efficiency, thus improving assay precision.Citation25-27 Digital PCR is based on limiting dilution of a sample into a large number of separate PCR reactions. With sufficient dilutions, many reactions will not contain a template DNA and the number of positive reactions allows for template quantification using Poisson statistics without requiring a standard curve.Citation28 Investigators have compared the performance of digital and real time PCR for conventional DNA templates; however, there is little information on the suitability of digital PCR for methylation specific PCR that involves single strand amplification of bisulfite treated DNA.Citation29 Here, we compare ddPCR and real time qPCR in assessing the CD3Z pan T cell marker in whole blood from glioma patients and controls who we also studied using FACS analyses. Using FACS derived T cell counts as a gold standard we found that the ddPCR assay of CD3Z demethylation displays improved reproducibility and less bias in reflecting FACS measured T cells compared with real time qPCR.
Results and Discussion
Blood samples were collected from 38 glioma patients and 119 non-glioma control subjects, with T cell estimates determined using each of the 3 methods (Supplementary Table 1). As the aims of the study were to compare the performance of the 2 methylation assays directly to the FACS gold standard, all participants with non-missing data were included. Ten non-glioma controls and 2 case subjects reported a history of HIV infection. The resultant study group displayed a wide range of T cell numbers.
Serial dilution of purified bisulfite converted T cell DNA measured with ddPCR and real time qPCR revealed highly linear relationships between DNA input and cell copies as indicated by the numbers of demethylated CD3Z copies (Supplementary Fig. 1). We observed no positive droplets (total droplets counted = 120,000) in the 9 non template control wells. The false positive rate for wells using the CD3Z assay is extremely low and consistent with a background of 0.1 to 0.4 false positive events per non template control as reported using non-bisulfite treated DNA templates.Citation30-32 By contrast, T cell lymphopenia (<200 CD3/uL) is associated with approximately 400–700 positive droplets using our protocol; thus, the ddPCR provides ample sensitivity for applications in clinically relevant settings. The results of T cell proportions were highly correlated between the 2 assays (; R2 = 0.75). Repeated measures (N = 19) of CD3Z demethylation were performed on samples from a single individual. The coefficient of variations (%CV) for repeated measures was nearly 7-fold lower using ddPCR (3.5% CV) compared with real time qPCR (25% CV). Dramatic reductions in day-to-day reproducibility (by a factor of seven) were also reported in comparing ddPCR and qPCR for assay of serum microRNA quantitation.Citation33
Figure 1. Scatter plot with fitted linear regression line of T cell proportions measured by ddPCR (Y-axis) and qPCR (X-axis) in all samples.
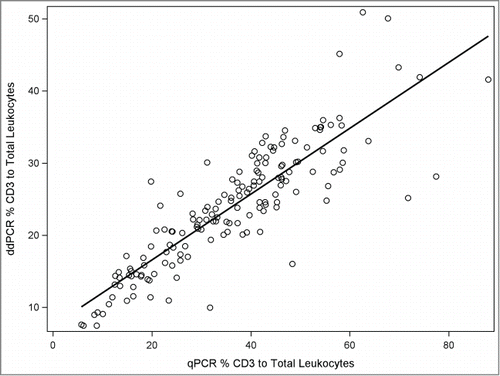
Ultimately, the validity of methylation assays of immune cells is determined by comparison with gold standard assays such as FACS. compares the mean T cell proportions determined by FACS, ddPCR, and qPCR. The methylation based assays revealed consistently higher means in the sample population, qPCR had 18% higher T cell/total leukocytes and ddPCR had 5% higher T cell/total leukocytes. To examine the nature of these differences we analyzed individual T cell measurements for ddPCR and qPCR compared with FACS results. shows the Bland-Altman plots depicting the methylation results (X-axis) and the difference between the methylation result and the gold standard FACS result (Y-axis). This analysis revealed that the systematically higher T cell values for methylation assays were consistent across the levels of T cell values for ddPCR (i.e., constant around 5%) but varied for qPCR with an apparent increasing divergence of methylation results compared with FACS at the higher values of T cells in the blood samples. Thus, the qPCR technique showed an apparent bias in its estimation of T cells that varied with T cell level, whereas ddPCR, while slightly higher, was consistent across T cell inputs. The validity of the assumption of a linear association between T cell proportions measured by FACS and those proportions measured by ddPCR or qPCR was assessed in plots as shown in . These analyses indicated that the coefficient of variation of the linear model of the FACS data used for predicting ddPCR (15.8%) was substantially less than the coefficient used to predict the qPCR data (25.2%). Thus, both repeated measure variation and correlation with gold standard flow analyses reveal higher precision for ddPCR than for qPCR. Further, we computed a concordance correlation coefficientCitation34 for agreement between each pair of methods (Supplementary Table 2). The results provide additional support of a stronger agreement between ddPCR and FACS (concordance estimate = 0.78, deviation from 45 degree line = 0.87 [no deviation = 1]) than for qPCR and FACS (concordance estimate = 0.36, deviation from 45 degree line = 0.43). A limitation of our studies is that we have only evaluated a single T cell marker in detail and hence we cannot determine whether the bias in qPCR would be seen with other gene targets. The performance of MS PCR is affected by the sequence context of CpG and non-CpG sites. Furthermore, many researchers use SYBR Green instead of a TaqMan chemistry to perform MS-PCR; we did not evaluate this chemistry but, given that the 2 methods are quantitatively equivalent, we believe the bias we found with TaqMan is likely similar to that which would be observed in applying the SYBR Green chemistry.
Figure 2. Jittered box and whisker plots for the % CD3 to total leukocyte values for flow cytometry (FACS), ddPCR and qPCR. Individual values are depicted by open circles, the bottom of the box is the 25th percentile, the line within the box is the 50th percentile (median) and the top of the box is the 75th percentile of the data. The top and bottom whiskers represent the value at 1.5 times the interquartile range (the distance between the 25th and 75th percentile). Outliers appear outside of the whiskers.
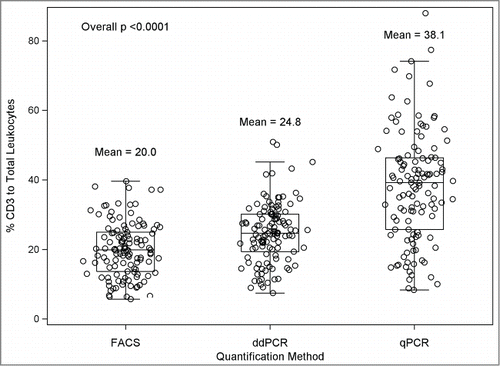
Figure 3. Bland Altman plots for visual assessment of the concordance of 2 methods. Difference in values (y-axis) measured by different methods among controls, e.g., ddPCR value minus flow cytometry (FACS) value, and qPCR value minus FACS value, are plotted against the gold standard FACS values (x-axis) as plots (A) and (B), respectively. Concordance between the values measured by each method is reflected by the scatter around the horizontal value of zero such that perfect concordance is depicted when all points lie along the zero horizontal.
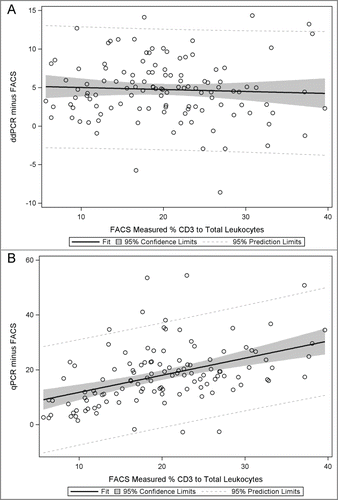
Figure 4. Plots of observed versus predicted values from linear regression of the ddPCR and qPCR measured ratio of T cells to Total leukocytes (x-axis) to the flow cytometry (FACS) measured ratio (y-axis) among controls. A tighter cluster of points around the line depicts good fit and linearity whereas systematic deviation of points suggests nonlinearity of the association e.g. as seen in (B) where data suggest larger errors are associated with larger predicted values. (A) Fitted regression equation for ddPCR (y) and FACS (x) for plot: Yhat = 5.26 + 0.97*X. (B) Fitted regression equation for qPCR (y) and FACS (x) for plot: Yhat = 5.53 + 1.62*X.
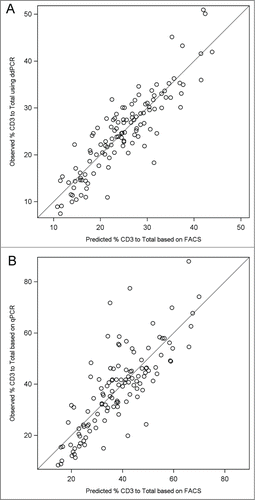
We conclude that while both ddPCR and qPCR platforms for DMR detection of T cells in human blood are highly correlated with each other and with FACS measured T cells, there are advantages in using the ddPCR approach. Digital PCR is technically simpler to perform than real time qPCR and does not require standard dilution curves to be estimated from cycle threshold data. In the current application to bisulfite treated DNA, as in previous studies using conventional DNA templates, we found clear evidence of increased precision of ddPCR in repeated measures and in the validation phase of the study that correlated DMR results with a gold standard. The bias in qPCR measurement also appeared to increase with increasing level of T cells, which presents a more serious concern in the practical clinical application of the method. We were unable to explain the bias as the qPCR reaction performed very well with high inputs using the control (C-less) primers and probes. We would like to note an earlier study using FOXP3 methylation qPCR that also reported a systematic overestimation of FACS-defined T regulatory cells.Citation35 The increase in CD3Z estimated T cells using ddPCR was consistently about 5% and this may reflect the fact that some Natural Killer (NK) populations may utilize CD3Z as a signaling component and display a demethylated promoter region.Citation4 The ddPCR bias, however, was consistent across individuals. In conclusion, DNA methylation analysis provides an alternative to automated cell counting and FACS analysis and can be used when intact cells are not available, as in the case of archival blood specimens. DNA methylation based assay of immune cells using ddPCR offers a new avenue for evaluating T cell profiles in clinical and epidemiologic research.
Methods
Blood samples for DMR and FACS analyses
Blood samples were obtained from participants of the San Francisco Adult Glioma StudyCitation36 who were recruited between 2010 and 2012. All glioma cases were adult (>18 years of age) patients of the UCSF Neuro-oncology Clinic with newly diagnosed histologically confirmed glioma (International Classification of Disease for Oncology, morphology codes 9380-9481). Participants without brain tumors (controls) were enrolled through the UCSF general medicine phlebotomy clinic. Whole blood was drawn in sodium EDTA vacutainer tubes. All participants provided written informed consent and in compliance with the study protocol approved by the UCSF Institutional Review Board.
FACS analyses
Flow cytometry was performed on whole blood within 24 hours of blood draw. The whole blood samples were directly stained with anti-human CD45 PerCP-Cy5.5 antibody (eBioscience, San Diego, CA, cat #45-0459-41), anti-human CD3 FITC antibody (eBioscience, cat #11-0038-41), and anti-human CD4 APC antibody (eBioscience, cat #17-0048-41) using a red blood cell lysis and fix, no wash protocol. Accucheck counting beads (Life Technologies, Carlsbad, CA, cat #PCB100) were also added for absolute quantification of cell types. Isotype controls were included in each run to determine gating and background. Individual compensation controls were also routinely run. Samples were run on the FACSCalibur flow cytometer using Cell-Quest Software (Becton Dickinson, Franklin Lakes, NJ). 10,000 events were collected on the lymphocyte gate that was set on the forward scatter versus side scatter dot plot. Analysis of flow data was done using Flowjo software (Treestar Inc, Ashland, OR).
DNA extraction and bisulfite conversion
Organic DNA extraction was performed on whole blood samples using the automated machine AutogenFlex Star (Autogen, Holliston, MA). DNA was quantified using Quant-iT Picogreen dsDNA Assay Kit (Life Technologies, Carlsbad, CA, cat #P11496). One microgram of DNA was then used for bisulfite conversion using the EZ-96 DNA Methylation Kit (Zymo Research Corporation, Irvine, CA, cat #5004). Bisulfite converted DNA was stored at −80°C.
CD3Z demethylation assay using droplet digital PCR (ddPCR)
Demethylated copies of CpG regions of CD3Z and total DNA (using C-Less primers) were quantified using the QX100 Droplet Digital PCR system (Bio-Rad, Hercules, CA). Master mixes for CD3Z and C-Less were prepared for each run. Master mixes included: Droplet PCR Supermix (Bio-Rad, cat #186-3024), CD3Z /C-Less primers and probe (Applied Biosystems, Foster City, CA), and EB buffer (Qiagen, Venlo, Netherlands, cat #19086). The CD3Z primers and probe sequences were: forward primer 5′- GGATGGTTGTGGTGAAAAGTG -3′, reverse primer 5′- GGATGGTTGTGGTGAAAAGTG -3′, and probe 6FAM-5′- CCAACCACCACTACCTCAA -3′-NFQ. The C-Less primers and probe sequences were: forward primer 5′- TTGTATGTATGTGAGTGTGGGAGAGA -3′, reverse primer 5′- TTTCTTCCACCCCTTCTCTTCC -3′, and probe 6FAM-5′- CTCCCCCTCTAACTCTAT -3′-NFQ (Weisenberger, Trinh et al. 2008, Campan, Weisenberger et al. 2009). CD3Z and C-Less master mixes were aliquoted out into a 96-well plate. CD3Z PCR experiments were performed in monoplex using 100ng of bisulfite converted sample DNA. Samples were then transferred one column at a time to an 8 channel Droplet Generator Cartridge (Bio-Rad, cat #186-3008). QX100 Droplet Generator Oil (Bio-Rad, cat #186-3005) was then added, and droplets were then generated using the QX100 Droplet Generator (Bio-Rad). Typically 10,000 to 14,000 droplets are generated. Droplets were then transferred from the cartridge to an Eppendorf 96-well PCR plate (Eppendorf, Hamburg, Germany, cat #951020362), foil heat sealed, and placed into a S1000 thermal cycler (Bio-Rad). The PCR cycling conditions were as follows: 95°C for 10 minutes, followed by 40 cycles of 94°C for 30 seconds and 61°C for 1 minute, and ending with 98°C for 10 minutes and kept at 4°C until detection. At detection the PCR plate was placed into the QX100 Droplet Reader (Bio-Rad) and droplets were subsequently read as being either positive or negative for fluorescence/amplification. The data was analyzed using QuantaSoft software (Bio-Rad).
CD3Z demethylation real time qPCR
For methylation specific qPCR analyses of CD3Z we used the absolute quantification method as previously described.Citation5 Copy number of the target locus in each sample was determined by reference to a 4-point standard curve, which was based on known copies of bisulfite converted template. Quantification of total bisulfite DNA copies for the standard and in each sample was determined by reference to the C-Less assay as described previously.Citation37,38 Purified CD3 T cells were obtained from healthy blood donors using antibody based negative selection and magnetic bead technology (Miltenyi, San Diego, CA).Citation5 Purity of the T cells was confirmed by FACS analysis. T cell DNA was bisulfite converted, quantified with C-less assay, and diluted to create a standard curve. Primers and fluorescent major grove binding probes were obtained from Applied Biosystems (Applied Biosystems, Foster City, CA), and prepared as 300 and 100 μM solutions, respectively, in H2O. PCR reactions used TaqMan Gold/Buffer A Pack from Applied Biosystems.
Statistical Analysis
Data were analyzed using SAS (SAS Institute Inc, Cary, NC) and the epitools package in R (R Foundation for Statistical Computing, Vienna, Austria). Non-parametric methods including Wilcoxon rank sum test and Spearman rank correlation were used in preliminary analyses to examine the association between T cell variables measured on a continuous or ordinal scale. Because standard statistical correlation tests often show strong correlation between different methods used to measure the same quantity, even when there are large systematic differences, we used additional statistical methods, Bland-Altman plots and the Lin concordance correlation, to examine agreement and concordance of the different methylation assay techniques. The Bland-Altman plot examines the differences between values measured from the different methods (Y-axis) plotted against the mean of these values if neither is considered a gold standard or against the measure that is considered the gold standard (X-axis). Here, we plotted the difference between FACS values (gold standard) and either ddPCR or qPCR values against FACS. In these plots, concordance between the measures is reflected by the scatter around the horizontal value of zero such that perfect concordance is depicted when all points lie along the zero horizontal. The Bland-Altman approach also allows for visual assessment of systematic biases, i.e., patterns of displacement above and below the horizontal that can be further examined in regression analyses. The second statistical method, the Lin concordance correlation statistic,Citation34 combines measures of both precision and accuracy to determine how far the observed data deviate from the line of perfect concordance (that is, the line at 45 degrees on a square scatter plot i.e. a slope of 1.0). Lin's coefficient increases in value as a function of the nearness of the data to the line of perfect concordance (the accuracy of the data) and of the tightness of the data about the linear best fit (the precision). Correlational analyses were followed up by simple and multivariable linear regression analyses with graphical examination of the validity of the linearity assumption.
Disclosure of Potential Conflicts of Interest
There were no potential conflicts of interest to disclose.
967589_Supplementary_Materials.zip
Download Zip (107.5 KB)Funding
This work was supported by the National Institutes of Health (grant numbers R50CA097257, R01CA52689, R01CA126831 and R01CA139020), as well as the National Center for Research Resources and the National Center for Advancing Translational Science, National Institues of Health through UCSF-CTSI (grant number UL1 RR024131), and the National Brain Tumor Foundation, the UCSF Lewis Chair in Brain Tumor Research, the UCSF Robert Magnin Newman chair in Neuro-Oncology, and by donations from families and friends of John Berardi, Helen Glaser, Elvera Olsen, Raymond E. Cooper, and William Martinusen; and a gift from the Johnson and Johnson Company. Its contents are solely the responsibility of the authors and do not necessarily represent the official views of the NIH.
Supplemental Material
Supplemental data for this article can be accessed on the publisher's website.
References
- Khavari DA, Sen GL, Rinn JL. DNA methylation and epigenetic control of cellular differentiation. Cell Cycle 2010; 9:3880-3; PMID:20890116; http://dx.doi.org/10.4161/cc.9.19.13385
- Bocker MT, Hellwig I, Breiling A, Eckstein V, Ho AD, Lyko F. Genome-wide promoter DNA methylation dynamics of human hematopoietic progenitor cells during differentiation and aging. Blood 2011; 117:e182-9; PMID:21427290; http://dx.doi.org/10.1182/blood-2011-01-331926
- Christensen BC, Houseman EA, Marsit CJ, Zheng S, Wrensch MR, Wiemels JL, Nelson HH, Karagas MR, Padbury JF, Bueno R, et al. Aging and environmental exposures alter tissue-specific DNA methylation dependent upon CpG island context. PLoS Genet 2009; 5:e1000602; PMID:19680444; http://dx.doi.org/10.1371/journal.pgen.1000602
- Accomando WP, Wiencke JK, Houseman EA, Butler RA, Zheng S, Nelson HH, Kelsey KT. Decreased NK cells in patients with head and neck cancer determined in archival DNA. Clin Cancer Res 2012; 18:6147-54; PMID:23014525; http://dx.doi.org/10.1158/1078-0432.CCR-12-1008
- Wiencke JK, Accomando WP, Zheng S, Patoka J, Dou X, Phillips JJ, Hsuang G, Christensen BC, Houseman EA, Koestler DC, et al. Epigenetic biomarkers of T-cells in human glioma. Epigenetics 2012; 7:1391-402; PMID:23108258; http://dx.doi.org/10.4161/epi.22675
- Accomando WP, Wiencke JK, Houseman EA, Nelson HH, Kelsey KT. Quantitative reconstruction of leukocyte subsets using DNA methylation. Genome Biol 2014; 15:R50; PMID:24598480; http://dx.doi.org/10.1186/gb-2014-15-3-r50
- Wieczorek G, Asemissen A, Model F, Turbachova I, Floess S, Liebenberg V, Baron U, Stauch D, Kotsch K, Pratschke J, et al. Quantitative DNA methylation analysis of FOXP3 as a new method for counting regulatory T cells in peripheral blood and solid tissue. Cancer Res 2009; 69:599-608; PMID:19147574; http://dx.doi.org/10.1158/0008-5472.CAN-08-2361
- Houseman EA, Accomando WP, Koestler DC, Christensen BC, Marsit CJ, Nelson HH, Wiencke JK, Kelsey KT. DNA methylation arrays as surrogate measures of cell mixture distribution. BMC Bioinformatics 2012; 13:86; PMID:22568884; http://dx.doi.org/10.1186/1471-2105-13-86
- Koestler DC, Marsit CJ, Christensen BC, Accomando W, Langevin SM, Houseman EA, Nelson HH, Karagas MR, Wiencke JK, Kelsey KT. Peripheral blood immune cell methylation profiles are associated with nonhematopoietic cancers. Cancer Epidemiol, biomarkers & prevention 2012; 21:1293-302.; http://dx.doi.org/10.1158/1055-9965.EPI-12-0361
- Langevin SM, Koestler DC, Christensen BC, Butler RA, Wiencke JK, Nelson HH, Houseman EA, Marsit CJ, Kelsey KT. Peripheral blood DNA methylation profiles are indicative of head and neck squamous cell carcinoma: an epigenome-wide association study. Epigenetics 2012; 7:291-9; PMID:22430805; http://dx.doi.org/10.4161/epi.7.3.19134
- Baron U, Floess S, Wieczorek G, Baumann K, Grutzkau A, Dong J, Thiel A, Boeld TJ, Hoffmann P, Edinger M, et al. DNA demethylation in the human FOXP3 locus discriminates regulatory T cells from activated FOXP3(+) conventional T cells. Eur J Immunol 2007; 37:2378-89; PMID:17694575; http://dx.doi.org/10.1002/eji.200737594
- Parney IF. Basic concepts in glioma immunology. Adv Exp Med Biol 2012; 746:42-52; PMID:22639158; http://dx.doi.org/10.1007/978-1-4614-3146-6_4
- Rolle CE, Sengupta S, Lesniak MS. Mechanisms of immune evasion by gliomas. Adv Exp Med Biol 2012; 746:53-76; PMID:22639159; http://dx.doi.org/10.1007/978-1-4614-3146-6_5
- Waziri A. Glioblastoma-derived mechanisms of systemic immunosuppression. Neurosurg Clin N Am 2010; 21:31-42; PMID:19944964; http://dx.doi.org/10.1016/j.nec.2009.08.005
- Grossman SA, Ye X, Lesser G, Sloan A, Carraway H, Desideri S, Piantadosi S, Consortium NC. Immunosuppression in patients with high-grade gliomas treated with radiation and temozolomide. Clin Cancer Res 2011; 17:5473-80; PMID:21737504; http://dx.doi.org/10.1158/1078-0432.CCR-11-0774
- Gustafson MP LY, LaPlant B, Liwski CJ, Maas ML, League SC, et al. . Immun monitoring using the predictive power of immune profiles. J ImmunoTher Cancer 2013; 1:11; PMID:24829748; http://dx.doi.org/10.1186/2051-1426-1-7
- Gousias K, von Ruecker A, Voulgari P, Simon M. Phenotypical analysis, relation to malignancy and prognostic relevance of ICOS+T regulatory and dendritic cells in patients with gliomas. J Neuroimmunol 2013; 264:84-90; PMID:24071056; http://dx.doi.org/10.1016/j.jneuroim.2013.09.001
- Tredan O, Manuel M, Clapisson G, Bachelot T, Chabaud S, Bardin-dit-Courageot C, Rigal C, Biota C, Bajard A, Pasqual N, et al. Patients with metastatic breast cancer leading to CD4+ T cell lymphopaenia have poor outcome. Eur J Cancer 2013; 49:1673-82; PMID:23265706; http://dx.doi.org/10.1016/j.ejca.2012.11.028
- Ray-Coquard I, Cropet C, Van Glabbeke M, Sebban C, Le Cesne A, Judson I, Tredan O, Verweij J, Biron P, Labidi I, et al. Lymphopenia as a prognostic factor for overall survival in advanced carcinomas, sarcomas, and lymphomas. Cancer Res 2009; 69:5383-91; PMID:19549917; http://dx.doi.org/10.1158/0008-5472.CAN-08-3845
- Peron J, Cropet C, Tredan O, Bachelot T, Ray-Coquard I, Clapisson G, Chabaud S, Philip I, Borg C, Cassier P, et al. CD4 lymphopenia to identify end-of-life metastatic cancer patients. Eur J Cancer 2013; 49:1080-9; PMID:23246297; http://dx.doi.org/10.1016/j.ejca.2012.11.003
- Moore C, Eslin D, Levy A, Roberson J, Giusti V, Sutphin R. Prognostic significance of early lymphocyte recovery in pediatric osteosarcoma. Pediatric blood & cancer 2010; 55:1096-102; PMID:20734401; http://dx.doi.org/10.1002/pbc.22673
- Ceze N, Thibault G, Goujon G, Viguier J, Watier H, Dorval E, Lecomte T. Pre-treatment lymphopenia as a prognostic biomarker in colorectal cancer patients receiving chemotherapy. Cancer Chemother Pharmacol 2011; 68:1305-13; PMID:21448592; http://dx.doi.org/10.1007/s00280-011-1610-3
- Borg C, Ray-Coquard I, Philip I, Clapisson G, Bendriss-Vermare N, Menetrier-Caux C, Sebban C, Biron P, Blay JY. CD4 lymphopenia as a risk factor for febrile neutropenia and early death after cytotoxic chemotherapy in adult patients with cancer. Cancer 2004; 101:2675-80; PMID:15503313; http://dx.doi.org/10.1002/cncr.20688
- Yovino S, Grossman SA. Severity, etiology and possible consequences of treatment-related lymphopenia in patients with newly diagnosed high-grade gliomas. CNS Oncol 2012; 1:149-54; PMID:23828734; http://dx.doi.org/10.2217/cns.12.14
- Sykes PJ, Neoh SH, Brisco MJ, Hughes E, Condon J, Morley AA. Quantitation of targets for PCR by use of limiting dilution. Biotechniques 1992; 13:444-9; PMID:1389177
- Bustin SA, Nolan T. Pitfalls of quantitative real-time reverse-transcription polymerase chain reaction. J Biomol Tech 2004; 15:155-66; PMID:15331581
- Hayden RT, Gu Z, Ingersoll J, Abdul-Ali D, Shi L, Pounds S, Caliendo AM. Comparison of droplet digital PCR to real-time PCR for quantitative detection of cytomegalovirus. J Clin Microbiol 2013; 51:540-6; PMID:23224089; http://dx.doi.org/10.1128/JCM.02620-12
- Hindson BJ, Ness KD, Masquelier DA, Belgrader P, Heredia NJ, Makarewicz AJ, Bright IJ, Lucero MY, Hiddessen AL, Legler TC, et al. High-throughput droplet digital PCR system for absolute quantitation of DNA copy number. Anal Chem 2011; 83:8604-10; PMID:22035192; http://dx.doi.org/10.1021/ac202028g
- Clark SJ, Harrison J, Paul CL, Frommer M. High sensitivity mapping of methylated cytosines. Nucleic Acids Res 1994; 22:2990-7; PMID:8065911; http://dx.doi.org/10.1093/nar/22.15.2990
- Pinheiro LB, Coleman VA, Hindson CM, Herrmann J, Hindson BJ, Bhat S, Emslie KR. Evaluation of a droplet digital polymerase chain reaction format for DNA copy number quantification. Anal Chem 2012; 84:1003-11; PMID:22122760; http://dx.doi.org/10.1021/ac202578x
- Strain MC, Lada SM, Luong T, Rought SE, Gianella S, Terry VH, Spina CA, Woelk CH, Richman DD. Highly precise measurement of HIV DNA by droplet digital PCR. PLoS One 2013; 8:e55943; PMID:23573183; http://dx.doi.org/10.1371/journal.pone.0055943
- Kiselinova M, Pasternak AO, De Spiegelaere W, Vogelaers D, Berkhout B, Vandekerckhove L. Comparison of Droplet Digital PCR and Seminested Real-Time PCR for Quantification of Cell-Associated HIV-1 RNA. PLoS One 2014; 9:e85999; PMID:24465831; http://dx.doi.org/10.1371/journal.pone.0085999
- Hindson CM, Chevillet JR, Briggs HA, Gallichotte EN, Ruf IK, Hindson BJ, Vessella RL, Tewari M. Absolute quantification by droplet digital PCR vs. analog real-time PCR. Nat Methods 2013; 10:1003-5; PMID:23995387; http://dx.doi.org/10.1038/nmeth.2633
- Lin LI. A concordance correlation coefficient to evaluate reproducibility. Biometrics 1989; 45:255-68; PMID:2720055; http://dx.doi.org/10.2307/2532051
- Tatura R, Zeschnigk M, Adamzik M, Probst-Kepper M, Buer J, Kehrmann J. Quantification of regulatory T cells in septic patients by real-time PCR-based methylation assay and flow cytometry. PLoS One 2012; 7:e49962; PMID:23209626; http://dx.doi.org/10.1371/journal.pone.0049962
- Wrensch M, Jenkins RB, Chang JS, Yeh RF, Xiao Y, Decker PA, Ballman KV, Berger M, Buckner JC, Chang S, et al. Variants in the CDKN2B and RTEL1 regions are associated with high-grade glioma susceptibility. Nature Genet 2009; 41:905-8; PMID:19578366; http://dx.doi.org/10.1038/ng.408
- Weisenberger DJ, Trinh BN, Campan M, Sharma S, Long TI, Ananthnarayan S, Liang G, Esteva FJ, Hortobagyi GN, McCormick F, et al. DNA methylation analysis by digital bisulfite genomic sequencing and digital MethyLight. Nucleic Acids Res 2008; 36:4689-98; PMID:18628296; http://dx.doi.org/10.1093/nar/gkn455
- Campan M, Weisenberger DJ, Trinh B, Laird PW. MethyLight. Methods Mol Biol 2009; 507:325-37; PMID:18987824; http://dx.doi.org/10.1007/978-1-59745-522-0_23