Abstract
DNA methylation data assayed using pyrosequencing techniques are increasingly being used in human cohort studies to investigate associations between epigenetic modifications at candidate genes and exposures to environmental toxicants and to examine environmentally-induced epigenetic alterations as a mechanism underlying observed toxicant-health outcome associations. For instance, in utero lead (Pb) exposure is a neurodevelopmental toxicant of global concern that has also been linked to altered growth in human epidemiological cohorts; a potential mechanism of this association is through alteration of DNA methylation (e.g., at growth-related genes). However, because the associations between toxicants and DNA methylation might be weak, using appropriate quality control and statistical methods is important to increase reliability and power of such studies. Using a simulation study, we compared potential approaches to estimate toxicant-DNA methylation associations that varied by how methylation data were analyzed (repeated measures vs. averaging all CpG sites) and by method to adjust for batch effects (batch controls vs. random effects). We demonstrate that correcting for batch effects using plate controls yields unbiased associations, and that explicitly modeling the CpG site-specific variances and correlations among CpG sites increases statistical power. Using the recommended approaches, we examined the association between DNA methylation (in LINE-1 and growth related genes IGF2, H19 and HSD11B2) and 3 biomarkers of Pb exposure (Pb concentrations in umbilical cord blood, maternal tibia, and maternal patella), among mother-infant pairs of the Early Life Exposures in Mexico to Environmental Toxicants (ELEMENT) cohort (n = 247). Those with 10 μg/g higher patella Pb had, on average, 0.61% higher IGF2 methylation (P = 0.05). Sex-specific trends between Pb and DNA methylation (P < 0.1) were observed among girls including a 0.23% increase in HSD11B2 methylation with 10 μg/g higher patella Pb.
Abbreviations
ANOVA | = | analysis of variance |
DMR | = | differentially methylated region |
ELEMENT | = | early life exposures in Mexico to environmental toxicants |
GEE | = | generalized estimating equation |
GLM | = | general linear model |
H19 | = | H19, imprinted maternally expressed transcript (non-protein coding) |
HSD11B2 | = | hydroxysteroid (11-β) dehydrogenase 2 |
IGF2 | = | insulin-like growth factor 2 |
K-XRF | = | K X-ray fluorescence |
LINE-1 | = | long interspersed element-1 |
OLS | = | ordinary linear regression |
Pb | = | lead |
PCR | = | polymerase chain reaction |
Introduction
Environmentally-induced epigenetic alterations at susceptible life-stages, such as in utero development, may persist and impact disease risk later in life. Epigenetic changes have been associated with early-life exposures to chemicals including bisphenol A, genistein, persistent organic pollutants, arsenic, maternal smoking, and cadmium in miceCitation1-4 and in human birth cohorts.Citation5-10 Lead (Pb) exposure is of global public health concern, and recent studies suggest that Pb changes DNA methylation patterns among rodents following perinatal exposureCitation11,12 and is associated with differences in DNA methylation in humans.Citation13-17 However, the impact of in utero Pb exposure on the epigenome of neonates is understudied, and its impact on specific genes related to health outcomes associated with Pb exposure remains unknown.
DNA methylation is the most widely studied epigenetic modification in human cohorts due in part to its implications for gene regulation and developmentCitation18,19 and the multitude of technologies designed to examine DNA methylation. Epigenome-wide technologies interrogate methylation at thousands to millions of CpG sites,Citation20 and quantitative and semi-quantitative technologies such as pyrosequencing,Citation21 Sequenom EpiTYPER,Citation22 and methylation-specific PCR Citation23 interrogate methylation at specific loci. While epigenome-wide platforms have many advantages, candidate loci approaches are economical and particularly beneficial for studies with hypothesized genes of interest or for validation purposes. As such, candidate loci approaches are well suited for human cohort studies that investigate specific health outcomes, and are preferable for large numbers of samples due to lower cost.
Technologies for epigenome-wide and candidate approaches differ not only in terms of study questions (discovery vs. testing of specific pathways) and cost, but also in data quality management. While statistical and bioinformatics pipelines for managing data from epigenome-wide technologies are constantly being evaluated, quality control practices for candidate loci approaches have been much less systematic. For example, data analysis tools and methods have been developed to address experimental batch effect, biases in array probe design, and dye bias in epigenome-wide data.Citation24-27 Normalization to internal controls and correction for batch effect are also standard to the data analysis pipeline for molecular biology methods including Real-Time quantitative PCR.Citation28,29 However, for candidate loci methylation technologies, such data quality control methods are rarely discussed. While in some instances, batch effects are considered and use of rigorous quality control is reported,Citation30 often the data are used as is and quality control consists of 2 known methylated controls (ambiguously called ‘low’ and ‘high’) on each experimental plate.
In environmental epigenetics studies involving humans, the biological significance of results is complicated by limitations including source tissue available for DNA, timing of sample collection for DNA extraction and exposure assessment, and small effect sizes observed between exposures and epigenetic modifications. While the field works to address these issues, there is also a need to improve and standardize quality control and statistical procedures for handling candidate gene methylation data. The reliability of the data and the ability to observe associations with small effect sizes can be improved by implementing appropriate quality control methods and by selecting statistical methods that will maximize statistical power.
Using data from the Early Life Exposures in Mexico to Environmental Toxicants (ELEMENT) birth cohort, this article pursues 2 goals. First, we compared approaches for quality control and data analysis relevant to environmental epigenetic studies utilizing quantitative candidate loci methylation technologies such as pyrosequencing. Models were compared using simulated data that has similar characteristics to the data observed in the ELEMENT cohort. Since data are simulated according to a known toxicant-DNA methylation association, analysis approaches can be objectively evaluated. In particular, we discuss the need for batch effect adjustments and modeling CpG site variance and dependence when studying the relationship between environmental factors and DNA methylation. Second, we evaluate the influence of in utero Pb exposure (measured via 3 biomarkers) on neonate DNA methylation (via bisulfite sequencing candidate regions in umbilical cord blood leukocyte DNA with the pyrosequencing platform). In this cohort, we found modest evidence for associations, often sex-and CpG site-specific, between in utero leukocyte DNA methylation at long interspersed elements 1 (LINE-1), as well as growth-related genes IGF2 and HSD11B2.
Results
We first give descriptive analyses of the ELEMENT study data that motivated our primary objective, followed by the results of the simulation studies that demonstrate best practices for analyzing this type of data, and finally show results of the Pb-methylation associations in the ELEMENT study.
Descriptive statistics of ELEMENT study data
Characteristics of the study population (n = 247 infant-mother pairs) can be found in . The majority of infants were full term (96.3%, gestational age ≥37 weeks) and male (58.1%). Few mothers smoked as assessed immediately following pregnancy (3.6% at one month post-partum). All subjects have data from at least one Pb biomarker and methylation from one genic region. 243 participants have methylation data for all genic regions, and 202 have Pb measures in all 3 exposure biomarkers.
Table 1. Characteristics and Pb biomarker levels of study population.
Lead exposure biomarkers reveal a wide range in cord blood Pb (1.2-29.9 μg/dL), maternal mid-tibia shaft Pb (−20.8-51.0 μg/g of bone), and maternal patella Pb (−29.9-77.7 μg/g). Negative point estimates for bone Pb can result when the true value is close to zero due to normalization to bone density. Negative values passing other quality control checks are retained here as this has been shown to be a better use of this type of data in epidemiological studies.Citation31,32 Tibia and patella Pb levels quantified at one month postpartum (Pearson r = 0.26, P < 0.001) and patella and log-transformed cord blood Pb (r = 0.19, P = 0.006) are significantly correlated while cord blood Pb and tibia Pb do not correlate strongly (r = 0.11, P = 0.1).
shows CpG site-specific and average percent methylation across sites of repetitive elements (LINE-1) and 3 candidate genes quantified via pyrosequencing using bisulfite converted DNA from umbilical cord blood leukocytes. Means and between-subject variance in percent methylation differed across sites from the same genic region. Supplemental Table S1 provides correlations of methylation among CpG sites for LINE-1 and 3 genes. While some pairs of sites are highly correlated with respect to their percent methylation, others are not, and yet others exhibit negative correlations.
Table 2. Percent of methylated cells at four candidate regions. Site-specific methylation and average of 4 CpG sites for repetitive elements, LINE-1, 4 sites within H19 and HSD11B2, and 3 sites for IGF2 are reported. Standardized values were adjusted for batch. LINE-1 was adjusted for batch by subtracting the 0% control value for each CpG site from the experimental plate each sample was run on. IGF2, H19, and HSD11B2 values were adjusted to a standard curve of control DNA (ranging between 0 and 100%) run for each CpG site on the corresponding experimental plate for each sample.
ANOVA revealed batch effects in both DNA methylation and lead biomarkers. Significantly different average methylation levels by experimental plate for all 4 candidate regions were found (Table S2). IGF2 batches produced the largest plate-to-plate methylation difference (8.8% difference between plates with highest and lowest averages). Tibia Pb and patella Pb levels also differed when comparing groups of subjects in each pyrosequencing batch (Table S2) with a difference as large as 9.93 μg/g average patella Pb observed between the subjects on 2 LINE-1 plates. Although differences in lead biomarkers were not related to the pyrosequencing procedure, not accounting for these differences could lead to inflated Type I error, thus complicated data analysis.
Comparisons of modeling approaches and batch adjustment
Statistical model comparisons
Using a simulation study, we compare ordinary least squares (OLS) regression (where the average among CpG sites is taken to obtain a single outcome measure per individual) and 2 marginal models for repeated measures, the General Linear Model (GLM) and generalized estimating equations (GEE) (where the actual values at each CpG site are treated as a multivariate outcome) with regard to their performance (bias and power) when estimating the association between a toxicant and DNA methylation.Citation33 Repeated measures models have utility in DNA methylation studies because, in addition to estimating the toxicant-DNA methylation relationship averaged across sites, they can also be used to rigorously test whether the association varies across CpG sites, and may have higher power than OLS.
Figure S1 compares several approaches to modeling DNA methylation data in the absence of batch effects using simulated data. While all approaches yield consistent estimates of the toxicant-DNA methylation association (not shown), the General Linear Model (GLM) for repeated measures that explicitly models both the site-specific variances and an unstructured correlation matrix among sites yields higher power to detect associations when data have differences in variances across sites similar to those observed in the ELEMENT data. Differences in power are more pronounced for HSD11B2 () compared to IGF2 () due to more pronounced differences in the variances (Table 2) and pairwise correlations (Table S1) among sites. In the absence of differences in variances across sites, the GLM does not lose power compared to other approaches (not shown). Since differences in variance across sites are common, and pairwise correlations among sites do not necessarily follow a particular pattern, the GLM is preferred over GEE, and much more so than OLS.
Figure 1. Simulation study findings. Bias in estimated coefficients and power to detect significant toxicant-DNA methylation associations from 5 approaches (see ), when data are generated to follow a similar structure to IGF (A and B) and HSD11B2 (C and D) methylation in the ELEMENT study (see Table S3 for simulation settings). For the batch-adjustment method using control samples, the scenario with standard curves having R2 = 0.99 (as we had in the ELEMENT study) is shown here. Bias and power are calculated from the average of 1000 data sets, each with 220 subjects.
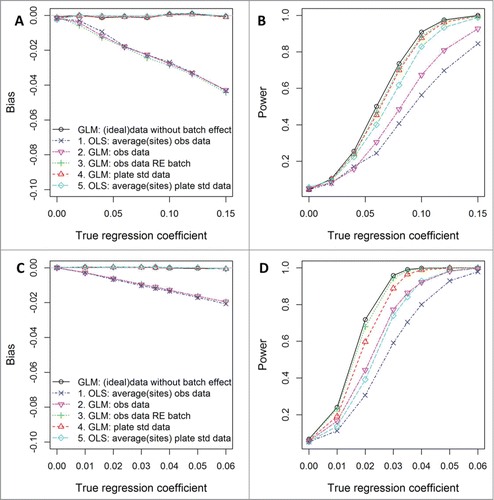
Batch effect adjustment methods
Two methods to control for batch effect are compared to no batch correction. One approach is to add a random intercept for each experimental plate to the above described models. Another approach is to standardize the data using plate- and site-specific standardization curves constructed using methylation data from control samples with known methylation values. shows the bias and power that can be expected using these methods for batch correction (numbered according to the models described in ); results when using the GLM approach in the ideal case of no batch effects and when using OLS are included as reference. Figure 1 shows that although both approaches have similar power, the approach using only random effects for plate yields biased effect estimates. The extent of the bias when the raw data are used depends on the extent to which the plate- and site-specific standardization curves deviate from the 45 degree line: if the standard reference curves are a straight line with slope other than one, biased associations will be obtained when using raw data.
Table 3. Statistical models tested. Models used to examine associations between Pb biomarker levels and DNA methylation at LINE-1, IGF2, H19, or HSD11B2. Model #4 was considered the best approach though additional modeling types that are sometimes used with candidate gene methylation data were run for comparison purposes.
Using standard curves to adjust for batch effects will consistently give unbiased associations. However, this approach may suffer loss of power depending on the fit of the standard curves (Fig. S2). If the standard curves fit well, then the standardization process will lead to estimating unbiased associations without loss of power. When appropriate, including a random intercept for batch to Model 4 may help gain power back, while keeping associations unbiased (Fig. S2, Model 4RE).
Associations between in utero lead exposure and DNA methylation in the ELEMENT study
Primary findings
and S4 show the estimated associations between 3 lead exposure biomarkers and DNA methylation using the GLM for repeated measures using plate- and site- specific standardized methylation measures (i.e., model #4 in ) adjusting for gestational age, sex, and maternal folate intake. We found the patella lead biomarker was associated (P = 0.05) with IGF2 hypermethylation (0.6% methylation per 10 μg/g patella Pb) and marginally (P = 0.06) associated with hypermethylation of the HSD11B2 promoter (0.1% methylation per 10 μg/g patella Pb).
Table 4. IGF2 and HSD11B2 models. Estimates and p-values for the regression of methylation at IGF2 or HSD11B2 on each Pb biomarker are noted for a series of models described in . All models adjusted for sex, gestational age, and maternal folate intake, and models 2–4 also adjust for CpG site. Models included all subjects with available data (n = 223, 237, 221 for IGF2 models with umbilical cord blood, tibia, or patella Pb, respectively; n = 227, 241, 224 for HSD11B2 models with umbilical cord blood, tibia, or patella Pb, respectively).
Site-specific effects
Differences in CpG site-specific relationships with Pb were tested with a type III test of fixed effects for the interaction between Pb and CpG site in a given genic region. Results revealed several significant interactions between Pb and CpG site: IGF2 and tibia Pb, LINE-1 and patella Pb, H19 and patella Pb. Only the interaction between patella Pb and LINE-1 sites remained significant following Bonferonni correction for multiple testing (P ≤ 0.004, Table S5). LINE-1 CpG site #2, but not other sites, exhibits hypermethylation with higher patella Pb levels, a relationship also apparent when separate linear regression models are run for each CpG site (Table S5).
Sex-specific effects
To explore sex-specific relationships, an interaction term was added between Pb and sex in Model #4. Results are reported for models with a Pb-methylation association with P < 0.1 in at least one sex following stratification (Supplemental Table S6). Three suggestive relationships between Pb biomarker levels and methylation were observed solely among females. For example, a natural-log unit higher cord blood Pb among females is associated with 3% lower methylation in IGF2. This decrease (P = 0.04) would not withstand correction for multiple hypothesis testing and is not observed among the males (). HSD11B2 hypermethylation was noted with higher patella Pb levels among both males and females, though effect size was greater and only marginally significant among females (, and S6).
Figure 2. Umbilical cord blood Pb and IGF2 methylation stratified by sex. Percent methylation in umbilical cord blood leukocyte DNA of the imprinted gene, IGF2, decreased with cord blood Pb solely in girls. After adjusting for gestational age and maternal folate intake, one natural-log unit increase in cord blood Pb was associated with a 3% decrease in IGF2 methylation among girls (P = 0.04) and the decrease among boys was not significant (P = 0.66). IGF2 data were first adjusted by a standard curve of known methylated or unmethylated controls from each experimental batch.
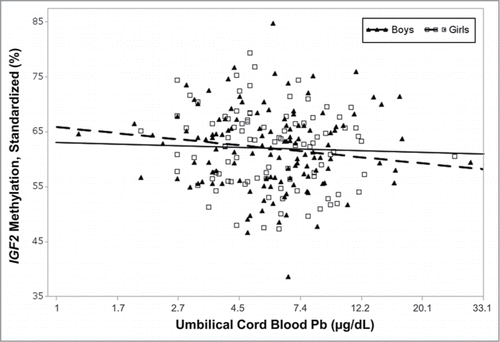
Figure 3. Maternal patella Pb and HSD11B2 methylation stratified by sex. Percent methylation upstream of the HSD11B2 promoter in blood leukocyte DNA increased with maternal patella Pb levels, indicative of in utero exposure. In a repeated measures model adjusting for gestational age and maternal folate intake, a 10 μg/g increase in maternal patella Pb of the girls was associated with a 0.23% increase in HSD11B2 methylation (near significant, P = 0.07). The increase was less notable among boys (P = 0.41). All methylation values were first standardized to known control curves from the same experimental batch.
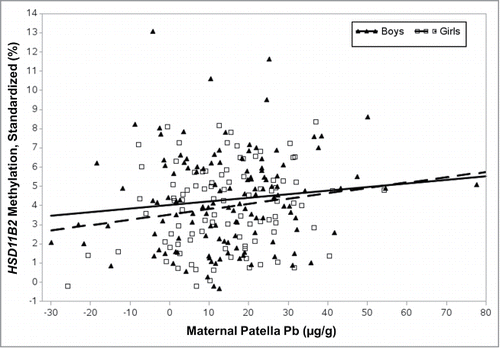
Sensitivity analyses
Relationships between Pb and methylation remained consistent (direction, attainment of statistical significance) between continuous and categorical bone Pb models (data not shown). Furthermore, exclusion of one patella Pb outlier in the continuous Pb models did not substantially alter reported results; in IGF2 and HSD11B2 models, statistical significance increased when excluding the outlier. Because it is unknown if true methylation differences due to exposures are in an absolute scale or if the exposure effects are proportional to the variance at the CpG site, we created Z-scores for each CpG site and estimated Pb-methylation relationships using Model #4 with the Pb-by-site interaction term. Similar relationships were observed (see one example in Table S5).
Modeling ELEMENT data using other approaches
As an example of the difference in inferences that can be obtained with other common statistical approaches using real data, we also fitted the remaining models described in . In all cases, the models were adjusted for gestational age, sex, and maternal folate intake (see and S4 for results). Substantial changes to direction of effect and statistical significance occur when batch is accounted for via standardized methylation values. For example, the relationship between hypomethylation of LINE-1 and patella Pb apparent with Model #1 disappears (Table S4, Model 1 vs. Model 5 both using OLS). In contrast, an association between HSD11B2 hypermethylation with higher patella Pb emerges (, Model 1 vs. either Model 4 or Model 5 depicted in ). Similarly, substantial changes to effect size and significance level were noted for associations between patella Pb and both IGF2 and HSD11B2 methylation () when comparing to no batch adjustment (Model #2). Controlling for batch via a random intercept (Model #3) in many cases diminished the estimated association ( and S4) as can be expected given the simulation study findings.
Discussion
This study highlights the need to carefully consider statistical modeling and batch adjustment approaches in environmental epigenetic studies with candidate region methylation data obtained using platforms such as pyrosequencing. Based on results from both real data examples and simulation studies that objectively compared modeling and batch adjustment approaches, we draw several recommendations that are particularly geared toward the environmental epigenetics field and other studies expecting small effect sizes for the variables of interest on DNA methylation. We recommend: 1) carefully checking for and correcting for batch effects; 2) utilizing models for repeated measures that incorporate the actual values at each CpG site in the interrogated region and, within those models, specifically modeling site-specific means and variances in methylation; and 3) evaluating site-specific and sex-specific effects.
In the ELEMENT study data, we observed modest evidence for an association between in utero Pb exposure and changes to umbilical cord blood leukocyte DNA methylation. IGF2 and HSD11B2 methylation was higher among those with higher maternal patella Pb levels, and several Pb-methylation relationships were observed among newborn girls but not boys. Although none of these relationships remain significant after adjusting for multiple hypothesis testing (P ≤ 0.004) except a significant interaction between LINE-1 CpG site and patella Pb driven by the second CpG site, these findings point out the importance of exploring site- and sex-specific effects to better understand potential biological mechanisms. Alterations to DNA methylation, specifically of growth-related genes, from in utero Pb exposure (for which patella Pb serves as a biomarkerCitation34) have the potential to impact childhood growth and development. In ELEMENT and other cohort studies, in utero Pb exposure has been shown to impact size at birth,Citation35-37 growth trajectories and size throughout infancyCitation38, and childhoodCitation39 in a sex-dependent fashion.Citation39 Further study of the impact of Pb exposure on the DNA methylome is warranted as previous perinatal rodent studiesCitation11,12 and adult cohort studiesCitation13,14,16,17 found links between Pb exposure and percent DNA methylation at various lifestages, and only 3 genes and LINE-1 repetitive elements were interrogated in this study.
Experimental batch effects are known to be problematic and are now routinely corrected for in molecular biology techniques including gene expression analysis with Real Time quantitative-PCRCitation28,29 and array-based platforms for interrogating DNA methylation across the epigenome.Citation24,26 By contrast, quality control measures vary widely for pyrosequencing and other high-throughput platforms for quantifying DNA methylation at candidate regions. Many laboratories have developed stringent validation procedures for new pyrosequencing assays that examine full standard curves from commercially available unmethylated and methylated standards or various homemade standards (e.g., plasmid control DNA,Citation40 multiple displacement based whole genome amplification with or without SssI-methylase treatmentCitation41). While some high-throughput studies standardize sample methylation levels to controls included in each batch,Citation30 this is not a widespread practice. In multiple batch studies, we highly recommend statistically testing for batch effects in data from each candidate region before proceeding with further data analysis to avoid reporting spurious results. Certain regions may be more prone to batch effects in bisulfite sequencing than others. Batch effects likely stem from the PCR step as biased amplification of bisulfite converted DNA can occur when the melting temperature differs by methylation status of the template.Citation42 Repetitive elements such as LINE-1 represent a special case in which a consensus region of thousands of LINE-1 elements are amplified, and the proportion of specific LINE-1s amplified each time can differ leading to slight variations in quantified methylation.
Accounting for batch effects is important in environmental epigenetics studies where the magnitude of change from exposure is expected to be small (<0 .5 SD as we observed) or is similar to the batch effect size. Adjusting for batch effects will often remove the potential for bias in associations and increase power to detect associations since between-batch variation is removed. Adding batch as a random effect when evaluating associations between variables of interest (e.g., chemical exposures) and DNA methylation is one potential correction method (see Model #3, ). However, in the ELEMENT data we discovered that exposure levels happened to vary by group of subjects included in the experimental batches (Table S2). Samples were placed in experimental batches (PCR plates) ordered by subject ID, a common practice in high-throughput analyses. Batches of subjects had different Pb levels which may have correlated with recruitment factors (e.g., timing, region). Randomizing subjects by exposure level on experimental plates is one practice that would eliminate this problem. Between-batch differences in Pb levels prompted us to consider and evaluate other approaches to correct for batch effects.
We found through simulation studies that standardizing to a control curve has comparable power to including random effects for batch, and in addition removes attenuation bias in the estimated effect size that remains when adjusting for batch using random effects. In the emerging environmental epigenetic literature, the practical importance of small effect sizes may be called into question since detected associations may be small. Not standardizing to batch standard-curves will yield attenuated associations when standard curves have slopes less than 1.
Platforms such as pyrosequencing and Sequenom EpiTYPER can quantify methylation at several CpG sites within the same region. Typically, percent methylation at neighboring CpG sites is highly correlated, though individual CpG sites can be differentially methylated and/or have roles in gene regulation (due to location in a transcription factor binding site, for example).Citation43,44 Further, between-subject variation may differ across CpG sites. Statistical analysis often involves averaging methylation across all quantified sites (see Models #1 and 5, ). Along with others in the field, we recommend further evaluation of CpG site-specific effects by treating methylation measures at each site as a repeated measure using a model that explicitly models the variances and correlations across sites (Models #2–4).Citation1,5,Citation45-47 This approach yields higher power to detect associations compared with the OLS approach that averages site methylation data (Model #5). Incorporating the correct variance-covariance structure in repeated measures models is known to increase the precision of effect.Citation33 Utilizing an unstructured covariance matrix allowed us to best approximate the true relationships between CpG sites in an interrogated region which differed widely by genic region (Table S1), and did not incur loss of power due to estimating 6–10 variance-covariance parameters. Difference in within-gene CpG site variances and correlations across assayed regions has also been observed in other studies.Citation48
An additional advantage of the repeated measures model is the ability to test whether specific CpG sites have distinct relationships with exposure by adding an interaction term between site and exposure to the model (Table S5), a method others have found useful in determining labile CpG sites.Citation1 Upon performing this analysis, a relationship between patella Pb and hypermethylation of LINE-1 CpG site #2 was identified (Table S5). Although this relationship could also be identified in individual models run for each LINE-1 CpG site, a formal assessment of whether the associations differ across sites cannot be readily computed. The repeated measures model has the advantage of estimating and testing differences in site-specific relationships within a single model.
DNA methylation patterns differ between the sexes.Citation5,49,50 Sex-specific relationships between toxicant exposures and DNA methylation have been observed in animalsCitation11 and humans.Citation7,9,51 In this study, LINE-1 was hypomethylated in girls compared to boys regardless of exposure (ANOVA P = 0.04, data not shown), a pattern previously observed in the Center for the Health Assessment of Mothers and Children of Salinas (CHAMACOS) cohortCitation5 and among adults.Citation50 Results following sex stratification suggested that DNA methylation in girls at LINE-1, IGF2, and HSD11B2 may be more sensitive to change following in utero Pb exposure, though sample size was small and significance marginal (Table S6, and ). Including sex stratification or exposure-sex interaction terms in statistical procedures is crucial when evaluating relationships between DNA methylation and exposure or health outcomes.
While many environmental epigenetic studies report associations between percent DNA methylation at specific genes and exposures, the effect size is often small in both epidemiological birth cohort studiesCitation6-8,Citation52 and adult cohort studies.Citation16,17 For example, given the estimated 0.14% difference in HSD11B2 methylation per 10 μg/g higher patella lead, we would expect those with the 75th percentile patella Pb concentration to differ by 0.27% methylation (or 0.45% methylation among girls) compared to those with the 25th percentile of patella Pb. The biological significance of such a small change on gene expression, recruitment of binding proteins, other epigenetic modifications, or downstream health outcomes is uncertain. Even so, this difference of 0.27% is equivalent to 0.2SD of HSD11B2, and an entire range increase (minimum to maximum) is expected to produce a 0.9 SD increase in HSD11B2 methylation among girls. Certain regions of the genome exhibit greater methylation variability and may be responsive to environmental influences, especially during early in utero development. If environmental factors such as Pb influenced >0.5 SD of percent methylation in these regions, termed metastable epialleles,Citation53-55 the change could be biologically significant.
Environmental epigenetics has emerged as a field with immense promise to identify 1) early life exposures causing persistent changes to the epigenome that may impact disease susceptibility,Citation56 2) epigenetic biomarkers of past exposures that cannot be measured,Citation6,17 or 3) reversible epigenetic modifications to target therapeutically or nutritionally.Citation57 Thus, despite limitations and uncertainty in the current state of environmental epigenetics research, important links have been made between early life exposures and epigenetic modification in animal models Citation1-4 and humans.Citation5-10 Incorporation of stringent quality control measures in the laboratory and careful statistical analysis of data following recommendations described in this article will increase confidence in results obtained from quantitative bisulfite sequencing of candidate regions. This will enable researchers to better evaluate associations between exposures and DNA methylation at key genes. Coupling methylation data with gene expression and health outcome data (statistically via meditational analyses) will further improve our understanding of the biological implications of observed changes.
Materials and methods
ELEMENT study
Sample population
In 1994 and 1995, mother-infant pairs were recruited at delivery at 3 Mexico City hospitals as the first cohort of the Early Life Exposures in Mexico to Environmental Toxicants (ELEMENT) study. ELEMENT Cohort I was originally designed to test the ability of calcium supplementation to decrease maternal to infant Pb mobilization during lactation. Exclusion criteria included living outside the metropolitan area, multiple fetuses, and maternal conditions that could interfere with in utero development (e.g., preeclampsia, gestational diabetes, seizure disorders, psychiatric, kidney or cardiac diseases). Full exclusion criteria and cohort details are described elsewhere.Citation36,38
Umbilical cord blood samples were collected from the neonate. Maternal and neonate anthropometry was recorded within 12 hours of delivery by trained obstetric nurses as previously detailed.Citation35,36 Mother-infant pairs visited the research center at one month postpartum (±5 days) for further evaluation and maternal bone Pb measurement. Of 617 mother-infant pairs who participated in the calcium supplementation trial, 248 umbilical cord blood samples were available for DNA extraction for the current investigation. Of these, high quality DNA was extracted from 247 infants, and these subjects are included in the analyses described herein.
Study procedures and strategies for Pb exposure reduction were explained to participating mothers, and mothers provided written consent. The research protocol was approved by the Human Subjects Committee of the National Institute of Public Health of Mexico, participant hospitals, and the Internal Review Board at all participating institutions including the University of Michigan.
Questionnaires
Mothers were interviewed at one-month postpartum to obtain information on demographic characteristics, health status of the mother and infant, smoking, and reproductive history. Gestational age was extracted from the medical record. A semi-quantitative food frequency questionnaire was administered to estimate maternal intake of nutrients during pregnancy. This questionnaire was previously validated in women living in Mexico City.Citation58
Lead (Pb) measurements
In utero Pb exposure was approximated via Pb levels in 3 biomarkers: umbilical cord blood, maternal tibia, and maternal patella using methods previously described.Citation36,38 In brief, blood Pb was quantified via atomic absorption spectroscopy with a Perkin-Elmer 3000 (Chelmsford, MA) at the American British Cowdray Hospital in Mexico City. Good precision and accuracy was obtained when comparing measurements with blinded replicates performed at the Wisconsin State Laboratory of Hygiene for quality control purposes.Citation36 Cortical (left mid-tibia shaft) and trabecular (left patella) bone Pb measurements were obtained from mothers at one month post-partum using a spot-source 109Cd K-XRF instrument. Details on the physical principles, technical specifications, and validation of the method were previously described.Citation31,59,60 The Pb fluorescent signal is normalized to an indicator of bone density - the scattered γ-ray signal stemming from calcium and phosphorus in bone mineral – which results in negative values in some instances. Each K-XRF measurement also comes with an estimate of the uncertainty (e.g., measurement error which is increased by factors such as low bone density and obesity). Here, bone Pb measurements were excluded if the uncertainty estimate was >15 for patella Pb or >10 for tibia Pb.
Epigenetic analyses
DNA was isolated from umbilical cord blood leukocytes by the Harvard-Partners Center for Genetics and Genomics using PureGene kits (Gentra Systems) and stored at −80°C before transfer to the University of Michigan on dry ice.Citation61 DNA concentration was quantified with a NanoDrop 2000 (ThermoFisher Scientific). Genomic DNA (0.5–1 μg) was bisulfite converted according to standard methods with EpiTect Bisulfite Kits (Qiagen). This treatment converts unmethylated cytosine to uracil (and ultimately thymine following PCR) while leaving methylated cytosine intact.Citation62
DNA methylation (percent of methylated cells) was quantified via pyrosequencing at global repetitive elements (LINE-1) and 3 genes important for early growth (IGF2, H19, HSD11B2).Citation63,64 Methylation at 4 CpG sites in LINE-1 was quantified via our previously published assay.Citation46 Differentially methylated regions (DMRs) of the imprinted genes, IGF2 (upstream of imprinted promoter at IGF2 exon 3) and H19 (upstream of H19 within the imprint control center), were interrogated based on validated assays.Citation65 PyroMark Assay Design Software version 2.0 was used to design the pyrosequencing assay for HSD11B2 to include 4 CpG sites in a CpG island upstream of the gene promoter (region described byCitation66). For each region, the sequence was amplified from approximately 50 ng bisulfite converted DNA by HotStartTaq Master Mix (Qiagen) and forward and reverse-biotinylated primers. Percent of methylated cells at each region was quantified by the PyroMark MD Pyrosequencer Platform (Qiagen) and computed by Pyro Q-CpG Software. The software incorporates controls to check for completed bisulfite conversion and adequate signal over background noise. All samples were run in duplicate, and duplicate reads were averaged. Each assay was validated in the lab by running 6 point standard curves made from serial dilutions of 0 and 100% methylated human control DNA (Qiagen). Every experimental plate (batch) contained at least 4 point standard curves, except LINE-1 plates which only included 0 and 100% methylated control DNA. Measures of methylated control DNA were precise across 96-well plates (e.g., <10% coefficient of variation, CV, for 100% controls). Duplicate samples had good precision for IGF2 (mean 1.8% CV), H19 (1.8% CV), and LINE-1 (1.4% CV). Methylation of HSD11B2 was consistently low (<10%) and variable (36% CV among duplicate samples).
Simulation study to evaluate modeling and batch adjustment approaches
Modeling strategies
Ordinary least squares (OLS) regression is commonly used to estimate relationships among DNA methylation and covariates; in this approach data from multiple CpG sites for a given locus are averaged to obtain a single outcome measure per individual. This approach ignores differences in mean, variance, and pair-wise correlations among CpG sites. Instead, models for multivariate data (i.e., multiple outcome measures per individual) can be used, to directly use the actual values at each CpG site.Citation66 Furthermore, models for repeated measures can be used to rigorously test if the toxicant-DNA methylation association varies across CpG sites. While separate OLS models can be fit to each site, a test of whether the associations differ by site cannot be readily obtained.
Models for repeated measures include random effects (RE) models or marginal models. The general linear model (GLM) for repeated measures and generalized estimating equations (GEE) are 2 marginal models that, in the case of continuous outcomes, primarily differ in how variances across sites are modeled: widely available software implementations of GEE typically assume variances are equal across sites, whereas implementations of GLM explicitly require a model for the variances as well as the correlations. A random effects model with a random intercept is equivalent to a GLM with a compound symmetry assumption, and gives the same estimated association as GEE with exchangeable correlation structure. Although robust standard error estimators are available to guard against incorrect modeling of the variance-covariance structure among repeated measures in the GEE approach, it is well known that mis-specified variance-covariance have lower power.Citation33 We used simulated data where toxicant-DNA methylation associations are known to compare these modeling approaches.
Batch adjustment
The most common method for batch adjustment is to consider batch effects as randomly shifting the means of the methylation values by a similar amount for all samples in a plate and to model the data including a random intercept for plate in mixed effects models. However, this adjustment method may not be appropriate because the batch effects can potentially vary by CpG site, not only in the mean but also by shifting the scale of the measure (see intercepts and slopes in Table S3). An alternative is to correct for batch effects by standardizing methylation data at each CpG site to a standard curve for that site constructed using measures for known unmethylated and methylated control DNA run on each experimental plate. In the ELEMENT data, the standard curves for H19, HSD11B2, and IGF2 were linear with slopes less than one; i.e., observed methylation for control sample = â + ŝ*known value of control sample, with ŝ < 1 being the estimated slope of the plate- and site-specific curve, and â the estimated intercept, Table S3). For the subjects in the study, the standardized values are calculated as (observed sample- â)/ŝ.
Simulated data
To objectively evaluate the modeling strategies and batch adjustment approaches, we simulated data that were similar to the ELEMENT data, but where the association between a toxicant and DNA values were known. First, data were simulated without batch effects (called “true data”) using Yij,true = β0j + β1 Xi + eij, where β0j is the mean of site j (See ), Xi had a normal distribution with mean 14.4 and SD = 14.8 (similar to patella bone lead), and eij had correlation structure similar to observed in the ELEMENT data (Table S1). The standard deviations of eij were set equal to those observed in ELEMENT () or fixed to be equal for all sites (as the average of the standard deviations across the sites). The value of the association, β1, was set to vary from 0 to 0.15; within this range, the power to detect the known associations ranges from 0.05 (Type I error) to 1.
“Observed data” with batch effects were simulated as Yij,obs = akj + skj * Yij,true, where akj and skj were random batch effects specific to site j and plate k generated from a normal distribution mean and standard deviation similar to what was observed in the ELEMENT data (see Table S3). To mimic the fact that in a real situation the intercept and slopes of the standard curve are estimated, we generated standardized data as Yijk,std =(Yij,obs − âkj)/ŝkj, where âkj = akj + δkja and ŝk = sk + δkjs include estimation errors, δkja and δkjs; if we used the generated akj and skj we would recover exactly the “true data” Yij,true. Following simple linear regression formulae, the variance of the estimation errors, δkja and δkjs, were chosen such that the R2 of the fitted standard curves were, on average R2 = 0.999, 0.99 and 0.88. For each combination of parameters (β1, R2, variance and correlation structure of eij) 1000 datasets, each with 220 subjects, were generated.
Models fitted and model performance measures
We fitted the models described under “Modeling strategies” to show the performance of the various modeling approaches. Average bias (i.e., difference between estimated and known β1 across the 1000 simulated data sets) and empirical power (the proportion of times the hypothesis H0: β1 = 0 was rejected in the 1000 simulated datasets) were computed. The GLM model with unstructured variance-covariance matrix was shown to have the best performance (high power and unbiased estimates). Next, we fitted the GLM to Yij,obs, Yij,std, and also the GLM with a random intercept for batch to examine impact of the adjustment method on bias and power. For comparison to what is typically done, we also fitted OLS to person-specific average (averaged across site).
Statistical analyses of ELEMENT study data
All statistical analyses were performed with SAS 9.3 (SAS Institute). Univariate statistics and distributions of variables were examined. Cord blood Pb was natural log-transformed in all analyses. Lead exposure biomarkers and methylation values for each candidate gene were compared across experimental batches (one batch = same PCR plate and same pyrosequencing date) by ANOVA (used Welch's ANOVA if variances were not homogenous). Bivariate and multivariable analyses were conducted to examine the association between biomarkers of in utero Pb exposure (cord blood Pb, maternal tibia Pb, maternal patella Pb) and DNA methylation levels at 4 candidate regions (LINE-1, IGF2, H19, and HSD11B2). Given the results of the simulation study, Model 4 () was used as the primary modeling approach. Results were considered statistically significant at the P < 0.05 level, but also discussed in the context of multiple hypothesis testing (12 Pb-gene relationships) with a P-value ≤0.004. Multivariable analyses using different modeling strategies and methods for batch adjustments () were also employed to compare the methods in a real data example.
Covariates
All models included child's sex, gestational age, and estimated maternal folate intake during pregnancy, which are biologically relevant predictors of methylation. Inclusion of additional covariates (indicators of maternal socioeconomic status, maternal smoking history) selected using backward elimination for specific genes did not substantially change reported results.
Site- and sex-specific effects
Model #4 was run with an interaction term between CpG site and Pb to assess whether individual CpG sites had different relationships with Pb. To examine sex-specific effects which are of increasing importance in public health research, Model #4 was also run with an interaction term between sex and Pb.Citation67 If the interaction term and/or main effect of Pb had P < 0.1, the model was run following sex stratification.
Sensitivity analyses
Sensitivity analyses revealed 3 highly influential data points for LINE-1, IGF2, and H19 with percent methylation outside of the expected range. These data points were removed in all reported analyses. Model #4 was also run after removing one patella Pb outlier. Further sensitivity analyses involved using quartiles of tibia and patella Pb instead of the continuous variable to remove any potential influence of the negative bone Pb measures (which were classified as the lowest quartile). Since range and variance of methylation values within genes differed across CpG sites, and it is unknown if toxicants influence methylation in the absolute scale (e.g., equal % difference across all sites), or in a scale relative to standard deviation (e.g., effect is in units of % difference/standard deviation at the CpG site), a separate analysis was performed (Model #4 with and without Pb by CpG site interaction term) using z-scores for each CpG site (i.e., data were converted to have mean 0, SD 1 for each site).
Disclosure of Potential Conflicts of Interest
No potential conflicts of interest were disclosed.
Supplemental_Tables_and_Figures.docx
Download MS Word (458.6 KB)Acknowledgments
The authors acknowledge the research staff at participating hospitals. We thank the mothers and children for participating in the study. The contents of this publication are solely the responsibility of the grantee and do not necessarily represent the official views of the US EPA or the NIH. Further, the US EPA does not endorse the purchase of any commercial products or services mentioned in the publication.
Funding
This publication was made possible by U.S. Environmental Protection Agency (US EPA) grants RD834800 and RD83543601 and National Institute for Environmental Health Sciences (NIEHS) grants P20 ES018171, P01 ES02284401, R01 ES007821, R01 ES014930, R01 ES013744, P42 ES05947, and P30 ES017885. JMG was also supported by the National Center for Advancing Translational Sciences (grant no. 2UL1TR00433).
Supplemental Material
Supplemental data for this article can be accessed on thepublisher's website.
References
- Weinhouse C, Anderson OS, Jones TR, Kim J, Liberman SA, Nahar MS, Rozek LS, Jirtle RL, Dolinoy DC. An expression microarray approach for the identification of metastable epialleles in the mouse genome. Epigenetics 2011; 6:1105-13; PMID:21829099; http://dx.doi.org/10.4161/epi.6.9.17103
- Dolinoy DC, Huang D, Jirtle RL. Maternal nutrient supplementation counteracts bisphenol A-induced DNA hypomethylation in early development. Proc Natl Acad Sci U S A 2007; 104:13056-61; PMID:17670942; http://dx.doi.org/10.1073/pnas.0703739104
- Dolinoy DC, Weidman JR, Waterland RA, Jirtle RL. Maternal genistein alters coat color and protects avy mouse offspring from obesity by modifying the fetal epigenome. Environ Health Perspect 2006; 114:567-72; PMID:16581547; http://dx.doi.org/10.1289/ehp.8700
- Anderson OS, Nahar MS, Faulk C, Jones TR, Liao C, Kannan K, Weinhouse C, Rozek LS, Dolinoy DC. Epigenetic responses following maternal dietary exposure to physiologically relevant levels of bisphenol A. Environ Mol Mutagen 2012; 53:334-42; PMID:22467340; http://dx.doi.org/10.1002/em.21692
- Huen K, Yousefi P, Bradman A, Yan L, Harley KG, Kogut K, Eskenazi B, Holland N. Effects of age, sex, and persistent organic pollutants on DNA methylation in children. Environ Mol Mutagen 2014; 55:209-22; PMID:24375655; http://dx.doi.org/10.1002/em.21845
- Joubert BR, Haberg SE, Nilsen RM, Wang X, Vollset SE, Murphy SK, Huang Z, Hoyo C, Midttun O, Cupul-Uicab LA, et al. 450K epigenome-wide scan identifies differential DNA methylation in newborns related to maternal smoking during pregnancy. Environ Health Perspect 2012; 120:1425-31; PMID:22851337; http://dx.doi.org/10.1289/ehp.1205412
- Kippler M, Engstrom K, Mlakar SJ, Bottai M, Ahmed S, Hossain MB, Raqib R, Vahter M, Broberg K. Sex-specific effects of early life cadmium exposure on DNA methylation and implications for birth weight. Epigenetics 2013; 8:494-503; PMID:23644563; http://dx.doi.org/10.4161/epi.24401
- Koestler DC, Avissar-Whiting M, Houseman EA, Karagas MR, Marsit CJ. Differential DNA methylation in umbilical cord blood of infants exposed to low levels of arsenic. Environ Health Perspect 2013; 121:971-77; PMID:23757598
- Murphy SK, Adigun A, Huang Z, Overcash F, Wang F, Jirtle RL, Schildkraut JM, Murtha AP, Iversen ES, Hoyo C. Gender-specific methylation differences in relation to prenatal exposure to cigarette smoke. Gene 2011; 494:36-43; PMID:22202639; http://dx.doi.org/10.1016/j.gene.2011.11.062
- Rager JE, Bailey KA, Smeester L, Miller SK, Parker JS, Laine JE, Drobná Z, Currier J, Douillet C, Olshan AF, et al. Prenatal arsenic exposure and the epigenome: Altered microRNAs associated with innate and adaptive immune signaling in newborn cord blood. Environ Mol Mutagen 2014; 55:196-208; PMID:24327377; http://dx.doi.org/10.1002/em.21842
- Faulk C, Barks A, Liu K, Goodrich JM, Dolinoy DC. Early-life lead exposure results in dose- and sex-specific effects on weight and epigenetic gene regulation in weanling mice. Epigenomics 2013; 5:487-500; PMID:24059796; http://dx.doi.org/10.2217/epi.13.49
- Dosunmu R, Alashwal H, Zawia NH. Genome-wide expression and methylation profiling in the aged rodent brain due to early-life pb exposure and its relevance to aging. Mech Ageing Dev 2012; 133:435-443; PMID:22613225; http://dx.doi.org/10.1016/j.mad.2012.05.003
- Hanna CW, Bloom MS, Robinson WP, Kim D, Parsons PJ, Vom Saal FS, Taylor JA, Steuerwald AJ, Fujimoto VY. DNA methylation changes in whole blood is associated with exposure to the environmental contaminants, mercury, lead, cadmium and bisphenol A, in women undergoing ovarian stimulation for IVF. Hum Reprod 2012; 27:1401-10; PMID:22381621; http://dx.doi.org/10.1093/humrep/des038
- Kovatsi L, Georgiou E, Ioannou A, Haitoglou C, Tzimagiorgis G, Tsoukali H, Kouidou S. p16 promoter methylation in Pb2+ -exposed individuals. Clin Toxicol (Phila) 2010; 48:124-8; PMID:20199129
- Pilsner JR, Hu H, Ettinger A, Sanchez BN, Wright RO, Cantonwine D, Lazarus A, Lamadrid-Figueroa H, Mercado-Garcia A, Tellez-Rojo MM, et al. Influence of prenatal lead exposure on genomic methylation of cord blood DNA. Environ Health Perspect 2009; 117:1466-71; PMID:19750115; http://dx.doi.org/10.1289/ehp.0800497
- Li C, Yang X, Xu M, Zhang J, Sun N. Epigenetic marker (LINE-1 promoter) methylation level was associated with occupational lead exposure. Clin Toxicol (Phila) 2013; 51:225-29; PMID:13528182; http://dx.doi.org/10.3109/15563650.2013.782410
- Wright RO, Schwartz J, Wright RJ, Bollati V, Tarantini L, Park SK, Hu H, Sparrow D, Vokonas P, Baccarelli A. Biomarkers of lead exposure and DNA methylation within retrotransposons. Environ Health Perspect 2010; 118:790-5; PMID:20064768; http://dx.doi.org/10.1289/ehp.0901429
- Bogdanovic O, Veenstra GJ. DNA methylation and methyl-CpG binding proteins: developmental requirements and function. Chromosoma 2009; 118:549-65; PMID:19506892; http://dx.doi.org/10.1007/s00412-009-0221-9
- Razin A, Cedar H. DNA methylation and gene expression. Microbiol Rev 1991; 55:451-8; PMID:1943996
- Umer M, Herceg Z. Deciphering the epigenetic code: an overview of DNA methylation analysis methods. Antioxid Redox Signal 2013; 18:1972-86; PMID:23121567; http://dx.doi.org/10.1089/ars.2012.4923
- Tost J, Gut IG. DNA methylation analysis by pyrosequencing. Nature Protocols 2007; 2:2265-75; PMID:17853883; http://dx.doi.org/10.1038/nprot.2007.314
- Ehrich M, Nelson MR, Stanssens P, Zabeau M, Liloglou T, Xinarianos G, Cantor CR, Field JK, van den Boom D. Quantitative high-throughput analysis of DNA methylation patterns by base-specific cleavage and mass spectrometry. Proc Natl Acad Sci U S A 2005; 102:15785-90; PMID:16243968; http://dx.doi.org/10.1073/pnas.0507816102
- Herman JG, Graff JR, Myohanen S, Nelkin BD, Baylin SB. Methylation-specific PCR: a novel PCR assay for methylation status of CpG islands. Proc Natl Acad Sci U S A 1996; 93:9821-6; PMID:8790415; http://dx.doi.org/10.1073/pnas.93.18.9821
- Marabita F, Almgren M, Lindholm ME, Ruhrmann S, Fagerstrom-Billai F, Jagodic M, Sundberg CJ, Ekstrom TJ, Teschendorff AE, Tegner J, et al. An evaluation of analysis pipelines for DNA methylation profiling using the illumina HumanMethylation450 BeadChip platform. Epigenetics 2013; 8:333-46; PMID:23422812; http://dx.doi.org/10.4161/epi.24008
- Teschendorff AE, Marabita F, Lechner M, Bartlett T, Tegner J, Gomez-Cabrero D, Beck S. A beta-mixture quantile normalization method for correcting probe design bias in illumina infinium 450 k DNA methylation data. Bioinformatics 2013; 29:189-96; PMID:23175756; http://dx.doi.org/10.1093/bioinformatics/bts680
- Morris TJ, Butcher LM, Feber A, Teschendorff AE, Chakravarthy AR, Wojdacz TK, Beck S. ChAMP: 450k chip analysis methylation pipeline. Bioinformatics 2014; 30:428-30; PMID:24336642; http://dx.doi.org/10.1093/bioinformatics/btt684
- Pidsley R, Y Wong CC, Volta M, Lunnon K, Mill J, Schalkwyk LC. A data-driven approach to preprocessing illumina 450K methylation array data. BMC Genomics 2013; 14:293; PMID:23631413; http://dx.doi.org/10.1186/1471-2164-14-293
- Bustin SA, Benes V, Garson JA, Hellemans J, Huggett J, Kubista M, Mueller R, Nolan T, Pfaffl MW, Shipley GL, et al. The MIQE guidelines: Minimum information for publication of quantitative real-time PCR experiments. Clin Chem 2009; 55:611-22; PMID:19246619; http://dx.doi.org/10.1373/clinchem.2008.112797
- Hellemans J, Mortier G, De Paepe A, Speleman F, Vandesompele J. qBase relative quantification framework and software for management and automated analysis of real-time quantitative PCR data. Genome Biol 2007; 8:R19; PMID:17291332
- DeRoo LA, Bolick SCE, Xu Z, Umbach DM, Shore D, Weinberg CR, Sandler DP, Taylor JA. Global DNA methylation and one-carbon metabolism gene polymorphisms and the risk of breast cancer in the sister study. Carcinogenesis 2014; 35:333-8; PMID:24130171; http://dx.doi.org/10.1093/carcin/bgt342
- Hu H, Aro A, Rotnitzky A. Bone lead measured by X-ray fluorescence: epidemiologic methods. Environ Health Perspect 1995; 103 Suppl 1:105-10; PMID:7621788; http://dx.doi.org/10.1289/ehp.95103s1105
- Lamadrid-Figueroa H, Tellez-Rojo MM, Angeles G, Hernandez-Avila M, Hu H. Bias correction by use of errors-in-variables regression models in studies with K-X-ray fluorescence bone lead measurements. Environ Res 2011; 111:17-20; PMID:21092947; http://dx.doi.org/10.1016/j.envres.2010.10.011
- Fitzmaurice GM, Laird NM, Ware JH. Applied Longitudinal Analysis. 2nd ed. Hoboken, New Jersey: John Wiley & Sons, Inc 2011.
- Hernandez-Avila M, Smith D, Meneses F, Sanin LH, Hu H. The influence of bone and blood lead on plasma lead levels in environmentally exposed adults. Environ Health Perspect 1998; 106:473-77; PMID:9681974; http://dx.doi.org/10.1289/ehp.98106473
- Hernandez-Avila M, Peterson KE, Gonzalez-Cossio T, Sanin LH, Aro A, Schnaas L, Hu H. Effect of maternal bone lead on length and head circumference of newborns and 1-month-old infants. Arch Environ Health 2002; 57:482-88; PMID:12641193; http://dx.doi.org/10.1080/00039890209601441
- Gonzalez-Cossio T, Peterson KE, Sanin LH, Fishbein E, Palazuelos E, Aro A, Hernandez-Avila M, Hu H. Decrease in birth weight in relation to maternal bone-lead burden. Pediatrics 1997; 100:856-62; PMID:9346987; http://dx.doi.org/10.1542/peds.100.5.856
- Xie X, Ding G, Cui C, Chen L, Gao Y, Zhou Y, Shi R, Tian Y. The effects of low-level prenatal lead exposure on birth outcomes. Environ Pollut 2013; 175:30-4; PMID:23321271; http://dx.doi.org/10.1016/j.envpol.2012.12.013
- Sanin LH, Gonzalez-Cossio T, Romieu I, Peterson KE, Ruiz S, Palazuelos E, Hernandez-Avila M, Hu H. Effect of maternal lead burden on infant weight and weight gain at one month of age among breastfed infants. Pediatrics 2001; 107:1016-23; PMID:11331680; http://dx.doi.org/10.1542/peds.107.5.1016
- Afeiche M, Peterson KE, Sanchez BN, Cantonwine D, Lamadrid-Figueroa H, Schnaas L, Ettinger AS, Hernandez-Avila M, Hu H, Tellez-Rojo MM. Prenatal lead exposure and weight of 0- to 5-year-old children in mexico city. Environ Health Perspect 2011; 119:1436-41; PMID:21715242; http://dx.doi.org/10.1289/ehp.1003184
- Wong HL, Byun HM, Kwan JM, Campan M, Ingles SA, Laird PW, Yang AS. Rapid and quantitative method of allele-specific DNA methylation analysis. BioTechniques 2006; 41:734-9; PMID:17191619; http://dx.doi.org/10.2144/000112305
- Hughes S, Jones JL. The use of multiple displacement amplified DNA as a control for methylation specific PCR, pyrosequencing, bisulfite sequencing and methylation-sensitive restriction enzyme PCR. BMC Mol Biol 2007; 8:91; PMID:17939862; http://dx.doi.org/10.1186/1471-2199-8-91
- Warnecke PM, Stirzaker C, Melki JR, Millar DS, Paul CL, Clark SJ. Detection and measurement of PCR bias in quantitative methylation analysis of bisulphite-treated DNA. Nucleic Acids Res 1997; 25:4422-6; PMID:9336479; http://dx.doi.org/10.1093/nar/25.21.4422
- Turker MS. The establishment and maintenance of DNA methylation patterns in mouse somatic cells. Semin Cancer Biol 1999; 9:329-37; PMID:10547341
- Weaver IC, Diorio J, Seckl JR, Szyf M, Meaney MJ. Early environmental regulation of hippocampal glucocorticoid receptor gene expression: Characterization of intracellular mediators and potential genomic target sites. Ann N Y Acad Sci 2004; 1024:182-212; PMID:15265782; http://dx.doi.org/10.1196/annals.1321.099
- Byun HM, Motta V, Panni T, Bertazzi PA, Apostoli P, Hou L, Baccarelli AA. Evolutionary age of repetitive element subfamilies and sensitivity of DNA methylation to airborne pollutants. Part Fibre Toxicol 2013; 10:28; PMID:23855992; http://dx.doi.org/10.1186/1743-8977-10-28
- Virani S, Dolinoy DC, Halubai S, Jones TR, Domino SE, Rozek LS, Nahar MS, Padmanabhan V. Delivery type not associated with global methylation at birth. Clin Epigenetics 2012; 4:8; PMID:22682523; http://dx.doi.org/10.1186/1868-7083-4-8
- Tarantini L, Bonzini M, Apostoli P, Pegoraro V, Bollati V, Marinelli B, Cantone L, Rizzo G, Hou L, Schwartz J, et al. Effects of particulate matter on genomic DNA methylation content and iNOS promoter methylation. Environ Health Perspect 2009; 117:217-22; PMID:19270791; http://dx.doi.org/10.1289/ehp.11898
- Vilahur N, Bustamante M, Byun HM, Fernandez MF, Santa Marina L, Basterrechea M, Ballester F, Murcia M, Tardon A, Fernandez-Somoano A, et al. Prenatal exposure to mixtures of xenoestrogens and repetitive element DNA methylation changes in human placenta. Environ Int 2014; 71:81-7; PMID:24980756; http://dx.doi.org/10.1016/j.envint.2014.06.006
- Xu H, Wang F, Liu Y, Yu Y, Gelernter J, Zhang H. Sex-biased methylome and transcriptome in human prefrontal cortex. Hum Mol Genet 2014; 23:1260-70; PMID:24163133; http://dx.doi.org/10.1093/hmg/ddt516
- Zhang FF, Cardarelli R, Carroll J, Fulda KG, Kaur M, Gonzalez K, Vishwanatha JK, Santella RM, Morabia A. Significant differences in global genomic DNA methylation by gender and race/ethnicity in peripheral blood. Epigenetics 2011; 6:623-629; PMID:21739720; http://dx.doi.org/10.4161/epi.6.5.15335
- Goodrich JM, Basu N, Franzblau A, Dolinoy DC. Mercury biomarkers and DNA methylation among michigan dental professionals. Environ Mol Mutagen 2013; 54:195-203; PMID:23444121; http://dx.doi.org/10.1002/em.21763
- Murphy SK, Adigun A, Huang Z, Overcash F, Wang F, Jirtle RL, Schildkraut JM, Murtha AP, Iversen ES, Hoyo C. Gender-specific methylation differences in relation to prenatal exposure to cigarette smoke. Gene 2012; 494:36-43; PMID:22202639; http://dx.doi.org/10.1016/j.gene.2011.11.062
- Dolinoy DC, Das R, Weidman JR, Jirtle RL. Metastable epialleles, imprinting, and the fetal origins of adult diseases. Pediatr Res 2007; 61:30R-37R; PMID:17413847; http://dx.doi.org/10.1203/pdr.0b013e31804575f7
- Waterland RA, Kellermayer R, Laritsky E, Rayco-Solon P, Harris RA, Travisano M, Zhang W, Torskaya MS, Zhang J, Shen L, et al. Season of conception in rural gambia affects DNA methylation at putative human metastable epialleles. PLoS Genet 2010; 6:e1001252; PMID:21203497; http://dx.doi.org/10.1371/journal.pgen.1001252
- Harris RA, Nagy-Szakal D, Kellermayer R. Human metastable epiallele candidates link to common disorders. Epigenetics 2013; 8:157-63; PMID:23321599; http://dx.doi.org/10.4161/epi.23438
- Heijmans BT, Tobi EW, Stein AD, Putter H, Blauw GJ, Susser ES, Slagboom PE, Lumey LH. Persistent epigenetic differences associated with prenatal exposure to famine in humans. Proc Natl Acad Sci U S A 2008; 105:17046-9; PMID:18955703; http://dx.doi.org/10.1073/pnas.0806560105
- Mai A, Altucci L. Epi-drugs to fight cancer: From chemistry to cancer treatment, the road ahead. Int J Biochem Cell Biol 2009; 41:199-213; PMID:18790076; http://dx.doi.org/10.1016/j.biocel.2008.08.020
- Hernandez-Avila M, Romieu I, Parra S, Hernandez-Avila J, Madrigal H, Willett W. Validity and reproducibility of a food frequency questionnaire to assess dietary intake of women living in mexico city. Salud Publica Mex 1998; 40:133-40; PMID:9617194; http://dx.doi.org/10.1590/S0036-36341998000200005
- Aro AC, Todd AC, Amarasiriwardena C, Hu H. Improvements in the calibration of 109Cd K x-ray fluorescence systems for measuring bone lead in vivo. Phys Med Biol 1994; 39:2263-71; PMID:15551552; http://dx.doi.org/10.1088/0031-9155/39/12/009
- Hu H, Milder FL, Burger DE. The use of K X-ray fluorescence for measuring lead burden in epidemiological studies: High and low lead burdens and measurement uncertainty. Environ Health Perspect 1991; 94:107-10; PMID:1954919; http://dx.doi.org/10.2307/3431301
- Hopkins MR, Ettinger AS, Hernandez-Avila M, Schwartz J, Tellez-Rojo MM, Lamadrid-Figueroa H, Bellinger D, Hu H, Wright RO. Variants in iron metabolism genes predict higher blood lead levels in young children. Environ Health Perspect 2008; 116:1261-6; PMID:18795173; http://dx.doi.org/10.1289/ehp.11233
- Grunau C, Clark SJ, Rosenthal A. Bisulfite genomic sequencing: Systematic investigation of critical experimental parameters. Nucleic Acids Res 2001; 29:E65-5; PMID:11433041; http://dx.doi.org/10.1093/nar/29.13.e65
- Seckl JR. Glucocorticoids, feto-placental 11 beta-hydroxysteroid dehydrogenase type 2, and the early life origins of adult disease. Steroids 1997; 62:89-94; PMID:9029721
- Lui JC, Finkielstain GP, Barnes KM, Baron J. An imprinted gene network that controls mammalian somatic growth is down-regulated during postnatal growth deceleration in multiple organs. Am J Physiol Regul Integr Comp Physiol 2008; 295:R189-96; PMID:18448610; http://dx.doi.org/10.1152/ajpregu.00182.2008
- Hoyo C, Murtha AP, Schildkraut JM, Jirtle RL, Demark-Wahnefried W, Forman MR, Iversen ES, Kurtzberg J, Overcash F, Huang Z, et al. Methylation variation at IGF2 differentially methylated regions and maternal folic acid use before and during pregnancy. Epigenetics. 2011; 6:928-36; PMID:21636975; http://dx.doi.org/10.4161/epi.6.7.16263
- Alikhani-Koopaei R, Fouladkou F, Frey F, Frey B. Epigenetic regulation of 11 beta-hydroxysteroid dehydrogenase type 2 expression. J Clin Invest: 114(8):1146-57; PMID:15489962
- National Institutes of Health (NIH) Office of Research on Women's Health. Moving into the future with new dimensions and strategies: a vision for 2020 women's health research. 2010; Report 10-7606.