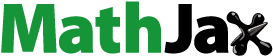
Abstract
In this paper, Penaeus orientolis prawn freshness rapid determination method using electronic nose (e-nose) and non-linear data processing technique is studied. E-nose responses to prawns stored at 4°C are measured. Meanwhile, physical/chemical indexes (firmness, pH, total volatile basic nitrogen (TVB-N), total viable count (TVC), and human sensory evaluation) are examined to provide freshness references for e-nose analysis. E-nose measurement data is analyzed by principal component analysis (PCA), stochastic resonance (SR), and double-layered cascaded serial stochastic resonance (DCSSR). PCA partially discriminates prawns under different storage time. SR and DCSSR signal-to-noise ratio (SNR) spectrum eigen values discriminate prawns successfully. Multi-variables regressions (MVR) are conducted between physical/chemical indexes and SR/DCSSR output SNR minimal (SNR-Min) values. Results indicate that SNR-Min values present more significant linearity relation with physical/chemical indexes. Prawn freshness forecasting model is developed via Harris fitting regression on DCSSR SNR-Min values. Validating experiments demonstrate that forecasting accuracy of this model is 94.29%.
Introduction
Penaeus orientolis prawn is particularly rich in protein, essential amino acids, and essential minerals (Fe, Zn, Se, etc.). It is now the most important shrimp cultivated in the world in terms of volume. It contains rich nutrients such as protein and micronutrients necessary for human body. However, it is hard to preserve and usually deteriorates in a few days. And protein, the main component of white shrimp, will produce toxic amine compounds such as trimethylamine, etc., after deterioration. The intake of deteriorated prawns brings great harm to human body, which has been one of the most serious problems in food safety area. Traditional aquatic products quality analysis methods mainly include instrumental analysis, physical/chemical examination, and human sensory evaluation. Instrumental analysis methods, such as VIS/NIR spectroscopy, gas chromatography-mass spectrometry (GC-MS), and impedance spectroscopy, are usually utilized for aquatic products freshness detection under laboratory conditions.Citation1-3 However, these methods have some disadvantages, such as high cost, time-consuming, etc. Moreover, skilled operators are required to perform instrumental analysis. Physical/chemical examination methods, such as conductivity/pH measurement and TVB-N, can examine freshness of aquatic products according to relative standards.Citation4,5 However, these methods present some disadvantages, such as high cost, time-consuming, low reproducibility, etc. Moreover, skilled operators are required to perform the instrumental analytical experiments. So a convenient, fast and robust method is badly needed for prawn freshness determination.
E-nose, imitating human olfactory sense, is a kind of electronic instrument.Citation6,7 In the past 2 decades, e-nose has been successfully applied in diverse fields such as food quality analysis, environmental control, industrial applications, and clinical diagnostics. Pattern recognition (PR) methods used by e-nose can be divided into supervised and non-supervised methods although a combination of both can be used.Citation8,9 The major supervised techniques are linear discriminant analysis (LDA), soft independent modeling of class analogy (SIMCA), partial least squares discriminant analysis (PLS-DA), k-nearest neighbors (KNN), artificial neural networks (ANN), and classification and influence matrix analysis (CAIMAN). Unsupervised techniques include cluster analysis (CA), artificial neural networks (ANN) and PCA.Citation10
In 2001, a portable electronic nose was used to detect fish freshness by estimating the number of storage days.Citation11 An electronic nose system was built based on metal oxide semiconductor (M.O.S) sensors to evaluate freshness of sardines under cold storage.Citation12 Results showed that the sensor signals were well correlated with the number of days that sardines had undergone. Discriminant factor analysis (DFA) and fuzzy ARTMAP neural networks were used to build classification models. Limbo et al. applied chemical methods and electronic nose to predict the decay and shelf life of European sea bass.Citation13 A new optoelectronic nose was developed to monitor shelf-life of fresh sea bream under cold storage.Citation14 In 2004, this group evaluated freshness of Atlantic salmon using optoelectronic nose.Citation15 Hui et al. predicted grass carp quality by an e-nose combined with non-linear SR.Citation7 The system presented good predicting accuracy for grass carp samples.
In this paper, prawn freshness rapid analysis method based on e-nose technique and non-linear SR is studied. Prawns stored at 4°C under different storage time were measured by e-nose. Physical/chemical indexes (firmness, pH, TVB-N, TVC, and human sensory evaluation) are examined in order to characterize prawns’ freshness. E-nose measurement data is processed by PCA, SR, and DCSSR. MVR results demonstrate that DCSSR eigen values present more significant linearity relation with physical/chemical indexes. Prawn freshness forecasting model is developed based on Harris fitting regression of DCSSR SNR-Min values. Validating experiments demonstrate that the developed model forecasts prawn freshness with an accuracy of 94.29%.
Results and Discussions
Firmness
Prawn firmness examination results are displayed in . Firmness increases slightly in day 2. After day 2, firmness keeps declining and reaches less than 70N in day 6 finally. A possible explanation for this phenomenon lies in the changes of ATP content within shrimps during cold storage. ATP determines the state of actin and myosin. After prawns’ death, glide between actin and myosin happens because of ATP decomposition with the increase of storage days, which leads to muscle stiffness. After reaching stiffness maximal value, endogenous proteases within muscles and exogenous proteases induced by spoilage bacteria make Z line of myofibrillar more weak or broken. So prawns’ muscle becomes soft, which leads to the decline in firmness.
pH
Prawn's inner chemical environment changes with the decrease of its quality during storage. pH measurement results are displayed in . pH value is about 7.2 in day 0, and then declines in day 1. From day 2 to day 8, pH keeps increasing. During storage, prawns undergo anaerobic glycolysis procedure. Glycogen degradation and lactic acid formation lead to the rapid decline of pH. When the prawns get deteriorated, trimethylamine oxide within the samples is reduced to trimethylamine with the help of microbes. The formation of trimethylamine leads to slight decline of pH. During further storage, protein within prawns decomposes and releases alkaline substances (ammonia and amines, etc.), which increases pH from day 2 to day 8.
TVB-N
The essential of prawn freshness changes is that the protein decomposes into various chemical substances and metabolites by function of microorganisms. TVB-N index effectively characterizes this decomposing procedure. TVB-N examination results are shown in . TVB-N increases gradually with the increase of storage days. The contents of TVB-N in the first 3 days are 16.50 mg/100 g, 18.60 mg/100 g, and 19.20 mg/100 g, respectively, which accords to China national standard (<20.00 mg/100 g).Citation16 The results demonstrate that prawns from day 0 to day 2 are fresh and edible. However, TVB-N content of prawns in day 3 is about 20.10 mg/100 g, which exceeds GB/T 5009.44-2003. After day 3, the content of TVB-N increases dramatically, and reaches 39.00 mg/100 g in day 8. The results indicate that prawns from day 3 to day 8 are not fresh.
TVC
Microbial propagation is a crucial factor influencing quality of aquatic products. During storage, prawns deteriorate gradually due to microbiology propagation. Microbiological metabolism leads to emission of feature gases, which provides measurement basis of e-nose analysis. So it is important to trace the microbiological propagation on prawns. TVC values of samples are monitored and displayed in . Initial TVC value is about 2.1×10Citation4 cfu•g−1. The TVC value increases dramatically fast after day 3. TVC value in day8 is about 2.2×107 cfu•g−1.
Human sensory evaluation
Sensory attributes of prawn samples were divided into 5 elements, whose preference scores were scored from 1 to 5; the higher preference score, the better quality of prawn (see ). All samples started with score of 5 in day 0.
Table 1. Sensory scheme for evaluating the jumbo squid during the refrigerated storage
In , preference scores decrease with the increase of storage days. Choosing 3.5 as the acceptable limitation, the prawns can maintain its shelf life by 3 days, and the preference score in day 3 is about 3.39. Sensory evaluation results accord to TVB-N results well. Combined with TVB-N and TVC examination results, sensory evaluation results might be affected by microbial activities and changes of prawns’ self components.
E-nose analysis results
Original responses and PCA results
In e-nose measurement, gas sensors are subjected to time drift. E-nose's stability is evaluated by conducting blank sample measurement. Zero gas, obtained by filtering air through active carbon, is used as blank sample. E-nose responses to blank sample are measured for 7 days. Blank sample measurement results demonstrate that e-nose responses to zero gas remain stable. Meanwhile, the sensors’ time drift during measurement has no effect to e-nose measurement. E-nose responses prawns are recorded. shows e-nose gas sensor responses on day 7. With the increase of measurement time, 8 sensors’ responses increase gradually. The responses of the sensors to sample's headspace gases are similar. Obvious increase occurs in the time interval between 2.5 s and 25 s. Finally, the responses trend to be stable. Sensor S4, S1, S5, S7, S6, S8, and S3 show high responsiveness to all the samples. S4 shows the largest responses. Responses of S2 are much smaller. Gas sensors within e-nose present various responses to prawns, which constructs the analysis basis of e-nose.
Figure 2. E-nose detecting results: (a) prawn sample measurement; (b) PCA result: PC1 VS. PC2; (c) PCA result: PC1, PC2 VS. PC3; (d) SR SNR spectrum; (e) DCSSR SNR spectrum; (f) freshness predicting model.
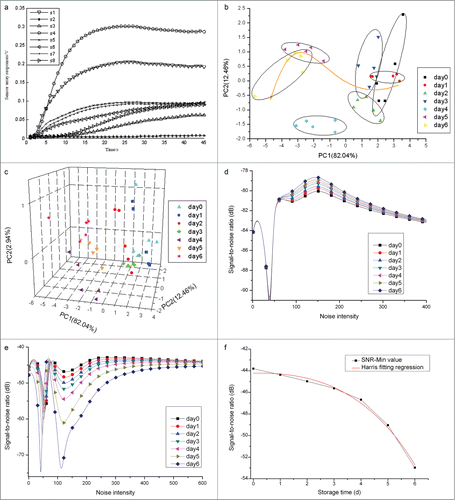
presents the PCA results. The first 2 principal components, PC1 and PC2, capture 94.5% of data variance. The figure shows the projections of PCA results on a 2-dimensional plane formed by the first 2 principal components. Combined with TVB-N examination results, prawns can be classed into 2 groups: fresh group and non-fresh group. Fresh group corresponds to the samples having undergone up to day 2. Non-fresh group corresponds to the samples from day 3 to day 6. From day 0 to day 2, the samples gather in the first and forth quadrant. Day 3 is the turning point. From day 3 to day 8, PC1 decreases quickly, and PC2 first decrease from day 3 to day 4. From day 4 to day 5, PC2 dramatically increases. In day 6, PC2 decrease slightly. However, Fresh and non-fresh samples have no obviously discriminating border. The samples under different storage time within the same group cannot be clearly discriminated from each other. PCA only provides a qualitative analysis on e-nose measurement data. PCA results on a 3-dimensional plane formed by the first 3 principal components are displayed in . The first 3 principal components, PC1 and PC2, capture 97.44% of data variance. All prawns under different storage time still cannot be totally discriminated from the 3-dimensional angle.
SR and DCSSR SNR spectrum
Prawn e-nose measurement data SNR spectrum calculated by SR as function of external white noise intensity is displayed in . A derivative vale arises at noise intensity of 37. Then SNR increases gradually with the increase of external noise intensity. Eigen peak appears at noise intensity of 65. The SNR maximal (SNR-Max) values range between −82 and −79 dB. SNR-Max values increase with the increase of storage time. Prawn samples under different storage days can be discriminated by SNR-Max values.
Prawn e-nose measurement data SNR spectrum calculated by DCSSR as function of noise intensity are displayed in . A derivative vale arises at noise intensity of 37. SNR increases gradually with the increase of stimulating noise intensity. A derivative peak appears at noise intensity of 74. Then SNR gradually decreases, and reaches its minimal value at noise intensity of 118. SNR-Min values range between −74 and −53 dB. SNR-Min values decrease with the increase of storage time. Prawns of different storage time can be clearly discriminated according to SNR-Min values. Compared with SR output SNR spectrum, DCSSR method especially improves output signal intensity, which contributes to prawn discrimination using SNR eigen values.
MVR between physical/chemical indexes and SR/DCSSR eigen values
Quality is a general description for prawns. The physical/chemical indexes, such as TVB-N, TVC, pH, firmness, have close relationship to prawns’ quality. MVR results between physical/chemical indexes and SR SNR-Max values are displayed as EquationEquation (1)(1)
(1) and .
indicates that SR eigen values have good linearity with prawn quality indexes. P < 0.05 and F = 77.1599, which indicate that SNR-Max values present significant linearity relation with physical/chemical indexes.
(1)
(1)
Table 2. Multiple variable regression between SR eigen values and physical/chemical indexes (F= 77.1599)
MVR results between physical/chemical indexes and DCSSR SNR-Min values are displayed as EquationEquation (2)(2)
(2) and .
demonstrates that DCSSR eigen values have better linearity with prawn quality indexes. P < 0.001 and F = 109.66052, which show that DCSSR SNR-Min values have more significant linearity relation with physical/chemical indexes than SR SNR-Max values. DCSSR SNR-Max values are more suitable for prawn freshness characterization.
(2)
(2)
Table 3. Multiple variable regression between DCSSR eigen values and physical/chemical indexes (F= 109.66052)
Freshness predicting model
shows the individual SNR-Min value of samples under different storage time. Prawn freshness forecasting model is established by non-linear Harris fitting regression of SNR-Min values. The fitting results are displayed as EquationEquation (3)(3)
(3) , and the regression coefficients R2 0.99375. After one-step's transform, EquationEquation (4)
(4)
(4) is used as freshness forecasting model. The input is e-nose measurement data DCSSR processed SNR-Min values, and the output is prawns’ prediction of storage time. According to EquationEquation (4)
(4)
(4) and physical/chemical examination results, we can achieve approximate quality of the measured samples. This method is suitable for most of samples following prawn freshness changing discipline. It is not suitable for quality forecasting of special samples.
(3)
(3)
(4)
(4)
Validation experiment results
Validating experiments are carried out to examine predicting accuracy of the developed model. EquationEquation (4)(4)
(4) is used to examine the freshness of prawns. Another 84 new prawns are prepared, and 35 samples are randomly selected for e-nose measurement. Results are displayed in . The forecasting accuracy of the predicting model is 94.29%. Validating experiment results demonstrate that the prawn forecasting model detects the prawns’ freshness with good accuracy.
Table 4. Freshness forecasting results (√ = right; x = wrong; / = not calculated)
Materials and Methods
Prawn samples
Fresh prawns, purchased from Wen’er aquatic market in Hangzhou, were transported to laboratory in ice box. 280 samples were prepared and packaged with sealing membrane and stored at 277 K for 7 days. Each sample weighs about 25 g. In each day, 40 samples were used for sensory preference, firmness, TVB-N, TVC examination, and e-nose measurement.
In validating experiments, 84 new prawns were selected and stored for 7 days. In each day, 12 samples were used for e-nose measurement. All samples were taken randomly.
Reagents and apparatus
NaOH was purchased from Sinopharm Chemical Reagent Co. Ltd., (Shanghai, China). Boric acid was purchased from Wuxi Jingke Chemical Co. Ltd., (Jiangsu, China). HCl was purchased from Hangzhou Chemical Reagent Co. Ltd., (Hangzhou, China). MgO was purchased from Tianjin Bodi Chemical Co. Ltd., (Tianjin, China). Methyl red and Bromocresol green were purchased from Shanghai SSS Reagent Co., Ltd., (Shanghai, China). Deionized water was used in all experiments. All chemicals were of analytically pure grade or better quality.
E-nose system
Prawn samples were measured by a portable e-nose. The structure is displayed in . It includes 3 main parts: data acquisition, modulating and transmitting unit (U1); sensor array and the chamber unit (U2); power and gas supply unit (U3). Gas sensor array consists of 8 metal oxide semiconductors (M.O.S) of different chemical compositions and thickness to provide selectivity toward different gases. The selectivity toward volatile compound classes of M.O.S sensors is indicated by the supplier: S1 (MQ-2, propane), S2 (MQ-3, ethanol), S3 (MQ-4, methane), S4 (MQ-5, propane, butane), S5 (MQ-6, butane), S6 (MQ-7, carbon monoxide), S7 (MQ-8, hydrogen), S8 (MQ-9, methane, carbon monoxide). The sensor response is expressed as sampling voltage (V). The M.O.S sensors rely on changes in conductivity induced by the adsorption of molecules in the gas phase and on subsequent surface reactions. They consist of ceramic substrate coated by metal oxide semiconducting film, and heated by wire resistor. Due to the high temperature (250–500°C), the volatiles transferred to the surface of the sensors are totally combusted to carbon dioxide and water, leading to a change in the resistance. The high temperature avoids water interference and provides sensors fast response and rapid recovery time. Polytetrafluoroethylene (PTFE) material is utilized to fabricate the chamber. Each sensor room is separated, which helps to avoid the cross-influence of the gas flow.
Methods
Firmness
TA.XT2i Texture Analyzer (Stable Micro Systems, UK) is used to conduct firmness measurement. Flat cylindrical probe p/5 (5 mm in diameter) was used. TPA mode was accepted. Speed in pre-measurement and after-measurement was 3 mm/s. Measurement speed was 1 mm/s. Compression degree was 50%. Residence time interval was 5 s. Load probe type was Auto-0.2 g. Data collection rage was 200.
pH
In pH measurement, 10 g prawn was smashed by a muller. Deionized water was added into the smashed sample to fully dissolve chemical substances. The sample and water were poured into conical flask. Muller was washed by deionized water, and washing liquid was also poured into conical flask. The mixture in the conical flask was finally diluted to 100 mL, and left to stand for a while. Then the mixture was filtered and measured by PHS-3C pH meter.
TVB-N
In TVB-N examination, magnesium oxide method was used in which contain (ammonia, mono-methyl amine, diethyl amine and tri-methyl-amine and other volatile amine). TVB-N examination was conducted by micro kieldahl apparatus (Hongji Instruments Company, Shanghai, China). The prawn meat was cut by pulper (Yide Electric Appliance Co., Ltd., Guangdong, China). According to China standard protocols GB/T 5009.44-2003 “Method for analysis of hygienic standard of meat and meat products,”Citation16 10 g sample was added to the heating flask containing 100 mL deionized water. After 30 minutes, the mixture was filtered. The filtrate was placed into refrigerator. 10 mL filtrate and 5∼6 drops of indicator were mixed and added into a heating flask. 5 mL of mixture and 5 ml magnesium oxide suspension (10 g/L) were added into distiller. After 5 minutes’ distill, hydrochloric acid solution (0.01 mol/L) was used for titration. The TVB-N was determined as follows:(5)
(5)
Where:
was the TVB-N index in mgN/100 g.
was the volume of hydrochloric acid used in sample titration in mL.
was the volume of control used in titration in mL.
was the concentration of hydrochloric acid in mol/L.
was the weight of sample in grams.
TVC
25 g of prawn meat was aseptically weighted and homogenized with 225 ml sterile 0.1% physiological saline for 1 min. The homogenized sample was serially diluted using 9 ml sterile saline for bacteriological analysis. TVC was determined in plate count agar by the spread plate method.Citation17
Sensory evaluation
According to previously reported method by Amerine et al.,Citation18 the sensory of prawn samples was evaluated by 6 experienced panelists (2 males and 4 females). Voting number is set at k, k ∈ (1,10). The quality of prawn is divided into m levels, and the score of a specific level is set at h, j ∈ (1, m). Prawn attributes are divided into n elements, and a specific element is set at ui, i ∈ (1,n). The contributory weight is determined by pairwise comparison of contribution weight of attributes is set at xi (∑xi = 1). If there is a specific relationship between 2 objects of h
and ui, the relation set (matrix) of f is calculated as follows:
(6)
(6)
Thus, the overall acceptability of prawn is calculated by the weight grade method as follows:(7)
(7)
E-nose measurement
In e-nose measurement, 25 g of samples were placed into 50 mL glass air-tight vials, and sealed with sealing membrane. The vials were stored at 277 K until analysis. The vials were equilibrated at room temperature for 45 min and analyzed in standardized conditions. After the power is turned on, washing pump and valve 2 are started, and sampling pump and valve 1 are close. The air is filtered by active carbon to obtain zero gas. Sensor array are washed by zero gas. When sensors’ response reach the baseline, the washing pump and valve 2 are closed. Then the sampling pump and valve 1 are started. The e-nose sucked the headspace gases of samples through the sensor array at 400 mL/min for 40 s. The measurement interval of e-nose was 0.05 s. When measurement is finished, the sensors are washed by zero gas for 600 s at a flow rate of 1000 mL/min prior to the next sample measurement.
Quality predicting method
SR
SR was proposed by Benzi to give an explanation for Earth climate periodical changes.Citation19-24 SR model can be described as follows:(8)
(8)
Where is the position of the Brownian particle,
is the time, M and C are adjustable parameters,
is an input signal
with an intrinsic noise
,
is the external noise, and
is the simplest double-well potential with the constants
and
.
(9)
(9)
EquationEquation (8)(8)
(8) can be transformed as
(10)
(10)
The minima of are located at
, where
. A potential barrier separates the minima with the height given by
. The barrier top is located at
. When three elements of SR interact coherently, the potential barrier can be reduced and the Brownian particle may surmount the energy barrier and enter another potential well. The intensity of signals will increase, which makes it possible that the weak signal can be detected from noise background.
Suppose the input signal is , where
is signal intensity,
is signal frequency.
is external noise intensity. SNR is the common quantifier for SR and it can be approximately described as:
(11)
(11)
Noise intensity is a parameter in SR model. This model is used for e-nose data analysis. denotes an input matrix. It has a sinusoid signal
, electronic nose response data
, and intrinsic noise
. SNR between the output and input is calculated. This model has been successfully used in food analytical applications.Citation19-22,25 The schematic diagram of SR is showed as .
DCSSR
Under adiabatic elimination condition, supposing signal amplitude is much smaller (A≪1), the Brownian particle is in one of potential wells because no enough driving energy is provided by the bistable system to drive the particle to jump from one potential well to another one in the absence of external noise. The signal period is longer than some characteristic intrawell relaxation time for the system. The existence of periodic forcing inclines the potential function, and forms the Brownian particle's transfer from one potential well to another one. So the potential function changes with the input signal and becomes
(12)
(12)
EquationEquation (12)(12)
(12) indicates the potential function gets time dependence. EquationEquation (13)
(13)
(13) displays the first-order and second-order derivation of
with respect to
, and let the equations equal to zero:
(13)
(13)
Setting noise intensity and
, the critical amplitude value of the periodic signal can be obtained:
. If
, the particle hovers around its original stable state and cannot jump from one potential well to another one. However, the particle can jump from one potential well to another one with the help of external noise even if
, which means the occurrence of SR phenomenon. We use fourth-order Runge-Kutta numerical algorithm to solve EquationEquation (13)
(13)
(13) :
(14)
(14)
(15)
(15)
(16)
(16)
(17)
(17)
(18)
(18)
is the nth numerical value of
, and
is the nth numerical value of
.
is the computation step. Much progress has been achieved on the applications of SR in the past few decades.
Single SR system connected in series forms the cascaded SR to obtain DCSSR (see ). According to EquationEquation (10)(10)
(10) , the relative Langevin equations of the cascaded bistable systems can be respectively written as:
(19)
(19)
In practical engineering measurement, measured data usually consists of signal and intrinsic noise. If an aimed weak signal is submerged in strong noise, we are not able to detect it. With the help of SR, the energy of intrinsic noise is lowered, and the embedded weak signal is amplified effectively so that the signal can be caught for measurement characterization. In some special measurement, the weak signal is so weak that the aimed signals are still embedded in the noise. DCSSR is designed to solve this problem. For a DCSSR system, several bistable systems are in serial. If the measurement signals are still embedded in the noise, the output of the former bistable system is taken as the latter bistable system. If the aimed signals are successfully obtained, the current number of bistable systems is the suitable for the information measurement. The schematic diagram of DCSSR is showed as .
Mechanism analysis of DCSSR
From above results, external white noise plays an important part in e-nose measurement data feature extraction. What is acting mechanism between external nose and weak feature signal? How can e-nose signals be effectively strengthened? Here, the weak signal amplification efficiency is theoretically evaluated. According to EquationEquation (9)(9)
(9) , EquationEquation (8)
(8)
(8) can be obtained:
(20)
(20)
In SR analysis, we suppose the initial intrinsic noise signal is twice the e-nose signal
strength. Then
. In DCSSR analysis, if the intrinsic noise signal can be reduced to 0.15 times the e-nose signal
, then
.
is defined as the signal amplification coefficient, and
. After simplification by
and
, EquationEquation (21)
(21)
(21) can be obtained:
(21)
(21)
According to EquationEquation (19)(19)
(19) , the limits of signal amplification coefficient
in theory, which demonstrates that the e-nose signal can be tremendously amplified in practical measurement even if the intrinsic noise
is faced to a minor reduction. As a non-linear signal processing method, SR transfers intrinsic noise energy into the weak input signal. So the input weak signal is strengthened and the intrinsic noise is effectively reduced. For prawn samples with different freshness, e-nose feature measurement information is easily submerged into the interfering signals induced by the interactions between other gases and gas sensor array. E-nose feature information gets too weak to be presented in the experiments when using SR. DCSSR analysis relies on the effective combination of 2 or more SR procedures. In this study, 2 SR models are cascaded in serial successively. Intrinsic noise can be tremendously reduced and e-nose feature information can be effectively amplified via DCSSR method.Citation25 Experimental results demonstrate that DCSSR method is more effective than SR method in prawn freshness determination utilizing e-nose.
Conclusions
In this paper, penaeus orientolis prawn freshness rapid determination using e-nose and non-linear SR technique is proposed. The results are listed in the following:
E-nose responses to prawn samples stored at 4°C under different storage time are measured. Physical/chemical indexes (firmness, pH, TVB-N, TVC, and human sensory evaluation) are examined to provide freshness references for e-nose analysis.
Firmness and sensory preference scores increases with the increase of prawn storage time. However, pH, TVB-N, and TVC indexes increases during experimental procedure. According to standard protocols, that prawns from day0 to day 2 are fresh and the rest samples are not fresh.
E-nose measurement data is processed by PCA, non-linear SR and DCSSR methods. PCA cannot discriminate prawns. SR/DCSSR SNR spectrum eigen values successfully discriminate all prawns.
MVR regression is conducted between SR/DCSSR output eigen values and physical/chemical indexes. MVR results demonstrate that DCSSR SNR-Min values have more significant linearity relationship with physical/chemical indexes.
Penaeus orientolis prawn freshness forecasting model
is developed via non-linear Harris fitting regression on DCSSR SNR-Min values. Validating experimental results demonstrate that the forecasting accuracy of the developed predicting model is 94.29%.
Aquatic products contain rich nutrition, but they deteriorate easily. According to food quality examination standards in operation, the analysis procedure is fussy and time-consuming. We are carrying further investigations on applications of e-nose technique in food quality rapid analysis.
Disclosure of Potential Conflicts of Interest
No potential conflicts of interest were disclosed.
Funding
This work is financially supported by National Spark Technology Project (Grant No. 2013GA700187), National Natural Science Foundation of China (Grant No. 81000645), Higher Education Research Project of Zhejiang Gongshang University (Xgy13080), Student Innovation Projects of Zhejiang Gongshang University (13-157, 13-158, 14-166, 14-193).
References
- Kimiya T, Sivertsen A, Heia K. VIS/NIR spectroscopy for non-destructive freshness assessment of Atlantic salmon (Salmo salar L.) fillets. JFood Eng 2013; 116:758-64
- Zhang Z, Li G, Luo L, Chen G. Study on seafood volatile profile characteristics during storage and its potential use for freshness evaluation by headspace solid phase microextraction coupled with gas chromatography–mass spectrometry. Anal Chim Acta 2010; 659:151-8; PMID:20103118; http://dx.doi.org/10.1016/j.aca.2009.11.024
- Pérez-Esteve E, Fuentes A, Grau R, Fernández-Segovia I, Masot R, Alcañiz M, Barat JM. Use of impedance spectroscopy for predicting freshness of sea bream (Sparus aurata). Food Control 2014; 35:360-5; http://dx.doi.org/10.1016/j.foodcont.2013.07.025
- Heising J, Bartels P, van Boekel M.A.J.S., Dekker M. Non-destructive sensing of the freshness of packed cold fish using conductivity and pH electrodes. J Food Eng 2014; 124:80-5.
- Cheng J, Sun X, Zeng H. Non-destructive and rapid determination of TVB-N content for freshness evaluation of grass carp (Ctenopharyngodon idella) by hyperspectral imaging. Innovative Food Sci Emer Tech 2014; 21:179-87
- Peris M, Escuder-Gilabert L. A 21st century technique for food control: electronic noses. Anal Chim Acta 2009; 638:1-15; PMID:19298873; http://dx.doi.org/10.1016/j.aca.2009.02.009
- Hui GH, Wang LY, Mo YH, Zhang LX. Study of grass carp (Ctenopharyngodon idellus) quality predictive model based on electronic nose. Sensor Actuat B-Chem 2012; 166-167:301-8
- Hammond J, Marquis B, Michaels R, Oickle B, Segee B, Vetelino J, Bushway A, Camire ME, Davis-Dentici K. A semiconducting metal-oxide array for monitoring fish freshness. Sensor Actuat B-Chem 2002; 84:113-22; http://dx.doi.org/10.1016/S0925-4005(02)00011-4
- Zhang L, Tian F, Pei G. A novel sensor selection using pattern recognition in electronic nose. Measurement 2014; 54:31-39; http://dx.doi.org/10.1016/j.measurement.2014.04.005
- Gredilla A, Fdez -Ortiz de Vall ejuelo S, Amigo J, de Diego A. Madariaga J. Unsupervised pattern-recognition techniques to investigate metal pollution in estuaries. Trend Anal Chem 2013; 46:59-69, http://dx.doi.org/10.1016/j.trac.2013.01.014
- O’Connell M, Valdora G, Peltzer G, Negri RM. A practical approach for fish freshness determinations using a portable electronic nose. Sensor Actuat B-Chem 2001; 80:149-54; http://dx.doi.org/10.1016/S0925-4005(01)00904-2
- Barbri NE, Amari A, Vinaixa M, Bouchikhi B, Correig X, Llobet E. Building of a metal oxide gas sensor-based electronic nose to assess the freshness of sardines under cold storage. Sensor Actuat B-Chem 2007; 128:235-44; http://dx.doi.org/10.1016/j.snb.2007.06.007
- Limbo S, Sinelli N, Torri L, Riva M. Freshness decay and shelf life predictive modelling of European sea bass (Dicentrarchus labrax) applying chemical methods and electronic nose. LWT - Food Sci Tech 2009; 42:977-84; http://dx.doi.org/10.1016/j.lwt.2008.12.011
- Zaragozá P, Fuentes A, Fernández-Segovia I, Vivancos J, Rizo A, Ros-Lis JV, Barat JM, Martínez-Máñez R. Evaluation of sea bream (Sparus aurata) shelf life using an optoelectronic nose. Food Chem 2013; 138:1374-80; PMID:23411257; http://dx.doi.org/10.1016/j.foodchem.2012.10.114
- Zaragozá P, Fernández-Segovia I, Fuentes A, Vivancos JL, Ros-Lis JV, Barat JM, Martínez-Máñez R. Monitorization of Atlantic salmon (Salmo salar) spoilageusing an optoelectronic nose. Sensor Actuat B-Chem 2014; 195:478-85; http://dx.doi.org/10.1016/j.snb.2014.01.017
- GB/T 5009.44-2003. Chinese national standard, Method for analysis of hygienic standard of meat and meat products. Beijing. PRC, 2003
- GB/T 4789.2-2010. Chinese national standard, Food microbiological examination: Aerobic plate count. Beijing. PRC, 2010
- Amerine MA, Pangborn RV, Roessler EB. Principles of sensory evaluation of food. Academic Press, New York, USA 1965; 602
- Benzi R, Sutera A, Vulpiana A. The mechanism of stochastic resonance. J Phys A 1981; 14:L453-6; http://dx.doi.org/10.1088/0305-4470/14/11/006
- Gammaitoni L, Hanggi P, Jung P, Marchesoni F. Stochastic resonance. Rev Modern Phys 1998; 70:223-87
- Dutta R, Das A, Stocks NG, Morgan D. Stochastic resonance-based electronic nose: A novel way to classify bacteria. Sensor Actuat B-Chem 2006; 115:17-27; http://dx.doi.org/10.1016/j.snb.2005.08.033
- Hui GH, Mi SS, Deng SG. Sweet and bitter tastants specific detection by the taste cell-based sensor. Biosens Bioelectron 2012; 35:429-38; PMID:22424755; http://dx.doi.org/10.1016/j.bios.2012.02.028
- Hui G, Ji P, Mi S, Deng S. Electrochemical impedance spectrum frequency optimization of bitter taste cell-based sensors. Biosens Bioelectron 2013; 37:164-70; PMID:23578970; http://dx.doi.org/10.1016/j.bios.2013.03.018
- Hui G, Mi S, Chen Q, Chen X. Sweet and bitter tastant discrimination from complex chemical mixtures using taste cell-based sensor. Sensor Actuat B-Chem 2014; 35:301-8
- Hui G, Jin J, Deng S, Ye X, Zhao M, Wang M. Winter jujube (Zizyphus jujuba Mill.) quality forecasting method based on electronic nose. Food Chem 2015; 170:484-91; PMID:25306374; http://dx.doi.org/10.1016/j.foodchem.2014.08.009