Abstract
Experimental techniques allow engineering of biological systems to modify functionality; however, there still remains a need to develop tools to prioritize targets for modification. In this study, agent-based modeling (ABM) was used to build stochastic models of complexed and non-complexed cellulose hydrolysis, including enzymatic mechanisms for endoglucanase, exoglucanase, and β-glucosidase activity. Modeling results were consistent with experimental observations of higher efficiency in complexed systems than non-complexed systems and established relationships between specific cellulolytic mechanisms and overall efficiency. Global sensitivity analysis (GSA) of model results identified key parameters for improving overall cellulose hydrolysis efficiency including: (1) the cellulase half-life, (2) the exoglucanase activity, and (3) the cellulase composition. Overall, the following parameters were found to significantly influence cellulose consumption in a consolidated bioprocess (CBP): (1) the glucose uptake rate of the culture, (2) the bacterial cell concentration, and (3) the nature of the cellulase enzyme system (complexed or non-complexed). Broadly, these results demonstrate the utility of combining modeling and sensitivity analysis to identify key parameters and/or targets for experimental improvement.
Introduction
Parallel advances in numerous fields (e.g., genomics, meta-genomics, bioprospecting, synthetic biology) have rapidly increased the knowledge and experimental tools available for biological research. When used in combination, fundamental knowledge can direct experiments to improve or modify native biological function.Citation1 Practically, this typically involves iterations between analyzing the known information, experimentally implementing a best guess, and adding to the knowledge pool through the generation of experimental results. For complex systems with a large number of variables, it is often difficult to even formulate what the initial best guess is to explore for the system. This is true for cellulose hydrolysis where native cellulolytic systems are highly variable between different cellulolytic organisms.Citation2 Conceptually, this study focuses on developing and demonstrating a computational approach to aid in the analysis of cellulolytic systems to identify key parameters that influence the efficiency of cellulose hydrolysis.
Identification of cellulase expression systems
Cellulolytic bacteria express and secrete cellulase enzymes to hydrolyze cellulose into consumable sugars.Citation3-Citation7 Two distinctly different enzyme systems have been identified for the production and secretion of cellulases for extracellular cellulose degradation: (1) complexed and (2) non-complexed.Citation6,Citation8 Organisms with a complexed hydrolysis system form a cell wall-bound extracellular structure called a cellulosome. Enzymes required for cellulose hydrolysis such as (1) endoglucanases, (2) exoglucanases, and (3) β-glucosidase are localized in the cellulosome.Citation3-Citation5,Citation8,Citation9 These enzymes have different hydrolysis mechanisms and act at specific sites within cellulose chains. Endoglucanases cut the amorphous sites in the middle while exoglucanases act on the crystalline reducing and non-reducing ends. Beta-glucosidases hydrolyze smaller cellulose chains (e.g., cellobiose) into individual monosaccharides.Citation6,Citation7 Under the non-complexed mechanism, cellulases are secreted into the outer cellular matrix (unbound to a cellulosome structure) to facilitate cellulose hydrolysis. Usually, aerobic organisms such as Acidothermus cellulolyticus (ATCC 43068), Cellulomonas flavigena (ATCC 482), and Thermobifida fusca (ATCC 27730) (among others) exhibit the non-complexed hydrolysis mechanism while anaerobic organisms such as Clostridium thermocellum (ATCC 27405), C. cellulolyticum (ATCC 35319), and Fibrobacter succinogenes (ATCC 19169) employ the complexed cellulose hydrolysis strategy. Recent research has suggested that organisms having a complexed cellulose hydrolysis enzyme system can degrade cellulose more efficiently than organisms with the non-complexed system.Citation4 This is primarily attributed to the synergistic mechanisms of different cellulases inside the cellulosome structure. The close physicality demonstrated by tightly packed enzyme clusters of the cellulosome may enable organisms with a complexed system to achieve better efficiency of degrading cellulose.Citation8 A third type of cellulase enzyme system also exists and is termed a “hybrid” system. In a hybrid system, both the complexed and non-complexed cellulose hydrolysis mechanisms are active simultaneously. For example, C. thermocellum ATCC 27405, has been shown to secrete two non-cellulosome bound cellulases in addition to expressing a cellulosome.Citation10
Advances in recombinant DNA technology have enabled the development of efficient and scalable expression systems to produce cellulase enzymes using more traditional industrial organisms such as Escherichia coli,Citation11,Citation12 Bacillus subtilis,Citation13 Saccharomyces cerevisiae,Citation14 Pichia pastoris,Citation15 Streptomyces lividans,Citation16 and species of Aspergillus and Trichoderma.Citation17 Cellulases originating from these organisms have a wide range of applications in the biotechnology industry. While some of the most important applications of cellulolytic enzymes from microorganisms are in food, brewing and wine making, animal feed, textile and laundry, pulp and paper industries, and agriculture,Citation18 ultimately a major goal of industrial biotechnology involves using renewable cellulose as the primary carbon source for fuels and chemicals.Citation5,Citation6,Citation17,Citation19-Citation24
Recent metabolic engineering approaches
Recent advances in metabolic engineering have uncovered several novel possibilities of engineering cellulase enzymes for use on the industrial scale. Techniques such as (1) high-throughput screening of microorganisms, (2) identification of novel cellulases, (3) enzyme engineering through site-directed mutagenesis, and (4) fermentation process optimization are all suggested to enhance the enzymatic activity and cellular production of desired cellulases.Citation19,Citation25 The enzymatic breakdown of cellulosic materials by cellulases for biofuel production is perhaps the most popular application currently being investigated. Conversion of lignocellulosic materials into value-added products (including ethanol) is a multistep process. These processing steps include: (1) pretreatment (mechanical, chemical, or biological), (2) hydrolysis of the complex polymers to produce monosaccharides, (3) bioconversion of hexose and pentose sugars to support microbial growth and production of biochemical products, and (4) the separation and purification of the desired products.Citation18,Citation21,Citation23,Citation26 Reducing the cost of production, improving cellulase performance, and increasing sugar yields are important considerations for increasing industrial production using microorganisms. Considerable efforts are under way to improve specific cellulase activities for non-complexed cellulase cocktails. These are often implemented through cellulase enzyme engineering based on rational design and/or directed evolution for each cellulase component.Citation27,Citation28 As traditional biomass conversion processes are multistep and challenging economically, consolidated bioprocessing (CBP) has received much of the current research focus.Citation7,Citation20,Citation24 But, the identification or engineering of microorganisms capable of performing single-stage cellulose hydrolysis and simultaneous fermentation of liberated hexose and pentose sugars has proven challenging. To improve overall efficiency, enzyme engineering of cellulases is considered a vital step toward engineering recombinant microorganisms suitable for CBP. The heterologous expression of cellulasesCitation29 is central to recombinant cellulolytic strategies, enabling microorganisms to exhibit high product yields and titers.Citation30 In addition, efforts to detect new enzymes with cellulase activity under adverse conditions are also under way.Citation22,Citation23,Citation26 However, whether these native or engineered cellulase enzymes should be expressed as part of a (1) complexed, (2) non-complexed, or (3) hybrid expression system has not been addressed, until this research through a unique modeling approach.
Agent-based modeling
In an agent-based modeling (ABM) system, an “agent” represents each component of the system that executes different behaviors according to deterministic rules that govern movement and interactions in the simulation matrix.Citation31-Citation33 Depending on the biological system being studied, an agent could be (1) a cell, (2) an enzyme or macromolecule, (3) a mitochondria, (4) a tissue, or (5) an entire organ or organism. In a two-dimensional ABM, the simulation matrix can be divided into a number of M × N smaller cells. These cells act as a coordinate system to measure the location of agents at any given instance of the simulation. Each iteration (or step) of the simulation may consider specific unit movement of individual agent to occupy a cell within its von Neumann neighborhood. This is shown in . This neighborhood is described as four cells orthogonally surrounding a central cell on a two-dimensional square lattice. In an ABM representing Michaelis-Menten kinetics, enzymatic reactions occur with a pre-determined probability if enzyme and substrate occupy neighboring cells. These local interactions mimic biochemical reactions performed under the close physical proximity present in real biological systems. By simulating the ABM over a large number of iterations, emergent behavior of the system (e.g., diffusion, enzymatic conversion, and cell growth) can be studied as a function of time given a set of physical parameters for each agent. In this research, agents are governed by potentially engineered parameters (e.g., cellulase half-life, expression system, enzyme production rate, among others discussed below). The ABM approach was used in this research to assess the rate of cellulose degradation and consumption in a closed system, given several different sets of physical parameters.
Global sensitivity analysis
Global sensitivity analysis (GSA) uses Monte Carlo simulations to calculate the outputs of a model over the entire range of all input parameters.Citation34,Citation35 This variance-based method calculates the contribution of each input parameter to the total variability of the model output. GSA differs significantly from the traditionally used method of partial gradient-based sensitivity analysis (SA). With traditional SA, the change in a model output is calculated by allowing only one parameter to vary, while keeping others constant. This variance in the model output is likely to change if all other parameters are held constant at different values. The GSA approach takes this into account and enables the consideration of multiple parameters simultaneously. To consider only a single parameter is a “first-order” analysis. Two parameters may be considered simultaneously to develop a measure of their interactions. Or, a single parameter can be considered with is interactions with all other parameters in the model. This is called the “total effect index.”Citation34,Citation35 Thus, the primary advantage of GSA over SA is that GSA accounts for the influence and interactions between input parameters over the entire input space. A simplified tutorial of GSA has been published for a deterministic ammonia emissions model.Citation36 In this research, GSA was performed to identify parameters that most significantly affect the degradation of cellulose and utilization of liberated sugars in a CBP. This study not only identifies previously known areas of significance but also sheds light on some obscure parameters affecting cellulose hydrolysis, providing novel guidelines for future experimental designs.
Identifying new metabolic engineering targets through agent-based modeling
Agent-based modeling was used in this research to effectively compare the efficiencies of (1) complexed, (2) non-complexed, and (3) hybrid cellulase expression systems. This analysis was also accompanied by an analysis of cellulase enzyme composition. Here, the composition of a cellulase enzyme cocktail, consisting of three primary cellulases: (1) endoglucanase, (2) exoglucanase, and (3) β-glucosidase, was varied to determine the impacts on cellulose hydrolysis rates in all three cellulase expression systems studied. This research was performed to investigate the hypothesis that complexed cellulase expression and cellulose hydrolysis mechanism is most efficient when compared with the non-complexed and hybrid systems. The agent-based modeling methods were then combined with GSA in effort to identify new metabolic engineering targets. While considerable research efforts are focused on cellulase enzyme engineering, the results of this research identified additional targets that may be metabolically engineered to improve the overall performance of the CBP.
Results and Discussion
Comparing cellulase enzyme expression systems
In formulating the ABM system for simulations of cellulose hydrolysis, it was found that different concentrations of bacterial cells introduced variability in the model output, making comparisons difficult. This is shown in . As the number of bacteria in the system was increased, the number of simulation steps required for cellulose hydrolysis and sugar consumption decreased and had a much smaller variance. This made the model more predictable and required less computational resources for each simulation run. Thus, a fixed starting concentration of at least 60 bacteria cells was required in the system. Above this threshold, the variance of the model reduced to be close to zero, as shown in . Using ABM, the following hypotheses were tested: (1) there is no difference between cellulose hydrolysis given complexed and non-complexed cellulase expression systems and (2) there is no difference between cellulose hydrolysis given complexed and hybrid cellulase expression systems. The ABM simulations were run for a total of 200 Monte Carlo iterations for each expression system. The standard student’s t test was used to compare the means of the different expression systems, assuming unequal variances and an α of 0.05. The results for t test are presented in . It is evident from the t test results that differences observed between the simulated hydrolysis efficiencies of the complexed and non-complexed systems are statistically significant. However, those observed between the complexed and hybrid systems was not found to be statistically significant. These results ultimately infer that complexed cellulose hydrolysis is more efficient compared with non-complexed cellulose hydrolysis. According to these calculations, this overall difference in efficiency is about 33%. These results may also indicate that having secreted cellulase enzymes in addition to a complexed cellulose hydrolysis system may not be beneficial to the organism. However, this is still under investigation because hybrid cellulose hydrolysis systems exist in nature (e.g., C. thermocellum). This means the hybrid system must convey a competitive advantage in a particular environment. However, this was not identified in the simulations performed in this research.
Figure 2. Number of iterations required for cellulose hydrolysis and sugar consumption for 200 independent simulations given bacteria cell density values ranging between 10 and 100 cells per simulation. Large variance was calculated at low cell densities.
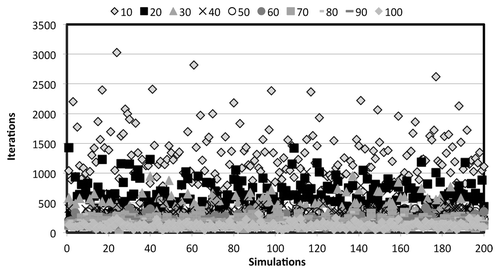
Figure 3. Variance of cellulose hydrolysis and sugar consumption over 200 iterations as a function of the bacteria cell density (number of cells) for the three different cellulase enzyme expression systems.
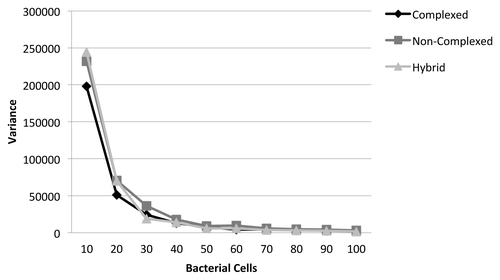
Table 2. The t test results comparing cellulase expression systems
Optimizing cellulase ratios
Previously published dataCitation37-Citation40 shows that the amount of β-glucosidase in the total cellulase enzyme composition (consisting of exoglucanases, endoglucanases, and β-glucosidase) is usually less than 20%. In this research, three different series of ABM simulations were performed by reducing the concentration of β-glucosidase from 20 to 0 in steps of 10. The concentrations of exoglucanases and endoglucanases were modulated. Results are shown in . The efficiency of cellulose hydrolysis increased as a result of increasing the concentrations of endoglucanase and decreasing concentrations of exoglucanase, given a constant β-glucosidase concentration. In addition, reducing β-glucosidase content, while maintaining a constant exoglucanase to endoglucanase ratio, showed to increase cellulose hydrolysis efficiency. This indicates a central role for endoglucanases in the cellulose hydrolysis process and suggests that a higher endoglucanase concentration leads to greater efficiency. However, the role of synergy is critical, as simulation results have also shown a cellulase cocktail will out-perform a single cellulase. Endoglucanases cut the cellulose chains in the middle making more reducing and non-reducing ends available for exoglucanases and smaller chains to β-glucosidase. This explains the increase in efficiency by increasing endoglucanase composition in the cellulase system. It has been shown recently that the endoglucanase is more important to the cellulase mixture when hydrolyzing cellulose with higher degree of polymerization.Citation41 An upper bound on the concentration of endoglucanase in the enzyme cocktail has been suggested due to the balancing act of exoglucanases as more chain ends become available. The model presented in this research does predict this upper bound on endoglucanase concentration and the balancing act of the endo-exo- synergy is evident in .
Global sensitivity analysis (first-order indices)
The first-order indices for each parameter are reported in as a percentage of the summation of all first-order index values. The three most significant parameters identified by the first-order index are (1) exoglucanase composition (9.29%), (2) enzyme half-life (11.16%), and (3) exoglucanase activity (12.78%). All three of these parameters are related to cellulases, and two specifically single-out the exoglucanase. In the previous section on optimizing cellulase ratios, it was revealed that increasing exoglucanase composition (at the expense of endoglucanase and β-glucosidase) negatively affects hydrolysis efficiency. The GSA results indicate that exoglucanase composition is responsible for about 9.29% of the cellulose hydrolysis efficiency variation, while exoglucanase activity is responsible for additional 12.78% of the variance. Thus, these results suggest that the exoglucanase composition of the cellulase is an important determinant of cellulose hydrolysis efficiency. Of course, this was confirmed by the previous set of simulations that showed minimizing the exoglucanase composition (while increasing the composition of endoglucanase) was an effective strategy for improving cellulose hydrolysis efficiency. However, exoglucanase activity was shown to account for the most first-order model variance of any parameter. Given a constant composition, increased exoglucanase activity would increase cellulose hydrolysis efficiency. Thus, in addition to optimizing cellulase composition, increasing the activity of the exoglucanase should be seen as a worthwhile enzyme engineering goal. In addition, enzyme engineering to increase the half-lives of all cellulases is also a worthwhile goal, but this result is more intuitive.
Global sensitivity analysis (total effect indices)
The GSA total effect indices are shown in and identified (1) glucose uptake (7.75%), (2) bacterial cell density (7.61%), and (3) the cellulase expression enzyme system (7.13%) as the three most significant parameters affecting cellulose hydrolysis. When interactions with other parameters are considered, the glucose uptake rate is the most important parameter identified by GSA to impact the rate of cellulose hydrolysis. Thus, when considering optimizing a CBP, it is important to not only hydrolyze cellulose efficiently but also to uptake and convert liberated sugars effectively. Thus, further optimization of glucose uptake systems can be considered a means of improving the performance of a CBP. The second most important parameter identified by the GSA total effect index was the bacterial cell density. This result is consistent with the initial findings of this study, as shown in , that varying the number of bacterial cells in the system can greatly impact the model output. Engineering greater cell densities is an intuitive means of improving the performance of a CBP. Finally, the type of enzyme system itself (complex, non-complexed, or hybrid) was also identified by GSA (correctly) as a source of great variance in the model output. This GSA result reinforced the motivation behind this study that the type of cellulase expression system used is critical to cellulose hydrolysis efficiency. The results of ABM have shown that the complexed system is statistically more efficient than the non-complexed cellulase expression system.
Using ABM and GSA results to guide metabolic engineering efforts
In this study, GSA has revealed important aspects of the cellulose hydrolysis process. Six parameters significantly affecting the hydrolysis were identified and can all be identified as valuable targets for enzyme and metabolic engineering. From these, the exoglucanase enzyme is of particular interest as both its activity and composition significantly affects cellulose hydrolysis efficiency. Modeling results suggest enzyme engineering efforts focused on improving the catalytic action of exoglucanase enzymes would be valuable while metabolic engineering strategies to optimize the exoglucanase to endoglucanase ratio is another area of importance. Few efforts to optimize these ratios are currently under wayCitation41,Citation42 though more efforts are anticipated in the future. Improving enzyme half-life is another area of great significance. Recent efforts in cellulase engineering have yielded cellulases with higher half-lives.Citation43-Citation48
Part of the benefit of a comprehensive computational analysis is to equally consider the effects of all possible variables within a system. There have been a number of studies seeking to improve cellulolytic efficiency, but much of this research is heavily skewed to focus on enzyme engineering. A PUBMED literature search (shown in ) was performed to illustrate that far more research to-date has been conducted in enzyme engineering (41 210 articles published to date) than in other identified areas (glucose transport, cell concentration) that can improve cellulolytic efficiency. Thus, the combination of ABM and GSA has been used in this case to identify critical potential metabolic engineering targets for optimizing a CBP that are relatively under-researched compared with cellulase enzyme engineering efforts.
Table 3. Number of articles published (as of January 2012) on topics related to improving a CBP
Conclusions
The focus of this computational research was to compare the efficiency of cellulose hydrolysis given different cellulase expression systems. The use of ABM and GSA also allowed the identification of the system parameters that significantly impact cellulose hydrolysis efficiency in a CBP. The randomness introduced by a sparsely populated modeling environment was addressed by assuming sufficient concentrations of bacterial cells. This variability was attributed to physical distances between interacting components of the system at the beginning of the simulation. The ABM predicts that a complexed (cellulosome bound) cellulase expression and cellulose hydrolysis mechanism is statistically different from a non-complexed (secreted) cellulase expression system with about a 33% increase in efficiency. The differences observed between the complexed and hybrid systems were not statistically significant, implying that additional cellulosome-free secreted cellulases may not be advantageous to a strictly complexed cellulase expression system.
From the ABM results of testing different cellulase ratios, it was concluded that the composition of endoglucanase in the system is directly proportional to the efficiency of hydrolysis while exoglucanase and β-glucosidase concentrations are inversely proportional. This can be explained by the fact that by randomly hydrolyzing the amorphous sites in the middle of a cellulose chain, endoglucanases are producing a greater number of shorter cellulose chains and exposing more reducing and non-reducing cellulose ends in the process, contributing to a higher cellulose hydrolysis efficiency.
The results of the GSA study revealed the importance of the several parameters affecting the cellulose hydrolysis process. Enzyme engineering was found to be a significant aspect of cellulose hydrolysis, as indicated by first-order indices of the GSA. However, while increasing the half-life of cellulase enzymes is an intuitive finding, the importance of the exoglucanase activity is less intuitive. These results correlate well with the amount of research currently focused in these areas. Important targets for metabolic engineering were identified as well. First, the production of optimized ratios of endoglucanases to exoglucanases was found significant according to first-order results. The glucose uptake rate was found to be a highly significant aspect of cellulose hydrolysis when total effect indices of the GSA were considered. Research determining the effects of substrate uptake on hydrolysis efficiency has received significantly less attention in the recent literature compared with cellulase enzyme engineering. Thus, the approach of ABM and GSA provides a valuable tool for the identification of new engineering targets. In the case of the CBP, all model parameters showed to contribute to the variance in cellulose hydrolysis efficiency. While these are all important and can yield an improved process if engineered, those parameters with higher sensitivities can yield greater returns if optimized and may represent better engineering targets for the near term.
Overall, the combined modeling approach of agent-based modeling and global sensitivity analysis was able to simulate the mechanisms and physicality of the cellulolse hydrolysis process. Key variables affecting the efficiency of cellulose hydrolysis were identified to suggest future avenues for experimental engineering to improve cellulolytic systems. Additionally, the modeling approach taken appears well-suited for studying biological processes, particularly for its ability to account for stochastic processes and physical biological constraints. AGM and GSA may be applied to ranges of problems from molecular binding events to macroscopic community dynamics (e.g., growth and crashes in large-scale algae ponds or microbial consortia).
Methods
Agent-based model construction
Agent-based modeling was used to study cellulose hydrolysis. The model was built using Netlogo software, version 4.1RC5 (October 13, 2009).Citation49 The agents of the system included: (1) bacteria, (2) cellulose, and (3) cellulase enzymes (produced by the bacteria). The individual parameters that defined the agents were varied and are given in . These parameters consisted of: (1) the cellulase enzyme production rate (by the bacteria); (2) the composition of exoglucanases, endoglucanases, and β-glucosidase; (3) the cellulase enzymatic activity and half-life; (4) the cellulase enzyme expression system; (5) the cellular uptake rates of glucose, cellobiose, and cellotriose sugars; (6) the concentration of bacteria cells present in the system; and (7) the concentration and average length of the cellulose chains present. These parameters are discussed in detail below.
Table 1. ABM parameters, bounds, and descriptions
To demonstrate the ABM method, six simulation steps involved in the enzymatic hydrolysis reaction are given in .Citation50 Here, the parameters S, P, E, SE, and PE stand for substrate, product, enzyme, substrate-enzyme complex, and product-enzyme complex, respectively. The probability of S, P, and E occupying one of their neighboring cells is 0.25. When S and E occupy neighboring cells, they can interact to form SE. A PE complex can form in similar way. The transitional probability for the substrate-enzyme complex, SE(i), to produce product-enzyme complex, PE(i), is assumed to be 0.5. It can also dissociate into S and E with a 0.5 probability, suggesting a reversible reaction. Differences in the transport (by diffusion) of cells, cellulose, and cellulase enzymes in the closed system, arising due to their respective sizes, were given special consideration. Diffusion constants for cellulose chains in aqueous media were set inversely proportional to the length of chain. Thus, a smaller chain was allowed to diffuse faster than a larger chain. Each simulation consisted of complete breakdown of all cellulose chains and cellular uptake of all liberated sugars. The number of steps (see ) required to complete each simulation was recorded for each set of parameters tested. Results for each set of parameters were averaged over 200 independent simulations. The average number of steps required for each case ultimately determined the efficiency of cellulose hydrolysis.
Agent-based modeling parameters
The following parameters were taken into consideration while building and simulating the model. The upper and lower bounds on the parameters and their functionalities are listed in . The parameters used in this modeling effort are described in detail below.
(1) Cellulase enzyme production rate (by the bacteria)
The rate at which enzymes are released outside the bacterial cell was controlled using the EnzymeProductionRate parameter for the non-complexed cellulase expression system. Similarly, the EnzymeProductionRateH parameter was used to control enzyme production rate for the hybrid system. These rates were expressed in terms of percentage probability between 1 and 100, with 100 representing the maximum production rate. The only known hybrid system to secret two non-cellulosome bound cellulases is C. thermocellumCitation10 Other organisms using a non-complexed system secrete about 20 different cellulases, on average. So, for this hybrid system, the secreted cellulase enzyme production rate was set to 10% of that of the other non-complexed systems.
(2) Composition of exoglucanases, endoglucanases, and β-glucosidase
The proportion of individual cellulases (exoglucanases, endoglucanases, and β-glucosidases) relative to the total amount of cellulase enzyme present in system was set using the CompositionExo, CompositionEndo, and CompositionGluco parameters. These parameters fix the percentage of a certain type of cellulase (exoglucanase, endoglucanase, or β-glucosidase) released from bacterial cells, and these values were set between 1 and 100 percent. The summation of individual cellulase levels (defined by the CompositionExo, CompositionEndo, and CompositionGluco parameters) was always equal to 100.
(3) Cellulase enzymatic activity and half-life
The ability of cellulase enzymes to catalyze biochemical reactions was expressed in terms of percentage probability. This probability dictates how likely a particular enzyme is to catalyze cellulose hydrolysis given the enzyme and cellulose are in physical proximity. The different factors such as (1) temperature, (2) pH, and (3) substrate concentration, which determine rate of a biochemical reaction, were combined into a single interaction probability for cellulases. This probability, expressed as a percentage, was allowed to vary between 1 and 100. By changing the PrbExo, PrbEndo, and PrbGluco parameters, the activities of the different types of cellulases (exoglucanses, endoglucanases, and β-glucosidases) were controlled. The cellulase enzyme half-life, which decides the decay in its activity over time before denaturation, was also built into the model. The cellulase enzymes were kept active for a specified number of steps during the ABM routine using the HalfLife parameter. This parameter was allowed to vary between 100 and 200 steps. The lower bound was chosen based on preliminary ABM simulations runs (results not shown), and the upper bound was chosen based on the possibility of extending the half-life of a cellulase enzyme by a factor of 2 through enzyme engineering.
(4) Cellulase enzyme expression system
The EnzymeSystem model parameter allowed simulation of either of the three enzyme systems: (1) complexed, (2) non-complexed, or (3) hybrid cellulose hydrolysis.
(5) Cellular uptake rates of glucose, cellobiose, and cellotriose sugars
Different factors such as the proton motive force and the kinetics of the membrane transporters responsible for sugar uptake were combined into a single probability model parameter that controlled the rate of sugar uptake. This probability was also expressed as percentage ranging between 1 and 100, and it dictates the likelihood of a sugar molecule being transported inside a bacterial cell located in the physical proximity. The parameters PrbGluIntake, PrbBioIntake, and PrbTriIntake determine the probabilities (and ultimately the rate) of (1) glucose, (2) cellobiose, and (3) cellotriose uptake by the cell. The potential range (0 to 100) was identical for each of these parameters, even though the probability (and rate) of glucose uptake exceeds that of cellobiose and cellotriose in observed cultures. However, allowing a full range for each parameter allows the in silico exploration of possibilities of cells engineered for increased cellobiose or cellotriose uptake.
(6) Concentration of bacteria cells present in the system
The number of bacteria in the system was varied through the BacteriaConcentration parameter. A concentration of 60 cells was used for the simulations to maintain a sparsely populated environment to mimic Michaelis-Menten kinetics. This choice was made based on an initial simulation study. The BacteriaConcentration parameter was varied between 1 and 100 cells for the GSA study.
(7) Concentration and average length of the cellulose chains present
The number and length of cellulose chains in the system was set using the CelluloseChains and CelluloseLength parameters, respectively. The number of cellulose chains in the system was varied between 1 and 10, and the cellulose chain length was varied between 1 and 20 glucose molecules. While these values are small compared with cellulose concentrations and especially chain length in a physical system, these small values enabled ABM on a realistic time-scale given the very large number of calculations involved. The upper bound on the cellulose chain length was also determined through initial sets of simulations in which longer chains were used at the expense of computational time (data not shown).
Global sensitivity analysis
As shown in , input parameters of the model are independent of one another. Due to the large number of parameters involved, the GSA was limited to calculating the first-order and total effect indices. The correlations between individual parameters were not calculated in this study. The first-order index measures the sensitivity of the model output to changes in that parameter over its entire range and the entire range of all other parameters. The total effect index measures the sensitivity of the model output for a particular parameter when all interactions of that parameter with all other parameters are considered. A step-by-step procedure for calculating above-mentioned indices has been published.Citation36 Additional details of the GSA calculation are provided in the Supplemental Materials. It was observed that the values of individual indices changed by increasing number of Monte Carlo simulations performed in the GSA. A sufficiently large number of simulations, 2000 in this case, were used to calculate first-order and total effect indices. This sample size was determined through initial sets of simulations (data not shown).
Abbreviations: | ||
ABM | = | agent based modeling |
GSA | = | global sensitivity analysis |
CBP | = | consolidated bioprocess |
SA | = | sensitivity analysis |
Additional material
Download Zip (646 KB)Disclosure of Potential Conflicts of Interest
No potential conflicts of interest were disclosed.
Acknowledgments
This research was supported by research grants from the Institute for Critical Technologies and Applied Science and the Biodesign and Bioprocessing Research Center at Virginia Tech.
References
- McArthur GH 4th, Fong SS. Toward engineering synthetic microbial metabolism. J Biomed Biotechnol 2010; 2010:459760; http://dx.doi.org/10.1155/2010/459760; PMID: 20037734
- Gowen CM, Fong SS. Exploring biodiversity for cellulosic biofuel production. Chem Biodivers 2010; 7:1086 - 97; http://dx.doi.org/10.1002/cbdv.200900314; PMID: 20491068
- Bayer EA, Kenig R, Lamed R. Adherence of Clostridium thermocellum to cellulose. J Bacteriol 1983; 156:818 - 27; PMID: 6630152
- Bayer EA, Lamed R, Himmel ME. The potential of cellulases and cellulosomes for cellulosic waste management. Curr Opin Biotechnol 2007; 18:237 - 45; http://dx.doi.org/10.1016/j.copbio.2007.04.004; PMID: 17462879
- Demain AL, Newcomb M, Wu JHD. Cellulase, clostridia, and ethanol. Microbiol Mol Biol Rev 2005; 69:124 - 54; http://dx.doi.org/10.1128/MMBR.69.1.124-154.2005; PMID: 15755956
- Dien BS, Cotta MA, Jeffries TW. Bacteria engineered for fuel ethanol production: current status. Appl Microbiol Biotechnol 2003; 63:258 - 66; http://dx.doi.org/10.1007/s00253-003-1444-y; PMID: 13680206
- Lynd LR, Weimer PJ, van Zyl WH, Pretorius IS. Microbial cellulose utilization: fundamentals and biotechnology. Microbiol Mol Biol Rev 2002; 66:506 - 77; http://dx.doi.org/10.1128/MMBR.66.3.506-577.2002; PMID: 12209002
- Shoham Y, Lamed R, Bayer EA. The cellulosome concept as an efficient microbial strategy for the degradation of insoluble polysaccharides. Trends Microbiol 1999; 7:275 - 81; http://dx.doi.org/10.1016/S0966-842X(99)01533-4; PMID: 10390637
- Raghothama S, Eberhardt RY, Simpson P, Wigelsworth D, White P, Hazlewood GP, Nagy T, Gilbert HJ, Williamson MP. Characterization of a cellulosome dockerin domain from the anaerobic fungus Piromyces equi. Nat Struct Biol 2001; 8:775 - 8; http://dx.doi.org/10.1038/nsb0901-775; PMID: 11524680
- Berger E, Zhang D, Zverlov VV, Schwarz WH. Two noncellulosomal cellulases of Clostridium thermocellum, Cel9I and Cel48Y, hydrolyse crystalline cellulose synergistically. FEMS Microbiol Lett 2007; 268:194 - 201; http://dx.doi.org/10.1111/j.1574-6968.2006.00583.x; PMID: 17227469
- Liu M, Gu J, Xie W, Yu H. Directed co-evolution of an endoglucanase and a β-glucosidase in Escherichia coli by a novel high-throughput screening method. [doi] Chem Commun (Camb) 2013; 49:7219 - 21; http://dx.doi.org/10.1039/c3cc42485e; PMID: 23841109
- Yan P, Su L, Chen J, Wu J. Heterologous expression and biochemical characterization of an endo-β-1,4-glucanase from Thermobifida fusca. [doi] Biotechnol Appl Biochem 2013; 60:348 - 55; http://dx.doi.org/10.1002/bab.1097; PMID: 23631559
- Toya Y, Hirasawa T, Morimoto T, Masuda K, Kageyama Y, Ozaki K, Ogasawara N, Shimizu H. (13)C-metabolic flux analysis in heterologous cellulase production by Bacillus subtilis genome-reduced strain. J Biotechnol 2014; 179:42 - 9; http://dx.doi.org/10.1016/j.jbiotec.2014.03.025; PMID: 24667539
- Liu L, Liu C, Zou S, Yang H, Hong J, Ma Y, Zhang M. Expression of cellulase genes in Saccharomyces cerevisiae via δ-integration subject to auxotrophic markers. Biotechnol Lett 2013; 35:1303 - 7; http://dx.doi.org/10.1007/s10529-013-1205-7; PMID: 23609230
- Akbarzadeh A, Ranaei Siadat SO, Motallebi M, Zamani MR, Barshan Tashnizi M, Moshtaghi S. Characterization and high level expression of acidic endoglucanase in Pichia pastoris. Appl Biochem Biotechnol 2014; 172:2253 - 65; http://dx.doi.org/10.1007/s12010-013-0672-6; PMID: 24347161
- Li JX, Zhao LM, Wu RJ, Zheng ZJ, Zhang RJ. High-level overproduction of Thermobifida enzyme in Streptomyces lividans using a novel expression vector. Int J Mol Sci 2013; 14:18629 - 39; http://dx.doi.org/10.3390/ijms140918629; PMID: 24025422
- Demain AL, Adrio JL. Contributions of microorganisms to industrial biology. Mol Biotechnol 2008; 38:41 - 55; http://dx.doi.org/10.1007/s12033-007-0035-z; PMID: 18060538
- Karmakar M, Ray RR. Current trends in research and application of microbial cellulases. Research Journal of Microbiology 2011; 6:41 - 53; http://dx.doi.org/10.3923/jm.2011.41.53
- Chandel AK, Chandrasekhar G, Silva MB, da Silva SS. The realm of cellulases in biorefinery development. Crit Rev Biotechnol 2012; 32:187 - 202; PMID: 21929293
- Fischer CR, Klein-Marcuschamer D, Stephanopoulos G. Selection and optimization of microbial hosts for biofuels production. Metab Eng 2008; 10:295 - 304; http://dx.doi.org/10.1016/j.ymben.2008.06.009; PMID: 18655844
- Gowen CM, Fong SS. Applications of systems biology towards microbial fuel production. Trends Microbiol 2011; 19:516 - 24; http://dx.doi.org/10.1016/j.tim.2011.07.005; PMID: 21871807
- Jüergensen J, Ilmberger N, Streit WR. Screening for cellulases with industrial value and their use in biomass conversion microbial metabolic engineering. In: Cheng Q, ed.: Springer New York, 2012:1-16.
- Kuhad RC, Gupta R, Singh A. Microbial cellulases and their industrial applications. Enzyme Res 2011; 2011:280696; http://dx.doi.org/10.4061/2011/280696; PMID: 21912738
- Stephanopoulos G. Challenges in engineering microbes for biofuels production. Science 2007; 315:801 - 4; http://dx.doi.org/10.1126/science.1139612; PMID: 17289987
- Li DC, Li AN, Papageorgiou AC. Cellulases from thermophilic fungi: recent insights and biotechnological potential. Enzyme Res 2011; 2011:308730; http://dx.doi.org/10.4061/2011/308730; PMID: 22145076
- Santos VT, Esteves PJ, Milagres AM, Carvalho W. Characterization of commercial cellulases and their use in the saccharification of a sugarcane bagasse sample pretreated with dilute sulfuric acid. J Ind Microbiol Biotechnol 2011; 38:1089 - 98; http://dx.doi.org/10.1007/s10295-010-0888-1; PMID: 20953894
- Percival Zhang YH, Himmel ME, Mielenz JR. Outlook for cellulase improvement: screening and selection strategies. Biotechnol Adv 2006; 24:452 - 81; http://dx.doi.org/10.1016/j.biotechadv.2006.03.003; PMID: 16690241
- Sørensen A, Teller PJ, Lübeck PS, Ahring BK. Onsite enzyme production during bioethanol production from biomass: screening for suitable fungal strains. Appl Biochem Biotechnol 2011; 164:1058 - 70; http://dx.doi.org/10.1007/s12010-011-9194-2; PMID: 21360092
- Koch A, Weigel CTO, Schulz G. Cloning, sequencing, and heterologous expression of a cellulase-encoding cDNA (cbh1) from Penicillium janthinellum. Gene 1993; 124:57 - 65; http://dx.doi.org/10.1016/0378-1119(93)90761-Q; PMID: 8440481
- Mazzoli R, Lamberti C, Pessione E. Engineering new metabolic capabilities in bacteria: Lessons from recombinant cellulolytic strategies. Trends Biotechnol 2012; 30:111 - 9; PMID: 21930321
- Bonabeau E. Agent-based modeling: methods and techniques for simulating human systems. Proc Natl Acad Sci U S A 2002; 99:Suppl 3 7280 - 7; http://dx.doi.org/10.1073/pnas.082080899; PMID: 12011407
- Scholl HJ. Agent-based and system dynamics modeling: A call for cross study and joint research. System Sciences, 2001 Proceedings of the 34th Annual Hawaii International Conference on 2001:8 pp.
- Van Dyke Parunak H, Savit R, Riolo R. Agent-based modeling vs. equation-based modeling: A case study and users’ guide multi-agent systems and agent-based simulation. In: Sichman J, Conte R, Gilbert N, eds.: Springer Berlin / Heidelberg, 1998:277-283.
- Saltelli A, Chan K, Scott M. Sensitivity analysis. Wiley 2000.
- Saltelli A, Ratto M, Andres T, Camplongo F, Cariboni J, Gatelli D, Saisana M, Tarantola S. Global sensitivity analysis: The primer. Wiley 2008.
- Ogejo JA, Senger RS, Zhang RH. Global sensitivity analysis of a process-based model for ammonia emissions from manure storage and treatment structures. Atmos Environ 2010; 44:3621 - 9; http://dx.doi.org/10.1016/j.atmosenv.2010.06.053
- Chen S, Wilson DB. Proteomic and transcriptomic analysis of extracellular proteins and mRNA levels in Thermobifida fusca grown on cellobiose and glucose. J Bacteriol 2007; 189:6260 - 5; http://dx.doi.org/10.1128/JB.00584-07; PMID: 17601791
- Dadi AP, Schall CA, Varanasi S. Mitigation of cellulose recalcitrance to enzymatic hydrolysis by ionic liquid pretreatment. Appl Biochem Biotechnol 2007; 137-140:407 - 21; http://dx.doi.org/10.1007/s12010-007-9068-9; PMID: 18478405
- Martinez D, Berka RM, Henrissat B, Saloheimo M, Arvas M, Baker SE, Chapman J, Chertkov O, Coutinho PM, Cullen D, et al. Genome sequencing and analysis of the biomass-degrading fungus Trichoderma reesei (syn. Hypocrea jecorina). Nat Biotechnol 2008; 26:553 - 60; http://dx.doi.org/10.1038/nbt1403; PMID: 18454138
- Raman B, Pan C, Hurst GB, Rodriguez M Jr., McKeown CK, Lankford PK, Samatova NF, Mielenz JR. Impact of pretreated Switchgrass and biomass carbohydrates on Clostridium thermocellum ATCC 27405 cellulosome composition: a quantitative proteomic analysis. PLoS One 2009; 4:e5271; http://dx.doi.org/10.1371/journal.pone.0005271; PMID: 19384422
- Levine SE, Fox JM, Clark DS, Blanch HW. A mechanistic model for rational design of optimal cellulase mixtures. Biotechnol Bioeng 2011; 108:2561 - 70; http://dx.doi.org/10.1002/bit.23249; PMID: 21702033
- Liao H, Zhang XZ, Rollin JA, Zhang YH. A minimal set of bacterial cellulases for consolidated bioprocessing of lignocellulose. Biotechnol J 2011; 6:1409 - 18; http://dx.doi.org/10.1002/biot.201100157; PMID: 21751395
- Badieyan S, Bevan DR, Zhang C. Study and design of stability in GH5 cellulases. Biotechnol Bioeng 2012; 109:31 - 44; http://dx.doi.org/10.1002/bit.23280; PMID: 21809329
- Binner R, Menath V, Huber H, Thomm M, Bischof F, Schmack D, Reuter M. Comparative study of stability and half-life of enzymes and enzyme aggregates implemented in anaerobic biogas processes. Biomass Conversion and Biorefinery 2011; 1:1 - 8; http://dx.doi.org/10.1007/s13399-010-0002-y
- Lin H, Li W, Guo C, Qu S, Ren N. Advances in the study of directed evolution for cellulases. Front Environ Sci Eng China 2011; 5:519 - 25; http://dx.doi.org/10.1007/s11783-011-0326-2
- Lozano P, Bernal B, Bernal JM, Pucheault M, Vaultier M. Stabilizing immobilized cellulase by ionic liquids for saccharification of cellulose solutions in 1-butyl-3-methylimidazolium chloride. Green Chem 2011; 13:1406 - 10; http://dx.doi.org/10.1039/c1gc15294g
- Myung S, Zhang XZ, Zhang YH. Ultra-stable phosphoglucose isomerase through immobilization of cellulose-binding module-tagged thermophilic enzyme on low-cost high-capacity cellulosic adsorbent. Biotechnol Prog 2011; 27:969 - 75; http://dx.doi.org/10.1002/btpr.606; PMID: 21630486
- Park JI, Kent MS, Datta S, Holmes BM, Huang Z, Simmons BA, Sale KL, Sapra R. Enzymatic hydrolysis of cellulose by the cellobiohydrolase domain of CelB from the hyperthermophilic bacterium Caldicellulosiruptor saccharolyticus. Bioresour Technol 2011; 102:5988 - 94; http://dx.doi.org/10.1016/j.biortech.2011.02.036; PMID: 21421309
- Wilensky U. Netlogo. 1999
- Apte AA, Cain JW, Bonchev DG, Fong SS. Cellular automata simulation of topological effects on the dynamics of feed-forward motifs. J Biol Eng 2008; 2:2; http://dx.doi.org/10.1186/1754-1611-2-2; PMID: 18304325