Abstract
Financial support is dear in academia and will tighten further. How can the research mission be accomplished within new restraints? A model is presented for evaluating source components of academic research productivity. It comprises six factors: funding; investigator quality; efficiency of the research institution; the research mix of novelty, incremental advancement, and confirmatory studies; analytic accuracy; and passion. Their interactions produce output and patterned influences between factors. Strategies for optimizing output are enabled.
In developed countries, there are increasing pressures from governments and their funding agencies to demonstrate impact from the spent R&D funds. This creates the need to understand better the productivity of public research, both in academic settings and in governmental institutes.
To optimize these goals, the fundamental factors determining the productive output should be analyzed. In the model presented here (), the sources of production are grouped into six top-tier, or alpha, variables: investments and ongoing funding; investigator experience and training; efficiency of the research environment; the research mix of novelty, incremental advancement, and confirmatory studies; analytic accuracy; and passion. Interactions exist between these variables: they operate similar to multiplicative (not additive) factors determining the total product of research output and productivity, and feedback loops and ratchet effects can link between their inputs and outputs. Relating the output to the input resources creates the measure of efficiency, productivity. Certain widely-discussed research characteristics can be reclassified more usefully as subsets of these six dominant variables, while others can be discarded as distractions or pertaining only to special situations. Institutions and nations should choose deliberately among alternative production goals and, in order for the endeavor to remain stable, must maintain a focus on the intended beneficiaries of the output.
Figure 1. A schematic summary of the factor model presented. Variables influencing the output can be nested within six parental conceptual containers, the “alphas” arrayed at the top of this schematic. The model is not additive, but treats alphas as multiplicative factors. A deficiency in an alpha will impair the output regardless of improvements made in other, more visible and popular input variables. Productivity is a rate and is an expression of efficiency that is independently meaningful only when made relative to a reference unit; it is not equivalent to total production or output.
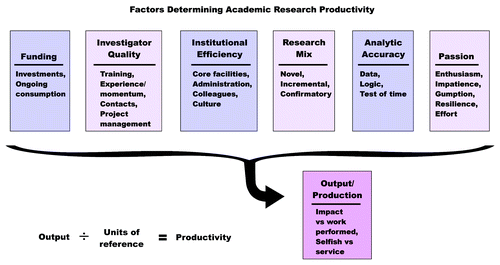
The Output
The numbers of publications, of patents, or of clinical trials in a time period are potential measures of output or production. When expressed as a ratio, such as output per dollar, per nation, per institution, per investigator… they become measures of efficiency or productivity. Choices thus are involved in the kind of output to be optimized. For example, optimizing the impact on a field of study is a laudable goal, similar to profitability or market share of a product in a commercial setting. In contrast, one might wish to measure a less ambiguous, more quantitative goal, perhaps work performed. The latter is independent of impact in a practical sense. Imagine two organizations that each measure the number of publications, patents and clinical trials. One organization may choose to focus on work performed as its premier goal, only eventually to fall behind an organization that sets impact as its.
It is difficult to measure impact, lest paradoxes emerge. For example, the list of Nobel laureates in medicine would not widely overlap the list of investigators that have the top ten percentile rank in publications, patent applications, or clinical trials. Research quality need not parallel quantity. As an example, Kary Mullis invented PCR, which was one of the most financially lucrative and scientifically important discoveries. Dr. Mullis, however, was not superlatively productive as a biochemist by other measures.
Whenever metrics are simple, they can become systematically influenced by learned behaviors. For example, even the weakest investigators learn that they must publish a certain number of articles so as to stay employed. Similarly, the “h-index,” a measure of an investigator's impact based on citation rates,Citation1 is influenced by self-citation or by the behavior of scientific fads, where even poor publications become highly cited due to other poor publications having cited it. To counteract this weakness, better and more intricate metrics can be contrived. For example the h-index is criticized for the ease with which it can be gamed. In response, an “h-squared index” could be proposed, in which the “h function” is applied twice serially, both to each citing publication and to the investigator. In the h-squared index, only highly cited publications (having their own high h-index according to Schubert’s h index of a single publication)Citation2 count toward calculations of an investigator's h-index. This would be extremely difficult to game. The h-index of the current author is 74, while his h-squared index is merely 35. That is, about 35 of his publications were in turn cited by at least 35 other impact-seeking reports of a particular level of acclaim, such that each report had at least 35 citations of its own.
Metrics can be gamed for good purposes, and it would be desirable to work with these tendencies. Well-designed metrics, encouraging desirable patterns of gaming, resistant to undesirable gaming, and easy to adopt, are worthy of attention and development.
Thus, among metrics, hierarchies exist. For example, despite any possible exceptions to the general rules, we are sure that the success of a Phase 3 clinical trial is a more rigorous measure of impact than is success at the Phase 1 level (i.e., success in a Phase 3 trial can be the basis of a change in the standard of care; success in a Phase 1 trial cannot). Numbers of citations can be more valuable than the number of publications. Patents awarded are more tangible in a commercial sense than patents applied for. Drugs administered have more impact than drugs proposed. To contrast with this focus on impact, when work performed is the measured output of choice in a research setting, one might prefer instead to measure Phase I trials, patents applied for, and numbers of lead compounds identified. The advantage of this latter approach is that it is less subject to conditional late events (such as the occurrence of unanticipated clinical adverse events, financial shocks, or patent office delays) not under the direct control of management and investigators.
Measures of output fall also into two other categories—selfish or service—depending on the beneficiary. Research benefits to the researcher, the reward system,Citation3 constitute the selfish measure. Most of the growing clamor concerning the proper measurement of academic productivity concerns such selfish measures and applies them only to compare individuals, often for the purpose of allotting promotions and for advising career development.Citation4 Benefits to the institution, funding agency, contractor, or other constituency (taxpayers, patient advocates, charities, public popularity, economic advancement, etc.) constitute the service. These latter aspects more clearly unite public policy and academic research productivity.Citation5 Once the research resources are in place, the best short-term predictor of success may be an anticipation of the selfish outputs. But if these discoveries, patents, and trials do not eventually appeal to others, the research may be downsized or terminated. An imbalance in the selfish and service benefits is to be expected, but large imbalances are inherently unstable. Unstable systems trend toward stability. It is interesting that at times when one observes that the research endeavor can seem unstable, it is often because it is merely threatening to move back toward stability. Thus, higher levels of academic discovery constitute a required, but insufficient, goal. The subsequent steps of knowledge diffusion, acceptance, adoption, commercialization, etc., determine the impact of the research. These considerations make the service outputs a significant predictor of long-term success.
The Factors
In the model are six dominant, or alpha, characteristics. Each contributes to the overall output of the research. As best as can be expected in a social science, the alphas are conceived as being independent. Each can be varied intentionally or might remain unmodified when left unattended. Alphas are rather few in number; it is generally most useful to nest any additional considerations within one of these parent categories, for long lists of associated variables are seldom very independent.Citation6 The paucity of the proposed alpha categories would prove convenient when using a model as an guide for analysis of a research endeavor or for planning interventions to improve productivity. The alphas serve as parent containers into which new subcategories should be nested.
The alphas are envisioned as factors: multiplicands that together determine the mathematical product, output. To depict the potential use of a mathematical theory for research productivity, imagine an idealized scenario with a scale applied to each factor, such that each increment in the scale “costs” the same in input resources. Specifically, let us imagine that the Investigator Quality alpha currently has a value of “2,” “Funding” has a value of “8,” and the institution has four units of enhancement to spread around. Additional contributions to the Investigator Quality alpha would elevate its value and the value of the mathematical product, output, by 200%, i.e., a large amount. In contrast, contributing to Funding would increase it and the output by a mere 50%. Interactions between the alphas might alter this expectation somewhat, and it is indeed whimsical to expect that the incremental contribution of a factor would cost the same irrespective of the factor’s identity or that each factor should be given equal weighting. Yet, it is the main message of this exercise, and of the proposed model itself, that a factor-based analysis of a research endeavor might allow one to reach a higher productivity than that produced by assuming the alphas to be merely additive (in which case the choice of a designated target for a new improvement might be essentially interchangeable) or by mere uninformed investments. As Socrates might have said, the unexamined investment is not worth investing.
The necessity of considering multiple variables simultaneously is not only intuitive, but repeatedly has found research support. Explanatory power is weak when either individual determinants of research productivityCitation7 or measures at an organizational levelCitation8 are considered separately. For this reason, the proposed six alphas, or factors, below are each explored separately and then, to illustrate their interactions, jointly. Alphas are listed in approximately decreasing order of attention, as judged roughly from official sources such as the programmatic initiatives of national research funding agencies and in the position papers of scientific associations (for example, see ref. Citation9).
(1) Funding
Monetary inputs can be used for prolonged consumption, termed an investment, or for immediate consumption in ongoing activities. Conventional assumptions are that increases and decreases in funding will produce proportionate changes in research output provided that a reasonable balance is maintained between investments and ongoing consumption. When the other five major variables are being utilized at “less than capacity” this predictable relationship may operate, but arguably such a scenario may be infrequent. Trained and experienced investigators may be limited in the short-term, projects well-honed to the required funding criteria may be few, or funding decisions and distribution processes may be inefficient, such that the full amount of the funding may not be readily utilized. This shortcoming is a threshold effect, with the available money “burning a hole” in an agency’s proverbial pocket until it can be “shoveled out the door.” Alternately, an unanticipated increase in funding may encourage a loosening of analytic accuracy and other quality criteria so as to facilitate spending.
(2) Investigator quality
The quality of the investigators is paramount. One suitable measure of quality is provided by observation of their recent successful research experience, or momentum. Experience conveys the concept of practical and informally learned knowledge, and training, the theoretical or formally learned. Investigator quality also encompasses creativity and other less-measurable attributes. This variable is meant to provide a handle on the stable qualities accumulated among the work force, rather than the transient influences on their productivity that might be contributed by the five other factors. For example, training new investigators does not provide the experience component. Both an initial recruitment effort for trainees and subsequent career retention are required to drive quality among the investigator pool. Successful training and later career momentum are highly related.Citation10
The number of career publications, number of students trained, and promotion or tenure are components often used to measure the value of the investigator, as limited as these metrics may be. In academic labs, the investigator rather than the institution nearly exclusively maintains the network of contacts with outside scientists, a measurable value. Ideally, thus, the metric could be broad, along the lines of “scientific and technical human capital” as proposed by Bozeman, Dietz and Gaughan.Citation11 Individual situations affect the metrics; Carayol and Matt confirmed prior reports that full-time researchers publish more, as expected.Citation12 The number of investigators is not an inherent feature of this variable, however, for it can be useful at times to consider their numbers (or the numbers of academic institutions or of scientific projects) as a mere reflection of the magnitude of the Funding variable.
(3) Institutional efficiency
The goal of an institution in optimizing efficiency is, in part, to systematically convert the routine and predictable needs into commodities such as core services and libraries, so as to free up the investigators for tasks requiring their training and creativity. In part, it is to create a predictable administration: a stable foundation permitting the investigators without distraction to focus on more difficult, perhaps longer-range, goals. The institution can facilitate the diffusion of scientific knowledge using proximities between scientists, visitors, vendors, etc. Finally, it provides a departmental effect, where individual productivity of incoming investigators, however diverse, soon conforms to that of their colleagues.Citation13 Thus, the quality of colleagues matters.
An efficient environment encompasses a set of conventional core functions often provided intentionally by the institution. In biomedical science, NIH-funded academic centers (such as a cancer center) are maintained by a grant devoted almost entirely to improving the efficiency of such core and administrative functions. Large academic centers often accomplish these well, but incompletely; small ones emulate the large centers to jumpstart their own efficiency. The typical components are diverse, including an efficient library/interlibrary loan service, a collection of diverse investigators having useful capabilities, centralized provision of complex multi-user equipment, physical and electronic facilities, a magnet labor pool from which to select new hires, discount-purchasing rapid-delivery systems, a technology-savvy legal office, ethics-review boards, etc. The comprised list also includes the collaborative atmosphere, which the institution cannot directly decree but which is essential to its efficiency.
In academia, the investigator rather than the institution embodies (or fails to embody) the project management skills, a key and yet overlooked component. The pattern may differ in large for-profit companies (such as GE), where ensuring the project management skills is a core function of the company itself (e.g., Six Sigma, performance reviews, milestone-based bonuses, etc.). In academia, no provisions for formal project management are generally found.
The pursuit of research efficiency thus should be extended to novel capabilities. Just as commercial businesses may hire consultants to import skills in project management, an academic institution might make available an expert business consultation service so as to achieve more efficient project management, rather than assuming it to be automatically vested in the investigator’s skills. In academia, providing state-of-the-art professional project-management support to investigators would offer a rather low-cost option for raising productivity. Or an institution might employ expert-scientist rapid-response teams to provide for short-term technical sprints, for which creating short-term employment positions cannot realistically be done de novo. The latter are maintained by some for-profit businesses and very often by sophisticated militaries and police departments, but rarely if ever by academic institutions. The efficient research institutions of the future may become sufficiently motivated to implement such functions.
Interactions immediately suggest themselves. When the investigator finds reinforcement from engaging separate goals (such as performing research alongside teaching duties or clinical care), the interaction might be overall positive.Citation14 In contrast, when the institution serves disparate goals, focused passion might be discouraged, and the split missions could distract the investigators. And if the institution relies excessively on short-term financial supports, the research foundation could be rendered too unstable to foster pursuits of the deeper scientific questions. Whatever the financial benefits of short-term funding, a price may be paid for it through a decreased institutional efficiency.
(4) Research mix
Useful science does three things. It makes novel discoveries, extends these discoveries incrementally, and confirms and self-corrects itself by enabling other investigators to repeat the reported experiments. It would be a fallacy to simplistically value novelty without incremental science, or to systematically prefer novelty over confirmatory studies and skeptical science.Citation15 Useful science must incorporate all three components. One of the greatest impediments in modern hypothesis-generating (rather than hypothesis testing) research is the persistent menagerie of unconfirmed novel discoveries and the wasted funding resources consumed to produce it. It takes compassion toward a field, and considerable strength, to muck out its stalls. Imbalance in the research mix is arguably not the natural state of individual scientists, but is often imposed on the scientific work force in self-serving and unavoidable tendencies among funding agencies, publishers, and research institutions. It would not only be refreshing, but it would improve the value of the research mix and the overall research productivity, if greater emphasis were placed on attaining balance among novel, extended, and confirming studies.
(5) Analytic accuracy
Science is accurate when the data and the logic underlying the conclusions are valid. Yet science is not inherently accurate, for it is primarily a social endeavor. Deep inaccuracies can persist for long periods of time. Essentially by definition, such patterns impair research productivity. Data exist to show that incorrect research continues to be cited even after a publication is retracted for fraud.Citation16 Some patterns of citation are best explained as a type of fad rather than a form of analytically rigorous knowledge pursuit.Citation15 A theoretical argument persuasively made the case that most published research was false.Citation17 While misleading science can be readily published, the insights of skeptical readers are seldom shared. Thousands of readers’ comments pertaining to the analytic accuracy of individual published articles are organized and published at www.biomedcriticalcommentary.com,Citation18 but outside the biomarker field such exchanges among readers are usually informal and sporadic. Primary authors seldom revisit the accuracy of their discoveries through future published follow-up reassessment, despite published suggestions that journals could uniformly inaugurate such a policy.Citation15,Citation19 The test of time is a responsibility of science, but a responsibility waived habitually.
Analytic accuracy thus is the Cinderella of science: too often ignored, deprecated, and unwanted. In a prominent recent example, investigators at Duke University Medical Center developed a biomarker panel technology to predict cancer treatment responses and instituted clinical trials using the markers.Citation20 A “forensic statistics” team at MD Anderson Cancer Center examined the database and found multiple large errors (such as “off-by-one” spreadsheet transformations of data) that invalidated the key biomarker associations, a direct challenge to the analytic accuracy of the publications and the ethics of the clinical trials then underway.Citation21 Separately and subsequently, a lead investigator of the Duke team was found to have incorrectly claimed an award in his training record; this was at best an indirect challenge to the ethics of the trials and was not a direct invalidator of the data used to justify the trial designs.Citation22 Duke University took no permanent actions upon learning of the analytic inaccuracy, first halting and then restarting the trials. Duke also sequestered from the scientific community the extent and relevant details of its investigation. Upon learning of the mistaken award claim, however, Duke University fired the lead investigator, terminated the remaining trials, and began sharing sensitive details publicly.Citation22 Until the point when a personality failure emerged, the short-shrift given to the issue of analytic accuracy was characteristic of this episode.
(6) Passion
Passion is proposed as an important factor in research success.Citation23 Passion is a compulsion to perform, impatience to see a result, uncompelled enjoyment and participation, gumption. Although it is not formally measured, a measurement is in theory possible. Passion is by nature malleable, as implied by the existence of words such as “invigorate” or “disenchantment.”
Taking care to sustain the passion of the work force is, it is argued here, generally placed low among the list of institutional scientific objectives. Experience shows that among the best investigators discussions of investigational passion are uncomfortable enough that they are conducted largely in private, and among some investigators these discussions incite anger.Citation24 Embodied in the design of the current model is a prediction, that greater attention by research institutions and funding agencies so as to officially value passion, to avoiding inducing disenchantment, and to convincingly supply a constructive vision for the future, may yield unexpected benefits even despite prolonged limitations of funding and workforce restrictions. It is a failure of leadership when such measures are not pursued with serious and open devotion.
The investigator embodies initiative or passion (or even inertia), which certainly helps determine the quality of the investigator. Yet, in academia the major determinant of a team's passion might instead be the spirit of the institution, the career tendencies of the work force, and the Zeitgeist of the field of study. These tendencies owe to the transient nature of most participants in academic research; they are students and postdoctoral trainees for the most part. It remains unclear whether, when recruiting students into the profession, graduate schools and mentors can at an early timepoint select for students likely to retain or acquire a “fire-in-the-belly” passion as their career development progresses. Thus, Passion in a research endeavor represents a truly independent variable—it is not merely a subset of the Investigator Quality variable.
To the extent that a lack of passion might be a serious bottleneck to research productivity, the causes should be investigated.Citation23 Robert Pirsig in Zen and the Art of Motorcycle Maintenance referred to a similar idea as a “gumption trap” and identified two types: external set-backs and internal hang-ups.Citation25 Institutions can better address an investigator’s external set-backsCitation7 (such as providing “bridge funding” in a predictable manner during temporary shortfalls in outside funding) and should seek to address any reproducible patterns of internal hang-ups (such as anxiety over career advancement opportunities) affecting their research labor force. Even concerning a very modern impediment such as cyberslacking (the personal use of the Internet at work), an expanding literature is examining its underlying causes, from employees’ perceptions of its acceptability to their own demotivational feelings.Citation26
There is a great need to understand what motivates investigators, how we can incentivize them, and what types of incentives produce overall-positive consequences over long time horizons.
Feedback Loops
When interesting phenomena need to be explained, multiple variables may have interplay. Feedback loops can be powerful phenomena emerging from fairly simple relationships of dependent and independent variables. It can be difficult to disentangle what is an independent variable (an input) and a dependent variable (an output), but it is valuable to try. Funding levels, for example, are both an input resource and a measure of output, for success in research (the output) begets improved funding (an input). Similarly, the experience of an investigator is both an input and an output; it determines success, but in turn, success permits career longevity. These relationships create positive-feedback loops which can be critical for long-term research success in academia, just as in business. The unfortunate aspect of a positive-feedback loop is that when a problem emerges, it magnifies, and it risks creating deep failure. Thus, a talented scientist leaving academic research for a few years after birth of offspring may find it nearly impossible to become re-established in the prior career. Can the detrimental patterns of feedback loops be recognized? Can detrimental positive-feedback loops be turned around, so that they become beneficial again? Are there also negative-feedback loops, and are they beneficial or detrimental? Perhaps a nation or institution could support its research better by focusing on these variables in a more systematic manner.
Ratchets
Interactions, especially when they change magnitude, can create a ratchet effect. These are often detrimental and should be managed with care. It might be valuable to explore in detail a theoretical “ratchet risk” scenario. For example, let us consider a ratchet constructed solely from the interplay of two alphas: Funding and Investigator Quality. We postulate a research community operating under certain premises. The investigators are of two classes. “Capable” investigators are potentially productive, are experienced and well trained, intelligent, analytic. Their number is finite and subject to slow change: increasing with incentives and falling with disincentives. Additional “not-as-capable” investigators are unlimited in number. They can be recruited from a large pool of trained degree-holders and, due to comparatively fewer attractive career alternatives, can enter the investigator pool more rapidly upon inducements and would be trapped in a foundering field at a higher rate than capable investigators when disincentives dominate or funding decreases.
Under a constant funding payline (i.e., assuming that a constant fraction of proposals become funded), one expects that for any given funding level, a dynamic equilibrium would establish itself so that the ratio of capable and not-so-capable investigators became stable over time. The ratio would be based on a multitude of sociologic considerations and essentially be unpredictable; the ratio would emerge empirically. In a second scenario in which an increase or a decrease in the payline was instead gradual, the pool of capable investigators might be able to grow or shrink along with the funding change. In a third and rapidly oscillating funding scenario, each increase in the payline sees the pool of funded investigators become diluted with a disproportional increase in not-so-capable investigators. Accompanying each payline decrease, the capable investigators would preferentially migrate to opportunities in other careers, again effectively producing a dilution as the proportion of not-so-capable investigators rises. As the cycle begins anew, the ratchet has clicked in the deleterious direction.
One would want to introduce positive ratchets and constructive feedback loops into the research setting, for it is not inherent in these interactions that they be solely deleterious. Loops and ratchets can be manipulated with incentives, evaluations, and other policy interventions.
Quantitation and Paradoxes
It would be valuable to take a quantitative look at academic research productivity. A rigorous look at the six alphas is likely to reveal paradoxes. Paradoxes are fascinating, for they are unusually instructive. For example, in biomedical science, naive investigators might produce the greater number of new biomarkers, a seemingly important indication of creativity and a measure of high output. Yet, experienced investigators having high impact may create most of the biomarkers that become clinically used and FDA-approved, possibly creating an inverse (paradoxical) relationship between numbers of biomarkers identified and impact, or between investigator experience and work produced. This paradox clues to us that an inappropriate metric had been chosen. As a second example, in resource-poor environments, a large research team may signify a very competitive group; at a well-endowed campus, however, it may be a sign of stagnation and evidence of protection from a competitive environment. Providing evidence of this paradox, in a large systematic study that at the Louis Pasteur University, the size of the lab was negatively associated with research performance.Citation12
As a third example, a highly interactive research group might find itself conscripted into participating in politically expedient but low-impact collaborative research and forced to attend geographically distant planning meetings. Meanwhile, an isolated group might paradoxically outperform, completing the same project without interference and without attending any inter-institutional meetings. The first group is obliged to operate at greater than “critical mass” and in a world of diminishing returns. The latter group is free to function with the number of participants of their choosing and thus remains near the optimal point on the efficiency curve. This represents a paradox in which higher quantitative measures of collaborations, satellite labs, affiliated scientists, and geographic locations prove detrimental.
As a fourth example, it is widely believed that breakthrough discoveries in a field are disproportionately produced by newly arrived, young, investigators. The phenomenon of early productivity is difficult to explain, however.Citation7 To address this apparent paradox, one could examine specific questions. Are early breakthroughs due to greater risk-taking or other systematic differences in the manner by which young investigators conduct their science? Might the phenomenon be more universal than suspected, even affecting experienced investigators? This could occur due to “experienced” investigators actually having little experience in the direction from which comes the breakthrough (i.e., when assessing the experience level in research, do we do so superficially by overlooking that research fields change over time, constantly producing waves of “inexperience”?). Is it a statistical quirk, due to the number of new investigators being disproportionately large in any given field? Is it a self-fulfillment fallacy, whereby the one making a breakthrough will become stably associated and productive within a field but a similar new investigator lacking a breakthrough may not,Citation27 or an impression affected by recall bias, in which breakthroughs by younger investigators are more memorable?
Values Not Modeled
Not covered by the proposed model are additional points of critique concerning values other than mere output, but found in viewpoints already published by others regarding focused public research. Should the size of an effort (the lab or grant or collaborative group) should be small or large? Should a project be led by a sole individual or be a team effort? Are certain types of research more appropriately performed by for-profit entities but not by academic or governmental projects? Should social goals be permitted to compete with raw meritocracy, such as spreading the wealth among famous and less-famous institutions, dictating a balance (a target ratio) between research conducted by younger and older generations, and or increasing the number of granted labs as a goal justifying placing restrictions governing the resources for more successfully funded labs, on the view that one group has too little and the other too much? Can the quality of peer review or grant management at the funding source be improved (not should, but can)? Who should own the products of publicly funded research? Should collaborative efforts be favored over an investigator’s freedom of association (and non-association)? How should the discoveries be commercialized? These additional points call for answers that may not be knowable or generalizable. One could not claim that they come from an unbiased application of an analytic model.
The six-alpha model also is qualitative rather than quantitative. It is anticipated that, for certain purposes, it might be valuable to explore quantitative measures and various means of weighting factors. This extension is beyond the scope of the current proposal.
Reclassifications
There are undeniably a multitude of influences that compete for attention in the research management literature. Yet, it is the bold claim of this model to be exclusive. Additional influences on research output are most constructive when re-cast into the six alphas in order that we dissect them into mechanical concepts, thereby elevating the analytic power. Let us take an example. Public policies have increasingly asked that research output be influenced through deliberate “priority-setting,” “research evaluation,” or “performance-based funding” by a research institution or funding agency.Citation5 Unfortunately, these terms do not readily guide the measurement of progress in individual variables. Let us take the first example: by what mechanisms is a “priority” subsequently made manifest, and are these mechanisms well balanced to optimize output? The application of the proposed model mandates separate accountings: whether the priority-setting created new investments or funding for ongoing research activities; whether the applicant pool of investigators improved upon publicity for the new priority; whether the institution reduced the existing inefficiencies in the priority area; whether the research mix was shuffled; whether increased scrutiny by international colleagues, accompanying the new publicity of the institution’s efforts, encouraged an improvement in analytic accuracy; and whether being the top institutional priority had bolstered raw gumption. To aid learning, the model invites examples of both successful and unsuccessful priority-setting.
A Case Study
It is illustrative to use the six-alpha model to analyze a real-world example. A case report from the University of Missouri Sinclair School of NursingCitation28 describes an inspiring, intentional re-engineering of an academic nursing unit from having no NIH funding to a top-20 ranking. The report is detailed and primarily factual, and to place it in a structured philosophical context entails re-interpreting the provided facts according to the model. After a somewhat accomplished past academic history, the faculty was no longer submitting grants to the NIH, and key members were approaching retirement. Investments had been made in clinical and educational space but not for devoted research activities. Funding for ongoing research salaries was available from split careers in which the compensation for clinical activities permitted borrowing of time for some research. The employment of full-time researchers being precluded, the momentum of research was self-limited and thus restricted the research quality of the Investigators. Any renewed attempts to reinvigorate new research initiatives using faculty-wide discussions were hijacked by subsets of persons remaining uncommitted to an increased research intensity—the group’s Passion had been doused by individuals lacking impatience or gumption to see the new research succeed. It became a top institutional priority to elevate the research activities. But specifically, how?
In order to raise the average passion of the key groups, the dean instituted a “passion filter;” specifically those few faculty proposing a new initiative (here, a Research Interest Group) were appointed to lead group meetings. To these groups, startup resources were made available. To raise the maximal quality and passion among the investigator pool, new hires were taken from applicants intending intensified research, and the pool of applicants was intentionally molded by publicizing research as a top institutional goal. The institution raised the efficiency of the research environment by appointing an Associate Dean for Research, offering seed funding for new projects, giving feedback on proposals intended for outside funding, organizing mock reviews, and hiring a professional editor to sharpen up the applications. Success in output (acquiring new NIH funding) beget new inputs into building investments, ongoing consumption including increased research personnel, quality of investigators, environmental efficiencies, and even more passion among the research applicants and investigators.
Although this institution apparently avoided the danger, change is risky. Thus, using a model will generate simple suggestions, and yet judgment must be employed lest one needlessly submit to the law of unintended consequences. For example, instituting a “passion filter” could select for superficial and highly kinetic individuals, eliminating circumspection; in contrast, experienced participants first might prefer to lay a more intellectually sound foundation prior to action. Also, hiring full-time researchers could weaken the thrust toward clinical relevance that had been previously maintained by the dual-careerists. Teaching might become sidelined while research ascends. Instituting broad change could drive away talented individuals whose contributions do not conform to generalisms, plans, or theory. Rapid growth could morph into chasing fads; indeed, the Missouri school resolved to “hire faculty with interests in fundable topics.”
The model is impartial, and so it also generates criticism and questions of this otherwise outstanding case report. First, the new Research Mix was not described. Was the research exclusively incremental, lacking novelty? Was the emergent research team compassionate enough to do confirmatory research? Did they have the authoritative chops to confront widespread but harmful notions while fostering a more accurate view? Second, the report omits mention of analytic accuracy. Thus, the concept of productivity became divorced from the essential concern of whether the new knowledge could stand the test of time, so as to achieve true value. Third, the output measure was not formally defined, but appeared to be work performed. There was no claim regarding the impact of the research. Did a new nursing practice become instituted nationwide on the heels of their new research and publications? Did local rates of intravenous line infections and other nursing deficiencies fall? Fourth, was the research merely selfish, serving in the main to propagate more research at this institution? If their research had never been performed, would the world be a lesser place? Or did the taxpayers, who supplied the NIH funds, get a compensatory benefit from the transaction? In theory, should the taxpayers want to continue supporting this research, provided that they could become well informed about it? In turn, this raises the question of stability. If their NIH funding were to become less available, would the research be compelling enough to attract other sources of funding? Is the research capable of self-support through licensing, entrepreneurship, or philanthropy; could it be sustained entirely funded by their own institution owing to the service the research provides to its academic and patient care missions?
Finally, while inputs rose (i.e., larger NIH funding amounts and institutional expenses to improve efficiency) and output also rose (i.e., production, as measured by publication numbers), actual productivity was not addressed. No particular ratio was defined as the measure of productivity. Did the output per research hour rise? Did the output per dollar, per person, or per square foot of input resources improve? Did the university, the NIH, and the taxpayer pay too much for the output? The model does not provide answers, but it sharpens the analysis.
Implications and Summary
The six-alpha model carries implications. A danger exists in focusing inappropriately on the most convenient of the component variables, for in a multiplicative model threshold effects (diminishing returns) occur when even single factors remain undeveloped. To institute changes intended to improve productivity requires that one consider the impact of the change on all six alphas. A final and optimistic implication lies in opportunities for improved research productivity, aside from any hoped-for improvements in funding levels. These opportunities can lie overlooked and yet available to be exploited.
Policy makers should want to build processes of continuous improvement and problem management. These would include the standard steps of finding the causes of problems, instituting a solution, measuring the effects of an intervention, and reiteration/feedback to re-assess all stages after the measured intervention. Such study and intervention requires a framework for the analysis. With this as the ultimate intent, the factor model is presented.
Acknowledgments
No financial conflicts to declare. This work has been supported by NIH grant CA62924 and the Everett and Marjorie Kovler Professorship in Pancreas Cancer Research. I appreciated the critical reading of the manuscript and suggestions by Phil Phan of the Johns Hopkins Carey Business School, Baltimore.
References
- Hirsch JE. An index to quantify an individual's scientific research output. Proc Natl Acad Sci USA 2005; 102:16569 - 72; http://dx.doi.org/10.1073/pnas.0507655102; PMID: 16275915
- Schubert A. Using the h-index for assessing single publications. Scientometrics 2009; 78:559 - 65; http://dx.doi.org/10.1007/s11192-008-2208-3
- Cole S, Cole JR. Scientific output and recognition: a study in the operation of the reward system in science. Am Sociol Rev 1967; 32:377 - 90; http://dx.doi.org/10.2307/2091085; PMID: 6046811
- McDade LA, Maddison DR, Guralnick R, Piwowar HA, Jameson ML, Helgen KM, et al. Biology needs a modern assessment system for professional productivity. Bioscience 2001; 61:619 - 25; http://dx.doi.org/10.1525/bio.2011.61.8.8
- Leisyte L, Horta H. Academic knowledge production, diffusion, and commercialization: policies, practices and perspectives. Sci Public Policy 2011; 38:422 - 4; http://dx.doi.org/10.3152/030234211X12960315267697
- Bland CJ, Ruffin MT. Characteristics of a productive research environment: Literature review. Acad Med 1992; 67:385 - 97; http://dx.doi.org/10.1097/00001888-199206000-00010; PMID: 1596337
- Stephan PE. The economics of science. J Econ Lit 1996; 34:1199 - 235
- von Tunzelmann N, Ranga M, Martin B, Geuna A. The effects of size on research performance: A SPRU review. Report prepared for the Office of Science and Technology. Department of Trade and Industry 2003.
- NCI. The nation's investment in cancer research: An annual plan and budget proposal for fiscal year 2012. National Cancer Institute, National Institutes of Health, U.S. Department of Health and Human Services 2011.
- Bland CJ, Schmitz CC. Characteristics of the successful researcher and implications for faculty development. J Med Educ 1986; 61:22 - 31; PMID: 3941419
- Bozeman B, Dietz JS, Gaughan M. Scientific and technical human capital: an alternative model for research evaluation. Int J Technol Manag 2001; 22:716 - 40; http://dx.doi.org/10.1504/IJTM.2001.002988
- Carayol N, Matt M.. Individual and collective determinants of academic scientists' productivity. Inform Econ Policy 2003; 18:55 - 72
- Long JS, McGinnis R. Organizational context and scientific productivity. Am Sociol Rev 1981; 46:422 - 42; http://dx.doi.org/10.2307/2095262
- Fox MF. Research, teaching and publication productivity: Mutuality versus competition in academia. Soc Educ 1992; 65:293 - 305; http://dx.doi.org/10.2307/2112772
- Brody JR, Kern SE. Stagnation and herd mentality in the biomedical sciences. Cancer Biol Ther 2004; 3:903 - 10; http://dx.doi.org/10.4161/cbt.3.9.1082; PMID: 15326377
- Campanario JM. Fraud: retracted articles are still being cited. Nature 2000; 408:288; http://dx.doi.org/10.1038/35042753; PMID: 11099018
- Ioannidis JP. Why most published research findings are false. PLoS Med 2005; 2:e124; http://dx.doi.org/10.1371/journal.pmed.0020124; PMID: 16060722
- Diamandis EP. Cancer biomarkers: can we turn recent failures into success?. J Natl Cancer Inst 2010; 102:1462 - 7; http://dx.doi.org/10.1093/jnci/djq306; PMID: 20705936
- Diamandis EP. Quality of the scientific literature: all that glitters is not gold. Clin Biochem 2006; 39:1109 - 11; http://dx.doi.org/10.1016/j.clinbiochem.2006.08.015; PMID: 17052701
- Potti A, Dressman HK, Bild A, Riedel RF, Chan G, Sayer R, et al. Retraction: Genomic signatures to guide the use of chemotherapeutics. Nat Med 2006; 12:1294 - 300; http://dx.doi.org/10.1038/nm1491; PMID: 17057710
- Coombes KR, Wang J, Baggerly KA. Microarrays: retracing steps. Nat Med 2007; 13:1276 - 7, author reply 7-8; http://dx.doi.org/10.1038/nm1107-1276b; PMID: 17987014
- Baggerly KA, Coombes K. Retraction Based On Data Given To Duke Last November, But Apparently Disregarded. Cancer Lett 2010; 36:1 - 4
- Kern SE. Where's the passion?. Cancer Biol Ther 2010; 10:655 - 7; http://dx.doi.org/10.4161/cbt.10.7.12994; PMID: 20686365
- Wylie C. Principal investigators weigh in. Cancer Biol Ther 2010; 10:1 - 10; http://dx.doi.org/10.4161/cbt.10.9.14054; PMID: 21361067
- Pirsig RM. Zen and the art of motorcycle maintenance. William Morrow, 1974.
- Vitak J, Crouse J, LaRose R. Personal Internet use at work: Understanding cyberslacking. Comput Human Behav 2011; 27:1751 - 9; http://dx.doi.org/10.1016/j.chb.2011.03.002
- Lightfield ET. Output and recognition of sociologists. Am Sociol 1971; 6:128
- Conn VS, Porter RT, McDaniel RW, Rantz MJ, Maas ML. Building research productivity in an academic setting. Nurs Outlook 2005; 53:224 - 31; http://dx.doi.org/10.1016/j.outlook.2005.02.005; PMID: 16226566