Abstract
Pancreatic ductal adenocarcinoma (PDAC) is one of the most malignant cancers with more than 94% mortality rate mainly due to the widespread metastases. To find out the somatically mutated genes related to the metastasis of PDAC, we analyzed the matched tumor and normal tissue samples from a patient diagnosed with liver metastatic PDAC using intensive exome capture-sequencing analysis (> 170× coverage). Searching for the somatic mutations that drive the clonal expansion of metastasis, we identified 12 genes with higher allele frequencies (AFs) of functional mutations in the metastatic tumor, including known genes KRAS and TP53 for metastasis. Of the 10 candidate genes, 6 (ADRB1, DCLK1, KCNH2, NOP14, SIGLEC1, and ZC3H7A), together with KRAS and TP53, were clustered into a single network (p value = 1 × 10−22) that is related to cancer development. Moreover, these candidate genes showed abnormal expression in PDAC tissues and functional impacts on the migration, proliferation, and colony formation abilities of pancreatic cancer cell lines. Furthermore, through digital PCR analysis, we revealed potential genomic mechanisms for the KRAS and TP53 mutations in the metastatic tumor. Taken together, our study shows the possibility for such personalized genomic profiling to provide new biological insight into the metastasis of PDAC.
Introduction
Pancreatic ductal adenocarcinoma (PDAC) is a lethal solid malignancy with a 5-year survival rate of less than 5%.Citation1 The major cause of PDAC death is distant metastasis, which is often coupled with resistance to targeted therapies available to date. Most patients with metastatic PDAC dies after six months of treatment.Citation2 Although clinically important, metastasis-related genomic alterations of PDAC are less understood. Recently, by analyzing the genomic sequences of PDAC metastases in seven patients, Yachida and colleagues revealed the genetic evolution timing of the distant metastases clonally originated from the primary tumor cells as well as the genetic heterogeneity of these clones.Citation3 Simultaneously, phylogenetic analysis of ten matched primary and metastases tumors by Campbell and colleaguesCitation4 supported the genetic heterogeneity of metastasis-initiating cells and their ongoing clonal evolutions. Both studies suggested that the genomic instability in metastatic initiating cells is the main origin of ongoing and parallel divergence among metastases.
Here, in order to reveal the somatically mutated genes related to the metastatic PDAC, we performed a study wherein the matched tumor and normal tissues of both primary and metastatic tumors from an individual with liver metastatic PDAC were analyzed by using both genome-wide SNP array and exome capture-sequencing.
Results
Sample assessment
The recruited patient was a 57-year-old man of Chinese Han ethnicity diagnosed with liver metastatic PDAC. Both the primary pancreatic tumor and liver metastasis were confirmed by histology examination. The DNA samples of the blood, primary tumor and its matched normal pancreatic tissue, metastatic tumor and its matched normal liver tissue were collected from the subject prior to any chemotherapeutic treatment. In addition, genomic DNA samples from the five specimens were genotyped by using SNP arrays. Consanguinity between these samples were estimated based on the IBD/IBS analysis and the high similarities over 99.9% indicated that the samples came from a same individual (Table S1). The heterozygosity comparisons indicated that the possibility of sample mix-up could be excluded.
Global genomic alteration analysis
The global genomic alteration analysis of the five samples by the SNP and CGH arrays consistently showed that the overall genomic profile of the primary tumor was similar to those of the blood and two normal tissue samples, whereas the metastasis tumor showed a highly unstable genome with many aberrations across different chromosomes (Fig. S1). Further CNV analysis by PennCNVCitation5 algorithm identified 1,208 CNVs in the metastatic tumor, but only 22 CNVs in the primary tumor (), supporting the higher level of genomic instability in the metastatic tumor. In addition, more than 50% of the duplications and deletions were larger than 100 Kb in both primary and metastatic tumors, and duplication events were more frequent than that of deletion (Table S2).
Table 1. Summary of genomic alterations in tumor samples
Global coding sequence variation analysis by exome capture-sequencing
The five samples were then subjected to the mutation analysis by exome capture-sequencing (with high depth of sequence coverage ranging from 173.1 to 210× ) (Table S3) using the single nucleotide variant (SNV) calling procedure that was optimized for the samples of heterogeneous cell populations (Supplementary Material). The direct comparison of the allele callings of more than 10,000 bases by both the exome capture-sequencing and SNP array analyses in the five samples revealed over 99% concordance between the genotypes of the two platforms and thus the high accuracy of the SNV callings by the capture-sequencing (Table S4). A total of 86,687, 89,913, 94,188, 114,697, and 89,131 SNVs were found in the blood, normal pancreas, primary tumor, normal liver, and metastasis tumor samples, respectively. After removing the SNVs reported in the dbSNP (build 131) (36,489 or 38.7% for the primary tumor and 36,461 or 40.9% for the metastasis tumor) and observed in the blood (53,034 or 56.3% for the primary tumor and 54,022 or 60.6% for the metastatic tumor), we found 23,610 and 19,479 somatic mutations within exons and untranslated regions (UTRs) in the primary and metastasis tumor samples, with ~74% of them being non-synonymous (~70% missense and ~4% nonsense mutations) and ~3% within the UTR or splice-sites (). These proportions are consistent with the previously published comprehensive study of 24 primary pancreatic cancer tumors.Citation6 Besides, we also identified mutations in genes which are commonly mutated in PDAC, such as KRAS,Citation7 TP53,Citation7 CDKN2A,Citation8 BRCA1/29, APCCitation10 and ATM,Citation11 indicating a typical PDAC case (Table S5).
Of the 2,362 overlapping somatic SNVs between the two tumors, 65 were found to be with significantly higher allele frequency (AF) in the metastatic tumor (p-value < 0.05, Table S6). Interestingly, the top ten coding variants with most significantly higher AF in metastasis include two previously reported variants, KRASG12V (AF = 0.057 in the primary and 0.565 in the metastasis; p value = 5.2 × 10−18) and TP53Y163C (AF = 0.069 in the primary and 0.673 in the metastasis; p value = 3.3 × 10−19). NOP14 was the most frequently mutated gene, with a total of 8 mutations with significantly higher AFs in the metastatic tumor, including seven non-synonymous and one nonsense SNVs. Among all the 65 variants, 13 mutations within 12 genes were likely functional, including 11 variants predicted to be damaging and two nonsense mutations ().
Table 2. Significantly enriched damaging mutations in the metastasis tumor sample
Mutation validation analyses by capillary sequencing, genotyping and digital PCR
Series of validations were performed to confirm the potential driver mutation candidates identified by the exome capture-sequencing analysis (seeSupplementary Material). The genotyping analysis of the 48 mutations with significantly higher AFs in metastasis by Sequenom yielded a confirmation rate of 97.9% in the metastasis tumor and 31.3% in the primary tumor (Table S7), while the capillary sequencing of the 13 functional SNVs validated 12 variants in the metastatic tumor, but none in the primary tumor (Fig. S2). The low confirmation rates in the primary are because the AFs of these SNVs in the primary are below the detection sensitivity of the Sequenom and capillary sequencing analyses (Supplementary Information). Of the same 13 variants, 10 were further analyzed in both the primary and metastatic tumors by digital PCR (3 failed in assay design), which had confirmed both the presence of these SNVs and their AFs in the two tumor samples (). Interestingly, the digital PCR results, together with the array analyses, further indicated that the mutation sites of KRASG12V and TP53Y163C also suffered some copy number aberrances (; Fig. S3 and Table S8). For the KRAS locus, the analyses revealed two types of chromosomes within the metastatic tumor cells, one carrying the KRASwild-type and the other one carrying both the KRASG12V and KRASwild-type alleles. Moreover, among those chromosomes carrying both mutant and wild-type KRAS, the Ct values for KRASG12V was one-cycle less than that for the wild-type allele, indicating a ratio of 2 between KRASG12V and KRASwild-type alleles, which is likely a result of intrachromosomal duplication to enrich the mutant KRAS. As for the TP53 locus, the analyses suggested a mix of different types of tumor cells with some of them carrying the loss of heterozygosity (LOH) of the locus (). These genomic aberrances may help to explain how these two mutations achieved their high frequencies (> 50%) in the metastatic tumor.
Figure 1. Structural variations indicated by Mutant (Mut) and Wild-type (WT) allele signal distributions on digital PCR array Y axis represents the ratio between the number of wells containing both mutant and wild-type allele signals (“Mut&WT”) to those containing only wild-type allele signals (“WT”), whereas X axis represents the ratio between the number of wells containing only mutant allele signals (“M”) over the wells with only wild-type allele signals (“WT”). The results from the germline heterozygous SNPs (red) show the distribution of the two ratios from the series of dilution of non-tumor samples (blood). The analysis of the KRAS variant shows a much higher ratio of the Mut&WT wells over the WT wells than what can be observed in the series of diluted non-tumor genomic DNAs, indicating that the high Mut&WT/WT ratio of the KRAS variant is unlikely due to the co-occupancy of the Mut and WT alleles within the same wells by chance. The ratio of the Mut wells than the WT wells is higher than the ratios observed for the germline heterozygous SNPs in the genomic DNAs of blood through the series of dilution, consistent with the estimated TP53 Mut allele frequency of more than 50%.
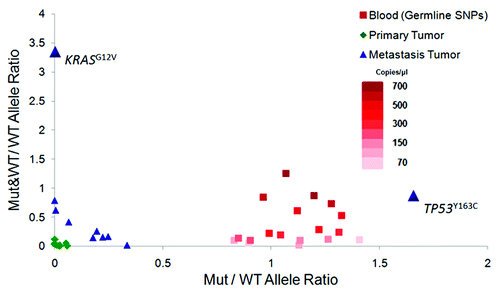
Pathway and functional analysis of metastasis-related candidate genes
Out of the 12 genes carrying the higher AFs of functional variants in the metastatic tumor over the primary one, six candidates KCNH2 (potassium voltage-gated channel, subfamily H (eag-related), member 2), ADRB1 (adrenergic receptor β 1), SIGLEC1 (sialic acid binding Ig-like lectin 1), DCLK1 (doublecortin-like kinase 1), NOP14 (nucleolar protein 14), and ZC3H7A (zinc finger CCCH domain-containing protein 7A), together with KRAS and TP53, were found to be connected into a single gene network by IPA analysis (p value = 1 × 10−22, ), which includes additional tumorigenesis or metastasis-related genes MAPK3, Myc and NF κB.Citation12-Citation14 The most significantly related functions of this network were cancer (p value = 1.6 × 10−6 – 3.2 × 10−2) and tumor morphology (p value = 6.3 × 10−5 to 6.8 × 10−3) (Table S9). While OR2W3 (olfactory receptor, family 2, subfamily W, member 3 pseudogene), SLC39A12 (solute carrier family 39 (zinc transporter), member 12), and LMF1 (lipase maturation factor 1) are not annotated in IPA, DKK2 (dickkopf 2 homolog) does not belong to this network, but has been shown to interact with Wnt signaling pathway and is involved in the progression of renal cell carcinoma.Citation15
Figure 2. Most significant pathway involved the metastasis-enriched genes. Twelve genes harboring a total of 13 mutations were imputed into Ingenuity Pathway Analysis (IPA). Eight out of these 12 genes (in green) were clustered together into the same pathway, i.e., cancer (p value = 1.6 × 10−6 to 3.2 × 10−2) and tumor morphology (p value = 6.3 × 10−5 to 6.8 × 10−3). Molecules are represented as nodes and the biological relationship between two nodes is represented as an edge (line). Various shapes of the nodes represent the functional class of the gene product. Direct interactions are shown as solid lines whereas indirect as dashed lines.
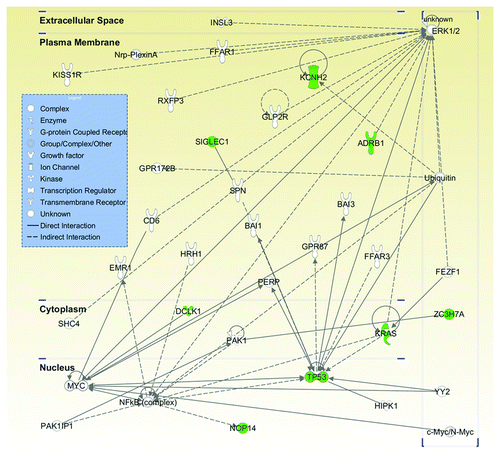
We further investigated the involvement of the newly discovered candidate genes in maintaining malignant features of metastatic tumor cells, especially in terms of their roles in cell migration and proliferation. The knock-down of NOP14 expression in several pancreatic cancer cell lines (derived from either primary or metastasis tumors) by siRNAs decreased the migration and proliferation activities of tumor cells () as well as upregulated the expression of MAPK3/ERK1 that was part of the gene network identified by the IPA above. Decreased cell migration and proliferation activities were also observed when the expressions of ADRB1, DCLK1, KCNH2, and SIGLEC1 were knocked down (Figs. S4–S6), although they had no effect on the expression of MAPK3/ERK1. Moreover, the colony formation ability of tumor cells was also decreased when these genes were knocked down (Fig. S9). Furthermore, we also managed to obtain the antibodies for KCNH2 and SIGLEC1 and performed the immunohistochemistry (IHC) in additional 38 PDAC tissues. Eight out of 38 (21%) PDAC tissues showed high expression level of KCNH2 in the tumor cells, but was only detected in one of the normal pancreatic tissues (; Table S10). SIGLEC1 was highly expressed in tumor cells in nine cases (23.7%), but could not be detected in any normal pancreatic cells. Also, high expression of SIGLEC1 was detected in the metastatic deposit of the lymph node around the pancreas, but not in the normal cells of the lymph node (). These indicated that the abnormal expressions of KCNH2 and SIGLEC1 were commonly observed in some proportion of pancreatic cancer patients. Taken together, our in vitro assays showed that the newly identified candidate genes, including NOP14, ADRB1, DCLK1, SIGLEC1, and KCNH2, might be involved in the metastasis by regulating proliferation, migration and colony formation abilities of pancreatic tumor cells.
Figure 3. Effects of NOP14 expression on the migration and proliferation of pancreatic cancer cells. A, western-blotting showed the downregulated expression of NOP14 by siRNA and its effect on the expression of MAPK3/ERK1; B, comparisons of cell migration with/without knockdown of NOP14 in pancreatic cancer cell lines; C, comparisons of proliferation rates with/without knockdown of NOP14 in pancreatic cancer cell lines.
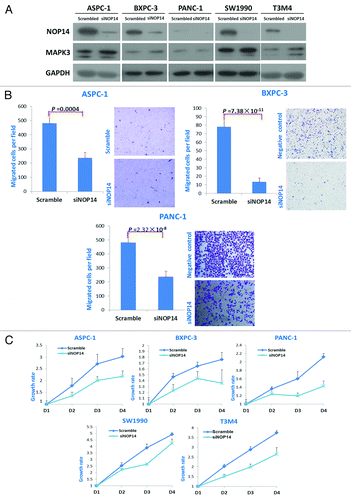
Figure 4. Immunohistochemistry staining of PDAC. A-B, IHC assays using antibody against KCNH2; arrows indicated expression of KCNH2 in pancreatic islet (A) and PDAC (B), but not in the normal pancreatic ductal and acinar tissues (A); C-E, IHC assays using antibody against SIGLEC1; arrows indicated expression of SIGLEC1 in pancreatic islet (C), PDAC (D) and metastasis lesion (E), but not in the normal pancreatic ductal and acinar tissue (C).
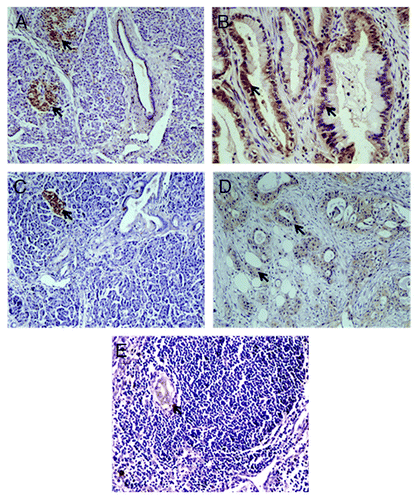
Discussion
Consistent with previous studies,Citation4,Citation16 our study has clearly showed the high level of genomic instability in the liver metastasis of PDAC. This is likely a consequence of the occurrences of tumor suppressor mutations, such as the TP53Y163C. Besides revealing this hallmark of the liver metastasis of PDAC, our study has further suggested that the high genomic instability can in turn promote the further amplifications. With the help of cell proliferation driver mutations like KRASG12V, such a self-enhancing mechanism may contribute to the “aggressiveness” of pancreatic cancer and thus its poor prognosis. Further study will be needed to investigate this possibility.
During the past decades, researchers have sought to identify the genomic alterations involved in the progression of pancreatic cancer metastasis. Genomic alterations of the KRAS oncogene and some tumor suppressor genes such as TP53,Citation17 CDKN2A,Citation18 DPC4Citation19 and BRCA2Citation20,Citation21 were commonly found in the metastasis of pancreatic cancer, which are often referred to as the “mountains” in the landscape of somatic mutation of cancer. In our study, we have identified the two well-known substitutions, KRASG12V and TP53Y163C, with dramatic higher AFs in the metastatic tumor sample, confirming the importance of these somatic “mountains” as the major drivers of the metastasis. The KRASG12V and TP53Y163C with higher AFs in the metastatic tumor observed in this study also supports the suggestion from mouse study that the mutation of TP53 facilitates the retention of the KRAS mutant-expressing cells and drives rapid progression of these premalignant cells to pancreatic tumor.Citation17 Furthermore, additional 10 genes were also found to be with higher mutant AFs in the metastatic tumor, and the subsequent in vitro analyses further supported their functional involvement in the metastasis. Because the mutations of these genes have not been reported in other patients, these candidate genes are more likely infrequently mutated and therefore a part of somatic “hills” (instead of “mountains”). Consistently, our IHC analysis showed that only a proportion of PDAC samples expressed the abnormal level of these candidate genes, such as KCNH2 and SIGLEC1. These “hill” SNVs may, however, contribute significantly to the genetic heterogeneity of pancreatic tumors by composing individual mutation spectrum (beyond the key drivers) in each patient, which may, in turn, contribute to patient’s differential response to treatment. Together with others,Citation22,Citation23 our study has highlighted the need and possibility for such ‘personalized’ genomic profiling in pancreatic cancer by using a cassette of diverse tools, which can provide new biological insight into the metastasis of pancreatic cancer and may facilitate personalized treatment. In addition, the identification of some novel metastasis-related candidate genes by our study may lead to novel targets for treatment through further functional and clinical investigations.Citation6,Citation24
Materials and Methods
Ethics statement
The study was approved by the institutional review board at Peking Union Medical College Hospital, Chinese Academy of Medical Sciences and Peking Union Medical College. Written informed consent was obtained from the recruited patient.
Sample preparation
The recruited patient was a 57-year-old man of Chinese Han ethnicity and diagnosed with PDAC (seeSupplementary Material). Distal pancreatectomy with splenectomy and hepatic metastasectomies were performed after written informed consent was obtained. The primary pancreatic cancer, liver metastasis, normal pancreas, normal liver as well as the blood were collected during the surgery prior to any chemotherapeutic treatment. The primary tumor was characterized by pathology as a moderately differentiated adenocarcinoma. The liver mass was characterized as the metastatic adenocarcinoma.
Genomic DNA from blood, primary and metastasized pancreatic tissue together with their matched normal tissues were extracted using QIAGEN DNeasy Blood and Tissue Kit (QIAGEN) according to the manufacturers protocol.
Exome-capture sequencing and variant calling
Isolated genomic DNA from blood, primary, metastasized pancreatic tissue and their matched normal tissues were captured by Roche’s Nimblegen SeqCap EZ Human Exome v2.0 library using in-solution hybridization and PCR to enrich exomes before sequencing. Sequencing was done on Illumina’s Genome Analyzer II (GAIIx) with each sample running on two lanes of paired-end 101 reads. Mapping of the reads followed Illumina’s sequencing pipeline v1.7 and alignment was based on human reference sequence Build 37. Local realignment around known indels using Burrows-Wheeler Aligner (BWA)Citation25 was performed to reduce misaligned reads that may result in spurious variants and a visual inspection on Integrative Genomics Viewer (IGV)Citation26 was performed afterwards to confirm the success of the realignment. Single nucleotide variant (SNV) calling was performed by SNVMix algorithmCitation27,Citation28 (see URLs) which was found to outperform three other algorithms in terms of its sensitivity and specificity in identifying point mutations from sequencing data of a pooled during our simulation study (see Supplementary Material). Accuracy of the sequencing data and the SNV calling algorithm were calculated based on the concordance of the SNVs found to overlap between our sequencing data and the Illumina’s OmniExpress data of the same sample. Somatic mutations were defined as mutations that were not present in the germline (blood DNA), the matched normal tissue and dbSNP (build 131). Annotation was done by them with SeattleSeq and Sorting Intolerant from Tolerant (SIFT) analysis tool (see URLs) to predict their functional effect.
Copy number variation analysis
B allele frequency (BAF) and Log R ratio (LRR) of the SNP array intensity data was normalized by GenomeStudio software (Illumina) and breakpoints of the CNV were obtained from PennCNV algorithm.Citation5 Agilent SurePrint G3 1M array CGH platform was performed and breakpoints of the copy number events were analyzed by ADM2 software provided by Agilent’s Genomic Workbench 5.0.
Mining of candidate metastasis driver mutations
Potential driver mutations for metastasis are the ones that are significantly enriched in the metastasized sample. We first mined the somatic mutations that overlap between the primary and the metastasized sample, then tested the significance of the allele frequency difference using a binomial test for proportion comparison as implemented by R statistical package under the function prop.test (see URLs). The mutations with significantly higher AFs are the ones with p value < 0.05. SNVs found to be with statistically significantly higher AFs in the metastasized sample as compared with the primary were further investigated for its predicted functional effects using SIFT and tested their network interaction in Ingenuity Pathway Analysis (IPA, Ingenuity Systems).
Mutation validation
First, we did traditional capillary sequencing on all five samples (blood, primary, metastasis, and their matched normal). PCR and sequencing primers were designed within 500 bp flanking sequence. Sequencing results were verified manually to identify those heterozygous mutations. Second, we validated these mutations using Sequenom’s MassARRAY system with its recently developed iPlex PRO kit, with improved sensitivity for detecting low frequency mutations. Assays were performed according to OncoCarta v1.0 Manual Analysis Guide (Sequenom). Finally, we also validated the mutations using Fluidigm’s 48.770 Digital Array Integrated Fluidic Circuit (Fluidigm) using TaqMan custom SNP genotyping probes (Applied Biosystems) for each assay. Experiment was performed according to manufacturer’s protocol. Data was analyzed on Fluidigm Digital PCR Analysis software v3.0.2 (Fluidigm).
Cell cultures and cell growth assays
Human pancreatic cancer cell lines PANC-1Citation29 and T3M4Citation30 were maintained in Dulbecco's modified Eagle's medium while ASPC-1,Citation31 BXPC-3Citation32 and SW1990Citation33 were maintained in 1640 medium supplemented with 10% FBS at 37°C. Cellular growth curves were plotted by using the cellular viability values assessed by the MTS method (Cell Titer 96 Aqueous One Solution Cell Proliferation Assay solution; Promega).
siRNA transfection
Three siRNAs oligos against human ADRB1, DCLK1, KCNH2, NOP14, SIGLEC1 and control siRNA were purchased from Ribobio Co.The targets sequences of each gene seeSupplementary Material. The efficiency of three siRNA oligos was validated by real time PCR and then the three siRNA oligos were pooled together for further study. Cells were seeded into each well of 6-well plates and incubated 24 h, and then transfected with the siRNA by RNAiMAX (Invitrogen). Ten µl of each siRNA oligos (at a final concentration of 50 nM) and 5 µl RNAiMAX were premixed in 500 µl OPTI-medium for 20 min and then added to the cells.
In vitro migration assays
Transwell assays were used to evaluate the migration abilities of the cells. Transwell assay was performed by inserting cell cultures with an 8-µm microporous filter without extracellular matrix coating (Becton Dickinson Labware), then loading it with 200 µl of serum-free medium containing 5.0 × 104 pancreatic cancer cells. The bottom chamber was loaded with 500 µl of medium containing 10% FBS. After incubating for 24 h, the cells on the lower surface of the filter were fixed and stained. Counting of migrated cells was done by selecting five random optical fields (×100 magnification) from triplicate filters.
Immunoblotting
An equal amount of protein from cell lysis for each sample was loaded to SDS-PAGE separation lane and blotted onto a polyvinylidenedifluoride membrane (Millipore). Membranes were probed with specific primary antibodies and respective secondary peroxidase conjugated antibodies (1:10,000). The bands were imaged by using a chemiluminescence method (Amersham Biosciences). See Supplementary Material for details.
Immunohistochemistry staining in pdac tumor tissues
For immunohistochemical (IHC) staining of KCNH2 and SIGLEC, paraffin-embedded tissues were sectioned at 5 μm and IHC staining was performed as described in Supplementary Information. Single comparisons were performed by Student’s t-test, or Chi-square test (2-tailed; p < 0.05 was considered significant).
Abbreviations: | ||
PC | = | pancreatic cancer |
AF | = | allele frequency |
CNV | = | copy number variation |
SNV | = | single nucleotide variant |
IHC | = | immunohistochemistry |
Additional material
Download Zip (8.9 MB)Disclosure of Potential Conflicts of Interest
No potential conflicts of interest were disclosed.
Acknowledgments
This work was partly supported by the National Laboratory of Molecular Biology Special Foundation (2060204), the National Natural Science Foundation of China (81141034) and by the Agency of Science, Technology and Research, Singapore. AI was supported by the Singapore International Graduate Award. We thanked Yi Liang, Xin-Jian Li, Juan WU and Yuan-Zhong Wu from Sun Yat-sen University Cancer Center, De-Tian Wang, Han-Xiang Zhan, Tian-Xiao Wang and Guan-Nan Zhang from Peking Union Medical College Hospital, and Linda Soo, Ishak Irwan, John Koh, Jia Nee Foo, Dennis Tan and Chang Hua Wong from Genome Institute of Singapore for their assistance in experiments and analysis. We also thanked Darryl Irwin from Sequenom Inc. for assistance in primer designs and data analysis.
Author Contributions
Conceived and designed the experiment: Y.P.Z., J.L. and J.X.B. Performed the experiments: J.X.B., J.L., A.I., Y.M.G. B.Z., G.C., T.Z., B.Z., L.Z.C. and Q.S.F. Analyzed the data: A.I., Y.M.G., B.Z., Q.W. and J.J.L. Wrote the paper: A.I., Y.M.G., B.Z., J.X.B., and J.L.
Supplemental Materials
Supplemental materials may be downloaded at:
Notes
† These authors contributed equally to the work.
‡ These authors jointly directed the work.
References
- Jemal A, Siegel R, Xu J, Ward E. Cancer statistics, 2010. in CA Cancer J Clin, Vol. 60 277-300 (2010 American Cancer Society, Inc., United States, 2010).
- Bayraktar S, Rocha-Lima CM. Advanced or metastatic pancreatic cancer: molecular targeted therapies. Mt Sinai J Med 2010; 77:606 - 19; http://dx.doi.org/10.1002/msj.20217; PMID: 21105124
- Yachida S, Jones S, Bozic I, Antal T, Leary R, Fu B, et al. Distant metastasis occurs late during the genetic evolution of pancreatic cancer. Nature 2010; 467:1114 - 7; http://dx.doi.org/10.1038/nature09515; PMID: 20981102
- Campbell PJ, Yachida S, Mudie LJ, Stephens PJ, Pleasance ED, Stebbings LA, et al. The patterns and dynamics of genomic instability in metastatic pancreatic cancer. Nature 2010; 467:1109 - 13; http://dx.doi.org/10.1038/nature09460; PMID: 20981101
- Wang K, Li M, Hadley D, Liu R, Glessner J, Grant SF, et al. PennCNV: an integrated hidden Markov model designed for high-resolution copy number variation detection in whole-genome SNP genotyping data. Genome Res 2007; 17:1665 - 74; http://dx.doi.org/10.1101/gr.6861907; PMID: 17921354
- Jones S, Zhang X, Parsons DW, Lin JC, Leary RJ, Angenendt P, et al. Core signaling pathways in human pancreatic cancers revealed by global genomic analyses. Science 2008; 321:1801 - 6; http://dx.doi.org/10.1126/science.1164368; PMID: 18772397
- Berrozpe G, Schaeffer J, Peinado MA, Real FX, Perucho M. Comparative analysis of mutations in the p53 and K-ras genes in pancreatic cancer. Int J Cancer 1994; 58:185 - 91; http://dx.doi.org/10.1002/ijc.2910580207; PMID: 8026879
- Caldas C, Hahn SA, da Costa LT, Redston MS, Schutte M, Seymour AB, et al. Frequent somatic mutations and homozygous deletions of the p16 (MTS1) gene in pancreatic adenocarcinoma. Nat Genet 1994; 8:27 - 32; http://dx.doi.org/10.1038/ng0994-27; PMID: 7726912
- Kim DH, Crawford B, Ziegler J, Beattie MS. Prevalence and characteristics of pancreatic cancer in families with BRCA1 and BRCA2 mutations. Fam Cancer 2009; 8:153 - 8; http://dx.doi.org/10.1007/s10689-008-9220-x; PMID: 18855126
- Horii A, Nakatsuru S, Miyoshi Y, Ichii S, Nagase H, Ando H, et al. Frequent somatic mutations of the APC gene in human pancreatic cancer. Cancer Res 1992; 52:6696 - 8; PMID: 1423316
- Geoffroy-Perez B, Janin N, Ossian K, Laugé A, Croquette MF, Griscelli C, et al. Cancer risk in heterozygotes for ataxia-telangiectasia. Int J Cancer 2001; 93:288 - 93; http://dx.doi.org/10.1002/ijc.1329; PMID: 11410879
- Reddy KB, Nabha SM, Atanaskova N. Role of MAP kinase in tumor progression and invasion. Cancer Metastasis Rev 2003; 22:395 - 403; http://dx.doi.org/10.1023/A:1023781114568; PMID: 12884914
- Wolfer A, Ramaswamy S. MYC and metastasis. Cancer Res 2011; 71:2034 - 7; http://dx.doi.org/10.1158/0008-5472.CAN-10-3776; PMID: 21406394
- Karin M. Nuclear factor-kappaB in cancer development and progression. Nature 2006; 441:431 - 6; http://dx.doi.org/10.1038/nature04870; PMID: 16724054
- Hirata H, Hinoda Y, Nakajima K, Kawamoto K, Kikuno N, Kawakami K, et al. Wnt antagonist gene DKK2 is epigenetically silenced and inhibits renal cancer progression through apoptotic and cell cycle pathways. Clin Cancer Res 2009; 15:5678 - 87; http://dx.doi.org/10.1158/1078-0432.CCR-09-0558; PMID: 19755393
- Armengol G, Capellà G, Farré L, Peinado MA, Miró R, Caballín MR. Genetic evolution in the metastatic progression of human pancreatic cancer studied by CGH. Lab Invest 2001; 81:1703 - 7; http://dx.doi.org/10.1038/labinvest.3780383; PMID: 11742040
- Morton JP, Timpson P, Karim SA, Ridgway RA, Athineos D, Doyle B, et al. Mutant p53 drives metastasis and overcomes growth arrest/senescence in pancreatic cancer. Proc Natl Acad Sci U S A 2010; 107:246 - 51; http://dx.doi.org/10.1073/pnas.0908428107; PMID: 20018721
- Schulz P, Scholz A, Rexin A, Hauff P, Schirner M, Wiedenmann B, et al. Inducible re-expression of p16 in an orthotopic mouse model of pancreatic cancer inhibits lymphangiogenesis and lymphatic metastasis. Br J Cancer 2008; 99:110 - 7; http://dx.doi.org/10.1038/sj.bjc.6604457; PMID: 18577984
- Iacobuzio-Donahue CA, Fu B, Yachida S, Luo M, Abe H, Henderson CM, et al. DPC4 gene status of the primary carcinoma correlates with patterns of failure in patients with pancreatic cancer. J Clin Oncol 2009; 27:1806 - 13; http://dx.doi.org/10.1200/JCO.2008.17.7188; PMID: 19273710
- James E, Waldron-Lynch MG, Saif MW. Prolonged survival in a patient with BRCA2 associated metastatic pancreatic cancer after exposure to camptothecin: a case report and review of literature. Anticancer Drugs 2009; 20:634 - 8; http://dx.doi.org/10.1097/CAD.0b013e32832b511e; PMID: 19433978
- Chalasani P, Kurtin S, Dragovich T. Response to a third-line mitomycin C (MMC)-based chemotherapy in a patient with metastatic pancreatic adenocarcinoma carrying germline BRCA2 mutation. JOP 2008; 9:305 - 8; PMID: 18469443
- Collisson EA, Sadanandam A, Olson P, Gibb WJ, Truitt M, Gu S, et al. Subtypes of pancreatic ductal adenocarcinoma and their differing responses to therapy. Nat Med 2011; 17:500 - 3; http://dx.doi.org/10.1038/nm.2344; PMID: 21460848
- Strimpakos AS, Syrigos KN, Saif MW. The molecular targets for the diagnosis and treatment of pancreatic cancer. Gut Liver 2010; 4:433 - 49; http://dx.doi.org/10.5009/gnl.2010.4.4.433; PMID: 21253292
- Jones SJ, Laskin J, Li YY, Griffith OL, An J, Bilenky M, et al. Evolution of an adenocarcinoma in response to selection by targeted kinase inhibitors. Genome Biol 2010; 11:R82; http://dx.doi.org/10.1186/gb-2010-11-8-r82; PMID: 20696054
- Li H, Durbin R. Fast and accurate short read alignment with Burrows-Wheeler transform. Bioinformatics 2009; 25:1754 - 60; http://dx.doi.org/10.1093/bioinformatics/btp324; PMID: 19451168
- Robinson JT, Thorvaldsdóttir H, Winckler W, Guttman M, Lander ES, Getz G, et al. Integrative genomics viewer. Nat Biotechnol 2011; 29:24 - 6; http://dx.doi.org/10.1038/nbt.1754; PMID: 21221095
- Goya R, Sun MG, Morin RD, Leung G, Ha G, Wiegand KC, et al. SNVMix: predicting single nucleotide variants from next-generation sequencing of tumors. Bioinformatics 2010; 26:730 - 6; http://dx.doi.org/10.1093/bioinformatics/btq040; PMID: 20130035
- Shah SP, Morin RD, Khattra J, Prentice L, Pugh T, Burleigh A, et al. Mutational evolution in a lobular breast tumour profiled at single nucleotide resolution. Nature 2009; 461:809 - 13; http://dx.doi.org/10.1038/nature08489; PMID: 19812674
- Lieber M, Mazzetta J, Nelson-Rees W, Kaplan M, Todaro G. Establishment of a continuous tumor-cell line (panc-1) from a human carcinoma of the exocrine pancreas. Int J Cancer 1975; 15:741 - 7; http://dx.doi.org/10.1002/ijc.2910150505; PMID: 1140870
- Okabe T, Yamaguchi N, Ohsawa N. Establishment and characterization of a carcinoembryonic antigen (CEA)-producing cell line from a human carcinoma of the exocrine pancreas. Cancer 1983; 51:662 - 8; http://dx.doi.org/10.1002/1097-0142(19830215)51:4<662::AID-CNCR2820510419>3.0.CO;2-X; PMID: 6821838
- Chen WH, Horoszewicz JS, Leong SS, Shimano T, Penetrante R, Sanders WH, et al. Human pancreatic adenocarcinoma: in vitro and in vivo morphology of a new tumor line established from ascites. In Vitro 1982; 18:24 - 34; http://dx.doi.org/10.1007/BF02796382; PMID: 7182348
- Tan MH, Nowak NJ, Loor R, Ochi H, Sandberg AA, Lopez C, et al. Characterization of a new primary human pancreatic tumor line. Cancer Invest 1986; 4:15 - 23; http://dx.doi.org/10.3109/07357908609039823; PMID: 3754176
- Kyriazis AP, McCombs WB 3rd, Sandberg AA, Kyriazis AA, Sloane NH, Lepera R. Establishment and characterization of human pancreatic adenocarcinoma cell line SW-1990 in tissue culture and the nude mouse. Cancer Res 1983; 43:4393 - 401; PMID: 6871872