Abstract
Colorectal cancer (CRC) is a major cause of mortality in many developed countries. Effective screening strategies were called for to facilitate timely detection and to promote a better clinical outcome. In this study, the role of fecal metabonomics in the non-invasive detection of CRC was investigated. Gas chromatography/time-of-flight mass spectrometry (GC/TOFMS) was utilized for the metabolic profiling of feces obtained from 11 CRC patients and 10 healthy subjects. Concurrently, matched tumor and normal mucosae surgically excised from CRC patients were profiled. CRC patients were differentiated clearly from healthy subjects based on their fecal metabonomic profiles (orthogonal partial least squares discriminant analysis [OPLS-DA], 1 predictive and 3 Y-orthogonal components, R2X = 0.373, R2Y = 0.995, Q2 [cumulative] = 0.215). The robustness of the OPLS-DA model was demonstrated by an area of 1 under the receiver operator characteristic curve. OPLS-DA revealed fecal marker metabolites (e.g., fructose, linoleic acid, and nicotinic acid) that provided novel insights into the tumorigenesis of CRC. Interestingly, a disparate set of CRC-related metabolic aberrations occurred at the tissue level, implying the contribution of processes beyond the direct shedding of tumor cells to the fecal metabotype. In summary, this work established proof-of-principle for GC/TOFMS-based fecal metabonomic detection of CRC and offered new perspectives on the underlying mechanisms.
Introduction
Colorectal cancer (CRC) is one of the leading causes of cancer-related death worldwide, accounting for 8% of all cancer mortality.Citation1 The prognosis of CRC worsens significantly with disease progression, with a drastic decline in 5-y survival rate from 90% in stage I to 12% in stage IV.Citation2 Timely detection of CRC by population screening hence becomes a crucial intervention that could reduce CRC mortality.Citation3 However, established screening methods such as colonoscopy or immunochemical-based fecal occult blood test (FOBT) are either invasive or lack sensitivity and specificity.Citation4 In recent years, fecal DNACitation5 and mRNA testingCitation6 have emerged as potential non-invasive screening tools, but further optimization and validation are required with respect to their clinical diagnostic value. These limitations have fueled ongoing research into complementary fecal-based approaches that would facilitate non-invasive screening for CRC.
While previous studies have explored DNA and mRNA aberrations as potential fecal markers of CRC, a knowledge gap exists regarding the role of post-transcriptional metabolic perturbations. As a result of the dysregulated cellular metabolism and transport that supports their untethered cell growth, cancer cells often carry unique metabolic fingerprints.Citation7-Citation10 Clinical metabonomic studies comparing matched tumor and healthy tissue from CRC patients have consistently revealed alterations in the levels of amino acids, nucleosides and carbohydrates.Citation11-Citation17 Besides host-related factors, dysbiosis of gut microbiota is another key feature of CRC. For instance, a lower abundance of Eubacterium rectale and an increased population of Enterococcus faecalis have been observed in the feces of CRC patients.Citation18,Citation19 Collectively, the repertoire of metabolic derangements derived from both cancer cells and gut microbes may culminate in a distinct metabolic phenotype (metabotype) that characterizes the pathology of CRC. During its direct transit through the gut lumen, it is likely that the feces assimilate this unique metabotype. We hence hypothesized that fecal metabonomics could distinguish CRC patients from healthy subjects and may be applied to the non-invasive characterization of the disease.
Previous studies have demonstrated the potential utility of nuclear magnetic resonance (NMR) spectroscopy in the metabolic profiling of fecal water in CRC patients.Citation20,Citation21 Notably, low fecal levels of acetate and butyrate were found in these patients. While promising, the inherently low sensitivity of NMR precludes the detection of low-abundance metabolites. To broaden the metabolic coverage, complementary analytical platforms such as gas chromatography/mass spectrometry (GC/MS) could be employed. Importantly, GC/MS offers several advantages in terms of reproducibility, sensitivity and reliable structural identification of metabolites.Citation22 Recently, gas chromatography/time-of-flight mass spectrometry (GC/TOFMS)-based analyses of plasma, serum, urine, and exhaled breath have shown promise in the non-invasive characterization of diabetes,Citation23,Citation24 hepatocellular carcinoma,Citation25 bladder cancer,Citation26 and asthma.Citation27 These studies showcased the feasibility of GC/TOFMS-based metabonomics in discovering diagnostic biomarkers of various diseases. At the time that our work was performed, no clinical studies have explored the use of GC/TOFMS for the non-invasive fecal metabolic profiling of CRC.
To fill this knowledge gap, we investigated the metabolic composition of feces from CRC patients and healthy subjects and determine if GC/TOFMS-based metabonomics could be a viable approach for the non-invasive detection of the disease. Additionally, tumor specimens and their matched normal mucosae from the same cohort of CRC patients were analyzed and compared with examine the concordance and variation of metabolic alterations at the fecal and tissue levels.
Results
Immunochemical FOBT
Fecal specimens from 3 CRC patients (CRC 4, CRC 6, and CRC 7, Table S1) contained levels of blood equivalent to more than 1 mg Hb/g stool. The remaining patients had occult levels (less than 1 mg Hb/g stool) of blood in their stool except for CRC 3 whose FOBT yielded undetectable level of blood in stool (Table S1). All 10 healthy subjects had undetectable or occult levels (less than 1 mg Hb/g stool) of blood in their stool.
Fecal metabonomic profiling of CRC
shows representative GC/TOFMS chromatograms of fecal specimens from a CRC patient and a healthy subject. After the exclusion of artifactual and irreproducible peaks, 398 well-resolved chromatographic peaks were detected.
Figure 1. Fecal metabonomic profile of CRC patients and healthy subjects. (A) Typical GC/TOFMS chromatograms of fecal specimens from a CRC patient and a healthy subject (Hl). (B) PCA model 2 differentiating CRC patients from healthy subjects (Hl). (C) OPLS-DA model 2 differentiating CRC patients from healthy subjects (Hl). (D) Validation plot of OPLS-DA model 2.
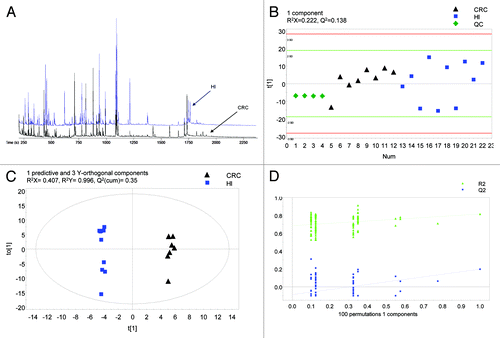
Separate models were constructed with and without the inclusion of patients with blood in stool beyond 1 mg Hb/g stool (models 1 and 2 respectively). The performance statistics of the models were displayed in . For PCA models 1 and 2, QC samples were clustered closely confirming the analytical validity of the method. This observation also confirmed that the clustering trends observed among the clinical observations (CRC patients vs. healthy subjects) were due to biological rather than analytical variations. While partial discrimination between CRC patients and healthy subjects was observed in the PCA scores plots (see for an illustration of PCA model 2), both OPLS-DA models clearly distinguished the two groups. As shown in , OPLS-DA model 1 showed the performance statistics of QCitation2 (cumulative) = 0.215, R2X = 0.373, and R2Y = 0.995 (1 predictive and 3 Y-orthogonal components) and the CRC patients and healthy subjects were classified accurately. On the other hand, OPLS-DA model 2 showed improved performance statistics of QCitation2 (cumulative) = 0.35, R2X = 0.407 and R2Y = 0.996 (1 predictive and 3 Y-orthogonal components) (; ). The robustness of both OPLS-DA models 1 and 2 was demonstrated by an area of 1 under the ROC curves. Permutation testing further indicated that both models were valid, as exhibited by the negative y-intercept of the QCitation2 regression lines (see for validation plot of OPLS-DA model 2). Nevertheless, it is important to note that OPLS-DA model 2 showed superior performance in terms of the number of permuted classifications with QCitation2 exceeding that on the actual classification of samples as compared with OPLS-DA model 1 (2 vs. 17, ). A total of 9 differential analytes characterizing feces of CRC patients were uncovered based on OPLS-DA model 2, among which, 3 marker metabolites (fructose, linoleic acid, and nicotinic acid) were putatively identified. All 3 identified metabolites were found at lower levels in the feces of CRC patients compared with healthy subjects ().
Table 1. Performance statistics of models 1 (all samples included) and 2 (fecal samples containing blood beyond 1 mg Hb/g stool excluded)
Table 2. Fecal and tissue marker metabolites characterizing CRC
Tissue metabonomic profiling of CRC
Tumor tissue was clearly distinct from matched normal mucosae in the PCA scores plot (). Based on a validated OPLS-DA model (see for OPLS-DA plot and for ROC curve), 33 analytes that differentiated the two groups were elucidated. The putative identities of 9 marker metabolites are presented in . Glucose, galactose, 3-phosphoglycerate, citric acid, inosine, and creatinine were reduced while uracil, uridine, and proline were elevated in the tumor tissue compared with matched normal mucosae ().
Discussion
Fecal metabonomic profiling of CRC
Developing an accurate and non-invasive screening tool has been a longstanding goal in the management of CRC. In this respect, fecal metabonomics represents a conceptually promising approach, given the intricate changes in the molecular environment of the gut lumen which could be induced by neoplasia and the underlying dysbiosis and reflected as metabolic alterations in the feces. Herein, we evaluated the use of a GC/TOFMS-based fecal metabonomic approach in detecting CRC.
Preliminary unsupervised PCA revealed a partial separation between the CRC patients and healthy subjects. The lack of complete separation between the two groups was not unexpected as the large inter-individual variability in terms of diet and gut microbial activity might possibly dilute the disease-related metabotype. To circumvent the systematic variation unrelated to pathological status and enhance class separation, a supervised OPLS-DA was employed.Citation28,Citation29 The resultant OPLS-DA models were robust in discriminating CRC patients from healthy subjects, as evident from the good QCitation2 and area under the ROC curves. Of note, CRC 3, a CRC patient who had undetectable blood in stool and might thus constitute a false-negative in conventional FOBT-based screening of CRC, was identified correctly in the OPLS-DA model (Table S1). Our current findings illuminated the potential utility of GC/MS-based fecal metabonomics in accurately identifying CRC patients. Furthermore, it reiterated the role of metabonomics as a powerful systems biology tool in disease classification even with a small sample size of approximately 20 subjects.Citation30-Citation32 Future studies may involve an external validation on a larger series of patients to further assess the predictive power of the model. Subjects with other cancer stages may also be included to ascertain the generalizability of the data.
Gut bleeding is a clinically prevalent phenomenon associated with CRC and other gut diseases. One of the strengths of our study was the additional consideration of the influence of blood in stool on the fecal metabonomic analysis. This crucial concept was first elucidated in a study demonstrating that gross bleeding (100 mg Hb/g feces) could potentially confound GC/MS-based fecal metabonomic studies while occult blood (1 mg Hb/g feces) exerted a negligible effect on the fecal metabotype.Citation33 Efforts were hence undertaken in the current study to construct and compare models with and without the inclusion of fecal samples containing blood beyond 1 mg Hb/g stool. Our data clearly verified the ability of fecal metabonomic analysis to reliably differentiate CRC subjects from healthy subjects regardless of the presence of samples containing blood beyond 1 mg Hb/g stool. Nevertheless, further comparison of QCitation2 and the outcome of permutation testing between models 1 and 2 indicated that class separation was slightly obscured in the presence of fecal samples containing blood beyond 1 mg Hb/g stool. The latter finding reinforced the variation conferred by gross blood that could influence the fecal metabotype characterizing CRC. Importantly, to accurately glean mechanistic insights into CRC pathogenesis, marker metabolites were extracted from model 2 that was constructed independently of the presence of blood-related metabolites.Citation33
Comparison of metabolic alterations at fecal and tissue levels
Fecal markers can be broadly classified as exfoliated markers, secreted markers, and leaked markers.Citation5 Exfoliated markers are derived from the shedding of colonocytes at the gut luminal surface; secreted markers are emanated from the epithelial cells lining the colonic lumen; and leaked markers are products of disturbed blood or lymph vessels following tumor growth. The use of model 2 precluded the possibility of leaked markers being elucidated. To shed light on whether the unique fecal metabotype of CRC patients originated, specifically, from the exfoliated tumor cells, the metabolic aberrations at both fecal and tissue levels were examined. Corroborating previous studies,Citation11-Citation16 our tissue metabonomic analysis showed elevated amino acid (e.g., proline) and nucleic acid constituents (e.g., uracil and uridine), and decreased carbohydrates (e.g., glucose and galactose) in the tumor reflective of their higher proliferative state. Interestingly, this set of marker metabolites was not manifested at the downstream fecal level. The discordant set of tissue and fecal markers implied that the unique metabotype differentiating exfoliated tumor cells from normal colonocytes was possibly diluted in the feces by other contributors to the fecal profile, for instance the abundant shedding of normal colonocytes. In other words, the fecal metabotype of CRC patients were possibly defined more extensively by processes beyond the direct shedding of tumor cells. These complex processes remained to be determined, although possible postulations were cancer-related dysbiosis of gut microbiota or the occurrence of distinct metabolic fluxes between tumor cells and the colonic lumen. Following the recently expounded theory of “tumor field effects”,Citation34 it may also be possible that morphologically normal colonocytes in CRC patients harbored metabolic changes induced by adjacent tumor activity and the exfoliation of these cells might contribute to the discrete fecal metabotype of CRC patients. Importantly, our intriguing finding opened new perspectives for future investigations into the origin of fecal metabolic markers and the multifactorial manifestation of CRC.
Marker metabolites from fecal metabonomic profiling
Concurrent with our publication, Weir et al. also described the GC/MS-based fecal metabonomic profiling of CRC but the influence of blood in stool was not considered in the elucidation of marker metabolites.Citation35 In our study, non-blood-related fecal marker metabolites were extracted from model 2 constructed using fecal samples that do not contain gross levels of blood. Based on these marker metabolites, further insights into the pathogenesis of CRC were yielded. While it might be premature to draw any conclusive association between the marker metabolites and their dysregulated pathways, the relevance of these markers was underscored by several prior studies.
Extensive expression of GLUT-5, a fructose transporter, has been implicated in different types of cancers, including CRC,Citation36 presumably in response to their heightened energy consumption. As both CRC and healthy subjects were not restricted in terms of dietary carbohydrate intake, the reduced fecal level of fructose observed in CRC patients might correspond with the augmented uptake via GLUT-5 in the colon. Linoleic acid has been shown recently to inhibit the growth of CRC cells by mediating oxidative stress and mitochondrial dysfunction.Citation37 The finding of a decreased level of linoleic acid in the feces of CRC patients, consistent with the observation by Weir et al.,Citation35 was potentially significant given its reported anti-oncogenic effect. Nevertheless, the mechanistic cause of the alteration remained unclear and may be of considerable interest in future studies. Finally, the diminished fecal level of nicotinic acid in CRC patients was in agreement with the previously established link between nicotinic acid deficiency and genomic instability.Citation38 The former raised the possibility of a suppression in the activity of nicotinic acid-producing bacteria such as bifidobacteriaCitation39 in relation to CRC. While further studies are required to substantiate the functional significance of these metabolic aberrations, the current work provided novel insights into the role of both tumor cells and gut microbes in CRC tumorigenesis.
Few previous reports on NMR-based fecal water analyses have identified metabolites such as acetate and butyrate as predominant markers differentiating CRC patients from healthy subjects.Citation20,Citation21 Notably, a different set of discriminating metabolites was uncovered in our study. The use of GC/MS hence contributed toward a broadened metabolic space for the discovery of fecal CRC markers.
Conclusion
This proof-of-concept study highlighted the ability of a GC/MS-based fecal metabonomic approach to accurately identify patients with CRC. Despite the small sample size, the discovery of a unique metabotype characterizing CRC opened new avenues in the non-invasive detection of the disease. In addition, the elucidation of marker metabolites provided a mechanistic handle to delineate the biochemical underpinnings of the disease.
Materials and Methods
Clinical population and samples
Eleven CRC patients (Duke stages B–C) and 10 healthy subjects were recruited at the outpatient specialist clinic from September 2011 to June 2012. All subjects were Han Chinese. The inclusion criteria for CRC patients were a diagnosis of sporadic CRC and an intention to undergo surgical tumor resection. Exclusion criterion was treatment with neoadjuvant chemotherapy or radiotherapy. Randomly selected healthy subjects were eligible for this study if a colonoscopy was performed within the past 5 mo to confirm the absence of CRC or pre-cancerous polyps. Exclusion criteria consisted of a history of CRC, a history of polyps within the past 3 y, the presence of inherited CRC syndromes (e.g., Lynch syndrome and familial adenomatous polyposis), and a family history of hereditary CRC. In both groups, subjects did not have coexistent inflammatory bowel disease. The clinical characteristics of the enrolled CRC patients and healthy subjects were summarized in . This study was approved by the Institutional Review Board at the Singapore General Hospital (2009/723/B). All subjects provided informed consent prior to participation in the study.
Table 3. Clinical characteristics of CRC patients and healthy subjects
Fecal specimens were collected domestically by CRC patients prior to surgical resection and by healthy subjects within a month of enrolment into the study. Subjects were given detailed instructions for sample collection. Briefly, a representative aliquot of feces was collected in a sealed plastic container. Care was taken to avoid contact with toilet water and urine. The specimens were transported to the hospital on the same day, or otherwise, kept at 4 °C and brought to the hospital within 2 d. All specimens were stored at −80 °C immediately upon receipt at the hospital.
Surgical tissues resected from the same cohort of CRC patients were snap-frozen in liquid nitrogen, microdissected, and stored at −80 °C until analysis. Careful microdissection of the excised tissue ensured that at least 90% of the tumor specimen comprised cancer cells. Matched normal mucosa samples were obtained from excised tissue at least 5 cm away from the edges of the tumor. The tumor specimens were also subjected to routine histological examination by a blinded pathologist to identify the stage and grade of the cancer. Due to limited sample availability, surgical tissues from 8 out of the 11 CRC patients were subjected to metabonomic profiling.
Materials
RIDASCREEN® Hemoglobin was purchased from R-Biopharm. Spectroscopy grade methanol, chloroform, and toluene were obtained from Tedia. N-methyl-N-(trimethylsilyl) trifluoroacetamide (MSTFA) with 1% trimethylchlorosilane (TMCS) and methoxyamine hydrochloride in pyridine (MOX) were products of Thermo Fisher Scientific. Standard alkane series (C10–C40) and sodium sulfate were purchased from Sigma-Aldrich Inc. Water was purified by a Milli-Q system (Millipore).
Immunochemical FOBT
Fecal hemoglobin (Hb) levels were measured using RIDASCREEN® Hemoglobin, an enzyme-linked immunosorbent assay (ELISA)-based immunochemical FOBT, according to the manufacturer’s instructions. Absorbance (extinction at 450 nm, reference wavelength of 620 nm) was measured on an Infinite M200 microplate reader (Tecan). RIDA®SOFT Win Software was used to convert absorbance values into Hb concentrations (mg Hb/g stool). Fecal Hb levels were stratified into the following categories: (1) not detected, (2) below 0.05 mg Hb/g stool, (3) 0.05–1 mg Hb/g stool, and (4) higher than 1 mg Hb/g stool.
GC/TOFMS metabonomic profiling of feces
A previously developed sample preparation protocol was adopted with slight modifications.Citation33 Fecal samples were freeze-dried and 80 mg of each lyophilized sample was ultrasonicated with 1 mL of ice-cold methanol:water (8:2) mixture in a bath ultrasonicator (Elma Transsonic 460) at 4 °C for 30 min, and vortex-mixed for 2 min. The samples were centrifuged subsequently at 18 000 g at 4 °C for 20 min. A 0.5 mL of the supernatant was obtained carefully. Additionally, quality control (QC) samples were prepared by combining equal volumes of supernatant from all CRC and healthy subjects. Each sample and QC supernatant was concentrated to complete dryness at 50 °C under a gentle stream of nitrogen gas (Turbovap LV, Caliper Life Sciences). Toluene (100 μL, kept anhydrous with sodium sulfate) was added to the dry residue, and dried fully again at 50 °C under nitrogen gas. Methoximation of the dried metabolic extract was performed with 50 μL of MOX (20 mg/mL) at 60 °C for 2 h. Following methoximation, 100 μL of MSTFA with 1% TMCS was introduced and the mixture was incubated at 60 °C for 45 min to produce trimethylsilyl derivatives. Finally, 100 μL of the cooled products was subjected to GC/TOFMS analysis.
GC/TOFMS metabonomic profiling of tissue
Tissue samples were processed using the method described by Mal et al.,Citation13 with slight modifications. Fresh frozen tissue (approximately 20 mg) was ultra-sonicated with 1 mL of ice-cold chloroform:methanol:water (2:5:2) mixture in a bath ultrasonicator at 4 °C for 150 min. After vortex-mixing for 2 min, the samples were centrifuged at 18 000 g at 4 °C for 6 min. A 0.65 mL of supernatant was extracted. Separately, QC samples were prepared by pooling equal volumes of supernatant from all tumor tissues and normal mucosae. Drying of the supernatants was accomplished as described above. Derivatization was performed using 100 μL of MSTFA with 1% TMCS followed by incubation at 70 °C for 30 min to produce trimethylsilyl derivatives. Finally, 50 μL of the cooled products was subjected to GC/TOFMS analysis.
GC/TOFMS analysis
GC/TOFMS analysis was performed on a Pegasus GC/TOFMS (LecoCorp.) connected to an Agilent 7890 gas chromatograph. A DB-1 (30 min × 250 µm i.d.) fused silica capillary column (Agilent J&W Scientific), with 0.25 µm film thickness, was operated with open split interface. Helium was employed as the carrier gas at a constant flow rate of 1.5 mL/min. The injector split ratio was set at 1:20 and 1:6 for fecal and tissue metabonomic analysis, respectively. A volume of 1 μL was injected. The injector, transfer line, and ion source temperatures were held at 220, 280, and 250 °C, respectively. Oven temperature was programmed at 70 °C for 0.2 min, escalated at 9 °C/min to 270 °C where it remained for 5 min, and further increased at 40 °C/min to 310 °C where it was maintained for 11 min. The MS functioned in the electron impact ionization mode at 70eV. Mass data was acquired in a full scan mode from m/z 40 to 600 with an acquisition rate of 20 spectra per second. Detector voltages were 1500 V and 1650 V for fecal and tissue metabonomic analysis, respectively. During the analysis, samples from CRC and healthy subjects were randomized, along with QC samples, to avoid analytical bias. To detect retention time shifts and facilitate kovats retention index (RI) calculation, standard alkane series mixture (C10–C40) was injected at regular intervals. RIs are relative retention times normalized to n-alkanes eluted adjacently.Citation40
Data pre-processing
All gas chromatograms were subjected to baseline correction, de-convolution, noise reduction, smoothing, library matching, and area calculation using ChromaTOF software (version 4.41, LecoCorp.). Only peaks with signal-to-noise ratio larger than 150 were analyzed. The following peaks were further discarded: artifactual peaks that were similarly present in blank samples, poorly resolved peaks, and peaks with poor shapes.
Analytes were identified putatively by comparing both the MS spectra and RI with those in the National Institute of Standards and Technology (NIST) library. If RIs were unavailable in the NIST library, RIs from the Fiehn library, Golm Metabolome Database (http://csbdb.mpimp-golm.mpg.de/gmd.html), Human Metabolome Database (http://www.hmdb.ca/) as well as reported literatureCitation41 were utilized for comparison.
Statistical analysis
Normalization to a constant total chromatographic peak area was made prior to chemometric analyses. Irreproducible peaks defined by a coefficient of variation greater than 30% among QC samples were excluded from further analysis. Chemometric analysis was performed using SIMCA-P version 12.0 software (Umetrics). The normalized data was mean-centered, unit variance scaled,Citation42 and subjected to unsupervised principal component analysis (PCA) to observe for outliers and inherent grouping trends. Following PCA, supervised orthogonal partial least squares discriminant analysis (OPLS-DA) was applied to build discriminating models and elucidate differential analytes that contributed to the separation. For fecal metabonomic profiling, two separate models were constructed. The first model (model 1) was built using the fecal metabolic profiles of all subjects regardless of the levels of blood in their stool measured using the FOBT. In the second model (model 2), fecal samples containing blood beyond 1 mg Hb /g stool were excluded. The performance of the models was indicated by the parameters QCitation2 and R2. The goodness-of-prediction parameter QCitation2 described the predictive ability of the model under 7-fold cross validation. A quantitative measure of the fraction of the variation in the data set that could be explained by the model, also known as goodness-of-fit, was given by the parameter R2.
To examine the robustness of the OPLS-DA models in classifying CRC patients and healthy subjects, and distinguishing tumor tissue from normal mucosae, receiver operator characteristic (ROC) analysis was performed using the cross-validated predicted y values. Corresponding areas under the curves (AUC) were determined. To check for overfitting of data in the OPLS-DA models, response permutation testing was performed with 100 repetitions. The validation was performed on the parent partial list squares discriminant analysis (PLS-DA) models that contained the same number of components. During this process, the sample classes (also known as y variables) were shuffled randomly while the x data were left intact. The validity of the models was (1) inversely related to the number of permuted classifications with QCitation2 values exceeding that on the actual classification of samples, and (2) indicated by a negative y intercept of the regression line fitted among the QCitation2 values.
Based on OPLS-DA, a list of differential analytes was identified using the following criteria: (1) variable importance for projection score of more than 1, (2) P value of less than 0.05 in the relevant t tests, and (3) absolute fold change of more than 2. Specifically, the independent Welch t test was used to compare analytes from fecal samples of CRC patients and healthy subjects, while paired samples t test was used to compare analytes from tumor tissue and matched normal mucosae (Microsoft Excel 2007).
Abbreviations: | ||
CRC | = | colorectal cancer |
FOBT | = | fecal occult blood test |
NMR | = | nuclear magnetic resonance |
GC/MS | = | gas chromatography/mass spectrometry |
GC/TOFMS | = | gas chromatography/time-of-flight mass spectrometry |
MSTFA | = | N-methyl-N-(trimethylsilyl) trifluoroacetamide |
TMCS | = | trimethylchlorosilane |
MOX | = | methoxyamine |
Hb | = | hemoglobin |
QC | = | quality control |
RI | = | retention index |
NIST | = | National Institute of Standards and Technology |
PCA | = | principal component analysis |
OPLS-DA | = | orthogonal partial least squares discriminant analysis |
ROC | = | receiver operator characteristic |
AUC | = | area under the curve |
PLS-DA | = | partial list squares discriminant analysis |
Additional material
Download Zip (47.3 KB)Disclosure of Potential Conflicts of Interest
No potential conflicts of interest were disclosed.
Acknowledgments
The authors thank Ms Jiamin Koh, Dr Ming Hian Kam, Ms Pauline Ngiik Hung Wong, and the Department of Colorectal Surgery at Singapore General Hospital for their support in subject recruitment, as well as Ms Elya, Ms Michelle Shu Mei Lo, Ms Wei Lin Goh, and Ms Grace Yu Hui Wong for their technical assistance and retrieval of the clinicopathological data. This project is funded by the Singapore Ministry of Education’s (MOE) Academic Research Grant (R-148-000-135-112 to ECY Chan and R -148-000-133-112 to HK Ho). LC Phua is supported by the NUS President Graduate Fellowship.
References
- Ferlay J, Shin HR, Bray F, Forman D, Mathers C, Parkin DM. GLOBOCAN 2008 v2.0, Cancer Incidence and Mortality Worldwide: IARC CancerBase No. 10 Lyon, France: International Agency for Research on Cancer, 2010.
- American Cancer Society Cancer Facts and Figures. American Cancer Society, 2012.
- Ouyang DL, Chen JJ, Getzenberg RH, Schoen RE. Noninvasive testing for colorectal cancer: a review. Am J Gastroenterol 2005; 100:1393 - 403; http://dx.doi.org/10.1111/j.1572-0241.2005.41427.x; PMID: 15929776
- Ransohoff DF, Sandler RS. Clinical practice. Screening for colorectal cancer. N Engl J Med 2002; 346:40 - 4; http://dx.doi.org/10.1056/NEJMcp010886; PMID: 11778002
- Osborn NK, Ahlquist DA. Stool screening for colorectal cancer: molecular approaches. Gastroenterology 2005; 128:192 - 206; http://dx.doi.org/10.1053/j.gastro.2004.10.041; PMID: 15633136
- Takai T, Kanaoka S, Yoshida K, Hamaya Y, Ikuma M, Miura N, Sugimura H, Kajimura M, Hishida A. Fecal cyclooxygenase 2 plus matrix metalloproteinase 7 mRNA assays as a marker for colorectal cancer screening. Cancer Epidemiol Biomarkers Prev 2009; 18:1888 - 93; http://dx.doi.org/10.1158/1055-9965.EPI-08-0937; PMID: 19505922
- Kroemer G, Pouyssegur J. Tumor cell metabolism: cancer’s Achilles’ heel. Cancer Cell 2008; 13:472 - 82; http://dx.doi.org/10.1016/j.ccr.2008.05.005; PMID: 18538731
- Hanahan D, Weinberg RA. Hallmarks of cancer: the next generation. Cell 2011; 144:646 - 74; http://dx.doi.org/10.1016/j.cell.2011.02.013; PMID: 21376230
- Vander Heiden MG, Cantley LC, Thompson CB. Understanding the Warburg effect: the metabolic requirements of cell proliferation. Science 2009; 324:1029 - 33; http://dx.doi.org/10.1126/science.1160809; PMID: 19460998
- Benjamin DI, Cravatt BF, Nomura DK. Global profiling strategies for mapping dysregulated metabolic pathways in cancer. Cell Metab 2012; 16:565 - 77; http://dx.doi.org/10.1016/j.cmet.2012.09.013; PMID: 23063552
- Chan EC, Koh PK, Mal M, Cheah PY, Eu KW, Backshall A, Cavill R, Nicholson JK, Keun HC. Metabolic profiling of human colorectal cancer using high-resolution magic angle spinning nuclear magnetic resonance (HR-MAS NMR) spectroscopy and gas chromatography mass spectrometry (GC/MS). J Proteome Res 2009; 8:352 - 61; http://dx.doi.org/10.1021/pr8006232; PMID: 19063642
- Mal M, Koh PK, Cheah PY, Chan EC. Metabotyping of human colorectal cancer using two-dimensional gas chromatography mass spectrometry. Anal Bioanal Chem 2012; 403:483 - 93; http://dx.doi.org/10.1007/s00216-012-5870-5; PMID: 22374317
- Mal M, Koh PK, Cheah PY, Chan ECY. Development and validation of a gas chromatography/mass spectrometry method for the metabolic profiling of human colon tissue. Rapid Commun Mass Spectrom 2009; 23:487 - 94; http://dx.doi.org/10.1002/rcm.3898; PMID: 19140133
- Ong ES, Zou L, Li S, Cheah PY, Eu KW, Ong CN. Metabolic profiling in colorectal cancer reveals signature metabolic shifts during tumorigenesis. Mol Cell Proteomics 2010; Forthcoming http://dx.doi.org/10.1074/mcp.M900551-MCP200; PMID: 20147338
- Piotto M, Moussallieh FM, Dillmann B, Imperiale A, Neuville A, Brigand C, Bellocq JP, Elbayed K, Namer IJ. Metabolic characterization of primary human colorectal cancers using high resolution magic angle spinning 1H magnetic resonance spectroscopy. Metabolomics 2009; 5:292 - 301; http://dx.doi.org/10.1007/s11306-008-0151-1
- Denkert C, Budczies J, Weichert W, Wohlgemuth G, Scholz M, Kind T, Niesporek S, Noske A, Buckendahl A, Dietel M, et al. Metabolite profiling of human colon carcinoma--deregulation of TCA cycle and amino acid turnover. Mol Cancer 2008; 7:72; http://dx.doi.org/10.1186/1476-4598-7-72; PMID: 18799019
- Lean CL, Newland RC, Ende DA, Bokey EL, Smith IC, Mountford CE. Assessment of human colorectal biopsies by 1H MRS: correlation with histopathology. Magn Reson Med 1993; 30:525 - 33; http://dx.doi.org/10.1002/mrm.1910300502; PMID: 8259052
- Balamurugan R, Rajendiran E, George S, Samuel GV, Ramakrishna BS. Real-time polymerase chain reaction quantification of specific butyrate-producing bacteria, Desulfovibrio and Enterococcus faecalis in the feces of patients with colorectal cancer. J Gastroenterol Hepatol 2008; 23:1298 - 303; http://dx.doi.org/10.1111/j.1440-1746.2008.05490.x; PMID: 18624900
- Wang T, Cai G, Qiu Y, Fei N, Zhang M, Pang X, Jia W, Cai S, Zhao L. Structural segregation of gut microbiota between colorectal cancer patients and healthy volunteers. ISME J 2012; 6:320 - 9; http://dx.doi.org/10.1038/ismej.2011.109; PMID: 21850056
- Monleón D, Morales JM, Barrasa A, López JA, Vázquez C, Celda B. Metabolite profiling of fecal water extracts from human colorectal cancer. NMR Biomed 2009; 22:342 - 8; http://dx.doi.org/10.1002/nbm.1345; PMID: 19006102
- Bezabeh T, Somorjai R, Dolenko B, Bryskina N, Levin B, Bernstein CN, Jeyarajah E, Steinhart AH, Rubin DT, Smith IC. Detecting colorectal cancer by 1H magnetic resonance spectroscopy of fecal extracts. NMR Biomed 2009; 22:593 - 600; http://dx.doi.org/10.1002/nbm.1372; PMID: 19259992
- Pasikanti KK, Ho PC, Chan EC. Gas chromatography/mass spectrometry in metabolic profiling of biological fluids. J Chromatogr B Analyt Technol Biomed Life Sci 2008; 871:202 - 11; http://dx.doi.org/10.1016/j.jchromb.2008.04.033; PMID: 18479983
- Lu J, Zhou J, Bao Y, Chen T, Zhang Y, Zhao A, Qiu Y, Xie G, Wang C, Jia W, et al. Serum metabolic signatures of fulminant type 1 diabetes. J Proteome Res 2012; 11:4705 - 11; http://dx.doi.org/10.1021/pr300523x; PMID: 22894710
- Li X, Xu Z, Lu X, Yang X, Yin P, Kong H, Yu Y, Xu G. Comprehensive two-dimensional gas chromatography/time-of-flight mass spectrometry for metabonomics: Biomarker discovery for diabetes mellitus. Anal Chim Acta 2009; 633:257 - 62; http://dx.doi.org/10.1016/j.aca.2008.11.058; PMID: 19166731
- Ye G, Zhu B, Yao Z, Yin P, Lu X, Kong H, Fan F, Jiao B, Xu G. Analysis of urinary metabolic signatures of early hepatocellular carcinoma recurrence after surgical removal using gas chromatography-mass spectrometry. J Proteome Res 2012; 11:4361 - 72; http://dx.doi.org/10.1021/pr300502v; PMID: 22768978
- Pasikanti KK, Esuvaranathan K, Ho PC, Mahendran R, Kamaraj R, Wu QH, Chiong E, Chan EC. Noninvasive urinary metabonomic diagnosis of human bladder cancer. J Proteome Res 2010; 9:2988 - 95; http://dx.doi.org/10.1021/pr901173v; PMID: 20337499
- Caldeira M, Perestrelo R, Barros AS, Bilelo MJ, Morête A, Câmara JS, Rocha SM. Allergic asthma exhaled breath metabolome: a challenge for comprehensive two-dimensional gas chromatography. J Chromatogr A 2012; 1254:87 - 97; http://dx.doi.org/10.1016/j.chroma.2012.07.023; PMID: 22835687
- Trygg J, Holmes E, Lundstedt T. Chemometrics in metabonomics. J Proteome Res 2007; 6:469 - 79; http://dx.doi.org/10.1021/pr060594q; PMID: 17269704
- Wiklund S, Johansson E, Sjöström L, Mellerowicz EJ, Edlund U, Shockcor JP, Gottfries J, Moritz T, Trygg J. Visualization of GC/TOF-MS-based metabolomics data for identification of biochemically interesting compounds using OPLS class models. Anal Chem 2008; 80:115 - 22; http://dx.doi.org/10.1021/ac0713510; PMID: 18027910
- Calvani R, Miccheli A, Capuani G, Tomassini Miccheli A, Puccetti C, Delfini M, Iaconelli A, Nanni G, Mingrone G. Gut microbiome-derived metabolites characterize a peculiar obese urinary metabotype. Int J Obes (Lond) 2010; 34:1095 - 8; http://dx.doi.org/10.1038/ijo.2010.44; PMID: 20212498
- Vallejo M, García A, Tuñón J, García-Martínez D, Angulo S, Martin-Ventura JL, Blanco-Colio LM, Almeida P, Egido J, Barbas C. Plasma fingerprinting with GC-MS in acute coronary syndrome. Anal Bioanal Chem 2009; 394:1517 - 24; http://dx.doi.org/10.1007/s00216-009-2610-6; PMID: 19172251
- Sharif AW, Williams HR, Lampejo T, Khan SA, Bansi DS, Westaby D, Thillainayagam AV, Thomas HC, Cox IJ, Taylor-Robinson SD. Metabolic profiling of bile in cholangiocarcinoma using in vitro magnetic resonance spectroscopy. HPB (Oxford) 2010; 12:396 - 402; http://dx.doi.org/10.1111/j.1477-2574.2010.00185.x; PMID: 20662790
- Phua LC, Koh PK, Cheah PY, Ho HK, Chan ECY. Global gas chromatography/time-of-flight mass spectrometry (GC/TOFMS)-based metabonomic profiling of lyophilized human feces. J Chromatogr B Analyt Technol Biomed Life Sci 2013; 937:103 - 13; http://dx.doi.org/10.1016/j.jchromb.2013.08.025; PMID: 24029555
- Jiménez B, Mirnezami R, Kinross J, Cloarec O, Keun HC, Holmes E, Goldin RD, Ziprin P, Darzi A, Nicholson JK. 1H HR-MAS NMR spectroscopy of tumor-induced local metabolic “field-effects” enables colorectal cancer staging and prognostication. J Proteome Res 2013; 12:959 - 68; http://dx.doi.org/10.1021/pr3010106; PMID: 23240862
- Weir TL, Manter DK, Sheflin AM, Barnett BA, Heuberger AL, Ryan EP. Stool microbiome and metabolome differences between colorectal cancer patients and healthy adults. PLoS One 2013; 8:e70803; http://dx.doi.org/10.1371/journal.pone.0070803; PMID: 23940645
- Godoy A, Ulloa V, Rodríguez F, Reinicke K, Yañez AJ, García MdeL, Medina RA, Carrasco M, Barberis S, Castro T, et al. Differential subcellular distribution of glucose transporters GLUT1-6 and GLUT9 in human cancer: ultrastructural localization of GLUT1 and GLUT5 in breast tumor tissues. J Cell Physiol 2006; 207:614 - 27; http://dx.doi.org/10.1002/jcp.20606; PMID: 16523487
- Zlobec I, Bihl MP, Schwarb H, Terracciano L, Lugli A. Clinicopathological and protein characterization of BRAF- and K-RAS-mutated colorectal cancer and implications for prognosis. Int J Cancer 2010; 127:367 - 80; PMID: 19908233
- Kirkland JB. Niacin requirements for genomic stability. Mutat Res 2012; 733:14 - 20; http://dx.doi.org/10.1016/j.mrfmmm.2011.11.008; PMID: 22138132
- Mayo B, van Sinderen D. Bifidobacteria: Genomics and Molecular Aspects. Caister Acad. Press, 2010.
- Vandendool H, Kratz PD. A Generalization of the Retention Index System Including Linear Temperature Programmed Gas-Liquid Partition Chromatography. J Chromatogr 1963; 11:463 - 71; http://dx.doi.org/10.1016/S0021-9673(01)80947-X; PMID: 14062605
- Verner P. Separation, quantification and identification of non-volatile organic acids in body fluids by gas chromatography. J Pharm Biomed Anal 1988; 6:131 - 50; http://dx.doi.org/10.1016/0731-7085(88)80040-2; PMID: 16867427
- van den Berg RA, Hoefsloot HC, Westerhuis JA, Smilde AK, van der Werf MJ. Centering, scaling, and transformations: improving the biological information content of metabolomics data. BMC Genomics 2006; 7:142; http://dx.doi.org/10.1186/1471-2164-7-142; PMID: 16762068