Abstract
In biological networks, a small number of "hub" proteins play critical roles in the network integrity and functions. The cell cycle network orchestrates versatile cellular functions through interactions between many signaling modules, whose defects impair diverse cellular processes, often leading to cancer. However, the network architecture and molecular basis that ensure proper coordination between distinct modules are unclear. Here, we show that the ubiquitin ligase NIRF (also known as UHRF2), which induces G1 arrest, interacts with multiple cell cycle proteins including cyclins (A2, B1, D1 and E1), p53 and pRB, and ubiquitinates cyclins D1 and E1. Consistent with its versatility, a bioinformatic network analysis demonstrated that NIRF is an intermodular hub protein that is responsible for the coordination of multiple network modules. Notably, intermodular hubs are frequently associated with oncogenesis. Indeed, we detected loss of heterozygosity of the NIRF gene in several kinds of tumors. When a cancer outlier profile analysis was applied to the Oncomine database, loss of the NIRF gene was found at statistically significant levels in diverse tumors. Importantly, a recurrent microdeletion targeting NIRF was observed in non-small cell lung carcinoma. Furthermore, NIRF is immediately adjacent to the single nucleotide polymorphism rs719725, which is reportedly associated with the risk of colorectal cancer. These observations suggest that NIRF occupies a prominent position within the cell cycle network, and is a strong candidate for a tumor suppressor whose aberration contributes to the pathogenesis of diverse malignancies.
Introduction
The cell cycle network is a highly sophisticated protein-protein interaction (PPI) network that integrates a large body of information, and thereby orchestrates versatile cellular functions. The cell cycle engine (cyclin-dependent kinase (CDK)-cyclin complexes) and the two major tumor suppressors (pRB and p53) play pivotal roles not only in cell proliferation, but also in cell growth, death, senescence and differentiation.Citation1 Thus, the cell cycle network is involved in multiple pleiotropic cellular activities, and occupies a central position within the global information network of the cell. However, little is known about the network architecture and molecular basis that facilitate the coordination between distinct functional modules. Here, as a starting point, we aimed to identify a cell cycle protein that may have a role in the network coordination.
In terms of systems biology, the PPI network is scale-free.Citation2 In the scale-free network, most proteins interact with few partners while a small number of hub proteins interact with many partners.Citation3 Hub proteins are more critical than most non-hub proteins for maintenance of the network integrity and functions.Citation4 Furthermore, hub proteins are classified into intermodular hubs and intramodular hubs.Citation5,Citation6 Intermodular hubs bind their different partners asynchronously and organize the proteome by connecting the biological modules to one another. In contrast, intramodular hubs interact with their partners simultaneously, and act inside functional modules. Importantly, the intermodular hubs are frequently associated with oncogenesis.Citation7 This is because mutations of intermodular hubs lead to alterations in the network modularity, causing dysfunction of the whole network.Citation7,Citation8 The multifaceted functions of the cell cycle network support the existence of an intermodular hub that may integrate the distinct functional modules.Citation9 Thus, the discovery of such a putative intermodular hub in the cell cycle network will provide important insights into the cell physiology with direct relevance to cancer pathogenesis.
Ubiquitin-mediated regulation of the cell cycle machinery is closely linked with the genesis of cancer.Citation10 Among the components of the ubiquitination system, E3 ligases are the primary determinants of target recognition.Citation11 The G1 cyclins (cyclins D and E) are basically degraded by Skp1/Cul1/F-box protein (SCF) complexes.Citation12 The SCF complexes use different F-box proteins to recognize distinct substrates. To date, several F-box proteins have been found to ubiquitinate either cyclin D1 or E. Specifically, FBX4/αB crystallin complex,Citation13 FBXW8Citation14 and FBXO31Citation15 target cyclin D1 while FBXW7Citation16 recognizes cyclin E. These SCF ubiquitin ligases contribute to the control at the G1/S boundary by promoting the degradation of the specific G1 cyclins. Impaired ubiquitination of either G1 cyclin causes its aberrant overexpression, ultimately leading to cancer.Citation17 In light of the strong associations between deregulated ubiquitination and human cancers,Citation18 identification of the ubiquitin ligase for G1 cyclins is of great importance.
We previously identified NIRF (Np95/ICBP90-like RING finger protein) as a ubiquitin ligase that interacts with the CDK2-cyclin E complex, and induces G1 arrest.Citation19,Citation20 NIRF has a multidomain composition, comprising a NIRF_N domain (ubiquitin-like domain), PHD finger domain, YDG/SRA domain and RING finger domain.Citation21 It is therefore suggested that NIRF exerts its cell cycle function via the formation of complexes with various signaling molecules. Here, we show that NIRF interacts with multiple cell cycle proteins including cyclins (A2, B1, D1 and E1), p53 and pRB, suggesting that it works in tight collaboration with the core cell cycle machinery. A bioinformatic network analysis suggested that NIRF is an intermodular hub with a high betweenness centralityCitation22 and occupies an important position in the cell cycle network.Citation23,Citation24 Notably, and consistent with the G1-arresting function of NIRF, it ubiquitinated both cyclins D1 and E1. This is in contrast to the SCF-type ubiquitin ligases that only target either cyclin D1 or E for ubiquitination.Citation13–Citation16 Thus, NIRF seems to be another ubiquitin ligase that functions at the G1/S boundary and has a possible relationship with tumorigenesis.
In accordance with this context, we found loss of heterozygosity (LOH) of the NIRF gene in several types of cancer. Our database mining analysis further revealed statistically significant losses in the DNA copy numbers of NIRF in various types of tumors. In addition, a recurrent microdeletion targeting NIRF in non-small cell lung carcinoma was observed. Of note, NIRF is in the immediate vicinity of the single nucleotide polymorphism (SNP) rs719725, which is reportedly associated with the risk of colorectal cancer.Citation25 Thus, the NIRF ubiquitin ligase targets both G1 cyclins, and is a candidate tumor suppressor with features of an intermodular hub in the cell cycle network.
Results
NIRF interacts with the cell cycle machinery and ubiquitinates G1 cyclins.
The cell cycle function of NIRF was analyzed by transfecting EGFP-tagged NIRF into 293 cells. At 48 h after transfection, the NIRF-transfected cell population showed an increase in G1 phase cells (), as described in our previous paper in reference Citation20. This increase compared with the untransfected populations ( and B) and empty vector-transfected cell population () suggested that NIRF induced cell cycle arrest in the G1 phase.
To examine how NIRF is involved in cell cycle control, we initially tried to identify its interacting partners. Using an antibody macroarray, we found that NIRF interacted with a variety of proteins involved in diverse cellular processes ( and S1 and Table S1). Among them, the strong interactors included the core components of the cell cycle machinery, i.e., CDKs, cyclins, PCNA, p53 and pRB (Table S2). These findings suggest that NIRF participates in cell cycle control in tight collaboration with the core cell cycle proteins. To date, no other ubiquitin ligases have been shown to exhibit such a wide accessibility to both cell cycle-promoting factors (cyclins and CDKs) and cell cycle-inhibitory factors (pRB and p53). In particular, the interacting partners for each of the cyclins,Citation12 pRBCitation26 and p53Citation27,Citation28 include ubiquitin ligase(s), indicating that all of them have a connection to the ubiquitination machinery. Therefore, we started our study with a focus on the above molecules.
To validate the above results, we examined whether NIRF binds to these cell cycle proteins in cells by co-immunoprecipitation (co-IP) assays. NIRF, but not the vector control, was reciprocally co-immunoprecipitated with cyclins A2, B1, D1 and E1 (), confirming the results obtained from the antibody macroarray analyses. In the presence of a proteasome inhibitor, MG-132, the signals for all the cyclins were intensified (), implying that these cyclins are destabilized upon interaction with NIRF. Among them, the signals for cyclins D1 and E1 were markedly intensified compared with those without MG-132 treatment. Of note, these signals were accompanied by tailing, which probably reflects polyubiquitinated species (). Interestingly, intense smearing signals were also detected with the anti-myc antibody (), which may represent autoubiquitination of the NIRF ubiquitin ligase, a phenomenon that often accompanies ubiquitination of a substrate.Citation29,Citation30 In contrast, the ubiquitination signals with cyclin A2 or B1 were only slightly visible in the presence of MG-132 (). These results suggest that cyclins D1 and E1 are the preferential targets of the NIRF ubiquitin ligase, consistent with its G1-arresting function. Furthermore, the two major tumor suppressors involved in G1 control, p53 and pRB, formed complexes with NIRF in co-IP assays ( and C), again supporting the results of the antibody macroarray. In contrast, Np95/ICBP90/UHRF1,Citation31,Citation32 a paralog of NIRF, did not interact with NIRF in co-IP assays (). Finally, the above interactions between NIRF and the G1 control proteins were validated by glutathione S-transferase (GST) pull-down assays ().
The NIRF-induced ubiquitination of G1 cyclins was further confirmed by cotransfecting Flag-tagged ubiquitin (), which clearly showed that NIRF enhanced the ubiquitination of both cyclins D1 and E1 (). To complement the in vivo ubiquitination assays, we performed in vitro ubiquitination assays to examine whether NIRF had direct ubiquitination activity. We found that NIRF directly ubiquitinated cyclins D1 and E1, as evidenced by the appearance of the tail (). In summary, the above findings suggest that NIRF tightly cooperates with the core cell cycle machinery and induces G1 arrest, which is accompanied by ubiquitination of cyclins D1 and E1.
NIRF occupies a central position in the cell cycle network.
Next, we attempted to evaluate the characteristics of NIRF by analyzing the network architecture involved. Based on the detected protein-protein interactions, network visualization and analysis were performed with the Cytoscape software.Citation33 Our interaction data were integrated with the already deposited network information for the core cell cycle proteins, which was retrieved from the Pathway Commons database.Citation34 The data sets available from this public database are largely derived from literature curation and massive screening projects (e.g., two-hybrid systemCitation35 and tandem affinity purificationCitation36). Hence, we assumed that our data could be merged with the database information.
At first, we used the PPI information for the core cell cycle proteins to create an interaction map depicting the interactions between NIRF and these cell cycle proteins (). All of the NIRF-interacting proteins (cyclins, pRB and p53) were regarded as hub proteins, since they have many interacting partners of their own.Citation3 Each hub protein together with its own interactors clearly constituted a network module,Citation6 and the whole cell cycle network appeared to be constructed as an assembly of these modules. In the deduced PPI network, NIRF was positioned at a nodal point connecting multiple hub proteins. These observations implied that the cell cycle network involving NIRF exhibits a hierarchical modular topologyCitation3 and that NIRF occupies the center of the interactome. In addition, the map indicated that no other molecules in the network occupied such a unique position as NIRF. For instance, pRB and p53 () are hub proteins cooperatively involved in the G1 checkpoint and genome stability.Citation37 Reflecting their functional cooperation, there was a group of proteins that interacted with both pRB and p53. However, the group size of the common interacting partners shared between pRB and p53 (n = 45) was much smaller than that of the unshared interactors (n = 499). Similarly, in accordance with the common structural and functional properties shared among cyclins A2, B1, D1 and E1 (), they had overlapping interactors.Citation38 However, probably because of the differences among their characteristics, the number of common interactors shared among all of these cyclins (n = 5: BRCA1, HERC5, CDK2, CDKN1A and CDKN1B) was extremely small compared with the total number of cyclininteracting proteins (n = 180). Likewise, the number of common interacting proteins shared between the cell cycle inhibitory factors (pRB and p53) and the cell cycle promoting factors (cyclins and CDKs) was very small (n = 2: BRCA1 and CDKN1A). Finally, multiple proteins in the interaction map () have been registered to interact with NIRF in the database. The registered interactors included PCNA and HDAC1,Citation39 both of which were also detected by the antibody array (Table S1), and nuclear proteins involved in epigenetic regulation. Since neither BRCA1 nor CDKN1A interacts with all of these proteins, NIRF was the only molecule located at the center of the network.
The above observations were further validated by applying network analyses (). Initially, we computed the basic properties of the NIRF PPI network. The network contained 2,466 protein nodes and 4,762 interaction edges, where the average node degree (i.e., average number of neighbors) was 3.66. When we analyzed the shortest path length distribution (), the average shortest path length was calculated to be 2.69. This small value suggested that it is a small-world network, in which the proteins are very closely linked.Citation40 The degree distribution followed a power-low (). This feature indicates a scale-free property of the network, signifying the presence of highly connected hub proteins.Citation2 Both the clustering coefficient distribution () and the topological coefficient distribution () decreased with degree, suggesting a hierarchical modular topology of the network.Citation3,Citation41 These observations imply that most non-hubs are part of highly connected modules, and that the communication between the different modules is maintained by a few hubs.Citation3 Thus, all of the characteristics suggested by the network analyses were in good agreement with the above findings obtained from the inspection of the interaction map. In addition, NIRF had high scores for centrality indices such as betweenness centralityCitation22 and closeness centrality.Citation42 Betweenness centrality represents how influential a node is in communicating between node pairs,Citation43 while closeness centrality is a measure of how fast information spreads from a given node to other nodes.Citation44 The betweenness centrality of NIRF was ranked in the top 0.45% of all the proteins in the integrated network ( legend). This observation indicated that NIRF is a high-betweenness protein, which represents a key connector protein controlling the connectivity of subnetworks to one another.Citation45 Similarly, the closeness centrality was also high, ranked in the top 1.2%. Thus, it is probable that NIRF is one of the most influential proteins in the cell cycle network. In addition, the degree of NIRF was ranked in the top 0.45%, confirming that NIRF is a hub protein. Taken together, these analyses suggest that NIRF is a hub protein with high betweennessCitation23 and occupies a unique central position in the cell cycle network.
Notably, it was evident that the expression profiles of the NIRF-interacting proteins (cyclins A2, B1, D1 and E1, pRB and p53) were mutually different. Therefore, NIRF could be classified as an intermodular hub protein connecting different functional modules.Citation5–Citation7 In this regard, most of the hub proteins with high betweenness are also intermodular hubs.Citation23 It is also known that intermodular hubs are frequently associated with oncogenesis.Citation7 Therefore, we hypothesized that NIRF has a high probability of being relevant to tumor formation.
NIRF is subject to genomic deletion in tumors.
The NIRF gene is localized to a region of chromosome 9p24 that is lost in numerous types of human malignancies at the highest frequency,Citation46 which strongly suggests that this particular region harbors a tumor suppressor gene. Considering its role in cell cycle inhibition, NIRF represents a potential candidate tumor suppressor encoded within this region. We performed a PCR-LOH analysis using the polymorphic markers D9S281 and D9S1852, which are located on the centromeric and telomeric sides of the NIRF gene, respectively (). We detected LOH in 13.4% (2/15) cases for both stomach and colorectal carcinomas, and in 6.7% (1/15) cases with breast carcinoma (). These findings indicate that the NIRF gene is hemizygously deleted in a subset of tumors originating from certain tissues.
Next, we further examined the cancerous aberrations of the NIRF gene by accessing wider information resources. First, we used the Oncomine databaseCitation47 to analyze the DNA copy number profiles of the NIRF gene in a glioblastoma data setCitation48 ( and ). This data set clearly indicated that the NIRF gene was deleted in a significant fraction of the tumor cases. The statistical indices for the mean copy number loss were quite significant, i.e., the fold change was −1.160 and the p-value was 4.65 × 10−41. Next, we applied a cancer outlier profile analysis (COPA) to this data set. COPA is an innovative bioinformatic method for analyzing cancer genes, which enables us to identify a cancerous aberration that occurs in only a subset of cases.Citation49,Citation50 In this glioblastoma data set, the COPA score at the 10 percentile was −52.222, which was ranked in the top 0.2% of the analyzed genes. Furthermore, this COPA score was statistically significant and had a p-value of less than 1 × 10−8. Therefore, the DNA loss of the NIRF gene in this glioblastoma data set was extremely significant, at least in a subset of cases. A data set for lung adenocarcinomaCitation51 () also showed a copy number loss of the NIRF gene. The mean copy number loss was again statistically quite significant, with a fold change of −1.084 and a p-value of 1.53 × 10−27. In contrast to the glioblastoma data set, the COPA score of this data set did not reach statistical significance, suggesting that the DNA copy number loss was not restricted to a subset of cases, but was a frequent phenomenon that occurred in a larger fraction of cases. In addition, as summarized in , the COPA analysis revealed DNA copy number loss of the NIRF gene in multiple kinds of malignancies originating from the brain,Citation48,Citation52,Citation53 breast,Citation54,Citation55 stomach,Citation55 kidney,Citation56 hematopoietic tissueCitation57 and lung.Citation55,Citation58,Citation59 In all of these data sets, the statistical indices for the COPA scores were again quite significant (p < 1 × 10−8).
In the next step, we aimed to characterize the chromosomal aberrations involving the NIRF gene. NIRF and its neighboring genes are localized on chromosome 9p24.1 (). According to previous publications, the nearby region harbors multiple cancer genes.Citation60–Citation68 To decipher the critical gene relevant to tumorigenesis, a multi-gene analysis (coexpression analysis applied to array comparative genomic hybridization (CGH) studies) was performed on Oncomine to identify the position and length of the deleted loci (). This analysis enabled us to compare the DNA contents across the entire length of the region. When a data set for non-small cell lung carcinomaCitation69 was analyzed, chromosomal deletions eliminating the NIRF gene were found (). The deletions involving NIRF spanned either a longer DNA fragment (e.g., from KANK1, 9p24.3 to CDKN2A, 9p21.3) or a shorter fragment (e.g., from IL33 to GLDC in 9p24.1; ). The shorter deletions clearly indicated the recurrent occurrence of a microdeletion targeting NIRF and GLDC (glycine decarboxylase). Since GLDC has not been inferred to have a linkage to tumors, we presume that the NIRF gene would be the main target of the deletion. According to the information provided by Compendia Bioscience, the incidence of deletion of the NIRF gene was estimated to be 13.3% (26 of 196 cases) on the basis of the log2 copy number units of less than −0.30 (), which represents hemizygous deletion. This incidence was comparable to those of the LOHs detected in cancers from the stomach and colon (). In summary, these findings suggest that NIRF is the focus of the microdeletion in a subset of this non-small cell lung carcinoma data set.
To examine the NIRF mRNA levels in related lung carcinoma entities, two data sets reporting squamous cell lung carcinomaCitation70 () and lung adenocarcinomaCitation71 () were analyzed. Both of these are subtypes of non-small cell lung carcinoma. Each data set revealed that the NIRF mRNA levels were reduced at the top rank among the genes localized in 9p24. In both cases, the reduction in the NIRF mRNA level was statistically significant (p = 1.15 × 10−7 in ; p = 0.0139 in ). Thus, there appear to be consistencies between the DNA copy number losses and the mRNA expression reductions of NIRF in nonsmall cell lung carcinoma.
The above observations collectively imply associations between the genetic status of NIRF and the genesis of several types of tumors. If so, it is possible that there is a certain genetic locus in the vicinity of the NIRF gene that is linked to the predisposition to tumors. shows a magnified map of the commonly deleted region found in the above-described non-small cell lung carcinoma data set, i.e., from IL33 to GLDC ( and B). Using literature mining, we found the SNP rs719725, which maps at 47 Mb upstream of the NIRF gene and has recently been reported to be associated with the risk of colorectal carcinoma.Citation25 This SNP was not only mapped close to NIRF but also localized just within the commonly deleted region observed in the nonsmall cell lung carcinoma data set (compare and ). Thus, these findings imply that there might be a certain relationship between NIRF, rs719725 and predisposition to cancer.
Discussion
NIRF appears to represent an intermodular hub protein that interacts with the core components of the cell cycle machinery and exhibits a unique centrality in the hierarchical modular architecture of the cell cycle network. NIRF ubiquitinates the two different classes of G1 cyclins (D1 and E1), and induces G1 arrest. DNA copy number loss in various malignancies was found for the NIRF gene, which was the target of a recurrent microdeletion in non-small cell lung carcinoma. In addition, the NIRF gene was closely linked with a susceptibility locus of colorectal carcinoma. These observations suggest that NIRF is a novel component of the core cell cycle machinery and is also a novel tumor suppressor.
Hub proteins hold the whole network together and are therefore important in both the topology maintenance and functioning of the network.Citation2,Citation4 The analysis of the NIRF PPI network indicated its scale-free property, and demonstrated the presence of a few highly connected hubs in the cell cycle network. Applying the top 20% as a general threshold,Citation23 the degree and centrality indices for NIRF were sufficiently high that NIRF can be judged to be a hub protein with high betweenness. While the degree and centrality indices of NIRF were not among the top-ranked ones, it is often mentioned that more extensively studied proteins are prone to investigative bias to have more interactions than less intensely studied proteins.Citation72–Citation74 In addition, the centrality indices of a node tend to be correlated with its degree,Citation44,Citation75 indicating that there are also biases in the centrality indices of the well-known proteins in the network. Accordingly, it is feasible that the centrality indices as well as the degree determined for NIRF in this study are all underestimated. More interacting partners will probably be found for NIRF in the near future, which will raise its centrality indices even higher.
The cell cycle network directs not only cell cycle progression, but also diverse cellular processes,Citation1 in which NIRF behaves as an intermodular hub protein.Citation5,Citation7 NIRF is a hub protein that characteristically interacts with the principal components of the cell cycle network, i.e., cyclins and their CDKs, pRB and p53. Each of these molecules is also a hub protein, with profound influences on different aspects of the cell cycle. These hub proteins individually interact with many other proteins composing the respective functional modules, which as a whole constitute a huge PPI network.Citation6 Eventually, the cell cycle network organized in this way not only regulates the cell cycle but also orchestrates versatile cellular functions such as cell growth, death, differentiation and senescence.Citation1 In this sense, the cell cycle network itself can be viewed as occupying a pivotal position in the cellular interactome network comprising a multitude of signaling pathways. On the other hand, NIRF is an intermodular hub protein with high betweenness, and is thereby supposed to occupy a unique critical position not only in the cell cycle network but also in the whole information network of the cell. As an intermodular hub protein connecting network modules, NIRF may be involved in the coordination of diverse information flows related to cell cycle control. Importantly, mutations of intermodular hubs are frequently associated with carcinogenesis.Citation7,Citation76 This is because such aberrations in intermodular hubs result in abnormalities in both network organization and information flow, culminating in global dysfunction of the network.Citation7,Citation8 Accordingly, it is possible that abnormalities in the NIRF gene may abrogate the integrity of the cell cycle network, which might ultimately bring about tumorigenesis.
NIRF is capable of ubiquitinating both cyclins D1 and E1 that cooperate with each other to control the G1/S transition.Citation1 This is consistent with the idea that NIRF is a cell cycle protein involved in G1/S inhibition.Citation20 The ubiquitination of these G1 cyclins has been assigned to the SCF-type ubiquitin ligases.Citation12,Citation17 Our findings demonstrate that NIRF is another ubiquitin ligase for cyclins D1 and E1. Intriguingly, there are some differences in the ubiquitinating properties between NIRF and the SCF ubiquitin ligases. First, the SCF ubiquitin ligases are only able to target either cyclin D1 or E, but not both,Citation18 thereby allowing individual control for each cyclin. In contrast, NIRF ubiquitinates both G1 cyclins, which could provide coordinated regulation at the G1/S boundary. Second, NIRF seems to directly bind to the G1 cyclins independently of phosphorylation, as shown by our in vitro assay results that NIRF binds to cyclin D1 and E1 and directly promotes their ubiquitination. In contrast, the SCF ligases recognize the cyclins only when they are phosphorylated.Citation13–Citation16 It is noteworthy that, in addition to the phosphorylation-dependent ubiquitination, phosphorylation-independent ubiquitination pathways have been proposed for both cyclins D1Citation13 and E.Citation77 To the best of our knowledge, NIRF is the first example of a single-subunit ubiquitin ligase grouped into the latter category. These differences between NIRF and the SCF ligases suggest a unique mechanism underlying the substrate recognition by NIRF. In addition, our results highlight a possible characteristic property of NIRF as a hub-type ubiquitin ligase targeting multiple substrates for ubiquitination. Thus, in cases with abnormalities of NIRF, the control for both cyclins D1 and E might become coincidentally abrogated, which could readily result in impaired control at the G1/S boundary. This is one possible scenario in which defects in an intermodular hub with ubiquitin ligase activity disrupt the integrity of the cell cycle control, ultimately leading to tumor formation. A similar related example has been proposed for BRCA1.Citation7
From the above considerations, we hypothesized that NIRF may be involved in the genesis of malignancies as a novel tumor suppressor. In support of this, the NIRF gene is localized in 9p24, which is deleted in numerous types of tumors at the highest frequencyCitation46 and where many publications have proposed the presence of various tumor-associated genes.Citation60–Citation66 Indeed, our database analyses provided findings compatible with our hypothesis. A significant loss of the DNA copy number of the NIRF gene was shown in several types of tumors. In particular, a recurrent microdeletion targeting NIRF was found in non-small cell lung carcinoma. Consistently, the NIRF mRNA was reduced in lung adenocarcinoma and squamous cell lung carcinoma, both of which are subtypes of non-small cell lung carcinoma. These analytic findings suggest that loss of the NIRF gene is associated with tumor formation, supporting the concept that NIRF is a tumor suppressor. This is in good agreement with the observation that NIRF induces G1 arrest, since many tumor suppressors function at the G/S boundary to inhibit inappropriate cell cycle progression.Citation1,Citation37
To our surprise, the SNP rs719725, a reported susceptibility locus for colorectal carcinoma,Citation25 is present in the immediate vicinity of the NIRF gene, further strengthening the possible relationship of NIRF with carcinogenesis. Of note, this SNP is localized just within the commonly deleted region observed in the non-small cell lung carcinoma data set. This observation indicates that this SNP locus is really a target of chromosomal aberration in a particular type of cancer. It also suggests that a single deletion event can simultaneously affect both the NIRF gene and this SNP locus. Although the SNP rs719725 has not been known to be linked with the risk of non-small cell lung carcinoma, it is not surprising that a single locus is associated with predispositions to multiple types of tumors. Therefore, the above observations meet our expectation that a chromosomal deletion of the NIRF gene might be involved in the formation of tumors, again raising the probability that NIRF functions in tumor suppression.
To characterize NIRF as a unique hub protein in the cell cycle network, several more points should be taken into account. First, NIRF interacts with the two major tumor suppressors (pRB and p53) as well as the two different G1 cyclins (cyclins D1 and E1), all of which are the critical regulators of the G1 checkpoint system. The G1 checkpoint is the most critical barrier that hinders cells from undergoing malignant transformation.Citation78 Given that NIRF exhibits several properties compatible with a tumor suppressor, these interactions suggest an intense functional interplay between NIRF and all of the above cell cycle proteins to ensure the precise functioning of the G1 checkpoint control. NIRF might cooperate with pRB and p53 to effectively induce G1 arrest in response to various insults that trigger DNA damage. Second, NIRF also interacts with the mitotic cyclins (cyclins A2 and B1), suggesting other roles for NIRF in the intra-S and G2/M controls. These are novel findings that demonstrate a distinguishing characteristic of NIRF, since no other ubiquitin ligases interact with all the members of the cyclin family. These observations also suggest the possibility that a common mechanism is responsible for the binding between NIRF and the different classes of cyclins (manuscript in preparation). Lastly, NIRF has a paralogous ubiquitin ligase, Np95/ICBP90/UHRF1, with opposing effects on cell cycle progression.Citation31,Citation32 This paralog has been reported to be involved in the epigenetic inheritance of DNA methylation through an association of its SRA domain with DNMT1.Citation79 While our study characterized the relationships of NIRF with the PPI network, ubiquitination and genetic aberration, corresponding studies regarding Np95/ICBP90/UHRF1 have not yet been reported. A comparative study will be informative to better define the role for this family in cell cycle control, in light of their associations with cancer. In addition, there is a high degree of sequence conservation between the SRA domains of NIRF and Np95/ICBP90/UHRF1,Citation21,Citation39 suggesting that NIRF also functions in epigenetic regulation. In this regard, a further study should be performed to examine the possible biological couplings among the cell cycle, ubiquitination and epigenetic regulations by NIRF and Np95/ICBP90/UHRF1.
In summary, based on the characteristic centrality within the hierarchical modular architecture of the cell cycle network, NIRF is supposed to influence multiple signaling modules related to cell cycle control. It is also suggested that NIRF induces G1 arrest by ubiquitinating both cyclins D1 and E1 and thereby acts as a tumor suppressor. We propose that NIRF occupies a pivotal position within the global information network of the cells, thus defining a critical nodal point connecting multiple signaling modules whose defect is associated with tumorigenesis.
Materials and Methods
Cell culture and reagents.
HEK293, HeLa and COS-7 cells were obtained from the ATCC and maintained in DMEM high glucose (4.5 g/L; Sigma) supplemented with 10% fetal calf serum.
Antibodies.
The following antibodies were obtained from commercial sources: anti-Flag (clone M2) and anti-cyclin D1 (DCS-6) from Sigma; anti-Myc (9E10) and anti-cyclin E (C-19) from Santa Cruz Biotechnology; anti-ICBP90 (Cat# 612264) and anti-Rb (Cat# 610884) from BD Biosciences. The secondary antibodies used were: alkaline phosphatase-conjugated antimouse IgG (Cat# 170-6520; Bio-Rad) and alkaline phosphataseconjugated anti-rabbit IgG (Cat# A-3687; Sigma).
Constructs.
The cDNAs for cyclins (A2, B1, D1 and E1), pRB and p53 were ligated into p3xFlag-CMV-10 (Sigma), pCMV-Myc (BD Biosciences) or pGEX4T1 (GE Healthcare, Buckinghamshire, UK). The NIRF cDNACitation21 was cloned into p3xFlag-CMV-10, pCMV-Myc and pEGFP-C1 (Clontech).
FACS analysis.
To assess the effects of NIRF on the cell cycle profile, pEGFP-C1-NIRF was transfected into HEK293 cells with the Effectene reagent (Qiagen) as recommended by the manufacturer. After 48 h, the cells were harvested by trypsinization, treated with 0.5% paraformaldehyde and fixed with 70% ethanol. After the fixation, the cells were stained with propidium iodide (PI), and subjected to scanning by a FACSCalibur (BD Biosciences). The transfected cells were gated by the EGFP fluorescence, and the PI fluorescence was measured for the cell cycle profiles.Citation20 A total of 1–5 × 105 cells were counted for each assay, and the cell cycle profiles were analyzed with the CellQuest and ModFit programs (BD Biosciences).Citation80
Screening of NIRF-interacting proteins.
The antibody macroarray membrane (Cat# HM3000; Hypromatrix Inc.) contained 400 antibodies spotted on it. Cell lysates were prepared from HEK293 cells transfected with either p3xFlag-NIRF or p3xFlag empty plasmid and overlaid on the membrane. The interactors were visualized using an alkaline phosphataseconjugated anti-Flag antibody (Sigma). The membrane was reacted with the CDP-Star reagent (GE Healthcare), and then exposed to X-ray film. The signal intensities were counted after scanning on an ES-10000G scanner (Epson).
Co-IP assay.
For co-IP assays, plasmids were transfected into COS-7, HEK293 or HeLa cells using the Effectene reagent. The medium was changed once at 24 h after transfection, and the cells were cultured for a further 24 h with or without MG-132 (Peptide Institute). The cells were lysed in 1 ml/dish of ice-cold lysis buffer [50 mM Tris-HCl pH 7.4, 150 mM NaCl, 5 mM EDTA, 0.5% NP-40, 1% protease inhibitor cocktail (Cat# P8340; Sigma)] for 30 min, followed by centrifugation at 14,000x g for 10 min each. The supernatants were used as cell extracts. The protein concentrations of the cell extracts were estimated using a BCA protein assay kit (Pierce), and cell extracts with an equivalent protein amount were used for immunoprecipitation.
Immunoprecipitation of Flag-tagged proteins was performed using a Flag-tagged protein immunoprecipitation kit (Sigma) according to the manufacturer's recommendations. Briefly, 30 µl of anti-Flag M2 affinity gel was added to 1 ml of cell extract, and the sample was shaken for 2 h at 0°C. After washing the gels three times with washing buffer (50 mM Tris-HCl pH 7.4, 150 mM NaCl, 5 mM EDTA, 0.1% NP-40) and once with phosphate-buffered saline, the Flag-tagged proteins were eluted using 50 µl of 3xFlag peptide solution (150 ng/µl). The eluted proteins were subjected to protein gel blot analysis, and the signals were detected with a Cool Saver (ATTO).
For immunoprecipitation of myc-tagged proteins, 1 ml of cell extract was incubated with 30 µl of anti-myc affinity gel (Santa Cruz Biotechnology). After the sample was shaken for 2 h at 0°C, the gel was washed three times with the washing buffer. The immunoprecipitates bound to the gel were dissolved in SDS-PAGE sample buffer and subjected to protein gel blot analysis.
GST pull-down assay.
GST pull-down assays were performed with a series of GST-tagged proteins expressed in bacteria and the 3xFlag-NIRF protein expressed in HEK293 cells as follows. The cDNAs for cyclin D1, cyclin E1, p53 and pRB379–928 (large pocket fragment of pRB) Citation81 were ligated in-frame into the pGEX4T1 vector to produce GST-tagged proteins in Escherichia coli strain BL21. Recombinant proteins were purified using glutathione-Sepharose (GE Healthcare) according to the manufacturer's recommendations. The 3xFlag-NIRF protein was expressed in HEK293 cells, and cell lysates were mixed with the GST-tagged proteins absorbed on 30 µl of the glutathione-Sepharose beads in 1 ml of binding buffer (phosphate-buffered saline, 5 mM EDTA, 1% Triton X-100, 1% protease inhibitor cocktail). After shaking for 2 h on ice, the beads were vigorously washed three times with the binding buffer, followed by two washes with the same buffer without detergent. The proteins on the beads were eluted by boiling with SDS-PAGE sample buffer and subjected to protein gel blot analysis. The 3xFlag-NIRF protein was visualized on the membrane with an anti-Flag antibody. As controls for bacterially expressed proteins, the SDS-PAGE gel was stained with Coomassie brilliant blue (CBB).
In vivo ubiquitination assay.
In vivo ubiquitination assays were conducted by transfecting COS-7 cells with expression plasmids encoding myc-tagged cyclin or 3xFlag-tagged ubiquitin, a NIRF-encoding plasmid or empty vector. At 24 h after transfection, the cells were treated with MG-132 or left untreated. The cells were cultured for another 24 h, and cell extracts were prepared. The extracts were immunoprecipitated using an anti-myc antibody, followed by protein gel blot analysis.
In vitro ubiquitination assay.
COS-7 cells were transfected with p3xFlag-NIRF, and the 3xFlag-NIRF protein (Flag-NIRF) was purified by immunoaffinity purification by combining the use of anti-Flag M2 beads (Sigma) and 3xFlag peptide (Sigma). GST-NIRF, GST-cyclin D1 and GST-cyclin E1 were purified from E. coli transformed with the pGEX4T1-fusion plasmid using glutathione-Sepharose. In vitro ubiquitination assays were performed by combining E1 (Merck), E2 (UbcH5a; Merck), GST-cyclin substrate, ATP, Flag-NIRF (for cyclin D1) or GST-NIRF (for cyclin E1) and Flag-ubiquitin (Sigma). The reaction mixture was incubated at 37°C for 1 h, followed by immunoprecipitation with an anti-Flag antibody. The ubiquitinated substrates were visualized by protein gel blotting.
Network analysis.
The Cytoscape softwareCitation33 was used to visualize and analyze the PPI network involving NIRF. The PPI information in humans for cyclins (A2, B1, D1 and E1), pRB and p53 were retrieved from the Pathway Commons database.Citation34 Our interaction data for NIRF were merged with the database information using the Advanced Network Merge plug-in (http://cytoscape.wodaklab.org) on Cytoscape. The deduced network was treated as an undirected network and subjected to an examination using the Network Analyzer plug-in based on the following means of computation.Citation82 The shortest path length L(n, m) is the minimum number of edges between two nodes n and m. The shortest path length distribution gives the number of node pairs (n, m) with L(n, m) = k for k = 1, 2,…. The degree of a node n is defined as the number of neighbors linked to n. The node degree distribution gives the number of protein nodes P(k) with a given degree k for k = 0, 1,…. The clustering coefficient is a measure of the tendency of proteins to form clusters or groups.Citation3 The clustering coefficient Cn of a node n is defined as Cn = 2en /[kn(kn − 1)], where kn is the number of neighbors of node n and en is the number of connected pairs between all neighbors of n. The average clustering coefficient distribution gives the average of the clustering coefficients, C(k), for all nodes with a given degree k for k = 2, 3,…. The topological coefficient is a relative measure of the extent to which a protein in the network shares interaction partners with other proteins.Citation41 The topological coefficient TCn of a node n with degree kn is defined as TCn = avg[J(n, m)]/kn, where J(n, m) is the number of neighbors shared between nodes n and m, plus one if there is a direct link between n and m. The value J(n, m) is defined for all nodes m that share at least one neighbor with n. The topological coefficient distribution gives the topological coefficient TC(k) with a given degree k for k = 2, 3,…
Centrality index.
Centrality measures of protein nodes in the network were computed on Cytoscape with the Network Analyzer plug-inCitation82 based on the following equations. Betweenness centrality represents how influential a node is in communicating between node pairs.Citation22 The betweenness centrality Cb(n) of node n was computed as follows: Cb(n) = ∑s≠n≠t [σst(n)/σst], where s and t are nodes in the network different from n, σst is the number of shortest paths from s to t, and σst(n) is the number of shortest paths from s to t that n lies on.Citation82 Closeness centrality is a measure of how fast information spreads from a given node to other nodes.Citation44 The closeness centrality Cc(n) of node n is defined as the reciprocal of the average shortest path length and is computed as follows: Cc(n) = 1/avg[L(n, m)], where L(n, m) is the length of the shortest path between two nodes n and m.Citation82
Database mining.
The OncomineCitation47 Research Premium Edition (Compendia Bioscience) was used for retrieving the public cancer database information. Through Oncomine, the alterations in the DNA copy numbers and mRNA expression levels were retrieved.
COPA.
The COPA function implemented in Oncomine was used to assess the alterations in DNA copy numbers in tumor cases. COPA is an innovative bioinformatic method for analyzing cancer genes on a microarray.Citation49 Since tumors are extremely heterogeneous in their genetic alterations,Citation83 most alterations only occur in a small subset of cases.Citation84 Accordingly, conventional analytical methods such as Student's t-test often fail to identify the cancerous abnormalities. In contrast, COPA is considered to be a sensitive and specific means for identifying cancerous aberrations, even those that only occur in a subset of cases.Citation50
According to the database provider (Compendia Bioscience), the COPA scores at the 5, 10 and 25 percentiles of cases were used to determine the statistical significance.
Multi-gene analysis.
The multi-gene analysis function in Oncomine was applied to the array-CGH data set to examine the deleted loci on chromosomes. The obtained information regarding the genes located on chromosome 9p21–24 was sorted according to the chromosomal map of NCBI MAP Viewer, build 37.2 (www.ncbi.nlm.nih.gov/projects/mapview).
Statistical analysis.
Statistical analyses were performed using functions implemented in Oncomine. The unpaired Student's t-test was used.
Disclosure of Potential Conflicts of Interest
No potential conflicts of interest were disclosed.
Abbreviations
CBB | = | coomassie brilliant blue |
CDK | = | cyclin-dependent kinase |
CGH | = | comparative genomic hybridization |
COPA | = | cancer outlier profile analysis |
GST | = | glutathione S-transferase |
IP | = | immunoprecipitation |
LOH | = | loss of heterozygosity |
NIRF | = | Np95/ICBP90-like RING finger protein |
PPI | = | protein-protein interaction |
SCF | = | Skp1/Cul1/F-box protein |
SNP | = | single nucleotide polymorphism |
UHRF2 | = | ubiquitin-like with PHD and ring finger domains 2 |
Figures and Tables
Figure 1 NIRF induces G1 arrest. (A) Rapidly growing HEK293 cells were transiently transfected with pEGFP-NIRF (pEGFP-C1-NIRF). After 48 h, the cells were harvested, and the cell cycle profiles were analyzed by FACS as described by Li et al.Citation20 The green fluorescent signal of EGFP was employed as the transfection marker, while PI staining was used to count the DNA contents of the cells. (B) The pEGFP-C1 empty vector was transfected and the cell cycle was analyzed as described for (A).
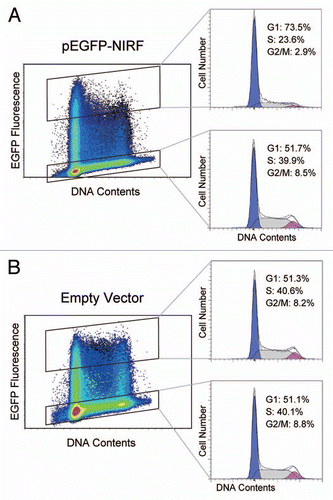
Figure 2 Antibody macroarray screening shows multiple binding partners of NIRF (see Table S1). A lysate of HEK293 cells transfected with Flag-NIRF (p3xFlag-NIRF) was overlaid on the membrane, and the spots for the interactors were visualized by an alkaline phosphatase-conjugated anti-Flag antibody. Enlarged images are also indicated for the areas corresponding to the cell cycle regulators with strong binding signals.
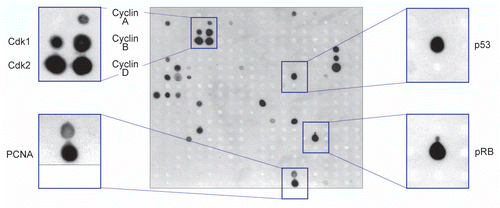
Figure 3 NIRF interacts with cyclins, p53 and pRB. (A) NIRF interacts with cyclins in vivo. COS-7 cells were cotransfected with Flag-NIRF (p3xFlag-NIRF) and myc-cyclins (pCMV-Myc-cyclin A2, B1, D1 or E1) or the empty vectors. MG-132 (5 µM) was added when required. Immunoprecipitation (IP) was performed with an anti-Flag antibody, and analyzed by protein gel blotting (Wesern blot) with the indicated antibodies (left). As a reciprocal IP, myc-NIRF (pCMV-Myc-NIRF) and Flag-cyclins (p3xFlag-cyclins) were used (right). Asterisks represent the ubiquitinated proteins. (B) NIRF interacts with p53 in vivo. HEK293 cells were cotransfected with Flag-NIRF and myc-p53 or the empty vectors. Immunoprecipitation was performed with an anti-Flag antibody, and analyzed by protein gel blotting with the indicated antibodies (left). Reciprocally, myc-NIRF and Flag-p53 were used (right). (C) NIRF interacts with pRB in vivo. HeLa cells were cotransfected with myc-NIRF and Flag-pRB or the empty vectors. Immunoprecipitation was performed with an anti-Flag antibody, and analyzed by protein gel blotting with the indicated antibodies (left). In a reciprocal experiment, Flag-NIRF or empty vector was transfected. Immunoprecipitation was performed with an anti-Flag antibody, and the immunoprecipitates were analyzed by protein gel blotting with the indicated antibodies (right). (D) NIRF interacts with cyclin D1, cyclin E1, p53 and pRB in vitro. The Flag-NIRF protein was expressed in HEK293 cells, and the cell lysate was used for GST pull-down assays with GST, GST-cyclin D1, GST-cyclin E1, GST-p53 or GST-pRB379–928 (large pocket fragment of pRB).Citation81 The SDS-PAGE gel was stained with Coomassie brilliant blue (CBB) while the protein gel blot was probed with an anti-Flag antibody.
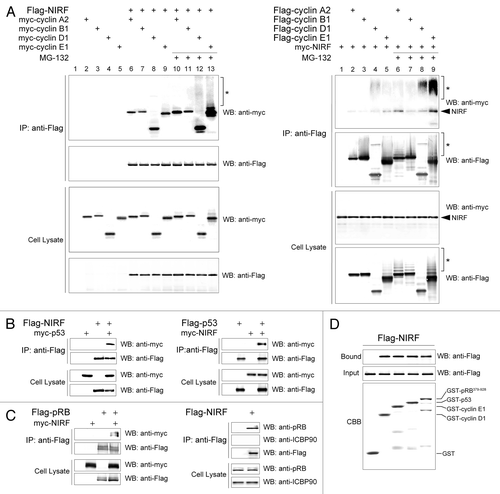
Figure 4 NIRF promotes ubiquitination of cyclins D1 and E1. (A) NIRF ubiquitinates cyclins D1 and E1 in vivo. COS-7 cells were cotransfected with the indicated combinations of plasmids, and then incubated with or without the proteasome inhibitor MG-132. Ubiquitinated cyclins were detected by immunoprecipitation (IP) with an anti-myc antibody, followed by protein gel blot (WB) analysis with the indicated antibodies. Asterisks denote the ubiquitinated proteins, while arrows correspond to nonspecific signals irrelevant to transfection. (B) NIRF ubiquitinates cyclins D1 and E1 in vitro. In vitro ubiquitination assays were performed with the indicated combinations of GST-tagged substrates, E1, E2 (UbcH5a), Flag-ubiquitin (Ub), ATP and either Flag-NIRF or GST-NIRF. After completion of the reaction, the mixtures were subjected to immunoprecipitation with an anti-Flag antibody. The ubiquitinated species (asterisks) were visualized by protein gel blotting with the antibodies against the respective cyclins. Note that the same amount of GST-cyclin substrates was used in each experiment. The arrowheads represent GST-cyclin D1 or E1 proteins captured during the process of immunoprecipitation.
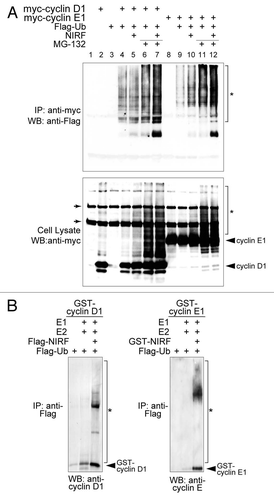
Figure 5 The NIRF interaction network. The PPI information for the core cell cycle proteins (cyclin A2, cyclin B1, cyclin D1, cyclin E1, pRB and p53) were retrieved from the Pathway Commons database.Citation34 Our NIRF interaction data were merged with the retrieved information using the Cytoscape softwareCitation33 as described in the Materials and Methods. The symbols are as follows: yellow, cyclins; yellow-green, CDKs; blue, two major tumor suppressors; green, NIRF-interacting proteins included in the retrieved database information. The thicker black lines indicate interactions demonstrated by more than two methods. The interactions between NIRF and PCNA or HDAC1Citation39 were shown by both the antibody macroarray and the database information. The thinner black lines denote interactions shown by either the antibody macroarray or the pre-existing information in the database. Gray lines indicate other PPI information from the database.
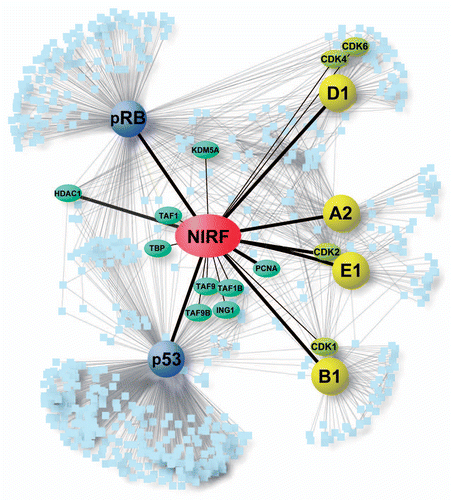
Figure 6 Topological properties of the NIRF interaction network. The NIRF PPI information was subjected to network analyses as described in the Materials and Methods. (A) Shortest path length distribution. The distribution of the shortest path lengths between protein nodes is indicated. The average shortest path length was 2.69. (B) Node degree distribution. The number of protein nodes P(k) with a given degree (k) approximates a power law: P(k) ∼ k−γ; γ = 0.971. Correlation = 0.933, R2 = 0.673. (C) Average clustering coefficient distribution. The average clustering coefficient C(k) of nodes with a given degree (k) follows a power law: C(k) ∼k−γ; γ = 1.210. Correlation = 0.597, R2 = 0.838. (D) Topological coefficient distribution. The topological coefficient TC(k) of nodes with a given degree (k) approximates a power law: TC(k) ∼k−γ; γ = 0.826. Correlation = 0.937, R2 = 0.931. The centrality indices of NIRF were as follows: betweenness centrality, 5.50 × 10−3 (ranked in the top 0.45%, 11th in 2,466 nodes); closeness centrality, 0.475 (top 1.2%, 30th); degree, 19 (top 0.45%, 11th).
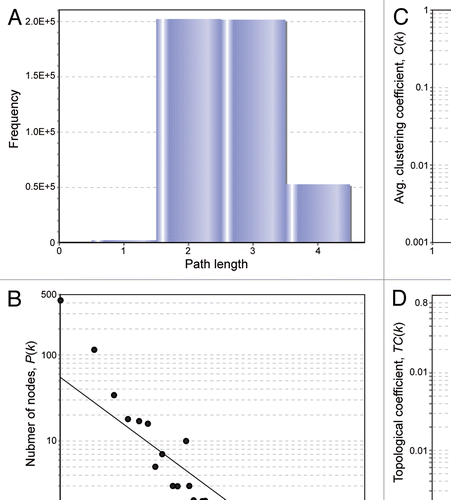
Figure 7 Loss of the NIRF gene in tumor cases. (A) LOH at the NIRF locus in tumors. The genomic DNA was extracted from tumors or the surrounding normal tissues. LOH was determined using the polymorphic markers D9S281 and D9S1852 (left). Summary of the LOH analyses. Fifteen samples were analyzed for each tumor type. LOHs were found in the indicated tumors at varying frequencies. The LOHs at both polymorphic markers coincided in all cases (right). (B) DNA copy number profiles of the NIRF gene in a glioblastoma data set registered in the Oncomine databaseCitation47 (TCGA Brain 2, brain glioblastoma one sample t-test).Citation48 The COPA score at the 10 percentile was −52.222, which was ranked in the top 0.2% of the analyzed genes (37th in 18,823 genes) (p < 1 × 10−8). (C) DNA copy number profiles of the NIRF gene in a lung adenocarcinoma data set in Oncomine (Weir Lung, lung adenocarcinoma one sample t-test).Citation51 The fold change in the mean copy number was −1.084 (p = 1.53 × 10−27).
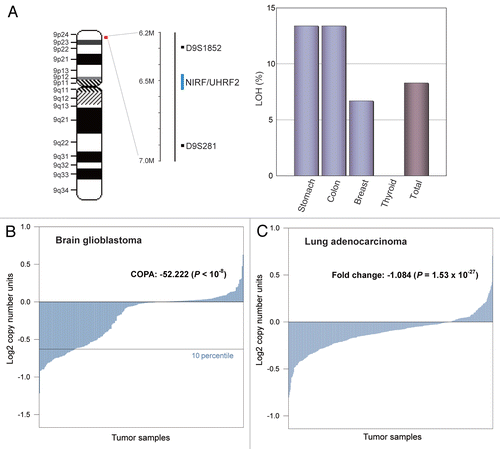
Figure 8 The NIRF gene is the target of a microdeletion in non-small cell lung carcinoma. (A) Chromosomal localization of a commonly deleted region at 9p24. The genes present in 9p21.3–24.3 are indicated according to NCBI MAP Viewer (www.ncbi.nlm.nih.gov/projects/mapview). The green box indicates the NIRF gene, while the gray box indicates the deleted region with a focus on this gene. (B) A recurrent micro-deletion targets the NIRF gene. A non-small cell lung carcinoma data set in Oncomine (Beroukhim Multi-cancer, non-small cell lung carcinoma one sample t-test) Citation69 was analyzed by applying a multigene analysis. The extents of the loss or increase in the DNA copy number are shown in blue and red, respectively, with graded intensities. The gray and green boxes correspond to those in (A).
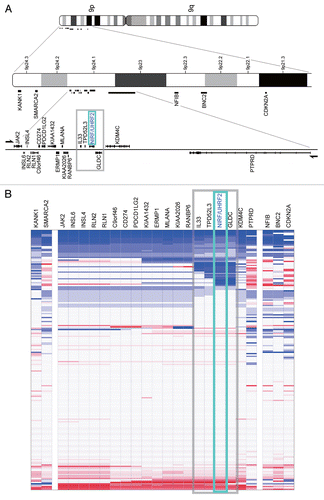
Figure 9 The SNP rs719725 is localized in the vicinity of the NIRF gene. The chromosomal region corresponding to the gray boxes in and B is shown. This region encompasses from IL33 to GLDC, and includes the SNP rs719725, which is localized at 47 kb upstream of the NIRF gene.Citation25
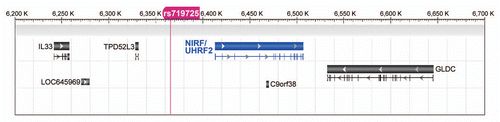
Table 1 Tumors with loss of the NIRF gene showing COPA scores ranked in the top 5%
Table 2 Underexpressed genes in 9p24 in squamous cell lung carcinoma compared with normal tissueCitation70
Table 3 Underexpressed genes in 9p24 in lung adenocarcinoma compared with normal tissueCitation71
Additional material
Download Zip (289.2 KB)Acknowledgments
We thank Y. Li, B. Zhang, Y. Chun, Y. Tohyama, K. Hoshi, Y. Yoshikawa, H. Minemura and Y. Yano for their help. We also thank Dr. M. Hatakeyama (Hokkaido University) for providing the pRB cDNA. We are especially grateful to Y. Xiao for the bioinformatic analysis, and Drs. I. Wada, Z. Yuan and Y. Hashimoto for critical advice.
References
- Massagué J. G1 cell cycle control and cancer. Nature 2004; 432:298 - 306; PMID: 15549091; http://dx.doi.org/10.1038/nature03094
- Albert R, Jeong H, Barabasi AL. Error and attack tolerance of complex networks. Nature 2000; 406:378 - 382; PMID: 10935628; http://dx.doi.org/10.1038/35019019
- Barabási AL, Oltvai ZN. Network biology: understanding the cell's functional organization. Nat Rev Genet 2004; 5:101 - 113; PMID: 14735121; http://dx.doi.org/10.1038/nrg1272
- Jeong H, Mason SP, Barabasi AL, Oltvai ZN. Lethality and centrality in protein networks. Nature 2001; 411:41 - 42; PMID: 11333967; http://dx.doi.org/10.1038/35075138
- Han JD, Bertin N, Hao T, Goldberg DS, Berriz GF, Zhang LV, et al. Evidence for dynamically organized modularity in the yeast protein-protein interaction network. Nature 2004; 430:88 - 93; PMID: 15190252; http://dx.doi.org/10.1038/nature02555
- Vidal M, Cusick ME, Barabasi AL. Interactome networks and human disease. Cell 2011; 144:986 - 998; PMID: 21414488; http://dx.doi.org/10.1016/j.cell.2011.02.016
- Taylor IW, Linding R, Warde-Farley D, Liu Y, Pesquita C, Faria D, et al. Dynamic modularity in protein interaction networks predicts breast cancer outcome. Nat Biotechnol 2009; 27:199 - 204; PMID: 19182785; http://dx.doi.org/10.1038/nbt.1522
- Wang YC, Chen BS. A network-based biomarker approach for molecular investigation and diagnosis of lung cancer. BMC Med Genomic 2011; 4:2; PMID: 21211025; http://dx.doi.org/10.1186/1755-8794-4-2
- Zabel C, Sagi D, Kaindl AM, Steireif N, Klare Y, Mao L, et al. Comparative proteomics in neurodegenerative and non-neurodegenerative diseases suggest nodal point proteins in regulatory networking. J Proteome Res 2006; 5:1948 - 1958; PMID: 16889417; http://dx.doi.org/10.1021/pr0601077
- Bashir T, Pagano M. Aberrant ubiquitin-mediated proteolysis of cell cycle regulatory proteins and oncogenesis. Adv Cancer Res 2003; 88:101 - 144; PMID: 12665054
- Pickart CM. Mechanisms underlying ubiquitination. Annu Rev Biochem 2001; 70:503 - 533; PMID: 11395416; http://dx.doi.org/10.1146/annurev.biochem.70.1.503
- Vodermaier HC. APC/C and SCF: controlling each other and the cell cycle. Curr Biol 2004; 14:787 - 796; PMID: 15380093; http://dx.doi.org/10.1016/j.cub.2004.09.020
- Lin DI, Barbash O, Kumar KG, Weber JD, Harper JW, Klein-Szanto AJ, et al. Phosphorylation-dependent ubiquitination of cyclin D1 by the SCF(FBX4-alphaB crystallin) complex. Mol Cell 2006; 24:355 - 366; PMID: 17081987; http://dx.doi.org/10.1016/j.molcel.2006.09.007
- Okabe H, Lee SH, Phuchareon J, Albertson DG, McCormick F, Tetsu O. A critical role for FBXW8 and MAPK in cyclin D1 degradation and cancer cell proliferation. PLoS ONE 2006; 1:128; PMID: 17205132; http://dx.doi.org/10.1371/journal.pone.0000128
- Santra MK, Wajapeyee N, Green MR. F-box protein FBXO31 mediates cyclin D1 degradation to induce G1 arrest after DNA damage. Nature 2009; 459:722 - 725; PMID: 19412162; http://dx.doi.org/10.1038/nature08011
- Koepp DM, Schaefer LK, Ye X, Keyomarsi K, Chu C, Harper JW, et al. Phosphorylation-dependent ubiquitination of cyclin E by the SCFFbw7 ubiquitin ligase. Science 2001; 294:173 - 177; PMID: 11533444; http://dx.doi.org/10.1126/science.1065203
- Nakayama KI, Nakayama K. Ubiquitin ligases: cell cycle control and cancer. Nat Rev Cancer 2006; 6:369 - 381; PMID: 16633365; http://dx.doi.org/10.1038/nrc1881
- Kitagawa K, Kotake Y, Kitagawa M. Ubiquitin-mediated control of oncogene and tumor suppressor gene products. Cancer Sci 2009; 100:1374 - 1381; PMID: 19459846; http://dx.doi.org/10.1111/j.1349-7006.2009.01196.x
- Mori T, Li Y, Hata H, Kochi H. NIRF is a ubiquitin ligase that is capable of ubiquitinating PCNP, a PEST-containing nuclear protein. FEBS Lett 2004; 557:209 - 214; PMID: 14741369; http://dx.doi.org/10.1016/S0014-5793(03)01495-9
- Li Y, Mori T, Hata H, Homma Y, Kochi H. NIRF induces G1 arrest and associates with Cdk2. Biochem Biophys Res Commun 2004; 319:464 - 468; PMID: 15178429; http://dx.doi.org/10.1016/j.bbrc.2004.04.190
- Mori T, Li Y, Hata H, Ono K, Kochi H. NIRF, a novel RING finger protein, is involved in cell cycle regulation. Biochem Biophys Res Commun 2002; 296:530 - 536; PMID: 12176013; http://dx.doi.org/10.1016/S0006-291X(02)00890-2
- Brandes U. A faster algorithm for betweenness centrality. J Math Sociol 2001; 25:163 - 177; http://dx.doi.org/10.1080/002.2250X.2001.9990249
- Yu H, Kim PM, Sprecher E, Trifonov V, Gerstein M. The importance of bottlenecks in protein networks: correlation with gene essentiality and expression dynamics. PLOS Comput Biol 2007; 3:59; PMID: 17447836; http://dx.doi.org/10.1371/journal.pcbi.0030059
- Gursoy A, Keskin O, Nussinov R. Topological properties of protein interaction networks from a structural perspective. Biochem Soc Trans 2008; 36:1398 - 1403; PMID: 19021563; http://dx.doi.org/10.1042/BST0361398
- Kocarnik JD, Hutter CM, Slattery ML, Berndt SI, Hsu L, Duggan DJ, et al. Characterization of 9p24 risk locus and colorectal adenoma and cancer: gene-environment interaction and meta-analysis. Cancer Epidemiol Biomarkers Prev 2010; 19:3131 - 3139; PMID: 20978172; http://dx.doi.org/10.1158/1055-9965.EPI-10-0878
- Ying H, Xiao ZX. Targeting retinoblastoma protein for degradation by proteasomes. Cell Cycle 2006; 5:506 - 508; PMID: 16552188; http://dx.doi.org/10.4161/cc.5.5.2515
- Lee JT, Gu W. The multiple levels of regulation by p53 ubiquitination. Cell Death Differ 2010; 17:86 - 92; PMID: 19543236; http://dx.doi.org/10.1038/cdd.2009.77
- Inuzuka H, Fukushima H, Shaik S, Wei W. Novel insights into the molecular mechanisms governing Mdm2 ubiquitination and destruction. Oncotarget 2010; 1:685 - 690; PMID: 21317463
- Lamothe B, Webster WK, Gopinathan A, Besse A, Campos AD, Darnay BG. TRAF6 ubiquitin ligase is essential for RANKL signaling and osteoclast differentiation. Biochem Biophys Res Commun 2007; 359:1044 - 1049; PMID: 17572386; http://dx.doi.org/10.1016/j.bbrc.2007.06.017
- Amemiya Y, Azmi P, Seth A. Autoubiquitination of BCA2 RING E3 ligase regulates its own stability and affects cell migration. Mol Cancer Res 2008; 6:1385 - 1396; PMID: 18819927; http://dx.doi.org/10.1158/1541-7786.MCR-08-0094
- Fujimori A, Matsuda Y, Takemoto Y, Hashimoto Y, Kubo E, Araki R, et al. Cloning and mapping of Np95 gene which encodes a novel nuclear protein associated with cell proliferation. Mamm Genome 1998; 9:1032 - 1035; PMID: 9880673; http://dx.doi.org/10.1007/s003359900920
- Hopfner R, Mousli M, Jeltsch JM, Voulgaris A, Lutz Y, Marin C, et al. ICBP90, a novel human CCAAT binding protein, involved in the regulation of topoisomerase IIalpha expression. Cancer Res 2000; 60:121 - 128; PMID: 10646863
- Cline MS, Smoot M, Cerami E, Kuchinsky A, Landys N, Workman C, et al. Integration of biological networks and gene expression data using Cytoscape. Nat Protoc 2007; 2:2366 - 2382; PMID: 17947979; http://dx.doi.org/10.1038/nprot.2007.324
- Cerami EG, Gross BE, Demir E, Rodchenkov I, Babur O, Anwar N, et al. Pathway Commons, a web resource for biological pathway data. Nucleic Acids Res 2011; 39:685 - 690; PMID: 21071392; http://dx.doi.org/10.1093/nar/gkq1039
- Legrain P, Selig L. Genome-wide protein interaction maps using two-hybrid systems. FEBS Lett 2000; 480:32 - 36; PMID: 10967325; http://dx.doi.org/10.1016/S0014-5793(00)01774-9
- Rigaut G, Shevchenko A, Rutz B, Wilm M, Mann M, Seraphin B. A generic protein purification method for protein complex characterization and proteome exploration. Nat Biotechnol 1999; 17:1030 - 1032; PMID: 10504710; http://dx.doi.org/10.1038/13732
- Sherr CJ. Principles of tumor suppression. Cell 2004; 116:235 - 246; PMID: 14744434; http://dx.doi.org/10.1016/S0092-8674(03)01075-4
- Takeda DY, Wohlschlegel JA, Dutta A. A bipartite substrate recognition motif for cyclin-dependent kinases. J Biol Chem 2001; 276:1993 - 1997; PMID: 11067844; http://dx.doi.org/10.1074/jbc.M005719200
- Unoki M, Nishidate T, Nakamura Y. ICBP90, an E2F-1 target, recruits HDAC1 and binds to methyl-CpG through its SRA domain. Oncogene 2004; 23:7601 - 7610; PMID: 15361834; http://dx.doi.org/10.1038/sj.onc.1208053
- Strogatz SH. Exploring complex networks. Nature 2001; 410:268 - 276; PMID: 11258382; http://dx.doi.org/10.1038/35065725
- Stelzl U, Worm U, Lalowski M, Haenig C, Brembeck FH, Goehler H, et al. A human protein-protein interaction network: a resource for annotating the proteome. Cell 2005; 122:957 - 968; PMID: 16169070; http://dx.doi.org/10.1016/j.cell.2005.08.029
- Maslov S, Sneppen K. Specificity and stability in topology of protein networks. Science 2002; 296:910 - 913; PMID: 11988575; http://dx.doi.org/10.1126/science.1065103
- Wang J, Zhang S, Wang Y, Chen L, Zhang XS. Disease-aging network reveals significant roles of aging genes in connecting genetic diseases. PLOS Comput Biol 2009; 5:1000521; PMID: 19779549; http://dx.doi.org/10.1371/journal.pcbi.1000521
- Newman MEJ. A measure of betweenness centrality based on random walks. Soc Networks 2005; 27:39 - 54; http://dx.doi.org/10.1016/j.socnet.2004.11.009
- Lee Y, Yang X, Huang Y, Fan H, Zhang Q, Wu Y, et al. Network modeling identifies molecular functions targeted by miR-204 to suppress head and neck tumor metastasis. PLOS Comput Biol 2010; 6:1000730; PMID: 20369013; http://dx.doi.org/10.1371/journal.pcbi.1000730
- Knuutila S, Aalto Y, Autio K, Bjorkqvist AM, El-Rifai W, Hemmer S, et al. DNA copy number losses in human neoplasms. Am J Pathol 1999; 155:683 - 694; PMID: 10487825; http://dx.doi.org/10.1016/S0002-9440(10)65166-8
- Rhodes DR, Kalyana-Sundaram S, Mahavisno V, Varambally R, Yu J, Briggs BB, et al. Oncomine 3.0: genes, pathways and networks in a collection of 18,000 cancer gene expression profiles. Neoplasia 2007; 9:166 - 180; PMID: 17356713; http://dx.doi.org/10.1593/neo.07112
- Cancer Genome Atlas Research Network. Comprehensive genomic characterization defines human glioblastoma genes and core pathways. Nature 2008; 455:1061 - 1068; PMID: 18772890; http://dx.doi.org/10.1038/nature07385
- MacDonald JW, Ghosh D. COPA—cancer outlier profile analysis. Bioinformatics 2006; 22:2950 - 2951; PMID: 16895932; http://dx.doi.org/10.1093/bioinformatics/btl433
- Ghosh D, Chinnaiyan AM. Genomic outlier profile analysis: mixture models, null hypotheses and non-parametric estimation. Biostatistics 2009; 10:60 - 69; PMID: 18539648; http://dx.doi.org/10.1093/biostatistics/kxn015
- Weir BA, Woo MS, Getz G, Perner S, Ding L, Beroukhim R, et al. Characterizing the cancer genome in lung adenocarcinoma. Nature 2007; 450:893 - 898; PMID: 17982442; http://dx.doi.org/10.1038/nature06358
- Beroukhim R, Getz G, Nghiemphu L, Barretina J, Hsueh T, Linhart D, et al. Assessing the significance of chromosomal aberrations in cancer: methodology and application to glioma. Proc Natl Acad Sci USA 2007; 104:20007 - 20012; PMID: 18077431; http://dx.doi.org/10.1073/pnas.0710052104
- Kotliarov Y, Steed ME, Christopher N, Walling J, Su Q, Center A, et al. High-resolution global genomic survey of 178 gliomas reveals novel regions of copy number alteration and allelic imbalances. Cancer Res 2006; 66:9428 - 9436; PMID: 17018597; http://dx.doi.org/10.1158/0008-5472.CAN-06-1691
- Haverty PM, Fridlyand J, Li L, Getz G, Beroukhim R, Lohr S, et al. High-resolution genomic and expression analyses of copy number alterations in breast tumors. Genes Chromosomes Cancer 2008; 47:530 - 542; PMID: 18335499; http://dx.doi.org/10.1002/gcc.20558
- Collins FS, Barker AD. Mapping the cancer genome. Pinpointing the genes involved in cancer will help chart a new course across the complex landscape of human malignancies. Sci Am 2007; 296:50 - 57; PMID: 17348159; http://dx.doi.org/10.1038/scientificamerican0307-50
- Beroukhim R, Brunet JP, Di Napoli A, Mertz KD, Seeley A, Pires MM, et al. Patterns of gene expression and copy-number alterations in von-hippel lindau disease-associated and sporadic clear cell carcinoma of the kidney. Cancer Res 2009; 69:4674 - 4681; PMID: 19470766; http://dx.doi.org/10.1158/0008-5472.CAN-09-0146
- Gunnarsson R, Isaksson A, Mansouri M, Goransson H, Jansson M, Cahill N, et al. Large but not small copy-number alterations correlate to high-risk genomic aberrations and survival in chronic lymphocytic leukemia: a high-resolution genomic screening of newly diagnosed patients. Leukemia 2010; 24:211 - 215; PMID: 19741724; http://dx.doi.org/10.1038/leu.2009.187
- Lu X, Zhang K, Van Sant C, Coon J, Semizarov D. An algorithm for classifying tumors based on genomic aberrations and selecting representative tumor models. BMC Med Genomics 2010; 3:23; PMID: 20569491; http://dx.doi.org/10.1186/1755-8794-3-23
- Sos ML, Michel K, Zander T, Weiss J, Frommolt P, Peifer M, et al. Predicting drug susceptibility of non-small cell lung cancers based on genetic lesions. J Clin Invest 2009; 119:1727 - 1740; PMID: 19451690; http://dx.doi.org/10.1172/JCI37127
- Sarkar S, Roy BC, Hatano N, Aoyagi T, Gohji K, Kiyama R. A novel ankyrin repeat-containing gene (Kank) located at 9p24 is a growth suppressor of renal cell carcinoma. J Biol Chem 2002; 277:36585 - 36591; PMID: 12133830; http://dx.doi.org/10.1074/jbc.M204244200
- Gunduz E, Gunduz M, Ali MA, Beder L, Tamamura R, Katase N, et al. Loss of heterozygosity at the 9p21–24 region and identification of BRM as a candidate tumor suppressor gene in head and neck squamous cell carcinoma. Cancer Invest 009; 27:661 - 668; PMID: 19488910; http://dx.doi.org/10.1080/07357900802563010
- Tefferi A, Vainchenker W. Myeloproliferative neoplasms: molecular pathophysiology, essential clinical understanding and treatment strategies. J Clin Oncol 2011; 29:573 - 582; PMID: 21220604; http://dx.doi.org/10.1200/JCO.2010.29.8711
- Kounalakis N, Goydos JS. Tumor cell and circulating markers in melanoma: diagnosis, prognosis and management. Curr Oncol Rep 2005; 7:377 - 382; PMID: 16091200; http://dx.doi.org/10.1007/s11912-005-0065-2
- Cao Q, Chen J, Zhu L, Liu Y, Zhou Z, Sha J, et al. A testis-specific and testis developmentally regulated tumor protein D52 (TPD52)-like protein TPD52L3/hD55 interacts with TPD52 family proteins. Biochem Biophys Res Commun 2006; 344:798 - 806; PMID: 16631610; http://dx.doi.org/10.1016/j.bbrc.2006.03.208
- Yang ZQ, Imoto I, Fukuda Y, Pimkhaokham A, Shimada Y, Imamura M, et al. Identification of a novel gene, GASC1, within an amplicon at 9p23–24 frequently detected in esophageal cancer cell lines. Cancer Res 2000; 60:4735 - 4739; PMID: 10987278
- Sato M, Takahashi K, Nagayama K, Arai Y, Ito N, Okada M, et al. Identification of chromosome arm 9p as the most frequent target of homozygous deletions in lung cancer. Genes Chromosomes Cancer 2005; 44:405 - 414; PMID: 16114034; http://dx.doi.org/10.1002/gcc.20253
- Smith-Sørensen B, Hovig E. CDKN2A (p16INK4A) somatic and germline mutations. Hum Mutat 1996; 7:294 - 303; PMID: 8723678; http://dx.doi.org/10.1002/(SICI)1098-1004(1996)7:4<294::AID-HUMU2>3.0.CO;2-9
- Murai R, Yoshida Y, Muraguchi T, Nishimoto E, Morioka Y, Kitayama H, et al. A novel screen using the Reck tumor suppressor gene promoter detects both conventional and metastasis-suppressing anticancer drugs. Oncotarget 2010; 1:252 - 264; PMID: 21304177
- Beroukhim R, Mermel CH, Porter D, Wei G, Raychaudhuri S, Donovan J, et al. The landscape of somatic copy-number alteration across human cancers. Nature 2010; 463:899 - 905; PMID: 20164920; http://dx.doi.org/10.1038/nature08822
- Talbot SG, Estilo C, Maghami E, Sarkaria IS, Pham DK, Oc P, et al. Gene expression profiling allows distinction between primary and metastatic squamous cell carcinomas in the lung. Cancer Res 2005; 65:3063 - 3071; PMID: 15833835
- Barrett T, Edgar R. Gene expression omnibus: microarray data storage, submission, retrieval and analysis. Methods Enzymol 2006; 411:352 - 369; PMID: 16939800; http://dx.doi.org/10.1016/S0076-6879(06)11019-8
- Barabási AL, Gulbahce N, Loscalzo J. Network medicine: a network-based approach to human disease. Nat Rev Genet 2011; 12:56 - 68; PMID: 21164525; http://dx.doi.org/10.1038/nrg2918
- Xu J, Li Y. Discovering disease-genes by topological features in human protein-protein interaction network. Bioinformatics 2006; 22:2800 - 2805; PMID: 16954137; http://dx.doi.org/10.1093/bioinformatics/btl467
- Yu H, Braun P, Yildirim MA, Lemmens I, Venkatesan K, Sahalie J, et al. High-quality binary protein interaction map of the yeast interactome network. Science 2008; 322:104 - 110; PMID: 18719252; http://dx.doi.org/10.1126/science.1158684
- Goh KI, Oh E, Kahng B, Kim D. Betweenness centrality correlation in social networks. Phys Rev E Stat Nonlin Soft Matter Phys 2003; 67:17101; PMID: 12636633; http://dx.doi.org/10.1103/PhysRevE.67.017101
- Akakura S, Nochajski P, Gao L, Sotomayor P, Matsui S, Gelman IH. Rb-dependent cellular senescence, multinucleation and susceptibility to oncogenic transformation through PKC scaffolding by SSeCKS/AKAP12. Cell Cycle 2010; 9:4656 - 4665; PMID: 21099353; http://dx.doi.org/10.4161/cc.9.23.13974
- Lu Z, Hunter T. Degradation of activated protein kinases by ubiquitination. Annu Rev Biochem 2009; 78:435 - 475; PMID: 19489726; http://dx.doi.org/10.1146/annurev.biochem.013008.092711
- Kastan MB, Bartek J. Cell cycle checkpoints and cancer. Nature 2004; 432:316 - 323; PMID: 15549093; http://dx.doi.org/10.1038/nature03097
- Sharif J, Muto M, Takebayashi S, Suetake I, Iwamatsu A, Endo TA, et al. The SRA protein Np95 mediates epigenetic inheritance by recruiting Dnmt1 to methylated DNA. Nature 2007; 450:908 - 912; PMID: 17994007; http://dx.doi.org/10.1038/nature06397
- Noonan EJ, Place RF, Basak S, Pookot D, Li LC. miR-449a causes Rb-dependent cell cycle arrest and senescence in prostate cancer cells. Oncotarget 2010; 1:349 - 358; PMID: 20948989
- Hatakeyama M, Weinberg RA. The role of RB in cell cycle control. Prog Cell Cycle Res 1995; 1:9 - 19; PMID: 9552350; http://dx.doi.org/10.1007/978-1-4615-1809-9_2
- Assenov Y, Ramirez F, Schelhorn SE, Lengauer T, Albrecht M. Computing topological parameters of biological networks. Bioinformatics 2008; 24:282 - 284; PMID: 18006545; http://dx.doi.org/10.1093/bioinformatics/btm554
- Akavia UD, Litvin O, Kim J, Sanchez-Garcia F, Kotliar D, Causton HC, et al. An integrated approach to uncover drivers of cancer. Cell 2010; 143:1005 - 1017; PMID: 21129771; http://dx.doi.org/10.1016/j.cell.2010.11.013
- Wu B. Cancer outlier differential gene expression detection. Biostatistics 2007; 8:566 - 575; PMID: 17021278; http://dx.doi.org/10.1093/biostatistics/kxl029
- Pekarsky Y, Croce CM. Is miR-29 an oncogene or tumor suppressor in CLL?. Oncotarget 2010; 1:224 - 227; PMID: 20936047