Abstract
Previously, we showed that high-energy metabolites (lactate and ketones) “fuel” tumor growth and experimental metastasis in an in vivo xenograft model, most likely by driving oxidative mitochondrial metabolism in breast cancer cells. To mechanistically understand how these metabolites affect tumor cell behavior, here we used genome-wide transcriptional profiling. Briefly, human breast cancer cells (MCF7) were cultured with lactate or ketones, and then subjected to transcriptional analysis (exon-array). Interestingly, our results show that treatment with these high-energy metabolites increases the transcriptional expression of gene profiles normally associated with “stemness,” including genes upregulated in embryonic stem (ES) cells. Similarly, we observe that lactate and ketones promote the growth of bonafide ES cells, providing functional validation. The lactate- and ketone-induced “gene signatures” were able to predict poor clinical outcome (including recurrence and metastasis) in a cohort of human breast cancer patients. Taken together, our results are consistent with the idea that lactate and ketone utilization in cancer cells promotes the “cancer stem cell” phenotype, resulting in significant decreases in patient survival. One possible mechanism by which these high-energy metabolites might induce stemness is by increasing the pool of Acetyl-CoA, leading to increased histone acetylation, and elevated gene expression. Thus, our results mechanistically imply that clinical outcome in breast cancer could simply be determined by epigenetics and energy metabolism, rather than by the accumulation of specific “classical” gene mutations. We also suggest that high-risk cancer patients (identified by the lactate/ketone gene signatures) could be treated with new therapeutics that target oxidative mitochondrial metabolism, such as the anti-oxidant and “mitochondrial poison” metformin. Finally, we propose that this new approach to personalized cancer medicine be termed “Metabolo-Genomics,” which incorporates features of both 1) cell metabolism and 2) gene transcriptional profiling. Importantly, this powerful new approach directly links cancer cell metabolism with clinical outcome, and new therapeutic strategies for inhibiting the TCA cycle and mitochondrial oxidative phosphorylation in cancer cells.
Introduction
Recently, we proposed a new mechanism by which the “Warburg effect” contributes to tumor metabolism.Citation1 To distinguish this new paradigm from the conventional Warburg effect, we have termed this new mechanism “the reverse Warburg effect.”Citation1 In this model, tumor myo-fibroblasts [a.k.a., cancer-associated fibroblasts (CAFs)] undergo autophagy and mitophagy, resulting in aerobic glycolysis and the fibroblastic production of energy-rich metabolites, which are then transferred to epithelial cancer cells.Citation2–Citation7 In the adjacent cancer cells, these energy-rich metabolites then enter the TCA cycle as Acetyl-CoA, resulting in high ATP production via mitochondrial oxidative phosphorylation.Citation4–Citation7
To determine if the end-products of glycolysis (such as ketones and lactate) can modulate tumor growth and metastasis, we next used MDA-MB-231 triple-negative breast cancer cells as a model cell line for xenograft injections.Citation8 Interestingly, 3-hydroxy-butyrate (a ketone body) significantly increased tumor growth (∼2.5-fold), without any increases in tumor angiogenesis.Citation8 In contrast, L-lactate increased experimental lung metastasis (by ∼10-fold), but did not affect primary tumor growth.Citation8 Finally, both ketones and lactate stimulated the migration of MDA-MB-231 cells, functioning as chemo-attractants.Citation8 Taken together, these results indicate that ketones and lactate can promote tumor growth and metastasis, providing additional evidence to support the “the reverse Warburg effect.”Citation8 Via an independent informatics approach, using published human tumor transcriptional profiling data, we showed that breast cancer cells normally upregulate gene transcripts that drive oxidative mitochondrial metabolism and the TCA cycle in vivo.Citation8 Thus, it appears that breast cancer cells actually use oxygen and mitochondrial metabolism, to generate high levels of energy which, in turn, fuel anabolic tumor growth.Citation7,Citation9,Citation10 In support of this hypothesis, it has been shown that cancer cells upregulate proteins that carry oxygen, such as myoglobin, hemoglobin, neuroglobin and cytoglobin.Citation11,Citation12 However, it remains unknown how lactate and ketones affect gene expression in cancer cells.
Here, we have used MCF7 cells as a second independent breast cancer cell model, to study the effects of ketones and lactate administration on gene expression. Treatment of MCF7 cells with high-energy metabolites (such a L-Lactate) is sufficient to stimulate mitochondrial biogenesis, as reflected by a dramatic increase in overall mitochondrial mass per MCF7 cancer cell.Citation4,Citation6,Citation10 Interestingly, we show that ketones and lactate both increase the transcriptional profiles of genes that are associated with “stemness” (neural, embryonic, and hematopoietic stem cells). Thus, the metabolic use of ketones and lactate could “fuel” the cancer stem cell phenotype, which may be responsible for promoting tumor growth and metastasis. In accordance with this notion, we show that the ketone- and lactate-induced “gene signatures” (generated using the luminal A-like MCF7 cell line) predict recurrence, metastasis, and reduced overall survival in the most common form of human breast cancer [the ER(+) luminal A subtype]. Thus, this new “metabolo-genomics” approach to personalized cancer medicine links biomarker stratification with novel energy-based therapeutic strategies, such as metformin.
Results
Lactate and ketones alter gene transcription in MCF7 cells.
MCF7 cells were cultured in the presence of ketones (3-hydroxybutyrate; 10 mM) or L-Lactate (10 mM), and subjected to transcriptional profiling using an exon-array approach. For simplicity, we focused on the gene sets that were upregulated in response to these high-energy metabolites. In the case of lactate, ∼4,131 genes were upregulated (fold-change (FC) >1.15; p < 0.05); using a cut-off of 1.5 for FC, nearly 2,000 genes were upregulated. Similarly, in the case of ketones, ∼ 4,141 genes were upregulated (FC > 1.15; p < 0.05); using a cut-off of 1.5 for FC, >2,500 genes were upregulated.
For a complete list of these upregulated genes, see Supplemental Tables 1 and 2.
Lactate and ketones induce transcriptional profiles associated with “stemness.”
To identify the cellular processes specifically associated with the lactate- and ketone-induced gene profiles, we next performed gene set enrichment analysis (GSEA) ( and ). Both the lactate- and ketone-induced gene profiles were most tightly associated with (1) stemness (neural, embryonic and hematopoietic), (2) a reduction in DNA damage and (3) breast cancer (ER-negative vs. ER-positive). The transcriptional activity of several well-known stem cell associated transcription factors (SP1, MAZ, MEIS1, MLLT7, LEF1, TCF3, ELK1, SREBF1, PAX4 and ESRRA) also appeared elevated. HeatMaps for the lactate-induced and ketone-induced stem cell associated genes are shown in and .
Next, we considered the overlap between the lactate- and ketone-induced gene profiles, by intersecting these two gene sets. Using this approach, ∼1,433 overlapping genes were identified; 2,698 genes were found to be lactate-specific, while 2,708 genes were found to be ketone-specific ( and Sup. Table 3). We then performed gene set enrichment analysis (GSEA) to identify the cellular processes associated with these three sets of gene profiles. All three gene sets (common/shared, lactate-specific and ketone-specific) remained associated with stemness, although some specific differences were noted. The lactate-specific gene profile was most similar to neural stem cells, while the ketone-specific gene profile was most similar to hematopoietic stem cells. Interestingly, the genes commonly upregulated by both lactate and ketones were most similar to embryonic stem cells ( and Sup. Table 3).
Finally, the lactate-specific gene profile also showed significant overlap with two clinical gene signatures that predict poor outcome in liver cancer (HCC) and multiple myeloma (MM).Citation13 HeatMaps for these lacate-only poor prognosis associated genes are shown in .
Lactate- and ketone-induced gene signatures are associated with breast cancer and predict poor clinical outcome.
MCF7 cells are an ER(+) breast cancer cell line. In accordance with these observations, both the lactate- and ketone-induced gene signatures are significantly correlated with breast cancer (relative to normal breast tissue), and are preferentially associated with ER(+) breast cancers (as compared with ER(−) breast cancers), with p-values between 10−4 and 10−5 ( and ). Similarly, both the lactate- and ketone-induced gene signatures are associated with significant reductions in overall survival in ER(+) breast cancer patients (p ∼ 10−3) ( and ).
Lactate- and ketone-induced gene signatures predict recurrence, metastasis and decreased overall survival in luminal A breast cancers.
MCF7 cells are an ER(+)/PR(+) human breast cancer cell line, but they lack HER2 amplification, specifically classifying them as a luminal A subtype of breast cancer, because of their high ER and PR expression. Thus, we further restricted our statistical analysis to luminal A breast cancer patients.
The MCF7 lactate- and ketone-induced gene signatures had high predictive value in luminal A breast cancer patients, and were specifically associated with increased metastasis, tumor recurrence, and dramatic reductions in overall patient survival ( and ). Overall patient survival was associated with p-values between 10−4 and 10−5.
Quantitatively similar results were obtained if we restricted our analysis to the lactate- and ketone-induced gene sets that were associated with “stemness” () or with lactate and other types of cancer, such as hepatocellular carcinoma (HCC) or multiple myeloma (MM) ().
Based on this analysis, it appears that lactate and/or ketone utilization by cancer cells may be a general phenomenon and thus that can be exploited to identify aggressive predictive gene signatures for poor clinical outcome in a wide-variety of different types of human cancers.
Lactate and ketones increase histone acetylation in MCF7 cancer cells.
Both ketones and lactate are converted to Acetyl-CoA intracellularly, so they can be used for energy metabolism via the TCA cycle and mitochondrial oxidative phophosphoryation. However, Acetyl-CoA is also utilized for the acetylation of proteins such as histones, which is implicated in the epigenetic regulation of gene expression. Histone acetylation neutralizes the positive charge on histones, promoting their dissociation from DNA, potentially increasing gene transcription.
Thus, we used acetylation-specific antibody probes to assess that status of histone acetylation in MCF7 cells treated with ketones and lactate. shows, in accordance with our predictions, that both ketones and lactate increased histone acetylation. Lactate had a more positive effect than ketones, and both resulted in a “laddering” effect, which most likely represents hyper-acetylation plus other protein modifications, such as phosphorylation, which can dramatically affect the mobility of proteins in SDS-PAGE gels.
Thus, we suggest that the lactate- and ketone-induced increases in histone acetylation may contribute to the changes we have observed in genome-wide transcriptional profiling.
Lactate and ketones promote the growth of bonafide ES cells in culture.
Gene set enrichment analysis (GSEA; See Sup. Table 3 and ) revealed that the genes commonly upreguated by both lactate and ketones in MCF7 cells are similar to genes expressed by embryonic stem cells. Thus, based on these findings, we predicted that both lactate and ketones would stimulate the growth of ES cells. To test this hypothesis, we cultured murine ES cells using wild-type fibroblasts as a feeder layer, and supplemented them with energy-rich metabolites (either L-Lactate or ketones [butanediol or 3-hydroxy-butyrate)]. Murine ES cells were seeded at 50 cells/well. and B directly shows that ES cell colony size and colony number were both significantly increased by lactate or ketone supplementation. Colonly size (diameter) was increased up to 25%, while colony number was increased by ∼2–3-fold, depending on the metabolite. Thus, lactate and ketones stimulate the growth of ES cells as predicted. These observations provides functional validation for our findings that high-energy metabolites may transcriptionally increase “stemness.”
Discussion
Here, we have systematically examined if clinical outcome in breast cancer patients is affected by lactate and ketone utilization by breast cancer cells. First, we used MCF7 cells treated with lactate or ketones to generate specific gene signatures associated with the utilization of these high-energy metabolites. Then, these lactate- and ketone-induced “gene signatures” were subjected to gene set enrichment analysis (GSEA). Interestingly, our results indicate that ketones and lactate transcriptionally increase the “stemness” of cancer cells, with striking similarities to neural, embryonic and hematopoietic stem cells (with p-values as low as 10−23). Finally, we applied these lactate- and ketone-induced gene signatures to human breast cancer patients. Our data suggest that increases in oxidative mitochondrial metabolism in cancer cells is associated with tumor recurrence, metastasis, and poor clinical outcome, in the most common form of human breast cancer (the luminal A sub-type) and possibly other cancers. In accordance with these findings, MCF7 cells are ER(+)/PR(+), but lack HER2 amplification, specifically classifying them as a luminal A type breast cancer cell line.
Implications for personalized cancer medicine.
Our results potentially have broad clinical and translational applications (). Firstly, our data show that it is possible to generate a transcriptional “gene signature” that predicts poor clinical outcome simply by treating a cancer cell line with high-energy metabolites, such as lactate or ketones. This suggests that other promising prognostic gene signatures could easily be generated for several types of cancers, by simply treating a given cancer cell line with L-lactate or ketones. This approach may help drive new biomarker discovery, and the more rapid development of personalized cancer medicine. We have termed this new approach to biomarker discovery “metabolo-genomics.”
What about the implications for cancer chemo-therapy? Perhaps, most importantly, these studies suggest that targeting oxidative mitochondrial metabolism in aggressive cancer cells, with the appropriate anti-metabolites, could help eradicate the “cancer stem cell phenotype.” The “Achille's heel” of the cancer stem cell may be its ability to use lactate and/or ketones as a fuel source, for driving oxidative mitochondrial metabolism. We suggest (; also see Discussion below) that these high-risk cancer patients could be effectively treated with metformin, an anti-oxidant and weak mitochondrial “poison,” and other compounds that inhibit oxidative mitochondrial metabolism in cancer cells.
High-energy metabolites, stemness and metastasis.
Interestingly, high lactate production has long been known to be a predictor of poor clinical outcome for a variety of malignancies.Citation14–Citation17 In fact, lactic acidosis is specifically associated with breast cancer metastasis.Citation18–Citation23 Consistent with the idea that cancer cells use their mitochondria to generate energy, the metastatic phenotype is directly associated with an increase in oxidative mitochondrial metabolism in cancer cells,Citation24,Citation25 especially in cancer cells that undergo brain metastasis.Citation26
The brain is a particularly lactate-rich microenvironment, because astrocytes take up glucose, and then secrete lactate to “feed” adjacent neurons.Citation27–Citation29 This is known as “neuron-glia metabolic coupling” and is analogous to “the reverse Warburg effect” in cancer.Citation30 Thus, metastatic cancer cells may be attracted to the lactate-rich microenvironment of the brain. In this context, it is interesting to note that we observed that lactate treatment of MCF7 cells induced a transcriptional profile similar to neuronal stem cells. Importantly, it has already been shown that neural stem cells can use lactate as a carbon source, during glucose starvation.Citation31
Similarly, the liver (a major site of ketogenesis) would provide a ketone-rich microenvironment for cancer cells, that could then use ketones as a metabolic fuel for oxidative mitochondrial metabolism. This may explain why cancer cells often metastasize to the liver, and the outcome of breast cancer patients with liver metastases is so poor. Here, we showed that treatment of MCF7 cells with ketones induces a transcriptional profile similar to hematopoietic stem cells. This is of interest because the liver is the site of fetal hematopoiesis, during embryogenesis. Thus, it is possible that ketone production in the liver could functionally contribute towards hematopoiesis. As such, we should explore the possibility that different metabolic microenvironments differentially affect stem cell growth and lineage commitment, during both development and cancer progression.
Another common site of metastasis is the lung, which would provide an oxygen-rich microenvironment. Thus, this site is also consistent with the idea that metastatic cancer cells use oxidative mitochondrial metabolism.
We showed here that both ketones and lactate promote the growth of embryonic stem (ES) cells. Consistent with these findings, a recent study showed that ES cells preferentially use mitochondrial oxidative metabolism, and that their dependence on mitochondria decreases as they undergo differentiation.Citation32,Citation33 This fits well with the idea that ES cells use lactate and ketones as fuel for the TCA cycle and oxidative mitochondrial metabolism, thereby stimulating stem cell growth.
Ketones and lactate are both converted to Acetyl-CoA (). Then, Acetyl-CoA enters the TCA cycle and undergoes mitochondrial oxidative metabolism. Alternatively, Acetyl-CoA may be used for histone acetylation, which is implicated in the epigenetic control of gene expression. Histone acetylation neutralizes the positive charge on histones, promoting their dissociation from DNA, increasing gene transcription. Interestingly, histone acetylation has also been implicated in controlling the “stemness” of ES cells.Citation34 Thus, ketones and lactate may link Acetyl-CoA with mitochondrial metabolism and the epigenetic control of gene expression.
Interestingly, [11C]Acetoacetate (a ketone body related to 3-hydroxy-butyrate) is currently being developed as an imaging agent for PET-scanning for the early detection of tumors.Citation35–Citation38
Metformin kills cancer stem cells by inhibiting mitochondrial metabolism and induces the conventional Warburg effect (aerobic glycolysis) in cancer cells.
Metformin is an anti-oxidant that is routinely used to treat diabetic patients. Metformin exerts its potent anti-oxidant effect by inhibiting mitochondrial complex I,Citation39 thereby preventing mitochondrial ROS production and mitochondrial oxidative phosphorylation. Thus, metformin functions as a “weak” mitochondrial poison.
In accordance with our assertion that cancer cells use mitochondrial oxidative phosphorylation for energy production, metformin treatment prevents and/or inhibits tumor formation both in diabetic patients and in mouse animal models.Citation40,Citation41 Moreover, metformin also kills “cancer stem cells”.Citation42–Citation45 These findings are all consistent with our proposal that the treatment of cancer cells with high-energy metabolites (lactate and ketones) drives mitochondrial oxidative phosphorylation, and increases the “stemness” of cancer cells. Moreover, we see that these high-energy metabolites induce a “stem-like” transcriptional profile that is specifically associated with tumor recurrence, metastasis and poor clinical outcome.
Remarkably, it has been shown that metformin prevents cancer cells from using their mitochondria, induces aerobic glycolysis and lactate production, and shifts cancer cells towards the conventional “Warburg effect.”Citation39,Citation46 Thus, metformin may exert its therapeutic effects in preventing and inhibiting tumor formation, by inducing the conventional “Warburg effect.”
Finally, it is quite ironic that such a promising anti-cancer drug (metformin) exerts its therapeutic effects by inducing a type of metabolism (aerobic glycolysis) that has been proposed to be the “root-cause” of cancer for the last 85 years. Thus, induction of aerobic glycolysis in cancer cells may not be the “cause of cancer,” but rather it may be the “cure for cancer.”
Experimental Procedures
Culture of MCF7 cells and treatment with lactate and ketones.
The estrogen receptor positive cell line MCF7 was purchased from ATCC. Cells were maintained in DMEM, with 10% Fetal Bovine Serum (FBS) and Penicillin 100 units/mL-Streptomycin 100 µg/mL (Invitrogen) [DMEM 10% FBS-Pen-Strep]. Cells were cultured in a humidified atmosphere containing 5% CO2 at 37°C. Briefly, 1.4 × 106 MCF7 cells were plated in 10 cm plastic dishes (Corning) in DMEM 10% FBS-Pen-Strep. After 24 hours, the media of these cells was changed to DMEM with 10% NuSerum (a low protein alternative to FBS; BD Biosciences) and Pen-Strep with or without 10 mM L-lactate (sodium salt; Sigma cat. #71718) or 10 mM R-3-hydroxy-butyrate (sodium salt; Sigma cat. #298360). Cells were maintained in this media for 48 hours before gene expression profiling was performed. Acetylation-specific antibodies directed against histone proteins were obtained from Cell Signaling Technology, Inc.
Genome-wide transcriptional profiling (exon-array).
Total RNA was extracted using the RNeasy protocol from Qiagen (Valencia, CA). RNA was prepared from three control MCF7 samples, and three MCF7 samples treated with lactate, and three MCF7 samples treated with ketones. RNA quality was evaluated using the Agilent 2100 Bioanalyzer (Agilent, Palo Alto, CA). RNA amplification and labeling was performed from 50 ng total RNA by the WT-Ovation Pico RNA amplification system (NuGen Technologies, Inc.). Amplified cDNA was purified with QIAGEN QIAquick PCR purification Kit. Sense transcript cDNA (ST-cDNA) was generated from 3 µg amplified cDNA using WT-Ovation Exon module (NuGen Technologies, Inc.). Purified ST-cDNA was assed for yield using the Nanodrop Spectrophotometer (NanoDrop Technologies, Inc.). 5 µg ST-cDNAs were fragmented and chemically labeled with biotin to generate biotinylated ST-cDNA using FL-Ovation cDNA biotin module V2 (NuGen Technologies, Inc.). Each Affymetrix Human Exon (HuEx-1.0-st-v2) array (Affymetrix, Santa Clara, CA) were hybridized with fragmented and biotin-labeled target (4.5 µg) in 200 µl of hybridization cocktail. Target denaturation was performed at 99°C for 2 min. and then 45°C for 5 min., followed by hybridization for 18 hrs. Arrays then were washed and stained using Genechip Fluidic Station 450, and hybridization signals were amplified using antibody amplification with goat IgG (Sigma-Aldrich) and anti-streptavidin biotinylated antibody (Vector Laboratories, Burlingame, CA). Chips were scanned on an Affymetrix Gene Chip Scanner 3000, using Command Console Software. Background correction and normalization were done using Robust Multichip Average (RMA) with Genespring V 11.5 software (Agilent, Palo Alto, CA). Results from one exon for each gene analyzed were selected for inclusion in Supplemental Tables 1 and 2. A p-value of <0.05 was considered statistically significant.
Gene set enrichment analysis (GSEA).
For the following lists of genes:
genes upregulated in the Ketones dataset (fold change ≥1.15 and p-value ≤ 0.05),
genes upregulated in the Lactate dataset (fold change ≥1.15 and p-value ≤ 0.05),
genes upregulated in the Ketones dataset but not in the Lactate dataset,
genes upregulated in the Lactate dataset but not in the Ketones dataset and
genes upregulated in both datasets,
we computed enrichments in gene sets contained in the latest release of Molecular Signatures Database (MSigDB v2.5, April 2008, reviewed in ref. Citation47). MSigDB is a database of gene sets:
collected from various sources, such as online pathway databases, publications and knowledge of domain experts,
comprising genes that share a conserved cis-regulatory motif across the human, mouse, rat and dog genomes,
identified as co-regulated gene clusters by mining large collections of cancer-oriented microarray data and
annotated by a common Gene Ontology (GO) term.
The enrichment analysis consisted of computing p-values for the intersections between a gene list of interest X and each gene set Y in MSigDB. First, we computed the overlap between X and Y. Then, we computed the probability (p-value) that the observed overlap between sets X and Y is less than or equal to the overlap between set X and a randomly-chosen set of size equal to the size of set Y. This probability was calculated by applying the cumulative density function of the t distribution on the observed overlap against the distribution of randomly observed overlaps obtained from 1,000,000 permutations.
In order to account for multiple hypothesis testing, we used randomly selected gene lists of the same size as the list under consideration to estimate the false discovery rate at a given p-value threshold. The final list of significant MSigDB gene sets was determined by setting a p-value threshold which ensures that the false discovery rate does not exceed 5%.
Analysis of clinical outcome in human breast cancer patients.
A microarray dataset that was previously compiled from the public repositories Gene Expression Omnibus (www.ncbi.nlm.nih.gov/geo/),Citation48 and ArrayExpress (www.ebi.ac.uk/arrayexpress/),Citation49 was used to evaluate the Ketone and Lactate expression signatures in the context of clinical samples.Citation50,Citation51 Only samples with ER IHC data were selected for analysis, including 959 ER-positive and 323 ER-negative, for a total of 1,282 samples. In addition, 102 normal, healthy breast tissue controls were used for comparison. Samples were first analyzed in subsets based on their ER IHC status. Additional subsets were defined by classifying samples among five canonical breast cancer subtypes, including luminal A, luminal B, normal-like, basal and Her-2-overexpressing disease. Samples were classified by computing their correlation against five expression profile centroids representing these breast cancer subtypes and assigning and assigning them to the subtype with the highest corresponding correlation coefficient.Citation52 Samples with a maximum correlation coefficient below 0.3 were considered unclassified. Differential expression of the averaged gene signature magnitude among these sample subsets was evaluated using two-tailed t-test. Kaplan-meier analysis was used to evaluate survival trends within sample subsets, including 627 samples with metastasis-free survival time (507 ER-pos., 120 ER-neg.), 637 with relapse free survival time (517 ER-pos., 120 ER-neg.) and 329 with overall survival time (219 ER-pos., 110 ER-neg.). The Log-rank test was used to evaluate differences in survival curves for high vs. low signature-expressing populations.
Murine embryonic stem (ES) cell culture.
R1 cells (a kind gift of Dr. S. McMahon, Thomas Jefferson University, Philadelphia, PA), are mouse embryonic stem cells and were cultured on tissue culture plates (BD Falcon, Franklin Lakes, NJ) coated with 0.1% (v/v) porcine gelatin (Sigma-Aldrich Corp., St. Louis, MO) in Dulbecco's modified Eagle's medium (DMEM; Invitrogen, Grand Island NY) in the presence of 15% fetal bovine serum (ES-tested Hyclone, Perbio, Logan, UT) 0.1 mM 2-mercaptoethanol, 0.1 mM non-essential aminoacids, 2 mM Glutamine, 0.1 mM sodium pyruvate, 10 mM HEPES and 1,000 unit/ml murine LIF (Chemicon International Inc., Tamecula, CA) 100 units/ml penicillin and 100 µ/ml streptomycin. Cells were trypsinized and re-plated every 2nd day and re-fed daily. In some experiments 5 mM or 10 mM of (R)-(-)-1, 3-Butanediol; (R)-(-)-3-Hydroxybutyric acid; Sodium L-lactate (Sigma-Aldrich Inc., St. Louis, MO) was added to the medium. In all the experiments, medium was replaced daily. Alkaline phosphatase (AP) staining was performed using Fast Red TR salt™ (Sigma) reagent, according to the manufacturer's protocol. Computer-assisted image analysis of alkaline phosphatase positive colonies was performed using an Olympus BX51 System Microscope (Olympus Corp., Miami, FL, USA) equipped with a Micropublisher 5.0 cooled CCD camera (QImaging Corp., BC). ImageJ software was used to analyze colonies size. MEFs were inactivated to be used as a feeder layer for mouse ES cells. Mitotic inactivation was performed by mitomycin C treatment. Briefly, fibroblasts were grown to 90% confluence and mitomycin was added to the growth medium at final concentration of 10 µg/ml. Cells were treated for 3 h. MEFs were trypsinized, counted, and frozen at the concentration of 3 × 106/ml. 1 ml of frozen feeders was used to coat a 24 well plate. All the ES cell experiments were performed at least 3 times in duplicate. Results were analyzed for statistical significance by the two tail Student's t-test.
Figures and Tables
Figure 1 HeatMaps for the intersection between MCF7 lactate-induced genes and transcripts that are highly expressed in neural stem cells. Note that these two gene sets have 461 intersecting genes in common. See Supplemental Table 4 for a detailed list. Overlapping genes are shown in (A and B).
Figure 2 HeatMaps for the intersection between MCF7 ketone-induced genes and transcripts that are highly expressed in hematopoietic stem cells. Note that these two gene sets have 311 intersecting genes in common. See Supplemental Table 5 for a detailed list.
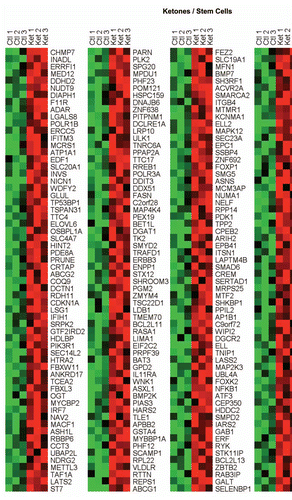
Figure 3 Venn diagrams for the transcriptional overlap between the lactate-induced and ketone-induced transcriptional profiles in MCF7 cells. Transcriptional overlap between the lactate- and ketone-induced gene profiles is shown. Using this approach, ∼1,433 overlapping genes were identified; 2,698 genes were found to be lactate-specific, while 2,708 genes were found to be ketone-specific. Gene set enrichment analysis (GSEA) revealed that all three gene sets (common, lactate-specific and ketone-specific) remained associated with stemness, although differences were noted. The lactate-specific gene profile was most similar to neural stem cells, while the ketone-specific gene profile was most similar to hematopoietic stem cells. The genes commonly upregulated by both lactate and ketones were most similar to embryonic stem cells. Finally, the lactate-specific gene profile also showed significant overlap with two clinical gene signatures that predict poor outcome in liver cancer and multiple myeloma. Associations with ER(+) and ER(−) breast cancers were also noted. See Supplemental Table 3.
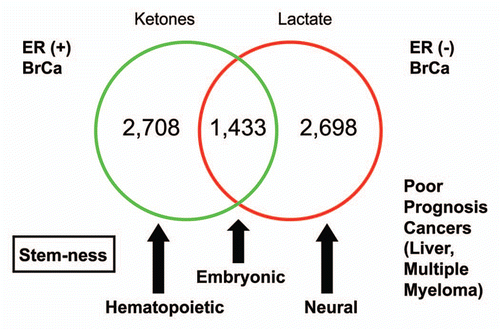
Figure 4 HeatMaps for the intersection between MCF7 lactate-specific genes and transcripts that are highly expressed in poor prognosis cancers. The lactate-specific gene profile showed significant overlap with two clinical gene signatures that predict poor outcome in and multiple myeloma (MM, 37 genes overlap) and liver cancer (HCC, 31 genes overlap). See Supplemental Tables 6 and 7 for the detailed lists.
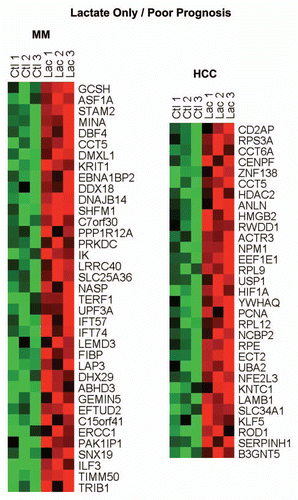
Figure 5 The lactate-induced gene signature is associated with ER(+) breast cancer and predicts poor clinical outcome. (A) Boxplots illustrate differential regulation of the lactate signature expression in breast cancer versus healthy breast tissue. Arrows indicate the directionality of differential regulation within each population. (B) Survival curves within low and high lactate signature-expressing populations are shown for overall survival in ER-positive breast cancer. This signature contains ∼4,131 genes (See Sup. Table 1).
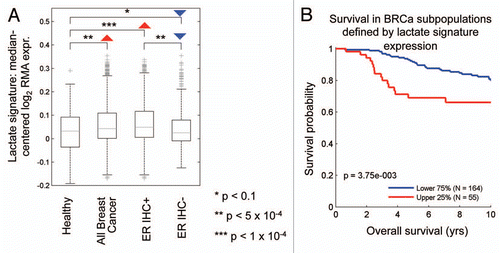
Figure 6 The ketone-induced gene signature is associated with ER(+) breast cancer and predicts poor clinical outcome. (A) Boxplots illustrate differential regulation of the ketone signature expression in breast cancer versus healthy breast tissue. Arrows indicate the directionality of differential regulation within each population. (B) Survival curves within low and high ketone signature-expressing populations are shown for overall survival in ER-positive breast cancer. This signature contains ∼4,141 genes (See Sup. Table 2).
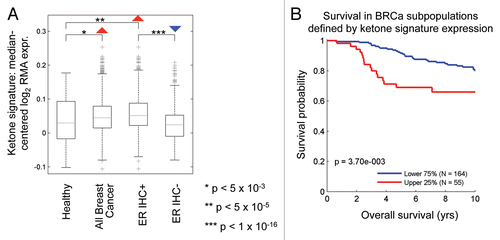
Figure 7 The lactate-induced gene signature predicts recurrence, metastasis and decreased overall survival in luminal A breast cancers. Survival curves within low and high lactate signature-expressing populations are shown within the luminal A subtype for (A) metastasis-free survival, (B) relapse-free survival and (C) overall survival. This signature contains ∼4,131 genes (See Sup. Table 1). In (C), it is important to note that overall survival data were available for a smaller number of luminal A breast cancer cases.
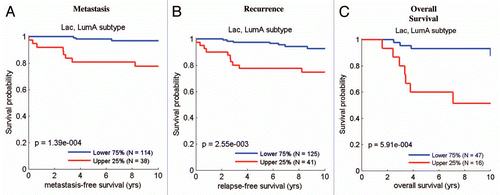
Figure 8 The ketone-induced gene signature predicts recurrence, metastasis and decreased overall survival in luminal A breast cancers. Survival curves within low and high ketone signature-expressing populations are shown within the luminal A subtype for (A) metastasis-free survival, (B) relapse-free survival and (C) overall survival. This signature contains ∼4,141 genes (See Sup. Table 2). In (C), it is important to note that overall survival data were available for a smaller number of luminal A breast cancer cases.
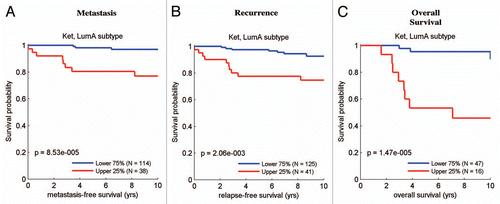
Figure 9 The ketone- and lactate-induced “stem cell” signatures predict decreased overall survival in luminal A breast cancers. Survival curves within low and high stem-cell specific ketone signature-expressing populations are shown for overall survival within (A) ER-positive disease and (B) the luminal A subtype. Similar results are shown for the stem-cell specific lactate signature in (C), with reductions in overall survival. These two signatures each contain ∼300–400 gene transcripts, see Supplemental Tables 4 and 5. In (C), it is important to note that overall survival data were available for a smaller number of luminal A breast cancer cases.
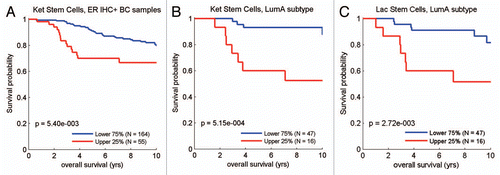
Figure 10 Survival analysis for the intersection between MCF7 lactate-specific genes and transcripts that are highly expressed in poor prognosis cancers. (A) Survival curves based on the intersection of the lactate and the HCC-derived signatures are shown within the luminal A subtype for overall survival. (B) Survival curves based on the intersection of the lactate and the MM-derived signatures are shown for overall survival. These two signatures each contain ∼30–40 gene transcripts, see Supplemental Tables 4 and 5. HCC, hepatocellular carcinoma; MM, multiple myeloma.
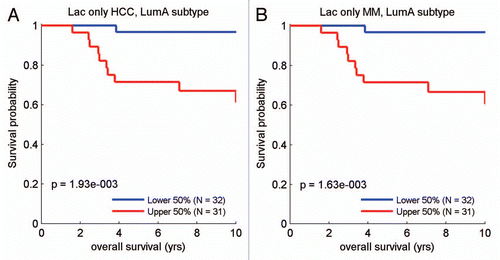
Figure 11 Lactate and ketones increase histone acetylation in MCF7 cancer cells. MCF7 cells were treated with ketones and lactate. Then, we used acetylation-specific antibody probes to assess that status of histone acetylation. Note that both ketones and lactate increased histone acetylation. However, lactate had a more positive effect than ketones, and both resulted in a “laddering” effect, which most likely represents hyper-acetylation plus other protein modifications, such as phosphorylation, which affects the mobility of proteins in SDS-PAGE gels. As such, the lactate- and ketone-induced increases in histone acetylation may contribute to changes in genome-wide transcriptional profiling. For histone H4, two parts are shown. One part shows the higher molecular weight “laddering” species; the other part shows a single band, which most likely represents the mono-acetylated species of histone H4. 3HB, 3-hydroxy-butryate; L-Lac, L-Lactate.
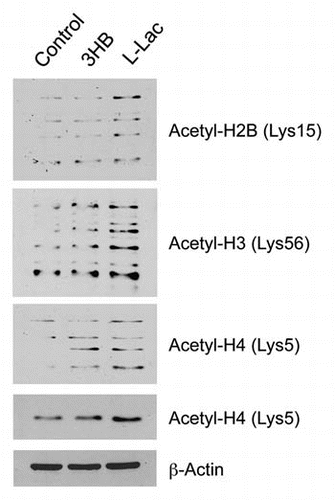
Figure 12 Ketones and lactate promote the growth of mouse embryonic stem (ES) cells in culture. (A) R1 murine ES cells were grown in the absence or presence of various metabolites, then colony size and number were quantitated. (B) Representative images of ES cell colonies are shown. Note that both colony size and number were significantly increased (p < 0.01). Importantly, colony number increased up to 3-fold. BD, butanediol; 3HB, 3-hydroxy-butryate; L-Lac, L-Lactate.
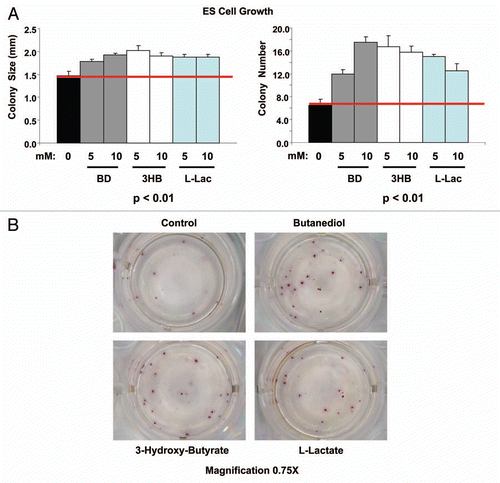
Figure 13 A new metabolic strategy for personalized cancer diagnosis and therapy. Here, we outline a general experimental strategy for personalized cancer medicine. Briefly, cancer cells of a particular tumor type are selected for study. Then, these cancer cells are cultured in the absence and presence of high-energy metabolites, such as ketones and/or lactate. This should allow the investigator to generate a new gene signature based on transcriptional profiling (exon-array). This signature could then be used to select the patients that are undergoing this type of tumor metabolism, and correlate tumor metabolism with clinical outcome, such as recurrence and metastasis. High-risk patients could then be identified at diagnosis and treated with new therapies that are designed to target oxidative mitochondrial metabolism, such as metformin which is a specific inhibitor of mitochondrial complex I.
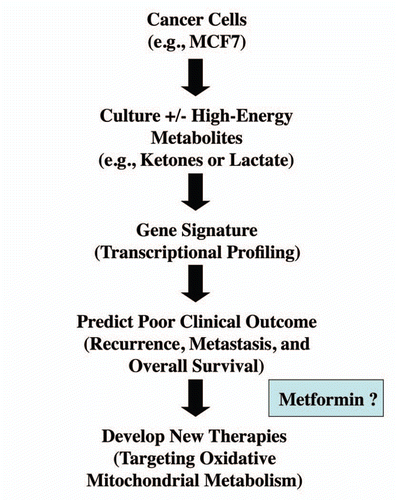
Figure 14 Possible mechanism(s) of action of ketones and lactate: Converging on Acetyl-CoA. Lactate and Ketones are converted to Acetyl-CoA, which can then be used via oxidative mitochondrial metabolism to generate high amounts of ATP. Alternatively, Acetyl-CoA could also be utilized for the acetylation of proteins, such as histones. Histone acetylation has been associated with increases in gene expression.
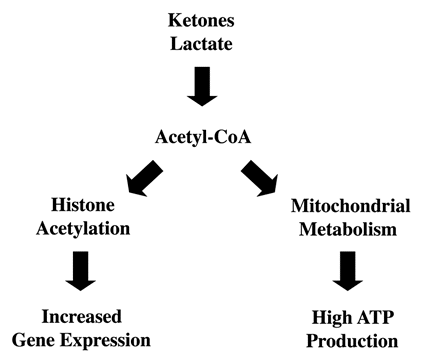
Table 1 Cellular processes increased in MCF7 cells treated with lactate
Table 2 Cellular processes increased in MCF7 cells treated with ketones
Additional material
Download Zip (2.9 MB)Acknowledgements
M.P.L. and his laboratory were supported by grants from the NIH/NCI (R01-CA-080250; R01-CA-098779; R01-CA-120876; R01-AR-055660), and the Susan G. Komen Breast Cancer Foundation. F.S. was supported by grants from the Breast Cancer Alliance (BCA), and a Research Scholar Grant from the American Cancer Society (ACS). R.G.P. was supported by grants from the NIH/NCI (R01-CA-70896, R01-CA-75503, R01-CA-86072 and R01-CA-107382) and the Dr. Ralph and Marian C. Falk Medical Research Trust. The Kimmel Cancer Center was supported by the NIH/NCI Cancer Center Core grant P30-CA-56036 (to R.G.P.). Funds were also contributed by the Margaret Q. Landenberger Research Foundation (to M.P.L.). This project is funded, in part, under a grant with the Pennsylvania Department of Health (to M.P.L.). The Department specifically disclaims responsibility for any analyses, interpretations or conclusions. This work was also supported, in part, by a Centre grant in Manchester from Breakthrough Breast Cancer in the UK (to A.H.) and an Advanced ERC Grant from the European Research Council.
References
- Pavlides S, Whitaker-Menezes D, Castello-Cros R, Flomenberg N, Witkiewicz AK, Frank PG, et al. The reverse Warburg effect: Aerobic glycolysis in cancer associated fibroblasts and the tumor stroma. Cell Cycle 2009; 8:3984 - 4001
- Pavlides S, Tsirigos A, Migneco G, Whitaker-Menezes D, Chiavarina B, Flomenberg N, et al. The Autophagic Tumor Stroma Model of Cancer: Role of Oxidative Stress and Ketone Production in Fueling Tumor Cell Metabolism. Cell Cycle 2010; 9:3485 - 3505
- Pavlides S, Tsirigos A, Vera I, Flomenberg N, Frank PG, Casimiro MC, et al. Loss of Stromal Caveolin-1 Leads to Oxidative Stress, Mimics Hypoxia and Drives Inflammation in the Tumor Microenvironment, Conferring the “Reverse Warburg effect”: A Transcriptional Informatics Analysis with Validation. Cell Cycle 2010; 9:2201 - 2219
- Martinez-Outschoorn UE, Balliet RM, Rivadeneira DB, Chiavarina B, Pavlides S, et al. Oxidative Stress in Cancer Associated Fibroblasts Drives Tumor-Stroma Co-Evolution: A New Paradigm for Understanding Tumor Metabolism, the Field Effect and Genomic Instability in Cancer Cells. Cell Cycle 2010; 9:3256 - 3276
- Martinez-Outschoorn UE, Pavlides S, Whitaker-Menezes D, Daumer KM, Milliman JN, et al. Tumor Cells Induce the Cancer Associated Fibroblast Phenotype Via Caveolin-1 Degradation: Implications for Breast Cancer and DCIS Therapy with Autophagy Inhibitors. Cell Cycle 2010; 9:2423 - 2433
- Martinez-Outschoorn UE, Trimmer C, Lin Z, Whitaker-Menezes D, Chiavarina B, Zhou J, et al. Autophagy in Cancer Associated Fibroblasts Promotes Tumor Cell Survival: Role of Hypoxia, HIF1 Induction and NFκB Activation in the Tumor Stromal Microenvironment. Cell Cycle 2010; 9:3515 - 3533
- Martinez-Outschoorn UE, Whitaker-Menezes D, Pavlides S, Chiavarina B, Bonuccelli G, Casey T, et al. The Autophagic Tumor Stroma Model of Cancer or “Battery-Operated Tumor Growth”: A Simple Solution to the Autophagy Paradox. Cell Cycle 2010; 9:4297 - 4306
- Bonuccelli G, Tsirigos A, Whitaker-Menezes D, Pavlides S, Pestell RG, Chiavarina B, et al. Ketones and Lactate “Fuel” Tumor Growth and Metastasis: Evidence that Epithelial Cancer Cells Use Oxidative Mitochondrial Metabolism. Cell Cycle 2010; 9:3506 - 3514
- Lisanti MP, Martinez-Outschoorn UE, Chiavarina B, Pavlides S, Whitaker-Menezes D, Tsirigos A, et al. Understanding the “lethal” drivers of tumor-stroma co-evolution: Emerging role(s) for hypoxia, oxidative stress and autophagy/mitophagy in the tumor microenvironment. Cancer Biol Ther 2010; 10:537 - 542
- Martinez-Outschoorn UE, Pavlides S, Howell A, Pestell RG, Tanowitz HB, Sotgia F, Lisanti MP. Stromalepithelial metabolic coupling in cancer: Integrating autophagy and metabolism in the tumor microenvironment. Int J Biochem Cell Biol 2011; In press
- Gorr TA, Wichmann D, Pilarsky C, Theurillat JP, Fabrizius A, Laufs T, et al. Old proteins—new locations: myoglobin, haemoglobin, neuroglobin and cytoglobin in solid tumours and cancer cells. Acta Physiol (Oxf) 2011; In press
- Kristiansen G, Rose M, Geisler C, Fritzsche FR, Gerhardt J, Luke C, et al. Endogenous myoglobin in human breast cancer is a hallmark of luminal cancer phenotype. Br J Cancer 2010; 102:1736 - 1745
- Moreaux J, Cremer FW, Reme T, Raab M, Mahtouk K, Kaukel P, et al. The level of TACI gene expression in myeloma cells is associated with a signature of microenvironment dependence versus a plasmablastic signature. Blood 2005; 106:1021 - 1030
- Brizel DM, Schroeder T, Scher RL, Walenta S, Clough RW, Dewhirst MW, Mueller-Klieser W. Elevated tumor lactate concentrations predict for an increased risk of metastases in head-and-neck cancer. Int J Radiat Oncol Biol Phys 2001; 51:349 - 353
- Walenta S, Wetterling M, Lehrke M, Schwickert G, Sundfor K, Rofstad EK, Mueller-Klieser W. High lactate levels predict likelihood of metastases, tumor recurrence and restricted patient survival in human cervical cancers. Cancer Res 2000; 60:916 - 921
- Walenta S, Mueller-Klieser WF. Lactate: mirror and motor of tumor malignancy. Semin Radiat Oncol 2004; 14:267 - 274
- Walenta S, Salameh A, Lyng H, Evensen JF, Mitze M, Rofstad EK, Mueller-Klieser W. Correlation of high lactate levels in head and neck tumors with incidence of metastasis. Am J Pathol 1997; 150:409 - 415
- Sculier JP, Nicaise C, Klastersky J. Lactic acidosis: a metabolic complication of extensive metastatic cancer. Eur J Cancer Clin Oncol 1983; 19:597 - 601
- Varanasi UR, Carr B, Simpson DP. Lactic acidosis associated with metastatic breast carcinoma. Cancer Treat Rep 1980; 64:1283 - 1285
- McConnell AA, Parfitt VL, Walker PR. An unusual case of shock in a young woman. Postgrad Med J 1989; 65:120
- Warner E. Type B lactic acidosis and metastatic breast cancer. Breast Cancer Res Treat 1992; 24:75 - 79
- Evans TR, Stein RC, Ford HT, Gazet JC, Chamberlain GV, Coombes RC. Lactic acidosis. A presentation of metastatic breast cancer arising in pregnancy. Cancer 1992; 69:453 - 456
- Cheng JC, Esparza SD, Knez VM, Sakamoto KM, Moore TB. Severe lactic acidosis in a 14-year-old female with metastatic undifferentiated carcinoma of unknown primary. J Pediatr Hematol Oncol 2004; 26:780 - 782
- Xu HN, Nioka S, Glickson JD, Chance B, Li LZ. Quantitative mitochondrial redox imaging of breast cancer metastatic potential. J Biomed Opt 2010; 15:36010
- Chen V, Shtivelman E. CC3/TIP30 regulates metabolic adaptation of tumor cells to glucose limitation. Cell Cycle 2010; 9:4941 - 4953
- Chen EI, Hewel J, Krueger JS, Tiraby C, Weber MR, Kralli A, et al. Adaptation of energy metabolism in breast cancer brain metastases. Cancer Res 2007; 67:1472 - 1486
- Magistretti PJ. Neuron-glia metabolic coupling and plasticity. J Exp Biol 2006; 209:2304 - 2311
- Magistretti PJ. Role of glutamate in neuron-glia metabolic coupling. Am J Clin Nutr 2009; 90:875 - 880
- Magistretti PJ, Pellerin L. The contribution of astrocytes to the 18F-2-deoxyglucose signal in PET activation studies. Mol Psychiatry 1996; 1:445 - 452
- Pavlides S, Tsirigos A, Vera I, Flomenberg N, Frank PG, Casimiro MC, et al. Transcriptional evidence for the “Reverse Warburg effect” in human breast cancer tumor stroma and metastasis: similarities with oxidative stress, inflammation, Alzheimer's disease, and “Neuron-Glia Metabolic Coupling”. Aging (Albany NY) 2010; 2:185 - 199
- Wohnsland S, Burgers HF, Kuschinsky W, Maurer MH. Neurons and neuronal stem cells survive in glucose-free lactate and in high glucose cell culture medium during normoxia and anoxia. Neurochem Res 2010; 35:1635 - 1642
- Birket MJ, Orr AL, Gerencser AA, Madden DT, Vitelli C, Swistowski A, et al. A reduction in ATP demand and mitochondrial activity with neural differentiation of human embryonic stem cells. J Cell Sci 2010; 124:348 - 358
- Harvey A, Gibson T, Lonergan T, Brenner C. Dynamic regulation of mitochondrial function in preimplantation embryos and embryonic stem cells. Mitochondrion 2011; In press
- Markowetz F, Mulder KW, Airoldi EM, Lemischka IR, Troyanskaya OG. Mapping dynamic histone acetylation patterns to gene expression in nanog-depleted murine embryonic stem cells. PLoS Comput Biol 2010; 6:1001034
- Fenselau A, Wallis K, Morris HP. Subcellular localization of acetoacetate coenzyme A transferase in rat hepatomas. Cancer Res 1976; 36:4429 - 4433
- Kallinowskil F, Davel S, Vaupell P, Baessler KH, Wagner K. Glucose, lactate and ketone body utilization by human mammary carcinomas in vivo. Adv Exp Med Biol 1985; 191:763 - 773
- Authier S, Tremblay S, Dumulon V, Dubuc C, Ouellet R, Lecomte R, et al. [11C] acetoacetate utilization by breast and prostate tumors: a PET and biodistribution study in mice. Mol Imaging Biol 2008; 10:217 - 223
- Prenen GH, Go KG, Zuiderveen F, Paans AM, Vaalburg W. An improved synthesis of carbon-11 labeled acetoacetic acid and an evaluation of its potential for the investigation of cerebral pathology by positron emission tomography. Int J Rad Appl Instrum A 1990; 41:1209 - 1216
- Dykens JA, Jamieson J, Marroquin L, Nadanaciva S, Billis PA, Will Y. Biguanide-induced mitochondrial dysfunction yields increased lactate production and cytotoxicity of aerobically-poised HepG2 cells and human hepatocytes in vitro. Toxicol Appl Pharmacol 2008; 233:203 - 210
- Pollak M. Metformin and other biguanides in oncology: advancing the research agenda. Cancer Prev Res (Phila) 2010; 3:1060 - 1065
- Memmott RM, Mercado JR, Maier CR, Kawabata S, Fox SD, Dennis PA. Metformin prevents tobacco carcinogen-induced lung tumorigenesis. Cancer Prev Res (Phila) 2010; 3:1066 - 1076
- Hirsch HA, Iliopoulos D, Tsichlis PN, Struhl K. Metformin selectively targets cancer stem cells, and acts together with chemotherapy to block tumor growth and prolong remission. Cancer Res 2009; 69:7507 - 7511
- Vazquez-Martin A, Oliveras-Ferraros C, Barco SD, Martin-Castillo B, Menendez JA. The anti-diabetic drug metformin suppresses self-renewal and proliferation of trastuzumab-resistant tumor-initiating breast cancer stem cells. Breast Cancer Res Treat 2011; 126:355 - 364
- Vazquez-Martin A, Oliveras-Ferraros C, Cufi S, Martin-Castillo B, Menendez JA. Metformin and energy metabolism in breast cancer: from insulin physiology to tumour-initiating stem cells. Curr Mol Med 2010; 10:674 - 691
- Vazquez-Martin A, Oliveras-Ferraros C, Cufi S, Del Barco S, Martin-Castillo B, Menendez JA. Metformin regulates breast cancer stem cell ontogeny by transcriptional regulation of the epithelial-mesenchymal transition (EMT) status. Cell Cycle 2010; 9:3807 - 3814
- Biradar V, Moran JL, Peake SL, Peter JV. Metformin-associated lactic acidosis (MALA): clinical profile and outcomes in patients admitted to the intensive care unit. Crit Care Resusc 2010; 12:191 - 195
- Subramanian A, Tamayo P, Mootha VK, Mukherjee S, Ebert BL, Gillette MA, et al. Gene set enrichment analysis: a knowledge-based approach for interpreting genome-wide expression profiles. Proc Natl Acad Sci USA 2005; 102:15545 - 15550
- Barrett T, Troup DB, Wilhite SE, Ledoux P, Rudnev D, Evangelista C, et al. NCBI GEO: mining tens of millions of expression profiles—database and tools update. Nucl Acids Res 2007; 35:760 - 765
- Brazma A, Parkinson H, Sarkans U, Shojatalab M, Vilo J, Abeygunawardena N, et al. ArrayExpress—a public repository for microarray gene expression data at the EBI. Nucleic Acids Res 2003; 31:68 - 71
- Ertel A. Bimodal gene expression and biomarker discovery. Cancer Inform 2010; 9:11 - 14
- Ertel A, Dean JL, Rui H, Liu C, Witkiewicz AK, Knudsen KE, Knudsen ES. RB-pathway disruption in breast cancer: differential association with disease subtypes, disease-specific prognosis and therapeutic response. Cell Cycle 2010; 9:4153 - 4163
- Hu Z, Fan C, Oh DS, Marron JS, He X, Qaqish BF, et al. The molecular portraits of breast tumors are conserved across microarray platforms. BMC Genomics 2006; 7:96