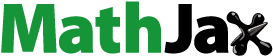
Abstract
High-grade serous ovarian cancer (HG-SOC), a major histologic type of epithelial ovarian cancer (EOC), is a poorly-characterized, heterogeneous and lethal disease where somatic mutations of TP53 are common and inherited loss-of-function mutations in BRCA1/2 predispose to cancer in 9.5–13% of EOC patients. However, the overall burden of disease due to either inherited or sporadic mutations is not known.
We performed bioinformatics analyses of mutational and clinical data of 334 HG-SOC tumor samples from The Cancer Genome Atlas to identify novel tumor-driving mutations, survival-significant patient subgroups and tumor subtypes potentially driven by either hereditary or sporadic factors.
We identified a sub-cluster of high-frequency mutations in 22 patients and 58 genes associated with DNA damage repair, apoptosis and cell cycle. Mutations of CHEK2, observed with the highest intensity, were associated with poor therapy response and overall survival (OS) of these patients (P = 8.00e-05), possibly due to detrimental effect of mutations at the nuclear localization signal. A 21-gene mutational prognostic signature significantly stratifies patients into relatively low or high-risk subgroups with 5-y OS of 37% or 6%, respectively (P = 7.31e-08). Further analysis of these genes and high-risk subgroup revealed 2 distinct classes of tumors characterized by either germline mutations of genes such as CHEK2, RPS6KA2 and MLL4, or somatic mutations of other genes in the signature.
Our results could provide improvement in prediction and clinical management of HG-SOC, facilitate our understanding of this complex disease, guide the design of targeted therapeutics and improve screening efforts to identify women at high-risk of hereditary ovarian cancers distinct from those associated with BRCA1/2 mutations.
Introduction
Epithelial ovarian cancer (EOC), of which high-grade serous ovarian carcinoma (HG-SOC) is the most prevalent, is one of the most lethal gynecological diseases in the world today. Despite dramatic progress in high-throughput biotechnology and oncogenomic studies, the genetic background of this complex disease is poorly understood, and the biomarkers for early detection, differential diagnostics, prognostic, and disease prediction have not been implemented in clinical practices.
Today, patients diagnosed with HG-SOC are confronted with a grim statistic that only 30% of them would survive beyond 5 years after initial diagnosis, even with standard chemotherapy and radiotherapy treatments.Citation1 The reasons are likely due to high tumor heterogeneity,Citation2 unknown tissue source site,Citation3 asymptomatic tumor growth, late clinical detection and diagnosis, as well as high susceptibility to recurrence after primary chemotherapy.Citation4
In fact, the heterogeneity of HG-SOC tumors and the absence of reliable early detection, prognosis and predictive biomarkers means that clinical status of the patients is varied, and the tumors often respond poorly to standard therapy. Therefore, identification of high-confidence molecular markers for risk assessment and risk of disease development/recurrence becomes important in various areas ranging from prophylactic to patient clinical management. Many published studies have investigated the tumor heterogeneity and identified biologically meaningful tumor subgroups.Citation5,Citation6 Recent technological advances have facilitated the study of this complex disease, and HG-SOC is one of the cancer diseases that have been comprehensively investigated by The Cancer Genome Atlas (TCGA) Research Network.Citation5 Their results showed that via expression profiling of mRNA data, patients can be classified into 4 biologically meaningful and distinct tumor/gene subgroups: differentiated, immunoreactive, mesenchymal, or proliferative. However, survival analysis did not show significant differences between these transcriptional sub-types in TCGA data set.Citation6 Based on meta-analysis of miRNA and mRNA expression profiles of TCGA and several other cohorts, HG-SOC patients have been reliably categorized into 3 prognostic subgroups in which patient’s overall survival correlates with specific pathways and treatment outcome.Citation6
Mutations are the most obvious risk factors of cancer and, consequently, become important candidate biomarkers. HG-SOC is characterized mostly by TP53 somatic mutations and high levels of genome instability.Citation7 At such pathobiological background, inherited loss-of-function mutations in BRCA1 and/or BRCA2 predispose to cancer in 9 to 13% of EOC patients. However, the overall burden of the disease due to either inherited mutations and/or somatic mutations is not known.
Recent mutational studies of TCGA’s HG-SOC patient cohort revealed mutated genes such as TP53, NF1, RB1, FAT3, CSMD3, GABRA6, CDK12, BRCA1, BRCA2, SMARCB1, KRAS, NRAS, CREBBP, and ERBB2.Citation5,Citation8 Other mutations of tumor suppressor genes such as BRIP, CHEK2, MRE11A, MSH6, NBN, PALB2, RAD50, and RAD51C were also identified via massive parallel sequencing in another study.Citation9 However, these and other mutations have not been systematically studied in context of their ability to provide prognosis of HG-SOC clinical outcomes. Studies have shown that in HG-SOC, TP53 somatic mutations were present in almost all HG-SOC patients, and while it would be useful in areas such as early diagnosis or risk prediction of developing the disease, their applications in patient survival prediction is restricted. Moreover, conventionally “driver” mutations of BRCA1 or BRCA2 were recently reported to be paradoxically associated with better patient survival relative to the wild-type variant.Citation5
It was reviewed by Hanahan and Weinberg that the 6 hallmarks of cancer include the enabling of replicative immortality, sustained proliferation signaling, cell death resistance, and evasion of growth suppressors, induction of angiogenesis, as well as activation of invasion and metastasis.Citation10 Interestingly, the first 4 hallmarks are associated with cell cycle regulation, which encompasses a myriad of cellular processes such as cell cycle arrest, cell cycle checkpoint, DNA integrity, and damage checkpoint control. In this work, we investigated the mutational aspect of genes involved in HG-SOC, and studied the impact of cell cycle-related genes in patient prognosis and subgroup identification. One of the most important genes involved in cell cycle checkpoint control, DNA damage response signaling, and apoptosis regulation is checkpoint kinase 2 (CHEK2), which is a nuclear serine/threonine-protein kinase. In the presence of DNA damage, CHEK2 phosphorylates downstream cell cycle regulators such as p53, Cdc25, and BRCA1 to activate checkpoint repair or recovery responses, as well as concurrently delay entry into mitosis.Citation11 Deviation from its normal physiological function is likely to contribute to disease pathogenesis.
The effects of CHEK2 mutations in ovarian cancer patient cohorts were previously studied by several other groups.Citation12-Citation14 In particular, the missense variant of CHEK2 I157T was significantly associated with ovarian cystadenomas, borderline ovarian cancers, and low-grade invasive cancers, but not high-grade ovarian cancer.Citation13 In another study, Baysal et al. performed single nucleotide polymorphism genotyping by pyrosequencing and identified del1100C and A252G variants of CHEK2.Citation12 However, as the statistical differences of the variant frequencies were insignificant when compared with controls, it was suggested that variations in CHEK2 were not associated with pathogenesis of ovarian cancer. In Russian ovarian cancer patients, the effects of CHEK2 1100delC on ovarian cancer pathogenesis were studied, but no associations were observed.Citation14 These studies were mainly focused on screening of some well-reported variants of the CHEK2 gene, e.g., del1100C, A252G, and I157T. Therefore, mutations of other regions of CHEK2, as well as its association with ovarian cancer pathogenesis and patient survival were not studied in detail. Moreover, in these previous reports, the authors studied the association of specific variants with respect to disease pathogenesis, but not with respect to patient survival events and times. To the best of our knowledge of literature reports, the association of CHEK2 mutations with prognosis of HG-SOC patients is currently unclear or insignificant.Citation12-Citation14
Nevertheless in several other diseases, there are evidences that CHEK2 mutations were correlated with adverse clinical outcomes. In superficial bladder cancer, the use of CHEK2 mutational status as a prognostic factor was suggested, as they were associated with tumor recurrence risk, the number of recurrences as well as presentation of a poorer clinical course.Citation15 In breast cancer, the clinical impact of CHEK2 alterations were studied in Bulgarian breast cancer patients, and results showed that CHEK2 mutations can increase the risk of death in these patients.Citation16 Results from both retrospective and prospective cohort studies of breast cancer patients revealed that CHEK2*1100delC germline mutation introduced additional risks of developing a second breast cancer, as well as unfavorable long-term recurrence-free survival rates and distant metastasis-free survival.Citation17,Citation18 In glioblastoma, while there was no association of CHEK2 mutations with disease formation, there was significant correlation of CHEK2 gene polymorphism with adverse patient prognosis.Citation19 In view of these published studies, which reported significant associations of CHEK2 mutations with adverse clinical outcomes, it is then important to investigate if CHEK2 could function as prognostic factors in HG-SOC patients.
However, as the interconnectivity and interactions of related genes is a common feature of biological processes in either normal or tumor tissues, other genes involved in the biological process or associated with prognostic significance would be studied as well, with the aim of defining a classifier capable of patient stratification.Citation20 In this aspect, new methods for prediction and identification of cancer risk assessment, stratification, overall survival prognosis, and therapy response prediction for patients with HG-SOC are urgently needed. In this study, we performed integrative bioinformatics and statistical analysis of genome-wide mutational and clinical data sets of HG-SOC patients from TCGA to identify prognostic genes (biomarkers) whose mutation status could stratify patients into distinct survival subgroups. We also aim to discover novel susceptible gene signatures related to poor prognosis of patients, where distinct tumor subgroups are characterized and potentially driven by germline or somatic mutations of these signature genes.
Results
Genome-wide mutational spectrum and statistical distribution of gene mutations
Exome sequencing experiments via Illumina or ABI SOLID sequencing platforms were performed for 334 HG-SOC tumor samples at the Human Genome Sequencing Centers (HGSCs): Baylor College of Medicine (BCM), Broad Institute Genome Center (BI) and Genome Institute at Washington University (WUSM). The data was analyzed by the TCGA research network as previously described.Citation5 We downloaded the processed level 2 mutational data from the TCGA data portal for further analysis (see “Materials and Methods”).
The data set contains 21 978 putative mutations across all studied genes and patients (Fig. S1). We removed 4339 data where the mutational status were unknown, and the remaining 17 639 mutations were comprised of germline, loss-of-heterozygosity (LOH) or somatic mutations across 9083 unique gene symbols (Fig. S1; Table S1). Each of these mutations was annotated with respect to their mutation status, variant types, and variant classification. For mutation status, somatic mutations comprised 93.3% of 17 639 mutations, whereas LOH and germline mutations comprised 1.2% and 5.5%, respectively (Table S2A). The single nucleotide polymorphism (SNP) was the most commonly observed variant type (94.8%) in comparison with DNP, deletion, or insertion (Table S2B). For variant classification, missense mutations, nonsense mutations, deletions, insertions, RNA, splice site mutation comprised a major fraction of all mutations (78%; Table S2C). In this part of our analysis, we included silent mutations (21.7%), as they could be conditional pathogenic mutations due to modifications of the underlying DNA sequences, which potentially could affect miRNA binding sites, regulatory signaling, RNA–protein binding, post-transcriptional events, and cytosol–nuclear transport.Citation21-Citation23
To provide genome-wide analysis of the relative frequency of occurrence of mutations within a gene and the relative frequency of gene mutations across the patient samples, we first generated a 2-dimensional gene-patient tumor sample association matrix, where the rows and columns correspond to 9083 unique gene symbols and 334 unique tumor sample IDs, respectively (Table S3).
For each gene in the matrix, we calculated the number of tumor samples with reported mutation in this gene, as well as the total number of mutation events across all tumor samples (Table S3). Subsequently, the marginal frequency distribution function of the number of mutated tumor samples that are distributed across individual genes (n = 9083) was estimated. shows that the frequency distribution of the number of tumor samples is skewed with a long right tail, representative of observations that few genes are highly mutated, whereas many other genes are less mutated in HG-SOC tumor samples. Such function belongs to a family of skewed probability distributions, which are observed often in many evolving and interactive (interconnecting) systems in which the birth–death processes are occurring and driving a system by evolution toward the complexity and self-organization (see “Materials and Methods”).Citation24,Citation25 In such models, the skewed form of the function is strongly population/sample size and scale-dependent. In the context of cancer driving mutations, Kolmogorov–Waring (K–W) model could help us to better understand the nature of enormous variability and plasticity of mutation events and the role of common and rare mutations in cancer origin and its progression.Citation24,Citation25 Practically, K–W model allows estimation of a fraction of the mutated genes
Figure 1. Statistical characteristics of gene mutations in HG-SOC. (A) Frequency distribution of mutations in susceptible driving genes. (B) Number of distinct mutations against number of mutated samples. Scatter plot of genes, where the vertical axis corresponds to the number of mutations across all samples and the horizontal axis corresponds to the number of samples with at least one mutation for a given gene. The diagonal represents the hypothetical scenario where number of mutations per sample for each gene is 1. Both axes are log10-transformed.
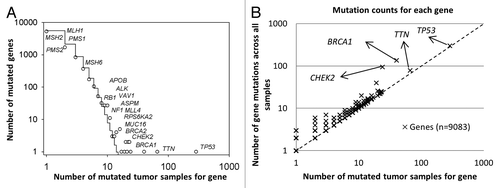
which could be observed if the numbers of mutated tumor samples are increased (see “Materials and Methods”). In this case, our best-fitted K–W function yields the following parameters:
and
Thus, the total number of susceptible target genes Ns could be estimated by the formula:
Ns = Nb/a = 9083 × 9.5/3.944 = 21887 genes
This result suggests that expected number of potential target genes for mutagenesis come up to entire set of protein-coding genes in humans. As analysis of TCGA data only revealed 9083 mutated genes, the discrepancies could be false negatives, and new rarely mutated genes in ovarian cancer could be observed via increasing sample sizes (the number of tumor samples) and improvement of technology.Citation8,Citation26
Examples of mutated genes with low, moderate and high frequency in the HG-SOC samples are shown in . In particular, shows the relative high frequencies of tumor samples having mutations in BRCA1 (40 of 334 tumor samples) or BRCA2 (23 of 334 tumor samples) genes in the TCGA patient cohort. In contrast, mutations in DNA-mismatch repair genes MLH1, MSH2, MSH6, PMS1, and PMS2 occurred in much fewer patients (1, 1, 4, 2, and 1 out of 334 patients, respectively). These genes are commonly associated with Lynch syndrome and accounts for a subset of hereditary ovarian cancers.Citation27,Citation28
We also counted an abundance of mutated sites for each gene across all samples and generated a scatter plot where each point represents each gene, and the axes represent the number of patient tumor samples with at least one mutation in that gene against the number of total mutation sites for the gene across all samples (; Table S3). The diagonal line represents a hypothetical situation where each gene was mutated on average once per sample, if any at all. Our results indicated that while TP53 is the most highly mutated gene and was observed in almost all HG-SOC patients, the number of mutations with the gene locus in each patient sample is relatively low, i.e., on average, only 1 TP53 mutation was observed for each patient (298 mutations across 285 HG-SOC patients). Altered or loss of function of this tumor suppressor appears to be critical for HG-SOC carcinogenesis. The other cancer susceptibility gene BRCA2 was less frequently mutated, and only 25 mutations were observed in 23 HG-SOC patients. CHEK2 and BRCA1 mutations appear to be generally mutually exclusive in HG-SOC patients, as only 18% (4 of 22) of patients with non-silent CHEK2 mutations harbored BRCA1 mutations (Table S4). Similarly, only 18% (4 of 22) of patients with non-silent CHEK2 mutations harbored BRCA2 mutations (Table S4).
A mutation cluster is defined by genes involved in various cell cycle-, apoptosis-, DNA damage-, and DNA repair-related processes
To study the structure of gene–patient tumor sample mutation associations, we performed unsupervised hierarchical clustering on the mutation association matrix (Table S3). Interestingly, all 14 experimentally verified mutated genes reported in previous TCGA studies were included in our subset of the TCGA significantly mutated genes, if at least 2 patients were considered as a confidence threshold (Table S3).Citation5,Citation8 This suggests that the false positive mutation rate could be greatly reduced if we consider only genes whose mutations are detected in at least 5 HG-SOC patients. Applying this threshold, a subset of 455 genes with observed mutations in at least 5 of 334 (1.5%) HG-SOC tumors was selected for unsupervised hierarchical clustering of the gene–patient tumor sample mutation association matrix (Table S3). The full heat map for 455 genes and 334 HG-SOC patients is shown in Figure S2. As expected, the TP53 mutations were observed in a majority of HG-SOC patients (85%, 285 out of 334). However, the intensity of TP53 mutations in each patient was low: generally only one TP53 mutation was observed in each p53 gene for a given patient. Interestingly, mutation sites along the TP53 locus appears to be randomly located across the exons, and there appears to be no strong positive clonal selection for any particular gene variant (Table S5). The frequencies of mutations of other genes such as BRCA1 (12.0%, 40 out of 334) and CHEK2 (7.2%, 24 out of 334) across all tumor samples were relatively smaller. However for affected patients, the intensity of these gene mutations per tumor sample is more than 3 times higher than for TP53 (on average, 3.38 and 3.96 mutations per patient for BRCA1 and CHEK2, respectively). and the heat map (Fig. S2) provide visual presentation of these findings. It also confirmed our previous finding that mutations in BRCA1 and in CHEK2 were generally mutually exclusive (Fig. S2; Table S4).
Results from hierarchical clustering also revealed a distinct gene–patient cluster associated with CHEK2 (Fig. S2). This sub-cluster includes 58 gene symbols and 22 HG-SOC patients (). Within this cluster, mutations of CHEK2 appear to dominate, as multiple regions of CHEK2 were observed to be mutated in each of these patients (). The annotation of these 58 gene symbols are listed in Table S6. Analysis of these 58 gene symbols via DAVID Bioinformatics revealed that these genes are significantly enriched in protein kinase activity (TBK1, PIK3C2B, MET, PRKCI, CHEK2, ALK, MAP3K6, PTK2B, RPS6KA2, MAPK15, PDGFRA, ROR2, TNK2, and INSR), adenyl and purine ribonucleotide binding (KIF4B, KIF3B, GCLC, TBK1, PIK3C2B, MET, PRKCI, TP53, CHEK2, ALK, ABCA3, MAP3K6, PTK2B, RPS6KA2, MAPK15, PDGFRA, ROR2, TNK2, CHD6, INSR, EP400, and MYO5C), and disease mutations (MAD1L1, HNF1A, GCLC, MET, TP53, ITGB2, CHEK2, GLI2, GLI3, ABCA3, ROR2, INSR, SPTB, and FN1) (Table S7A). Further analysis via Metacore revealed significant association with immune response and DNA damage pathways as well as apoptotic and cell cycle gene networks (Table S7B and C). Network analysis of these 58 genes further identified a tight direct interacting network of 21 genes mostly involved in apoptosis, cell cycle control, DNA damage response, and immune response (). These biological categories and networks are strongly assigned to well-studied functions such as DNA damage, repair, cell cycle, and check point regulation (Table S8).
Figure 2. (A) Extracted sub-cluster of mutation matrix belonging to 58 genes and 22 patients, arranged via hierarchical clustering (Kendall–Tau distance, complete linkage). The intensity of the plot corresponds to the number of mutations (inclusive of silent mutations) observed for that gene and patient. (B) Direct interaction gene network of a subset of 21 genes identified from the mutation sub-cluster.
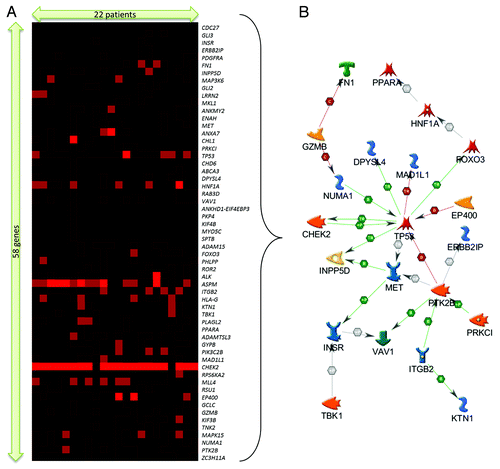
CHEK2 mutations are associated with poor prognosis of diagnosed HG-SOC patients
Our initial analyses of the mutational spectrum of patients diagnosed with HG-SOC revealed a distinct gene–patient cluster, where CHEK2 mutations appear to be highly concentrated in a few patients. Specifically, 97 mutations of CHEK2 were observed in 24 patient tumor samples, and these mutations encompassed allelic variants such as G- > A (27.8% of 97 CHEK2 mutations), C- > T (24.7%), G- > A (12.4%) or T insertion (1.0%) (Table S1). Focusing subsequent analysis on CHEK2, we examined if mutations in this gene were associated with patients’ overall survival times, and if it could be used as a prognostic survival factor for patients already diagnosed with HG-SOC.
We performed stratification of the TCGA HG-SOC patients based on the non-silent mutational status of the CHEK2 gene. In this analysis, a total of 311 patients with both mutational data and clinical information were studied (Table S9). Non-silent CHEK2 mutations were observed in 22 (7%) of 311 HG-SOC patients with clinical information. Kaplan–Meier survival curve of the patient subgroup with CHEK2 mutations exhibited significantly poorer overall survival times when compared with the subgroup with no CHEK2 mutations (P ≤ 0.01; ). Effectively, our results from retrospective study of TCGA data suggest that for patients already diagnosed with HG-SOC, non-silent mutations (germline, LOH or somatic) of the CHEK2 gene were greatly detrimental for patients’ overall survival times, as these patients did not survive beyond 5 y after initial pathologic diagnosis.
Figure 3. Kaplan–Meier survival curves of TCGA HG-SOC patients based on the non-silent mutational status of (A) CHEK2, (B) TP53, (C) BRCA1, and (D) MUC16.
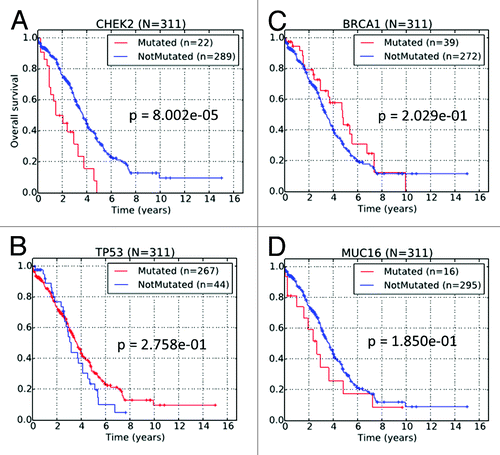
In TCGA HG-SOC data, genes such as TP53, BRCA1, or MUC16 were mutated with higher frequency than CHEK2, but unlike CHEK2, the mutational status of these genes could not independently stratify HG-SOC patients into survival-significant subgroups (). Despite the lack of statistical significance, there is some slight indication that mutation in MUC16, a known clinical biomarker of ovarian cancer, could be associated with poor patient survival. On the other hand, patient with BRCA1 mutation appears to be associated with better patient survival, which is consistent with several other published data.Citation29,Citation30 While TP53 is frequently mutated in HG-SOC and could be useful in disease diagnosis, our analysis revealed that in diagnosed patients, it was not effective as a prognostic marker of patients’ overall survival times ().
CHEK2 mutations are associated with poor response to therapy
In a previous study of breast cancer by Chrisanthar et al., it was reported that CHEK2 mutations were found to be associated with therapy resistance, which was defined as progressive disease on therapy.Citation31 Here, we investigate if the association between CHEK2 mutations with therapy resistance were significant in HG-SOC. From TCGA data, we categorized HG-SOC patients into 2 subgroups. The first subgroup consists of patients who exhibited progressive disease after primary therapy. The second subgroup consists of patients with partial response, stable disease, or complete response after primary therapy. Analysis via kappa correlation measure revealed that mutations in CHEK2 gene were associated with progressive disease with borderline significance (kappa = 0.1278, P = 0.05536; ). When silent mutations were excluded from the analysis, a slightly more significant correlation with therapy resistance was observed (kappa = 0.1422, P = 0.03769; ). Essentially, 25% of patients with CHEK2 mutations (5 of 20) showed disease progression, whereas only 8.8% of patients without CHEK2 mutations (21 of 237) showed disease progression. Therefore, our results indicate that CHEK2 mutations were associated with poor response to therapy.
Table 1. Kappa correlation of (A) CHEK2 mutations and (B) non-silent CHEK2 mutations with therapy resistance. Values in the contingency table represents the number of unique sample IDs corresponding to the row and column labels
Copy number and mRNA expression of CHEK2 do not appear to have significant influence on HG-SOC patient survival
To understand if other aspects of CHEK2 could be associated with patient survival, we consolidated patient information for CHEK2 across available data sets from copy number, mutation, expression, and clinical experiments (Table S9) and subsequently assessed their prognostic significance.
Copy number variation data was available for 356 patients. Analysis of copy number variation data for these patients revealed that CHEK2 was significantly amplified in 15 patients and deleted in 130 patients. The rest of the patients did not exhibit significant copy number variation. Subsequently, our analysis also showed that copy number of CHEK2 could not provide significant prognostic classification of HG-SOC patients (Fig. S3A). Also expectedly, samples with significant amplification of the CHEK2 region exhibited higher mRNA expression, whereas those with significant deletion have lower expression (Fig. S3B).
Expression data was available for 399 samples, which comprised 8 normal fallopian tube and 391 HG-SOC samples. Additionally, 370 of the 391 HG-SOC samples were described with tumor information such as histologic grade or tumor stage. Therefore, we investigated the expression profile of CHEK2 mRNA across the normal fallopian tube tissues and tumor tissues belonging to different histologic grades or tumor stages (Fig. S3C). The higher mRNA expression of CHEK2 in the tumors relative to the fallopian tube samples indicate the possible upregulation at early disease onset, probably due to compensatory actions, and suggest the possibility of using CHEK2 mRNA expression as an early diagnostic biomarker for HG-SOC. On the other hand, the prognostic ability of CHEK2 expression data to classify patients already diagnosed with HG-SOC into relatively low- or high-risk subgroups is limited (Fig. S3D). We applied our published computational algorithm, which assigns patients to relative low- or high-risk subgroups depending on an expression cut-off that was optimized by maximizing the separation of the 2 Kaplan–Meier survival curves,Citation32 to CHEK2 mRNA expression belonging to the 391 HG-SOC patients. While 370 of 391 samples were annotated with clinical information, 12 were incomplete, as they were without survival times and events. Therefore, survival analysis was performed on the 358 HG-SOC samples well annotated with clinical data. Our results suggest that mRNA expressions of CHEK2 were not significantly associated with HG-SOC patients’ prognosis (P = 0.2057; Fig. S3D).
Therefore, our results suggest that other aspects of CHEK2 such as expression or copy number variation could not be used as prognostic features for HG-SOC patients.
Observed mutations of CHEK2 are unlikely to alter phosphorylation events or protein structure
CHEK2 is a serine/threonine protein kinase which functions in the nucleus to regulate cell cycle, DNA repair, and apoptosis in response to DNA double-strand breaks.Citation11 As post-translational activation of Chk2 protein via phosphorylation events is required for its physiological function, we next checked if any of the CHEK2 mutations were localized at known or predicted phosphorylation sites. Known phosphorylation sites of CHEK2 were collected from the databases of UniProtCitation33 and Phospho.ELM.Citation34 Of all the mutations reported for CHEK2, only one mutation site was found to co-localize with a known phosphorylation site (Table S10). Mutation data from TCGA HG-SOC patients revealed that CHEK2 was mutated at a nucleotide coding for residue Thr-383 (hg18, chr22:27421808–27421808 at exon 11). It has been reported that auto-phosphorylation of Chk2 at Thr-383/Thr-387 within the activation loop of Chk2 kinase domain and at Ser-516 at the C-terminal region of Chk2 are essential for Chk2 activation.Citation35 However, mutations at the nucleotide coding for Thr-383 were observed in only 6 patients. Furthermore, as the mutations are synonymous, the same amino acid residue threonine would be coded, and, therefore, it does not currently appear that mutations here would lead to aberration of Chk2 function. Our results also showed that CHEK2 mutations are not co-localized with any other phosphorylation sites computationally predicted by NetPhos and PHOSIDACitation36,Citation37 (Table S10), which suggest that based on our current results, alteration of phosphorylation events may not be the key mechanisms leading to altered Chk2 behavior.
Next, we analyzed if the observed DNA mutations along CHEK2 could potentially modify the protein structure. Using data generated from RNA-sequencing experiments and downloaded from the Sage Bionetworks’ Synapse database,Citation38 we first examined the expression data across various CHEK2 isoforms and primary solid tumors belonging to 262 patients. We identified that the isoform uc003adu.1 (representing isoform 1 or A) is dominantly expressed when compared with other CHEK2 isoforms (Fig. S4). Then, we collected known secondary structures along the amino acid residues (isoform 1, UniProt ID: O96017) and compared it with the observed DNA mutations (Table S10). The DNA mutations at the 8 distinct sites of CHEK2 DNA could potentially alter the protein structure at 7 distinct amino acid residues (). Only one of the amino acid residues (Thr-383) occurred at a structured site of the protein. However, the secondary helix structure is unlikely to be disrupted, as the DNA mutation at this region was silent. For further visualization, we generated a representative protein crystal structure of physiological Chk2 and superimposed the 7 mutated residues to study the sites of mutations, relative to its surrounding 3-dimensional conformation (). From the initial crystallographic structure of Chk2 from Thr89 to Glu501 (PDB code 3i6u,Citation39 resolved at 3.0 Å), ModelerCitation40 was used to complete the few missing loops and to extend the C-terminal region of the kinase until Leu543. Molecular dynamics (MD) simulations were performed to obtain the relaxed state conformation of the protein structure at 50 ns (). From the figure, it could be observed that the mutated residues (represented by colored spheres) were mostly located at non-structured regions of the protein. Therefore, our results from protein modeling and MD simulations suggest that DNA mutations of CHEK2 were unlikely to disrupt the protein structure and affect its physiological function.
Figure 4. (A) Locations of DNA mutations along genomic schema of the CHEK2 locus. The exon blocks are numbered sequentially from 5′ to 3′. Inverted triangles represent the locations of mutation on the exon. The numbers above the inverted triangles indicate the number of patients with the mutation (inclusive of synonymous mutations). (B) Locations of the expected mutations on the amino acid sequence. The alphabet in the inverted triangle indicates the reference amino acid residue, whereas the numbers of patients with non-synonymous mutations are shown above the inverted triangle. The numbers in the rectangular blocks indicate the amino acid residues span. (C) A representative crystal structure of the relaxed state of Chk2 protein after computational modeling and molecular dynamics simulation. All Chk2 mutations are represented by colored spheres, which indicate the locations of residues corresponding to the DNA mutations after translation. The CHEK2 isoform 1 (NM_007194/NP_009125/O96017) was used as the reference isoform. The forkhead-associated (FHA) domain, kinase domain and nuclear localization signal (NLS) are marked in pink, blue and cyan, respectively. The Venn diagram compares the number of patients with the observed mutation at 2 distinct nucleotide positions. Figures are not drawn to scale.
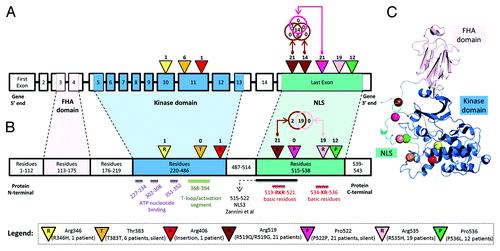
Observed mutations of CHEK2 could affect nuclear import of the protein
Next, as Chk2 is a nuclear protein and nuclear import requires the presence of nuclear localization signals (NLSs) along the amino acid sequence, we investigate if modifications of such signals were possible among these TCGA HG-SOC patients. It was previously reported that karyopherin-α2 (KPNA2) could recognize a NLS belonging to Chk2 and facilitate its nuclear translocation through the nuclear pore.Citation41 Specifically, of the 3 NLSs studied, Zannini et al. identified NLS3 as the key NLS involved in the nuclear localization of Chk2 in cells. The monopartite NLS3, which was computationally predicted via PSORT II,Citation42 occupies a stretch of short amino acids, spanning from residues 515–522 (amino-acid sequence: PSTSRKRP; ) of the protein. Mutation studies performed by Zannini et al. showed that mutation of this region resulted in the cytoplasmic localization of Chk2 protein, which suggested the inability of altered Chk2 in translocating to the nucleus. Interestingly, our results reveal that along this short NLS sequence, there were 3 distinct nucleotide (corresponding to 2 amino acid residues – R519 and P522) sites of mutation belonging to TCGA HG-SOC patients (; Table S10). The mutation observed at chromosomal coordinate chr22:27413951 was a silent mutation observed in 21 patients (P522P, labeled purple in ). The 2 non-silent mutations at contiguous nucleotide positions chr22:27413961 and chr22:27413962 were present in 14 and 21 patients, respectively (R519Q/R519G, labeled brown in ). In total, 21 patients were observed to exhibit mutations at either of these 2 sites, and together with findings that CHEK2 mutation are detrimental to patient’s survival, our results suggest a possibility that mutations in the NLS region could adversely affect the nuclear import of Chk2, reduce the protein level of effective and functional Chk2, impact Chk2-associated repair pathways, and eventually contributing to poor patient survival.
As there were 2 other mutation sites downstream of the NLS identified by Zannini et al., we used an alternative computational tool, cNLS,Citation43 to predict NLSs along the Chk2 protein sequence (isoform A, NP_009125 – 543 amino acid residues). Results revealed the possibility of a functional bipartite NLS from amino acid residues 517–538 (TSRKRPREGE AEGAETTKRP AV; ). This region encompasses 2 basic residue clusters connected by a 12-amino acid residue linker. Interestingly, of the 21 TCGA HG-SOC patients observed with mutations at the nucleotide coding for R519, concurrent mutations of nucleotide coding for R535 were observed for 90% (19 of 21) of the patients (labeled brown and pink in ). Results from this analysis suggest that the effective NLS region could be longer than the one identified by Zannini et al.Citation41 Moreover, the co-occurrences of mutations coding for residues at both key components (basic residues) of a bipartite NLS further implied the possibility of positive clonal selection in the tumor tissue samples of these 19 HG-SOC patients.
Supporting evidences that observed CHEK2 mutations are real events
Our current study investigated the spectrum of mutations reported by the TCGA Research Network and identified a prognostic signature that could significantly stratify patients into low- or high-risk subgroups. About 87% of mutations (15346 of 17639 mutations) reported by the TCGA Research Network have been validated. However, for CHEK2, only one SNP at nucleotide position 27422947 of chr22 (hg18) was among those independently validated by the TCGA Research Network (Table S1), and subsequent efforts should focus on experimental validation of CHEK2 mutations for either the same TCGA patient cohort or a new patient cohort. Nevertheless, several independent reports have indicated the presence of CHEK2 mutations in ovarian cancer as well as other diseases, which supports the possibility that the CHEK2 mutations observed in the TCGA ovarian cancer data could be real.
In an independent study by Walsh et al., they identified mutations in several genes in inherited ovarian, fallopian tube, and peritoneal carcinoma via massively parallel sequencing.Citation9 From their data, 16% (45 of 273) of ovarian cancer patients exhibited either BRCA1 or BRCA2 mutations, which compared similarly to our results from analysis of the TCGA HG-SOC study, where 57 of 334 patients (17%) were reported with mutations in either gene (Table S4). While we observed that 7% (22 of 334) of TCGA HG-SOC patients exhibited non-silent CHEK2 mutations (Table S4), only 2% (5 of 273) of ovarian cancer patients studied by Walsh et al. exhibited loss-of-function CHEK2 mutations. The difference in observations for the CHEK2 genes could be due to the fact that only loss-of-function CHEK2 mutations were reported by Walsh et al., who only included “damaging” variants, defined by yeast cultures whose “growth was significantly poorer than that of WT-CHEK2 and did not differ significantly from the negative control.”Citation9 Furthermore, as Walsh et al. only studied inherited germline mutations across all histological types (serous, carcinoma, undifferentiated, endometrioid, clear cell, and carcinosarcoma), these differences in study design and including all histological types could have resulted in the under-observed frequency of Chk2 mutations in their study when compared with our analysis of HG-SOC data from TCGA. Nevertheless, we noted that 4 of the 5 CHEK2 mutations observed by Walsh et al. fell within the 10th and 11th exons of isoform A (NM_007194), which, interestingly, also contained mutations from the TCGA HG-SOC data, albeit at different amino acid residues ().
Figure 5. Locations of CHEK2 mutations on the expected amino acid residues for various cancers and data sets. The CHEK2 isoform A (NM_007194) is used as the reference isoform. Red boxes indicate the location of mutations.
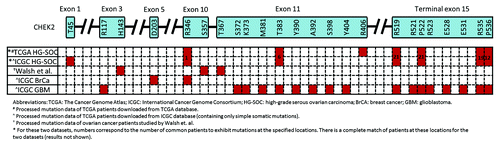
We next compared CHEK2’s mutation spectrum in other diseases studied by TCGA. As CHEK2 is frequently regarded as a third breast cancer-specific gene,Citation14 we investigated the spectrum of CHEK2 mutations in breast cancer patients. At exon 10 of isoform A (NM_007194), a nucleotide coding for arginine at residue 346 (arg-346) was found to be somatically mutated in breast cancer. Interestingly in HG-SOC, mutation at the adjacent nucleotide within the same codon was observed, possibly leading to alteration of the same arginine residue (). Next, we compared the sites of CHEK2 mutations in TCGA HG-SOC with those observed in TCGA glioblastoma. Exon 11 of isoform A was observed to exhibit mutations in both HG-SOC and glioblastoma. Also remarkably, at the terminal exon of CHEK2 isoform A, all the mutated sites observed in HG-SOC were also observed in glioblastoma.
In view of these circumstantial supporting evidences of CHEK2 mutations in other independent studies of ovarian cancer or other diseases, it suggests that the CHEK2 mutations observed in TCGA HG-SOC could be true signals of real mutational events.
Identification and characterization of a 21-gene mutational prognostic signature
Next, we studied the prognostic significance of the mutational status of 251 genes with observed mutations in the cancer tissue of at least 5 patients (accompanied with available patient survival information). Our results revealed that there are 21 genes that were non-silently mutated in the cancer tissues of at least 5 patients and can independently stratify HG-SOC patients into prognostically significant subgroups (P ≤ 0.05, ).
Table 2. Prognostic significance of the 21 survival significant genes based on non-silent mutational status (Log-rank statistic P value ≤ 0.05, #mutated ≥ 5 and #non-mutated ≥ 5)
The top 3 mutated genes with prognostic significance among these 21 genes include CHEK2, RPS6KA2, and MLL4 with non-silent mutations in 22, 23, and 20 patients, respectively (). Interestingly, our previous results from hierarchical clustering also revealed that these genes are clustered together (). Quantitatively, kappa correlation analysis further revealed the high co-occurrences of CHEK2 mutations with RPS6KA2 or MLL4 mutations (kappa ≥ 0.75, P ≤ 5E-20; Table S4).
Overall, 13 genes (CHEK2, RPS6KA2, MLL4, ENAH, ADAMTSL3, RSU1, LRRN2, MET, MAP3K6, MAPK15, GYPB, GLI2, and PTK2B) of the 21-gene list were significantly enriched in the CHEK2-associated mutation sub-cluster (fold enrichment = 4.89; P = 6e-08, ). As patients with CHEK2 mutations were generally observed to exhibit mutations of genes in the mutation cluster (), we focused on these 21 genes to create a combined mutational prognostic signature. Among these 21 genes, the mutation status of 20 genes exhibited pro-oncogenic behavior, where mutations were associated with poorer overall survival (). In contrast, only ERN2 exhibited tumor-suppressive behavior, where mutations were associated with better overall survival. Assuming the null hypothesis that each identified survival significant gene could either exhibit tumor-suppressive or oncogenic behavior with equal probability, results from the binomial test indicate that the bias is non-random and statistically significant (P = 1.049e-05). This indicates that the association of mutations with the prognostic function of these genes is biologically reasonable and relevant. Using these 21 mutational prognostic genes, the ovarian cancer patients were classified into the lower-risk subgroup if there were mutations in ERN2 or there were no mutations in all the 20 pro-oncogenic genes. On the other hand, patients with mutations in any of the 20 pro-oncogenic genes and without ERN2 mutations were classified as higher risk. Results from the Kaplan–Meier survival plots revealed that the 21-gene mutational prognostic signature-defined patient subgroups were significantly stratified and associated with overall survival times (P = 7.31e-08, ). Specifically, the 5-y overall survival rates of the relatively low- and high-risk subgroups are 37% and 6%, respectively. We further studied the clinical characteristics of these 2 subgroups of patients, and our results revealed that the high-risk patients defined by the 21-gene mutational prognostic signature are correlated with aggressive behavior of the disease. Specifically, patients defined as relatively high-risk of disease development by the 21-gene mutational prognostic signature was twice as likely to exhibit progressive disease in contrast to the relative low-risk subgroup (high risk: 8 of 50 patients = 15%; low risk: 18 of 208 patients = 8.7%; ). However, the statistical significance is borderline (kappa = 0.08984, P = 0.06065). Nevertheless, the trend suggests that mutations in these genes could be important factors in therapy resistance.
Figure 6. (A) Venn diagram of common genes between the identified gene mutation cluster and genes whose mutation status are prognostic significant. (B) Prognostic stratification based on mutational status of 21-gene signature. (C) Prognostic stratification based on the mutation of the CHEK2 gene and 20-gene signature.
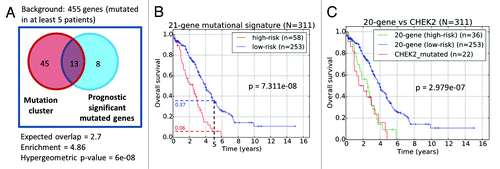
Table 3. Kappa correlation of patients classified by the 21 gene mutational signature with therapy resistance. Values in the contingency table represents the number of unique sample IDs corresponding to the row and column labels
The detailed annotations of the genes in the 21-gene mutational prognostic signature are listed in Table S11. Subsequently, we performed gene ontology analysis of the 21 genes of the signature using DAVID Bioinformatics. Results indicate that these genes are strongly enriched in functions associated with kinase activity, ATP binding, and phosphorylation (Table S12A). In parallel, analysis via MetaCore also revealed association of pathways associated with DNA damage-induced responses, as well as gene networks associated with cell cycle, DNA repair, and apoptosis (Table S12B and C).
Identification of 2 tumor subclasses from the signature-defined high-risk subgroup
Next, to investigate if the prognostic significance of the 21-gene mutational prognostic signature () could be due to the contribution of CHEK2 mutations alone, we excluded patients with CHEK2 mutations from the signature-defined high-risk subgroup. Our results indicated that patients diagnosed with either CHEK2 mutations, or mutations in any of the remaining 20-genes mutational prognostic signature (excluding CHEK2) exhibited rather similar overall survival patterns (). The poor prognosis of patients exhibiting mutations in any of the genes in the 20-gene mutational prognostic signature suggests that the aberrant functioning of these genes in the HG-SOC genome could inversely impact patients’ post-surgery response to therapy, independent and regardless of the effects of CHEK2 mutations.
To study the possible heterogeneity of the poor prognosis patient subgroups identified via CHEK2 or the 20-gene mutational prognostic signature, we generated a heatmap that represents the joint gene–patient mutation frequency matrix for the 21 genes and 58 high-risk patients (Fig. S5A). We further characterized these mutations in terms of germline, LOH, or somatic mutations, and our results showed that the mutations of genes in a subset of 22 patients with CHEK2 mutations appeared to be of germline or LOH origin, whereas that of the other 36 high-risk patients appeared to be somatic (Fig. S5B–D). Specifically, in the subset of 22 patients with CHEK2 mutations, 16 of the patients (73%) exhibited non-silent germline CHEK2 mutations (). Interestingly among these 16 patients, strong co-occurrences of germline mutations in RPS6KA2 and MLL4 genes were observed in 15 (94%) and 12 (75%) patients, respectively. Re-analysis of the entire joint gene–patient mutation matrix (of 455 highly mutated genes and 334 patients) also revealed similar findings that genes from the CHEK2-associated mutation sub-cluster were associated with germline or LOH rather than somatic mutations (results not shown).
Table 4. Genetic and clinical characteristics of CHEK2-MLL4-RPS6KA2 determined EOC tumor sub-class (G, germline; S, somatic; L, LOH)
Thus, our analysis revealed that among the high-risk patients identified via our 21-gene mutational prognostic signature, there could be 2 distinct tumor subclasses whose pathogenesis could be initially driven by either inherited germline mutations pre-determined by CHEK2, RPS6KA2, and MLL4, or mostly somatic mutations of the other signature genes (Fig. S5B–D).
Allelic changes in prognostic genes and high-risk patients are unique
From the entire list of 17639 mutations studied in this work, a subset of 313 mutations was associated with our 21-gene mutational prognostic gene signature and relative high-risk patients (Table S1). For both the entire and subset list of mutations (hereby termed “background set” and “high-risk subset”, we calculated the frequencies of tumor allelic changes with respect to the reference genome (Table S13). Fisher exact tests were performed to assess the enrichment of each variant in the high-risk subset (of genes and patients) when compared with that observed for the entire background set (Table S13). Our results indicate that while G- > A, C- > T, G- > C, C- > G, G- > T and C- > A mutations were mostly commonly observed in both the background as well as the high-risk subset, there were insignificant differences in their relative ratios (P ≥ 0.05). On the other hand, G- > GG insertion observed for MLL4 and PPP1CC genes in the background set were almost entirely found in our high-risk subset (94.7%, 18 of 19, fold-change = 53.4, P = 3.45E-31). Other variants such as T- > (C/C) and C- > (T/T) mutations were enriched in the high-risk subset. The 13-fold enrichment of the T- > (C/C) mutation (P = 9.22e-19) corresponds to 22 mutations (20 in RPS6KA2 and 2 in ATR); the 10-fold enrichment of the C- > (T/T) mutation (P = 8.8e-17) corresponds to 23 mutations (12 in CHEK2, 8 in PTK2B, 1 in GLI2, MET and TNC). Such strong deviations from background set appeared to be characteristics of the mutations associated with the poor disease outcome prognosis in HG-SOC. In future, the detailed study of enriched allelic variants in the high-risk subset (e.g., G- > GG, T- > C, C- > T, G- > C, G- > A, C- > G etc.) in these susceptible genes could potentially unravel upstream mechanisms contributing to these variants that are associated with poor patient prognosis in HG-SOC.
Discussion
This study focused on identification of mutational biomarkers which: (1) could be risk predictors of hereditary ovarian cancers distinct from those with BRCA1/BRCA2 germline mutations or mutations associated with Lynch syndrome and simultaneously; (2) can be used as novel prognostic factors for HG-SOC.
Overall, our results indicated that: (1) mutation of CHEK2 gene could be an important risk and poor prognostic factor for patients with HG-SOC; (2) a mutational signature comprising of 58 relatively frequent mutated genes in 7% of HG-SOC could identify HG-SOC patients significantly associated with poor prognosis; (3) a combined mutational signature comprising of 21 genes can significantly stratify a cohort of HG-SOC patients into relatively low- or high-risk subgroups; and (4) germline mutations of CHEK2 and/or RPS6KA2 and/or MLL4 genes could be used as risk factors in predicting healthy women’s risk to HG-SOC initiation and development.
Mutations of CHEK2 in HG-SOC could affect nuclear localization and lead to poor clinical outcomes
Many published mutational studies focus only on specific classes of mutations such as somatic or germline variants. The focus on germline or somatic mutations would be appropriate for specific studies when one is interested in inherited risk of developing a particular disease upon birth, or identification of driver mutations for disease development at later stages of life, respectively. For prognosis purpose, whether the mutation is due to early inheritance or later-stage environmental factors is of less relevance in our studies. As a result, we included all classes of mutations during prognosis stratification.
Interestingly, HG-SOC patients that carry Chk2 mutations are at higher risk of mortality. But importantly, it could also prompt further studies into alternative targeted therapy for these patients. A possible explanation of why Chk2 mutations are associated with adverse patient prognosis could be due to induction of chemo-resistance, of which we reported significant correlation of CHEK2 with therapy response (kappa = 0.1422, P = 0.03769, ). In fact, CHEK2 mutations’ contributions to chemo-resistance and continued disease progression could be inferred from a study of breast cancer. Epirubicin, as a chemotherapy drug, is one of the drugs used to treat breast cancer via slowing or stopping the growth of cancer cells. Chrisanthar et al. reported that germline CHEK2 mutations contributed to therapy resistance, and the mutation Arg95Ter completely abrogated Chk2 dimerization and kinase activity.Citation31 We observed that this mutated region corresponds to a β-strand structure (amino acid residues 94–98, ARLWA). However, in TCGA HG-SOC patients, there were no observed mutations within this region. Additionally, none of the observed mutations in TCGA HG-SOC patients occurred in annotated secondary structures, ATP-binding site, active site, FHA domain, or kinase domain (Table S10). Therefore, there appears to be insufficient evidence that CHEK2 mutations observed in TCGA HG-SOC patients could disrupt the protein structure or kinase activity and contribute to chemo-resistance. In ovarian cancer, cisplatin rather than epirubicin was used as the chemotherapeutic agent, and evidence of Chk2-induced chemo-resistance upon cisplatin treatment in ovarian cancer has been reported. Zhang et al. reported that cisplatin treatment could degrade Chk2 protein, and the reduced level of Chk2 could hinder cell cycle control, prevent cell apoptosis, and contribute to chemo-resistance of the tumors.Citation44 Chk2 degradation may be one of the primary mechanism by which a large number of clinically relevant tumors develop the acquired resistance to DNA damage agent. With regards to the patients who exhibited CHEK2 mutations, the loss of function of one copy via either somatic or germline mutation could result in reduced copies of CHEK2 in the nucleus, and subsequently upon cisplatin treatment, the effects of CHEK2 degradation could be accentuated and ultimately detrimental for patient survival. Interestingly, the reason why the observed mutations of CHEK2 might initially contribute to loss of protein functions could be attributed to the lack of protein localization in the nucleus. The lack of nuclear localization of Chk2 is likely to contribute to deviation from physiological activity and leads to undesirable effects. Our analysis revealed that in 21 HG-SOC patients of the TCGA cohort, CHEK2 mutations occurred within a NLS that was previously reported by Zannini et al. to be critical for nuclear import of the protein (). Mutations of the NLS were reported to inhibit nuclear import of the Chk2 protein,Citation41 leading to reduced functional copies of Chk2 in the nucleus. It appears plausible that mutations along the NLS of the CHEK2 gene could lead to reduced levels of Chk2 proteins in the nucleus, and upon cisplatin treatment, the protein levels would be further depleted, which could potentially lead to chemo-resistance of the tumors and adverse patient survival. Our results showed that HG-SOC patients who exhibited CHEK2 mutations were significantly associated with poor clinical outcomes and did not survive beyond 5 years after initial diagnosis (; ).
Observed CHEK2 mutations are unlikely to affect post-translational modifications
Next, we studied if CHEK2’s association with poor patient prognosis could be due to modification of the phosphorylation sites. However, none of the observed mutations in CHEK2 occur along any currently known and annotated phosphorylation sites. Therefore, we collected computationally identified phosphorylation motifs from the literature,Citation45,Citation46 and investigated if any of the key residues along the motifs are mutated in TCGA HG-SOC patients. Our results revealed that despite their close proximity, none of the observed mutations occurred at the phosphorylation sites or the key motifs surrounding the phosphorylation sites. Furthermore, our analysis revealed that the region surrounding the CHEK2 mutations does not seem to contain strong protein secondary structure, and therefore it may currently seem unlikely that aberrations of post-translational modification of the Chk2 protein are contributory factors leading to poor survival prognosis of affected patients. However, the effect of CHEK2 mutations on protein dimerization or physical interaction with other protein partners could be investigated in future studies.
Possible influences of silent mutations
While we hypothesize that the mutations observed along the CHEK2 could affect nuclear translocation of the translated protein, other mechanism involving silent mutations could also be involved.
In our study, we observed that 21 HG-SOC patients exhibited silent mutations at chr22:27413951 (P522P; ). Traditionally, silent DNA mutations which encode for the same amino acid residues were assumed to have negligible effect on a protein function. However, recent studies have suggested that silent mutations could affect downstream protein functionality via various mechanisms. For instance, alterations to the DNA triplet codon could alter the binding sites of miRNA, leading to alteration in translational repression efficiency and downstream signal networks.Citation21 When we investigated whether the mutation at chr22:27413951 (P522P; ) could potentially alter miRNA binding sites, our results from sequence alignment indicate that the specific region was not targeted by any of the currently known human mature miRNAs (results not shown). Therefore in our study, alteration of miRNA target sites via synonymous mutation is unlikely to have any effect on mRNA stability and its subsequent translation.
Next, single synonymous DNA mutation can affect mRNA secondary structure, folding, stability and, consequently, the regulation of the translated protein as was reported for the human dopamine receptor D2 gene.Citation22 It was also suggested that synonymous mutations could affect translational efficiency of the amino acid residue due to the variation and asymmetry of tRNA abundance in cells.Citation21 Even in cases where synonymous mutations do not affect mRNA or protein levels, the function of the translated protein could be altered. In MDR1 gene, it was shown that a synonymous polymorphism resulting in a rare triplet codon can alter substrate specificity of the MDR1 protein, possibly due to deceleration of the translation rate at that amino acid residue, which, in turn, affects protein folding.Citation23 The strong overlap in 14 common patients exhibiting both the silent mutation and non-silent mutation at the last exon () appears to suggest a possible positive selection of these mutations, and future studies could focus on elucidating the possible influence of silent mutations on eventual protein expression and functionality.
Potential clinical application of the 21-gene mutational signature for prognosis and therapeutics design
While CHEK2 mutation appears to be the most important with respect to patient classification based on their survival patterns, we identified a total of 21 genes that could independently and significantly stratify patients into low- or high-risk subgroups based on their mutational status (). Applying the 21-gene mutational prognostic classifier to the TCGA patient cohort resulted in significant stratification of patients into 2 survival significant subgroups where the 5-y overall survival rates for the low- and high-risk subgroups are 37% and 6%, respectively (P = 3.8E-09, ). Furthermore, stratification based on the mutational status of CHEK2 alone, or of the remaining 20-gene signature, allows us to reject the hypothesis that the prognostic value of the 21-gene mutational prognostic signature was due to the contribution of CHEK2 alone (). Rather, this shows that a poor prognosis subgroup could be identified based on a 20-gene signature even in the absence of CHEK2 mutations. For prognosis purpose, while the use of our 21-gene mutational prognostic signature in patient risk prediction appears promising from our retrospective study of the TCGA patient cohort, prospective studies would be eventually required to validate the use of the signature in a clinical setting.
Interestingly, among the 21 genes whose mutational statuses were most suitable for prognostic applications, gene functions associated with protein kinase activity, ATP-binding, phosphorylation, DNA damage response, apoptosis, or cell cycle regulation were enriched (Tables S11 and S12). Our results are perhaps unsurprising, as it has been reported that many kinases are potentially oncogenes and could contribute to etiology of cancers or other diseases.Citation47,Citation48 The design of inhibitors for various protein kinases is therefore an active field of cancer therapeutics research today.Citation49,Citation50 Our findings that kinases such as CHEK2 or RPS6KA2 are associated with patient survival are not only important for patient stratification, but probably could also spur future efforts in designing inhibitors if the deleterious influences of mutated copies are validated and confirmed. However, patients with characterized mutations of CHEK2 or RPS6KA2 only represent a subset of the HG-SOC patients. Generally, we observed that most HG-SOC patients were characterized by mutations in only a few genes (Figs. S2 and S5), which is consistent with the general consensus that patient–gene mutational profiles are heterogeneous and sparse.Citation51 Nevertheless, it has been postulated that individual patients could exhibit mutations in different genes that are functionally related via gene networks corresponding to cancer hallmarks.Citation10,Citation51 Therefore, any particular biological process could be impacted via aberrations of any of its member genes. The understanding of the heterogeneous nature of mutations in HG-SOC patients could present an opportunity for more effective and targeted treatments in future.
Potential clinical application of the 21-gene mutational prognostic signature for risk prediction of developing HG-SOC
Our analysis of 58 high-risk HG-SOC patients identified via the 21-gene mutational prognostic signature also revealed 2 distinct tumor subtypes, which could arise from 2 different tumor etiological factors. The first tumor subclass (or patient subgroup) was clearly characterized by germline mutations or LOH of genes such as CHEK2, RPS6KA2, and MLL4 (Fig. S5). In contrast, for the other tumor subclass (or patient subgroup), germline mutations of these genes were not observed. Rather, this tumor subclass appears to be the result of spontaneous somatic mutations of the other signature genes in the presence of TP53 mutations that typically characterized HG-SOC tumors.
In fact, ovarian cancer is highly heterogeneous, with various driver genes involved in the development of several cancer subtypes ().Citation7 Our results suggest that around 11.6% of HG-SOC tumors could possibly be initiated due to spontaneous somatic mutations of the genes in the signature in the presence of TP53 mutations. Also, within HG-SOC that is characterized by TP53 point mutations and genome instability, inherited germline CHEK2 mutations may confer susceptibility and be involved in the initiation, development, and progression of tumors in about 7.1% of HG-SOC patients (). Our results also suggest the possibility that germline mutations of CHEK2, RPS6KA2, and MLL4 could be used as risk factors to predict a person’s risk of developing HG-SOC. For CHEK2, studies have been conducted to study the effect of gene variants on ovarian susceptibility, but associations were reported to be insignificant, possibly due to the rare occurrence of CHEK2 mutations, small tumor sample size, lack of appropriate HG-SOC patient samples, or low resolution of variant detection of available samples.Citation12-Citation14,Citation52 Nevertheless, our results from analysis of a high quality HG-SOC data set from TCGA has enabled us to uncover a potential but previously uncharacterized association of disease susceptibility due to CHEK2 germline variants.
Potential clinical application of CHEK2 expression in early diagnosis of HG-SOC
Also, as our results revealed that CHEK2 mRNA was upregulated in tumor samples relative to normal tissues of the fallopian tube (), it also suggests the possibility that elevated CHEK2 mRNA expression may be used as early diagnostic marker of high-grade serous ovarian cancer. The elevated expression of CHEK2 could possibly due to response to DNA damage or genome instability associated with TP53 mutations in HG-SOC. The potential of Chk2 inhibitors as therapeutics has been proposed due to evidence that the levels of Chk2 in some human tumor cells may be elevated.Citation53 Specifically, it has been reported that inhibition of Chk2 expression could promote apoptotic response in human kidney embryonic HEK-293, breast adenocarcinoma MCF-7, colon adenocarcinoma HCT116, prostate adenocarcinoma PC3, or human epithelial ovarian carcinoma Caov-4 and SKOV-3 cell lines in the presence of cytotoxic agents such camptothecin or cisplatin.Citation54-Citation56 The induction of apoptosis in tumor cells via inhibiting Chk2 could be beneficial in preventing uncontrollable cell proliferation, which consequently could lead to better patient survival. However, a recent study found that Chk2 depletion in ovarian cancer cell lines diminished platinum sensitivity and raised further suspicions if Chk2 could be an effective therapeutic target in platinum-treated HG-SOC patients.Citation57 Further new studies should be performed to address the inconsistencies of the effects of Chk2 depletion.
Conclusion
While there is a general consensus within the scientific community that CHEK2 mutations are unlikely to confer additional risk of ovarian cancer development, the effect on survival prognosis of patients already diagnosed with HG-SOC is less clear. Our results revealed that CHEK2 mutations in HG-SOC patients are strong adverse indicator of patient survival prognosis and associated with therapy resistance. We hypothesize that it could be due to mutations of the nuclear localization signal, which prevents the nuclear import of the protein and subsequently leads to haploinsufficiency. We also identified a 21-gene mutational prognostic signature, which highly correlates with patient’s survival patterns (P = 7.311e-08). Among these genes, protein functions such as kinase activity or ATP binding are enriched, which possibly indicate that these processes play crucial roles in carcinogenesis, and targeting these processes might be an attractive therapeutic strategy to restore the imbalance in dysregulated cell proliferation associated with the higher-risk subgroups. Also, we observed unique allelic changes in the genes of 21-gene mutational prognostic signature in prognosed high-risk HG-SOC subgroup, proposing that future analysis of such enriched allelic changes could be important in studying upstream mutation mechanisms, leading to poor patient prognosis in HG-SOC. Finally, we identified 2 novel sub-classes of HG-SOC, which are characterized via either germline mutations of CHEK2, RPS6KA2, and MLL4 or mostly somatic mutations of the other signature genes. The presence of a subset of tumors characterized via germline mutations or LOH of CHEK2 could guide future potential screening efforts to identify women with high-risk of developing HG-SOC.
Materials and Methods
TCGA HG-SOC data source and pre-processing
Processed mutation data belonging to 334 TCGA HG-SOC patients were downloaded from the TCGA data portal on 24th November 2010. The sequences were generated by Human Genome Sequencing Centers (HGSCs) at Baylor College of Medicine (BCM), Broad Institute Genome Center (BI), and Genome Institute at Washington University (WUSM) based on either Illumina or ABI SOLID sequencing technologies. We analyzed Level 2 data downloaded from the TCGA data portal, and this release included putative mutations for 105 171 and 88 patients from BCM, BI and WUSM, respectively (Fig. S1). In total, 21 978 mutations spanning across 334 patients and 10489 RefSeq gene symbols were reported. 4339 mutations with unknown mutation status were removed. The remaining 17 639 mutations were observed in 9083 genes and these mutations are of either germline, somatic, or loss-of-heterozygosity (LOH) origins (Table S1). The variant types of these mutations include deletion, insertion, SNP, or DNP. The mutation statistics are shown in Table S2. The clinical information corresponding to each HG-SOC patient was also downloaded (Table S9).
In addition, mRNA expression data of 463 primary solid ovarian cancer tissue samples were obtained (from 11 batches of 21–47 samples each). Quality assessments were performed within each batch to identify poor-quality chips. Seventy-four poor-quality chips were removed from subsequent analysis. Background correction and normalization were done within each batch. Finally, batch effects were eliminated across batches using the non-parametric ComBat software.Citation58
Copy number variation analysis
Three hundred and five (305) tumor-blood paired samples downloaded from TCGA portal have been used in this study. The blood copy number variations have been used for normalization and estimation of the fold change enrichment/under-representation of copy number variation data for matched tumor samples. TCGA SNP array data (CNV platform 6) were processed via PARTEK 6.5 program at the parameters recommended by the company. Using PARTEK software, we identified genomic coordinates of the copy variation segments, which form statistically significant deleted or amplified genome regions. For each tumor sample these significant regions were mapped on the human genome coordinates, and normalized fold change of such signals were visualized via USCS Genome browser custom tracks. Changed copy numbers in ovarian tumors exhibit a high level of chromosomal instability. 20 573 genes representing about 70% of RefSeq protein-coding genes were overlapped with significant altered copy number regions.
Processed RNA-sequencing expression data
Processed RNA-sequencing expression data of genes and gene isoforms were downloaded from the Sage Bionetworks’ Synapse database.Citation38 This data set contains RNA-Seq expression data for 73598 gene isoforms and 266 samples corresponding to 263 patients. Of the 266 samples, 262 samples (from 262 patients) were collected from primary solid tumor, whereas the rest were collected from recurrent solid tumor.
Secondary data source
Mutational data of 273 ovarian cancer patients analyzed via massively parallel sequencing were collected from Walsh et al.Citation9 Mutation data of breast cancer and glioblastoma of the TCGA patient cohorts were downloaded from the International Cancer Genome Consortium (accessed on 30th October 2012).Citation59 Wherever necessary, all genomic coordinates were converted to reference hg18 (NCBI36) using the Batch Coordinate Conversion (liftOver) utility provided by the UCSC Genome Bioinformatics Group.Citation60
Protein annotation data comprising important functional sites, secondary structure, natural variants, mutagenesis experimental data, and phosphorylation sites was obtained from UniProt.Citation33 Additionally, known phosphorylation sites were downloaded from validated database Phopho.ELM.Citation34 Phosphorylation sites were further predicted using online tools NetPhos and PHOSIDA, which were based on machine learning techniques such as artificial neural network or support vector machine.Citation36,Citation37 NLSs were predicted via online computational tools PSORT II and cNLS Mapper.Citation42,Citation43
Mutation matrix across patients and genes
The mutation spectrum across the patients and genes are represented in a 2-dimensional matrix, M, comprised of 9083 rows and 334 columns, which represent gene symbols and patient sample IDs, respectively. Each entry in the matrix, Mij represents the number of unique mutation sites in the ith gene of the jth patient sample. The matrix is shown in Table S3.
Analysis of the frequency distribution of the number of mutated tumor samples for a susceptible gene
The Kolmogorov–Waring (K–W) probability function is used to fit the distribution of the number of mutated tumor tissue samples.Citation24,Citation25 The function is described as:
(Eq1):
where m = 0,1,2,… and b,a and θ are parameters of our model. B(x) is the Beta function as previously described.Citation24,Citation25 In the case where b > a > 0, the probability of non-observed events is estimated by the formula:
Equation 1 can be presented in the form of the following recursive formula for easy computational estimate of the model parameters:
(Eq2):
In order to apply the probability function (Eq1) or (Eq2) to the observed data, we assume that the random variable X is restricted to sample size and the rarest events are non-observed. Thus, random variable X is doubly truncated, i.e., the range 1,2,…, J (J < ∞). Using (Eq1), the probability distribution function of the resulting truncated distribution function is written as the following:
(Eq3)
This probability distribution function corresponds to a typical situation in analysis of mutagenesis data in a limited cohort where the occurrence values 0 and J+1, J+2,… are not detected. Details of the curve-fitting computational algorithm has been previously published.Citation24
Hierarchical clustering
A numerical matrix that represents the mutation pattern across patients and genes are generated. Rows and columns correspond to genes and patients, respectively. Each numerical value in the matrix represents the number of distinct locations with reported mutations for that patient and gene. Hierarchical clustering analysis was performed using Kendall-Tau as the similarity metric and complete linkage as the clustering method. The mathematical procedure was implemented in Gene Cluster 3.0 and visualized via Java TreeView.Citation61,Citation62 The intensity of the plot corresponds with the number of distinct mutated locations for that patient and gene.
Gene enrichment and network analysis
Gene functional enrichment analysis was performed via DAVID Bioinformatics and MetaCore from GeneGo Inc.Citation63 The default human genome genes were used as the background set. Default parameters were used. The gene network was generated via MetaCore via direct interacting network algorithm. The legend of the network figure can be assessed from (http://ftp.genego.com/files/MC_legend.pdf).
Survival analysis
Survival analyses of patient subgroups were performed with reference to their overall survival times (years to last follow up) and survival event (vital status at last follow up). Comparative survival times and events of patient subgroups were visualized using Kaplan–Meier survival curves, which represent the probability of patient survival at a given time after initial diagnosis.Citation64 The statistical significance of patient subgroup stratification across the full survival time range was evaluated using the log-rank test, which is based on the chi-sq distribution. The procedures were implemented using open source R programming language and packages.
Measure of agreement test
The correlations between ordered patient subgroups with clinical parameters such as therapy response were calculated using weighted kappa correlation measure. The statistical significance was estimated using Mantel–Haenszel (MH) test. The calculations were implemented using StatXact-9 (computed weight: quadratic difference, scores: equally spaced). All P values are one-sided (right-tailed), which indicates the probability that a random kappa correlation measure is greater than actually observed.
Protein structure modeling
The initial structure was taken from the crystal structure of the serine/threonine protein kinase Chk2. (PDB code 3i6u,Citation39 resolved at 3.0 Å). The crystallographic unit contains a dimeric protein (chains A and B). The crystal construct comprises from residue Thr89 to Glu501. The program ModelerCitation40 has been used to complete few missing loops and to extend the C-terminal region of the kinase until Leu543, in order to include the nuclear localization signal motif. PDB2PQRCitation65 was used for protonation of residues. MD simulations were set up using the antechamber and LEaP modules in the AMBER 12 package.Citation66 The system was solvated in a truncated octahedron TIP3P water box and neutralized with sodium ions. Minimization and MD simulations using the amber ff99SB all-atom force field,Citation67 were performed with the Sander module of the Amber12 package using the GPU-accelerated version of the program.Citation68 We followed a multistep scheme as previously described.Citation69 We have extracted the conformation at 50 ns and assumed that along the trajectory the kinase and especially the C-termini tail have adopted a relaxed state. PyMOLCitation70 has been used to visualize and generate the figures.
Abbreviations: | ||
CNV | = | copy number variation |
EOC | = | epithelial ovarian cancer |
HG-SOC | = | high-grade serous ovarian cancer |
K-W | = | Kolmogorov-Waring function |
MD | = | molecular dynamics |
NLS | = | nuclear localization signal |
OC | = | Ovarian cancer |
OS | = | overall survival |
P | = | P value |
RefSeq | = | reference sequence |
SNP | = | single nucleotide polymorphism |
TCGA | = | The Cancer Genome Atlas |
WT | = | wild-type |
Additional material
Download Zip (5.5 MB)Disclosure of Potential Conflicts of Interest
No potential conflicts of interest were disclosed.
Acknowledgments
We acknowledge the TCGA Research Network for access to data. We thank Dr Kwoh Chee Keong, Dr Chandra Verma, and Prof Ted Hupp for useful discussions and suggestions. We also thank Dr Tang Zhiqun for assistance in the preprocessing of TCGA mRNA expression data.
The study was funded by Agency for Science, Technology and Research (A*STAR), Singapore. The funding agency had no role in study design, data collection and analysis, preparation of the manuscript or decision to publish.
Author Contributions
V.A.K. conceived and designed the study with input from other authors. A.V.I. performed clinical data interpretation and data analysis. G.F. analyzed the protein structure and performed molecular dynamics simulation. G.S.O. performed analysis and wrote the initial draft of the manuscript. All authors have read, edited and approved the final version of the manuscript. V.A.K. performed data analysis and overall supervision and is the guarantor of the study.
References
- Siegel R, Naishadham D, Jemal A. Cancer statistics, 2012. CA Cancer J Clin 2012; 62:10 - 29; http://dx.doi.org/10.3322/caac.20138; PMID: 22237781
- Cho KR, Shih IeM. Ovarian cancer. Annu Rev Pathol 2009; 4:287 - 313; http://dx.doi.org/10.1146/annurev.pathol.4.110807.092246; PMID: 18842102
- Tuma RS. Origin of ovarian cancer may have implications for screening. J Natl Cancer Inst 2010; 102:11 - 3; http://dx.doi.org/10.1093/jnci/djp495; PMID: 20023201
- Mankoo PK, Shen R, Schultz N, Levine DA, Sander C. Time to recurrence and survival in serous ovarian tumors predicted from integrated genomic profiles. PLoS One 2011; 6:e24709; http://dx.doi.org/10.1371/journal.pone.0024709; PMID: 22073136
- Cancer Genome Atlas Research Network. Integrated genomic analyses of ovarian carcinoma. Nature 2011; 474:609 - 15; http://dx.doi.org/10.1038/nature10166; PMID: 21720365
- Tang Z, Ow GS, Thiery JP, Ivshina AV, Kuznetsov VA. Meta-analysis of transcriptome reveals let-7b as an unfavorable prognostic biomarker and predicts molecular and clinical subclasses in high-grade serous ovarian carcinoma. Int J Cancer 2014; 134:306 - 18; PMID: 23825028
- Matias-Guiu X, Davidson B. Prognostic biomarkers in endometrial and ovarian carcinoma. Virchows Arch 2014; 464:315 - 31; http://dx.doi.org/10.1007/s00428-013-1509-y; PMID: 24504546
- Lawrence MS, Stojanov P, Mermel CH, Robinson JT, Garraway LA, Golub TR, Meyerson M, Gabriel SB, Lander ES, Getz G. Discovery and saturation analysis of cancer genes across 21 tumour types. Nature 2014; 505:495 - 501; http://dx.doi.org/10.1038/nature12912; PMID: 24390350
- Walsh T, Casadei S, Lee MK, Pennil CC, Nord AS, Thornton AM, Roeb W, Agnew KJ, Stray SM, Wickramanayake A, et al. Mutations in 12 genes for inherited ovarian, fallopian tube, and peritoneal carcinoma identified by massively parallel sequencing. Proc Natl Acad Sci U S A 2011; 108:18032 - 7; http://dx.doi.org/10.1073/pnas.1115052108; PMID: 22006311
- Hanahan D, Weinberg RA. Hallmarks of cancer: the next generation. Cell 2011; 144:646 - 74; http://dx.doi.org/10.1016/j.cell.2011.02.013; PMID: 21376230
- McGowan CH. Checking in on Cds1 (Chk2): A checkpoint kinase and tumor suppressor. Bioessays 2002; 24:502 - 11; http://dx.doi.org/10.1002/bies.10101; PMID: 12111733
- Baysal BE, DeLoia JA, Willett-Brozick JE, Goodman MT, Brady MF, Modugno F, Lynch HT, Conley YP, Watson P, Gallion HH. Analysis of CHEK2 gene for ovarian cancer susceptibility. Gynecol Oncol 2004; 95:62 - 9; http://dx.doi.org/10.1016/j.ygyno.2004.07.015; PMID: 15385111
- Szymanska-Pasternak J, Szymanska A, Medrek K, Imyanitov EN, Cybulski C, Gorski B, Magnowski P, Dziuba I, Gugala K, Debniak B, et al. CHEK2 variants predispose to benign, borderline and low-grade invasive ovarian tumors. Gynecol Oncol 2006; 102:429 - 31; http://dx.doi.org/10.1016/j.ygyno.2006.05.040; PMID: 16828850
- Krylova NY, Ponomariova DN, Sherina NY, Ogorodnikova NY, Logvinov DA, Porhanova NV, Lobeiko OS, Urmancheyeva AF, Maximov SY, Togo AV, et al. CHEK2 1100 delC mutation in Russian ovarian cancer patients. Hered Cancer Clin Pract 2007; 5:153 - 6; http://dx.doi.org/10.1186/1897-4287-5-3-153; PMID: 19725991
- Słojewski M, Złowocka E, Cybulski C, Górski B, Debniak T, Wokołorczyk D, Matyjasik J, Sikorski A, Lubiński J. CHEK2 germline mutations correlate with recurrence rate in patients with superficial bladder cancer. Ann Acad Med Stetin 2008; 54:115 - 21; PMID: 19839522
- Angelova SG, Krasteva ME, Gospodinova ZI, Georgieva EI. CHEK2 gene alterations independently increase the risk of death from breast cancer in Bulgarian patients. Neoplasma 2012; 59:622 - 30; http://dx.doi.org/10.4149/neo_2012_079; PMID: 22862163
- Schmidt MK, Tollenaar RA, de Kemp SR, Broeks A, Cornelisse CJ, Smit VT, Peterse JL, van Leeuwen FE, Van’t Veer LJ. Breast cancer survival and tumor characteristics in premenopausal women carrying the CHEK2*1100delC germline mutation. J Clin Oncol 2007; 25:64 - 9; http://dx.doi.org/10.1200/JCO.2006.06.3024; PMID: 17132695
- de Bock GH, Schutte M, Krol-Warmerdam EM, Seynaeve C, Blom J, Brekelmans CT, Meijers-Heijboer H, van Asperen CJ, Cornelisse CJ, Devilee P, et al. Tumour characteristics and prognosis of breast cancer patients carrying the germline CHEK2*1100delC variant. J Med Genet 2004; 41:731 - 5; http://dx.doi.org/10.1136/jmg.2004.019737; PMID: 15466005
- Simon M, Ludwig M, Fimmers R, Mahlberg R, Müller-Erkwoh A, Köster G, Schramm J. Variant of the CHEK2 gene as a prognostic marker in glioblastoma multiforme. Neurosurgery 2006; 59:1078 - 85, discussion 1085; PMID: 17016233
- Barillot E, Calzone L, Hupé P, Vert J, Zinovyev A. Computation Systems Biology of Cancer. London: CRC Press, 2012.
- Czech A, Fedyunin I, Zhang G, Ignatova Z. Silent mutations in sight: co-variations in tRNA abundance as a key to unravel consequences of silent mutations. Mol Biosyst 2010; 6:1767 - 72; http://dx.doi.org/10.1039/c004796c; PMID: 20617253
- Duan J, Wainwright MS, Comeron JM, Saitou N, Sanders AR, Gelernter J, Gejman PV. Synonymous mutations in the human dopamine receptor D2 (DRD2) affect mRNA stability and synthesis of the receptor. Hum Mol Genet 2003; 12:205 - 16; http://dx.doi.org/10.1093/hmg/ddg055; PMID: 12554675
- Kimchi-Sarfaty C, Oh JM, Kim IW, Sauna ZE, Calcagno AM, Ambudkar SV, Gottesman MM. A “silent” polymorphism in the MDR1 gene changes substrate specificity. Science 2007; 315:525 - 8; http://dx.doi.org/10.1126/science.1135308; PMID: 17185560
- Kuznetsov VA. Family of skewed distributions associated with the gene expression and proteome evolution. Signal Process 2003; 83:889 - 910; http://dx.doi.org/10.1016/S0165-1684(02)00481-4
- Kuznetsov VA. Hypergeometric Model of Evolution of Conserved Protein Coding Sequences in the Proteomes. Fluctuation and Noise Letters 2003; 3:L295 - 324; http://dx.doi.org/10.1142/S0219477503001397
- Alexandrov LB, Nik-Zainal S, Wedge DC, Campbell PJ, Stratton MR. Deciphering signatures of mutational processes operative in human cancer. Cell Rep 2013; 3:246 - 59; http://dx.doi.org/10.1016/j.celrep.2012.12.008; PMID: 23318258
- Lynch HT, Lynch PM, Lanspa SJ, Snyder CL, Lynch JF, Boland CR. Review of the Lynch syndrome: history, molecular genetics, screening, differential diagnosis, and medicolegal ramifications. Clin Genet 2009; 76:1 - 18; http://dx.doi.org/10.1111/j.1399-0004.2009.01230.x; PMID: 19659756
- Bashashati A, Ha G, Tone A, Ding J, Prentice LM, Roth A, Rosner J, Shumansky K, Kalloger S, Senz J, et al. Distinct evolutionary trajectories of primary high-grade serous ovarian cancers revealed through spatial mutational profiling. J Pathol 2013; 231:21 - 34; http://dx.doi.org/10.1002/path.4230; PMID: 23780408
- Bolton KL, Chenevix-Trench G, Goh C, Sadetzki S, Ramus SJ, Karlan BY, Lambrechts D, Despierre E, Barrowdale D, McGuffog L, et al, EMBRACE, kConFab Investigators, Cancer Genome Atlas Research Network. Association between BRCA1 and BRCA2 mutations and survival in women with invasive epithelial ovarian cancer. JAMA 2012; 307:382 - 90; http://dx.doi.org/10.1001/jama.2012.20; PMID: 22274685
- McLaughlin JR, Rosen B, Moody J, Pal T, Fan I, Shaw PA, Risch HA, Sellers TA, Sun P, Narod SA. Long-term ovarian cancer survival associated with mutation in BRCA1 or BRCA2. J Natl Cancer Inst 2013; 105:141 - 8; http://dx.doi.org/10.1093/jnci/djs494; PMID: 23257159
- Chrisanthar R, Knappskog S, Løkkevik E, Anker G, Østenstad B, Lundgren S, Berge EO, Risberg T, Mjaaland I, Maehle L, et al. CHEK2 mutations affecting kinase activity together with mutations in TP53 indicate a functional pathway associated with resistance to epirubicin in primary breast cancer. PLoS One 2008; 3:e3062; http://dx.doi.org/10.1371/journal.pone.0003062; PMID: 18725978
- Motakis E, Ivshina AV, Kuznetsov VA. Data-driven approach to predict survival of cancer patients: estimation of microarray genes’ prediction significance by Cox proportional hazard regression model. IEEE Eng Med Biol Mag 2009; 28:58 - 66; http://dx.doi.org/10.1109/MEMB.2009.932937; PMID: 19622426
- Magrane M, Consortium U. UniProt Knowledgebase: a hub of integrated protein data. Database (Oxford) 2011; 2011:bar009; http://dx.doi.org/10.1093/database/bar009; PMID: 21447597
- Dinkel H, Chica C, Via A, Gould CM, Jensen LJ, Gibson TJ, Diella F. Phospho.ELM: a database of phosphorylation sites--update 2011. Nucleic Acids Res 2011; 39:D261 - 7; http://dx.doi.org/10.1093/nar/gkq1104; PMID: 21062810
- Yoda A, Xu XZ, Onishi N, Toyoshima K, Fujimoto H, Kato N, Oishi I, Kondo T, Minami Y. Intrinsic kinase activity and SQ/TQ domain of Chk2 kinase as well as N-terminal domain of Wip1 phosphatase are required for regulation of Chk2 by Wip1. J Biol Chem 2006; 281:24847 - 62; http://dx.doi.org/10.1074/jbc.M600403200; PMID: 16798742
- Blom N, Gammeltoft S, Brunak S. Sequence and structure-based prediction of eukaryotic protein phosphorylation sites. J Mol Biol 1999; 294:1351 - 62; http://dx.doi.org/10.1006/jmbi.1999.3310; PMID: 10600390
- Gnad F, Ren S, Cox J, Olsen JV, Macek B, Oroshi M, Mann M. PHOSIDA (phosphorylation site database): management, structural and evolutionary investigation, and prediction of phosphosites. Genome Biol 2007; 8:R250; http://dx.doi.org/10.1186/gb-2007-8-11-r250; PMID: 18039369
- Weinstein JN, Collisson EA, Mills GB, Shaw KR, Ozenberger BA, Ellrott K, Shmulevich I, Sander C, Stuart JM, Cancer Genome Atlas Research Network. The Cancer Genome Atlas Pan-Cancer analysis project. Nat Genet 2013; 45:1113 - 20; http://dx.doi.org/10.1038/ng.2764; PMID: 24071849
- Cai Z, Chehab NH, Pavletich NP. Structure and activation mechanism of the CHK2 DNA damage checkpoint kinase. Mol Cell 2009; 35:818 - 29; http://dx.doi.org/10.1016/j.molcel.2009.09.007; PMID: 19782031
- Sali A, Blundell TL. Comparative protein modelling by satisfaction of spatial restraints. J Mol Biol 1993; 234:779 - 815; http://dx.doi.org/10.1006/jmbi.1993.1626; PMID: 8254673
- Zannini L, Lecis D, Lisanti S, Benetti R, Buscemi G, Schneider C, Delia D. Karyopherin-alpha2 protein interacts with Chk2 and contributes to its nuclear import. J Biol Chem 2003; 278:42346 - 51; http://dx.doi.org/10.1074/jbc.M303304200; PMID: 12909615
- Nakai K, Horton P. PSORT: a program for detecting sorting signals in proteins and predicting their subcellular localization. Trends Biochem Sci 1999; 24:34 - 6; http://dx.doi.org/10.1016/S0968-0004(98)01336-X; PMID: 10087920
- Kosugi S, Hasebe M, Tomita M, Yanagawa H. Systematic identification of cell cycle-dependent yeast nucleocytoplasmic shuttling proteins by prediction of composite motifs. Proc Natl Acad Sci U S A 2009; 106:10171 - 6; http://dx.doi.org/10.1073/pnas.0900604106; PMID: 19520826
- Zhang P, Gao W, Li H, Reed E, Chen F. Inducible degradation of checkpoint kinase 2 links to cisplatin-induced resistance in ovarian cancer cells. Biochem Biophys Res Commun 2005; 328:567 - 72; http://dx.doi.org/10.1016/j.bbrc.2005.01.007; PMID: 15694385
- He Z, Yang C, Guo G, Li N, Yu W. Motif-All: discovering all phosphorylation motifs. BMC Bioinformatics 2011; 12:Suppl 1 S22; http://dx.doi.org/10.1186/1471-2105-12-S1-S22; PMID: 21342552
- Amanchy R, Kandasamy K, Mathivanan S, Periaswamy B, Reddy R, Yoon WH, Joore J, Beer MA, Cope L, Pandey A. Identification of Novel Phosphorylation Motifs Through an Integrative Computational and Experimental Analysis of the Human Phosphoproteome. J Proteomics Bioinform 2011; 4:22 - 35; http://dx.doi.org/10.4172/jpb.1000163; PMID: 21720494
- Lahiry P, Torkamani A, Schork NJ, Hegele RA. Kinase mutations in human disease: interpreting genotype-phenotype relationships. Nat Rev Genet 2010; 11:60 - 74; http://dx.doi.org/10.1038/nrg2707; PMID: 20019687
- Tsatsanis C, Spandidos DA. The role of oncogenic kinases in human cancer (Review). [Review] Int J Mol Med 2000; 5:583 - 90; PMID: 10812005
- Dancey J, Sausville EA. Issues and progress with protein kinase inhibitors for cancer treatment. Nat Rev Drug Discov 2003; 2:296 - 313; http://dx.doi.org/10.1038/nrd1066; PMID: 12669029
- Pearson MA, Fabbro D. Targeting protein kinases in cancer therapy: a success?. Expert Rev Anticancer Ther 2004; 4:1113 - 24; http://dx.doi.org/10.1586/14737140.4.6.1113; PMID: 15606337
- Hofree M, Shen JP, Carter H, Gross A, Ideker T. Network-based stratification of tumor mutations. Nat Methods 2013; 10:1108 - 15; http://dx.doi.org/10.1038/nmeth.2651; PMID: 24037242
- Williams LH, Choong D, Johnson SA, Campbell IG. Genetic and epigenetic analysis of CHEK2 in sporadic breast, colon, and ovarian cancers. Clin Cancer Res 2006; 12:6967 - 72; http://dx.doi.org/10.1158/1078-0432.CCR-06-1770; PMID: 17145815
- Jobson AG, Lountos GT, Lorenzi PL, Llamas J, Connelly J, Cerna D, Tropea JE, Onda A, Zoppoli G, Kondapaka S, et al. Cellular inhibition of checkpoint kinase 2 (Chk2) and potentiation of camptothecins and radiation by the novel Chk2 inhibitor PV1019 [7-nitro-1H-indole-2-carboxylic acid 4-[1-(guanidinohydrazone)-ethyl]-phenyl-amide]. J Pharmacol Exp Ther 2009; 331:816 - 26; http://dx.doi.org/10.1124/jpet.109.154997; PMID: 19741151
- Yu Q, Rose JH, Zhang H, Pommier Y. Antisense inhibition of Chk2/hCds1 expression attenuates DNA damage-induced S and G2 checkpoints and enhances apoptotic activity in HEK-293 cells. FEBS Lett 2001; 505:7 - 12; http://dx.doi.org/10.1016/S0014-5793(01)02756-9; PMID: 11557032
- Vakifahmetoglu H, Olsson M, Tamm C, Heidari N, Orrenius S, Zhivotovsky B. DNA damage induces two distinct modes of cell death in ovarian carcinomas. Cell Death Differ 2008; 15:555 - 66; http://dx.doi.org/10.1038/sj.cdd.4402286; PMID: 18064041
- Ghosh JC, Dohi T, Raskett CM, Kowalik TF, Altieri DC. Activated checkpoint kinase 2 provides a survival signal for tumor cells. Cancer Res 2006; 66:11576 - 9; http://dx.doi.org/10.1158/0008-5472.CAN-06-3095; PMID: 17178848
- Alkema NG, Tomar T, van der Zee AG, Everts M, Meersma GJ, Hollema H, de Jong S, van Vugt MA, Wisman GB. Checkpoint kinase 2 (Chk2) supports sensitivity to platinum-based treatment in high grade serous ovarian cancer. Gynecol Oncol 2014; http://dx.doi.org/10.1016/j.ygyno.2014.03.557; PMID: 24657486
- Johnson WE, Li C, Rabinovic A. Adjusting batch effects in microarray expression data using empirical Bayes methods. Biostatistics 2007; 8:118 - 27; http://dx.doi.org/10.1093/biostatistics/kxj037; PMID: 16632515
- Hudson TJ, Anderson W, Artez A, Barker AD, Bell C, Bernabé RR, Bhan MK, Calvo F, Eerola I, Gerhard DS, et al, International Cancer Genome Consortium. International network of cancer genome projects. Nature 2010; 464:993 - 8; http://dx.doi.org/10.1038/nature08987; PMID: 20393554
- Karolchik D, Hinrichs AS, Kent WJ. The UCSC Genome Browser. Curr Protoc Bioinformatics 2012; Chapter 1:Unit1 4.
- de Hoon MJ, Imoto S, Nolan J, Miyano S. Open source clustering software. Bioinformatics 2004; 20:1453 - 4; http://dx.doi.org/10.1093/bioinformatics/bth078; PMID: 14871861
- Eisen MB, Spellman PT, Brown PO, Botstein D. Cluster analysis and display of genome-wide expression patterns. Proc Natl Acad Sci U S A 1998; 95:14863 - 8; http://dx.doi.org/10.1073/pnas.95.25.14863; PMID: 9843981
- Huang W, Sherman BT, Lempicki RA. Systematic and integrative analysis of large gene lists using DAVID bioinformatics resources. Nat Protoc 2009; 4:44 - 57; http://dx.doi.org/10.1038/nprot.2008.211; PMID: 19131956
- Rich JT, Neely JG, Paniello RC, Voelker CC, Nussenbaum B, Wang EW. A practical guide to understanding Kaplan–Meier curves. Otolaryngol Head Neck Surg 2010; 143:331 - 6; http://dx.doi.org/10.1016/j.otohns.2010.05.007; PMID: 20723767
- Dolinsky TJ, Czodrowski P, Li H, Nielsen JE, Jensen JH, Klebe G, Baker NA. PDB2PQR: expanding and upgrading automated preparation of biomolecular structures for molecular simulations. Nucleic Acids Res 2007; 35:W522-5; http://dx.doi.org/10.1093/nar/gkm276; PMID: 17488841
- Case DA, Darden TA. T.E. Cheatham I, Simmerling CL, Wang J, Duke RE, et al. AMBER 12. University of California, San Francisco., 2012.
- Hornak V, Abel R, Okur A, Strockbine B, Roitberg A, Simmerling C. Comparison of multiple Amber force fields and development of improved protein backbone parameters. Proteins 2006; 65:712 - 25; http://dx.doi.org/10.1002/prot.21123; PMID: 16981200
- Götz AW, Williamson MJ, Xu D, Poole D, Le Grand S, Walker RC. Routine Microsecond Molecular Dynamics Simulations with AMBER on GPUs. 1. Generalized Born. J Chem Theory Comput 2012; 8:1542 - 55; http://dx.doi.org/10.1021/ct200909j; PMID: 22582031
- Pereira M, Verma CS, Fuentes G. Differences in the binding affinities of ErbB family: heterogeneity in the prediction of resistance mutants. PLoS One 2013; 8:e77054; http://dx.doi.org/10.1371/journal.pone.0077054; PMID: 24194858
- Schrodinger, LLC. The PyMOL Molecular Graphics System, Version 1.3r1. 2010