Abstract
In breast cancer, inactivation of the RB tumor suppressor gene is believed to occur via multiple mechanisms to facilitate tumorigenesis. However, the prognostic and predictive value of RB status in disease-specific clinical outcomes has remained uncertain. We investigated RB pathway deregulation in the context of both ER-positive and ER-negative disease using combined microarray datasets encompassing over 900 breast cancer patient samples. Disease-specific characteristics of RB pathway deregulation were investigated in this dataset by evaluating correlation among pathway genes as well as differential expression across patient tumor populations defined by ER status. Survival analysis among these breast cancer samples demonstrates that the RB-loss signature is associated with poor disease outcome within several independent cohorts. Within the ER-negative subpopulation, the RB-loss signature is associated with improved response to chemotherapy and longer relapse-free survival. Additionally, while individual genes in the RB target signature closely reproduce its prognostic value, they also serve to predict and monitor response to therapeutic compounds, such as the cytostatic agent PD-0332991. These results indicate that the RB-loss signature expression is associated with poor outcome in breast cancer, but predicts improved response to chemotherapy based on data in ER-negative populations. While the RB-loss signature, as a whole, demonstrates prognostic and predictive utility, a small subset of markers could be sufficient to stratify patients based on RB function and inform the selection of appropriate therapeutic regimens.
Introduction
Breast cancer is a major health concern, wherein approximately one in eight women will be diagnosed with the disease in their lifetime.Citation1 Unlike the majority of cancers, the prognosis and treatment of breast cancer is significantly informed by biomarkers.Citation2,Citation3 For example, estrogen receptor alpha (ER)-positive breast cancer, which makes up approximately 70% of cases, has a generally more favorable prognosis and can be successfully treated with endocrine therapy.Citation2 In contrast, ER-negative cancers are generally more aggressive and treatment options are more limited.Citation3,Citation4 The use of biomarkers for patient stratification has been immensely helpful in directing appropriate therapy to millions of women. Unfortunately, significant shortcomings remain in the capacity to optimally target therapeutic interventions. For example, although suppression of ER activity is the goal for both aromatase inhibitors (e.g., letrazole) and selective ER antagonists (e.g., tamoxifen), a significant number of ER-positive tumors will fail primary endocrine therapy.Citation2 As such, significant effort has been directed at defining those pathways which contribute to the resistance to endocrine therapy and means to identify and treat such patients more effectively.
Extensive analyses have demonstrated that a multitude of oncogenic lesions observed in breast cancer can contribute to the bypass of endocrine therapy in ER-positive disease.Citation2 For example, deregulation of ERBB2, EGFR, PTEN/AKT and MAPK/ERK pathways can all lead to the bypass of endocrine therapy in preclinical models.Citation5–Citation8 Correspondingly, such lesions in mitogenic signaling are associated with poor disease outcome when analyzed directly in clinical specimens. Importantly, mitogenic signaling pathways generally coalesce to impact the cell cycle machinery, and thus influence both the proliferative state of tumors and the cytostatic impact of endocrine therapy.Citation2 Specifically, such pathways have been shown to converge on the retinoblastoma tumor suppressor (RB) and related proteins that regulate the G1-S cell cycle transition.
The retinoblastoma tumor suppressor (RB) was first defined based on biallelic inactivation in retinoblastoma, but is inactivated in a host of solid adult cancers by multiple distinct mechanisms.Citation9,Citation10 In normal quiescent tissues, RB is active and can directly mediate the repression of a well-defined program of genes that are regulated by the E2F-family of transcription factors.Citation9,Citation11 Mitogenic or oncogenic signaling molecules impinge on RB by inducing the expression of D-type cyclins and consequently increasing CDK activity.Citation12,Citation13 These kinase complexes phosphorylate the RB protein, and thereby relieve RB-mediated transcriptional repression.Citation14 As a consequence, the expression of genes involved in DNA-replication, mitosis and cytokinesis are induced.Citation15 Thus, RB plays a critical role in coordinating the expression of genes that are required for cell cycle progression and cellular proliferation.
In breast cancer, the RB-pathway is believed to be inactivated via several mutually exclusive mechanisms. Foremost, overexpression or amplification of the cyclin D1 gene (CCND1) is observed in as many as 50% of breast cancers, wherein it is believed to drive aberrant phosphorylation/inactivation of RB protein.Citation16 Similarly, inactivation of the CDK-inhibitor p16ink4a (CDKN2A) contributes to the deregulation of RB phosphorylation and is silenced in a fraction of breast cancer cases.Citation17–Citation19 Lastly, loss of heterozygosity at the RB gene (RB1) locus has been defined in 20–30% of breast cancer and histological loss of RB protein has been documented with varying frequency.Citation10,Citation20 In spite of these analyses, studies have yet to generate a consistent portrait of the impact of RB-pathway dysregulation in breast cancer.
Results
To define the impact of RB-pathway dysregulation in breast cancer, the behavior and impact of the gene expression program coordinated by RB was investigated in a large and diverse cohort of breast cancer cases (Sup. Table 1). The RB-signature consists of 159 genes (Sup. Table 2) that were identified in common among at least two of the following three model systems: upregulated by RB deletion in fibroblastic models,Citation15 repressed by the activation of RB,Citation21 or upregulated with acute RB deletion in the murine liver (Bourgo et al. in preparation). This gene expression program is highly consistent with E2F-regulated gene sets, including factors involved in DNA replication, mitosis and cytokinesis.
To analyze the regulation and impact of this gene expression program in the context of breast cancer, a cohort of 2,254 breast cancer cases was compiled from public microarray databases. Of these, 1,740 were identified as ER-positive and 514 were identified as ER-negative. Relapse-free survival data was available for 936 unique samples, of which 790 were identified as ER-positive and 146 were identified as ER-negative. Analyses of the RB-loss signature among these samples produced three important findings. First, ER-negative tumors generally exhibited the highest expression values within the RB-loss signature (Sup. Fig. 1). These findings suggest that the most profound disruption of RB functions is occurring in ER-negative disease, which is consistent with studies demonstrating that histological RB loss is considerably more prevalent in ER-negative tumors.Citation19,Citation22,Citation23 Second, a majority of RB-loss signature genes in the human datasets are positively correlated. This finding indicates that the RB-loss signature as defined in simple model systems is largely maintained in the context of human tumor specimens. Third, these analyses revealed that the RB-loss signature expression in tumor samples is elevated relative to normal controls. However, there exists significant heterogeneity in both ER-positive and ER-negative disease, wherein a fraction of tumor samples exhibit levels consistent with normal tissue. Combined, these findings provided substantial opportunity to define those molecular factors and clinical outcomes that are specifically associated with deregulation of the RB-gene expression program.
Initially, we utilized gene expression data to determine how the RB target signature related to the expression level of primary members of the RB-pathway (e.g., RB1, CCND1 and CDKN2A), which would be expected to directly contribute to pathological deregulation of gene expression in breast cancer. Correlation between these pathway members was initially compared in simplified subsets of all normal breast samples and all tumor samples ( and B). In this context, moderate correlation between the RB-signature and the RB1 and CDKN2A genes was observed in both healthy and tumor samples, whereas moderate correlation was seen with CCND1 in healthy samples but not in tumor samples. To determine if this paradoxical association was a manifestation of the heterogeneity in breast cancer specimens, we analyzed these relationships in ER-positive and ER-negative breast cancer subsets (). Results indicate that CCND1 transcript, but not CDKN2A, is modestly correlated with the RB-signature in ER-positive disease (). Differential expression analyses revealed that in ER-positive breast cancer, CCND1 levels were generally higher than in ER-negative disease (), but the opposite is seen for CDKN2A (). The levels of RB transcript generally changed little between the tumors (), although in ER-positive disease RB transcript actually correlated with an elevated RB-loss signature. This finding is generally consistent with the concept that RB is only infrequently lost in ER-positive breast cancer, and that the transcript is positively regulated with deregulation of E2F activity.Citation24 In contrast, in ER-negative cancers there was no correlation between CCND1 or RB1 transcripts with the RB-loss signature, rather elevated CDKN2A transcript correlated with elevated RB-loss signature (). This finding suggests that ER-negative tumors harbor genetic lesions that enable the deregulation of RB-mediated transcription in the presence of high CDKN2A expression. Such a finding is consistent with histological assessment, wherein RB-negative tumors exhibit elevated p16ink4a expression.Citation17
To determine if the association between core RB pathway transcripts and distinct forms of breast cancer were representative of altered protein expression, the protein levels of RB, cyclin D1 and p16ink4a were initially evaluated in cell culture model systems (). These data revealed that high levels of p16ink4a were associated with undetectable levels of RB protein. Protein analysis was also performed on an independent tumor collection comprising 120 human breast cancer samples. Specifically, fluorescence-based AQUA immunostaining was employed to quantitatively determine the levels of Ki67, Cyclin D1, p16ink4a and RB protein in breast cancer specimens. AQUA immunohistochemical staining was reviewed for representative tumor cases () and protein levels were compared among normal breast, all tumors and ER-positive and ER-negative subgroups (). These data revealed that the relationships observed at the RNA level were, in fact, recapitulated by alterations in protein expression in clinical samples.
Functional enrichment analysis was used to identify biological functions that are coregulated with RB target genes by evaluating sets of genes that strongly correlated either positively or negatively with the RB-loss signature (Sup. Tables 3 and 4). Biological processes that were negatively correlated with the RB-loss signature included cell communication and signal transduction. Terms associated with positively correlated genes revealed proliferation as a dominant theme, including the GO biological processes for cell division and DNA replication. In addition to the RB-loss signature, there are a number of gene signatures that are associated with proliferation and poor prognosis in cancer. These gene sets that have been employed in previous breast cancer studies include the “proliferation signature” and “genomic grade index.” Interestingly, although each set is highly enriched for cell cycle regulated genes, only 32 genes are in common between the three gene lists (). This finding indicates that distinct experimental approaches define functionally similar yet largely unique sets of genes. In spite of this finding, the average gene expression signatures were virtually identical across normal breast tissue and breast cancer cases irrespective of ER-status (). Thus, although the specific genes are largely disparate and the proximal mechanism of cell cycle deregulation is most likely distinct in ER-positive and ER-negative breast cancers, there is extensive commonality in proliferative programs engaged.
To determine the pathogenic impact of RB, the association of the RB-loss signature with disease outcome was evaluated. Across our entire cohort of tumor specimens, deregulation of the RB pathway evident in high RB-loss signature expression was associated with poor disease prognosis (). Analyses of ER-positive versus ER-negative cases revealed a strikingly discordant impact of RB-pathway deregulation. In ER-positive disease there was a highly significant association between high RB-loss signature expression and poor disease outcome (), while in ER-negative disease the opposite trend was evident ( and D). These findings demonstrate that RB-pathway deregulation is not universally associated with poor disease outcome, and is potentially modified within disease sub-types.
Given the apparent differences in RB-pathway behavior in ER-positive versus ER-negative disease, these disparities could be reflective of distinct treatment regimens used among these subpopulations. Among the ER-positive patients, the relationship between RB-loss signature regulation and response to surgery alone or surgery with adjuvant tamoxifen therapy was evaluated. Among ER-positive patients treated with surgery in the absence of adjuvant therapy, the RB-loss signature was prognostic of unfavorable disease outcome (). This finding points towards greater metastatic potential in tumors with RB loss, and is supported by evidence that clinical tumor samples exhibiting overexpression of the RB-signature in ER-positive cases were significantly associated with distant metastasis events (p = 8.6 × 10−4). Analyses of ER-positive patients treated with tamoxifen revealed that the high RB-loss signature expression among these cases was associated with poor response to therapy, while patients with low RB-loss signature expression generally had good prognosis if treated with tamoxifen (). Among several independent patient cohorts, survival analysis of the surgery-only patient groups and groups receiving adjuvant tamoxifen therapy demonstrate prognosis that is inversely associated with the RB-loss signature magnitude (Sup. Fig. 2). These findings indicate that RB function is a general indicator of high-risk aggressive ER-positive breast cancer with a propensity for post-surgical recurrence.
The impact of high RB-loss signature in ER-negative cases was subsequently explored among patients with known chemotherapy treatment status. Survival analyses performed among ER-negative chemotherapy treatment populations do not include the low RB expression signature group due to an insufficient number of samples with known chemotherapy status for this group (which include only three recurrence events). The survival curves with respect to chemotherapy in ER-negative disease are therefore limited to samples with high RB-loss signature. In general, high RB-loss signature expression among ER-negative samples appears to have a better outcome than high RB-loss signature expression in the ER-positive samples (). However, once patients receiving chemotherapy are removed from the comparison, the ER-negative patients with high RB-loss signature expression have a 50% survival rate at five years, similar to the ER-positive population (). Additionally, there is little apparent difference between the survival profiles for low versus high RB-loss signature expression in the ER-negative patients receiving no chemotherapy (). While the number of ER-negative cases with known chemotherapy treatment is limited and provides low power in this dataset, there is a notable improvement in relapse-free survival when high RB expression patients are treated with the chemotherapy regimen of doxorubicin and cyclophosphamide (AC) (). To expand on this finding, we evaluated the response to chemotherapy for high versus low RB-loss signature in additional samples using pathological response as measure of disease outcome. In a patient group receiving doxorubicin in combination with fluorouracil and cyclophosphamide (FAC), the percentage of patients with pathological complete response (pCR) was found to increase in the high RB-loss signature group for both ER-positive and ER-negative disease ( and E). In ER-negative patients, a majority of those with high RB-loss signature expression experienced pCR, whereas progressive disease (PD) occurred in a majority of the low RB-loss signature expression group. This result supports the concept that high RB-loss signature expression is associated with better outcome in response to chemotherapy. In an ER-negative patient cohort receiving cisplatin treatment, however, the response in the high RB-loss signature group was heterogeneous while all patients in the low RB-loss signature group achieved pCR (). Consistency between the findings from the AC and FAC-treated cohorts indicate that while the high degree of proliferation associated with RB loss is associated with poor outcome in the ER-positive cases, the high RB-loss signature in ER-negative cases is associated with response to a subset of chemotherapy regimens.
Since the findings herein suggest that regulation of gene expression via the RB pathway could be important for cytostatic therapeutic responses, the efficacy of the CDK4/6 inhibitor PD-0332991 was evaluated in the context of breast cancer cell lines. This agent acts through the RB-pathway and has been postulated to have efficacy in the context of breast cancer specimens. In keeping with these findings, PD-0332991 treatment had a profound cytostatic effect on MCF-7 and MD-MB-231 cells, but not BT-549 (). In those instances, wherein PD-0332991 was effective at growth suppression there was a significant attenuation of gene products associated with RB-signature. For example, EZH2, Thymidylate Synthetase and Topo IIα were all suppressed in the context of PD-0332991 in cell lines retaining functional RB (). Thus, not only could the steady state levels of such markers be important for predicting therapeutic response, but could be employed as bio-monitors for therapeutic response. The potential of these individual genes in the RB-signature to predict disease outcomes was also evaluated in microarray dataset (). Interestingly, the expression profiles for EZH2, TYMS and TOP2A are effective at predicting disease outcome. Additionally, several prototypical genes such as MKI67, CCNB1, TOP2A, BIRC5 and FOXM1 demonstrated significant prognostic value among ER-positive samples, and were statistically significant across multiple independent patient populations (Sup. Figs. 3 and 4). Thus, a small subset of genes can provide highly significant predictive power as surrogates for RB/E2F pathway deregulation in ER-positive breast cancers.
Discussion
The RB pathway is frequently disrupted in cancer, where it is believed to play an inherent role in disease progression.Citation20 However, the predictive and prognostic significance of RB function in relation to breast cancer management has remained obscure. Here we demonstrate that there is intrinsic heterogeneity in RB-pathway regulation in breast cancer, and this heterogeneity is both associated with significant molecular aberrations and overall alterations in disease pathogenesis.
Prior studies have interrogated lesions in the RB-pathway by histological analyses. These studies have given rise to highly discordant data regarding the frequency of RB alterations in breast cancer and the relative impact on disease outcome. Here we took advantage of gene expression profiling approaches to investigate the down-stream influence of RB-pathway deregulation. Model systems of RB loss or E2F deregulation have given rise to a highly reproducible spectrum of genes that are downstream from RB.Citation10,Citation15,Citation21,Citation25–Citation27 The expression profiles of these target genes are highly correlated in breast cancer cases irrespective of disease sub-type. However, the magnitude of RB target gene deregulation varies among breast cancer sub-types. Specifically, in ER-negative breast cancer cases, the maximal deregulation of RB target gene expression is observed. This finding suggests that direct aberrations in RB function are occurring in this form of breast cancer. Interestingly, in ER-negative disease there is an upregulation of CDKN2A that correlates with the RB-signature. CDKN2A/p16ink4a is upregulated in concert with complete inactivation of the RB gene as occurs either through gene deletion or silencing with viral oncoproteins.Citation17,Citation28,Citation29 Thus, these combined findings suggest that in ER-negative disease the mechanisms underlying compromised RB function are analogous to RB gene deletion. Surprisingly, while LOH and histological loss of RB are frequently observed in ER-negative disease,Citation22,Citation30 there was only a marginal decrease of RB transcript expression in these tumors. This finding suggests that either RB dysregulation is due to mutations or to post-translational mechanisms that are not interrogated in our data sets. In contrast with the observations in ER-negative disease, the molecular underpinnings of RB-deregulation in ER-positive disease are more consistent with the aberrant mitogenic signaling. Specifically, there is a positive relationship with cyclin D1, but not CDKN2A. Correspondingly, histological loss of RB is relatively uncommon in ER-positive disease.Citation22 By investigating positively correlated/anti-correlated genes we sought to define pathways that could be driving RB-inactivation in ER-positive disease. Interestingly, the only positively correlating genes were additional proliferation associated genes, whilst the negatively correlated genes were in categories of cell communication and signal transduction. While it remains unclear what drives the functional inactivation of RB in the disease state, the associated consequences of increased proliferation and decreased signal transduction are hallmarks of cancer progression.
In general, it has been hypothesized that deregulation of RB pathway function would be associated with fast growing tumors that would have an intrinsically poor prognosis. Surprisingly, this is not uniformly the case. In ER-negative disease there was little impact of RB-functional status on disease outcome in patients receiving no chemotherapy. In ER-negative patients receiving chemotherapy, however, the loss of RB-functional status was associated with longer relapse-free survival, consistent with previous findings that RB loss can increase sensitivity to conventional chemotherapy that is widely utilized in the treatment of ER-negative disease.Citation10,Citation20,Citation22,Citation23,Citation30–Citation32 In ER-positive disease disruption of the RB-pathway was associated with a poor disease outcome in all patients. This was reflective of a particularly poor prognosis in the context of solely surgical intervention. Presumably, such an effect on survival could be due to the presence of micrometastasis that escape surgical interventions. In keeping with this concept, RB-deficiency was associated with a significantly increased risk for metastasis in the patient samples analyzed. Since most ER-positive disease is now treated with endocrine therapy, we evaluated how RB function associated with durable therapeutic response. These findings indicated that RB-pathway dysregulation is strongly associated with poor response to tamoxifen therapy, though patients with high RB-loss expression appear to benefit from tamoxifen therapy with 66% ten-year survival compared to 52% ten-year survival with surgery alone (p = 0.064). Even so, those tumors harboring such dysregulation could benefit from more aggressive therapeutic interventions. Of course, such rational decision making would be dependent on the ability to prospectively monitor RB-pathway deregulation. Specifically, in cases with high RB-loss signature where functional RB protein is retained (as might be expected for ER-positive breast cancers), compounds such as PD-0332991 may prove to be an effective intervention. In breast cancer cell lines treated with PD-0332991, reduced expression of RB targets is associated with the compound's cytostatic effect, demonstrating that the RB-loss signature may serve as a marker for therapeutic response.Citation33 These results demonstrate that while the RB-loss signature expression is associated with poor outcome in breast cancer, it denotes a favorable response to chemotherapy and may provide readout of the response to cytostatic treatment. An important caveat for the RB-loss signature to be applied in a clinical setting is that a small subset of RB target genes would provide a more practical clinical assay. As shown here, single genes harbored relatively strong predictive power which suggests that utilizing a relatively small panel of markers could be sufficient to stratify patients based on the RB-pathway and inform therapeutic utilization.
Together, these findings underscore the importance of the RB-pathway in the pathogenesis of breast cancer and as a critical determinant of therapeutic response. Furthermore, the strong predictive power of single gene profiles in the RB-loss signature suggests that predictive tests based on a small panel of RB pathway genes could be of high-significance in directing the care of breast cancer with both current and developing therapeutic strategies.
Materials and Methods
Microarray dataset selection and normalization.
Breast cancer microarray datasets with clinical disease-free survival data were downloaded from the Gene Expression Omnibus (GEO, www.ncbi.nlm.nih.gov/geo/)Citation34 and ArrayExpress (www.ebi.ac.uk/microarray-as/aer/).Citation35 A complete list of publically available microarray datasets and samples used for analysis is provided in Supplemental Table 1. Microarray sample CEL files from the Affymetrix U133A, U133Av2, U133plus2 and U133plus2HT GeneChips normalized in Matlab version 2009b (The MathWorks, Natick, MA) using the procedures applied inCitation36 and were merged into a combined dataset using the 22,768 probe sets in common among all of these platforms. The normalization process used is a modified Robust Multichip Average (RMA), which applies background adjustment to each CEL file and then applies quantile normalization and summarization using precomputed quantiles and row effects, respectively, from a large training set.Citation37,Citation38 Annotations for the 22,768 probesets in the normalized dataset were obtained from the HGU133A annotations file version na29, dated July 6th, 2009, downloaded from the Affymetrix website (www.affymetrix.com/). 13,004 unique genes were identified among the 22,768 probesets, based on gene symbol. Multiple probesets that mapped to a common gene were consolidated by averaging their rows together and scaling by the probeset with the largest standard deviation.
Predicting ER status.
Clinical ER status was available from 68% of the samples used for survival analysis in our combined microarray dataset. We evaluated transcript expression for the ER gene (ESR1) as a surrogate for clinical ER status in order to maximize the number of usable samples in our dataset. High correlation between ESR1 transcript levels and clinical status observed through immunohistochemical staining has been demonstrated previously.Citation39 We observed that the ESR1 transcript levels had a bimodal expression in the breast cancer datasets and fit a two-component normal mixture to the data and identified a high/low expression cutoff at the RMA expression value of 7.5. Samples were classified into ER-positive and ER-negative based on this expression cutoff, and predicted ER status was verified against the available clinical data, which demonstrated 91% accuracy. The predicted ER status based on ESR1 transcript expression threshold was used to represent ER status for the complete dataset.
The RB-loss signature.
The RB-loss signature was defined using genes that appeared in any two out of the following three gene sets identified in model systems: genes upreregulated by RB deletion in fibrobablastic models,Citation15 genes repressed by the activation of RB,Citation21 or genes upregulated with acute RB deletion in the murine liver (Bourgo et al. in preparation). The resulting RB-loss signature comprising 159 genes was mapped to the HGU133 platform by gene symbol, with 138 genes represented among the 22,768 common probesets on the array. The average RB-loss signature magnitude was computed by median centering expression profiles for each gene and then averaging over all 138 genes in the signature.
Clustering and correlation analysis.
The Pearson correlation between the average RB-loss signature and each gene on the HGU133 array was computed across all breast tissue samples and within healthy and tumor subsets. The Pearson correlation coefficient, R, was used to define four gene sets: genes with R ≥ 0.75 in tumor, genes with R ≥ −0.5 in tumor, genes with R ≥ 0.75 in healthy breast and genes with R ≤ −0.5 in healthy breast. Each of these gene sets was evaluated for overrepresented Gene Ontology (GO)Citation40 biological processes using the online Database for Annotation, Visualization and Integrated Discovery (DAVID).Citation41
Cell culture and PD-0332991 treatment.
All cell lines used in this study were obtained from the American Type Culture Collection (ATCC, Manassas, VA). MCF10A cells were cultured in DMEM/F12 media with 5% horse serum, supplemented with 20 ng/mL EGF, 0.5 mg/mL hydrocortisone, 10 µg/mL insulin, 100 U/mL penicillin/streptomycin and 2 mM L-glutamine. BT-549 cells were propagated in RPMI 1640 containing 10% fetal bovine serum, 100 U/mL penicillin/streptomycin and 2 mM L-glutamine. All other breast cancer cells lines were maintained in DMEM containing 10% fetal bovine serum, 100 U/mL penicillin/streptomycin and 2 mM L-glutamine. All cells were cultured at 37°C and 5% CO2. All cells were counted for experimental seeding using trypan blue exclusion. For all treatment experiments, cells were seeded at a density of 5 × 105 into 10 cm dishes and allowed to adhere overnight. PD-0332991 treatments were performed at 500 nM concentrations, reconstituted in DMSO, for 24 hours. Equal volumes of DMSO were used as treatment controls.
BrdU incorporation and FLOW cytometric analysis.
Cells were pulse-labeled with BrdU for one-hour prior to harvest. Cells were harvested and fixed in 70% EtOH overnight at 4°C. Cells were prepared for flow cytometry as previously described.Citation42 BrdU data is represented as a percentage of total population. All experiments were performed in triplicate from a minimum of two independent experiments.
Immunoblot analysis.
Cell lysates were resolved by SDS-PAGE and transferred to Immobilon-P membranes. Antibodies were used to detect the following proteins: Lamin B (Santa Cruz; M-20), Cyclin A (Santa Cruz; H-432), p16ink4a (Santa Cruz; H-156), Beta-tubulin (Santa Cruz; D-10), topoisomerase II-alpha (Santa Cruz; S-18), RB (Becton-Dickson; G3-245), Cyclin D1 (Neomarkers; Ab-3); EZH2 (Invitrogen; 49–1,043), Thymidylate Synthase (Abcam; TS-106).
RB protein expression.
A separate 180-sample breast cancer dataset was used to evaluate the expression of RB pathway proteins in breast cancer versus healthy tissue. This 180-sample dataset contains 40 healthy, 20 DCIS, 100 IDC and 20 metastatic samples.Citation43 Immunohistochemical labels were used to stain for proteins including ER (Dako; 1D5), p16 (mtm laboratories; E6H4™), cyclin-D1a (NeoMarkers; Ab-3), Ki67 (Dako; MIB1) and RB (Labvision; 1F8). The AQUA/PM2000 platform (HistoRx, New Haven, CT) was used to image the fluorescence-based immunostaining and generate intensity scores for each target protein. Missing values were imputed using the average value from the ten nearest-neighboring samples. Within the IDC and metastatic samples, ER AQUA scores were used to define ER-positive samples above the 30th percentile and ER-negative samples below the 30th percentile, based on the estimation that 30% of breast cancer cases are ER-negative.
Comparisons between the RB-loss signature and functionally related genes.
The RB-loss signature was evaluated against key members of the RB/p16/cyclin-D1 pathway, including p16 (CDKN2A), cyclin-D1 (CCND1), Ki67 (MKI67) and RB (RB1). The Pearson correlation coefficient for each of these genes relative to the RB-loss signature was computed within normal tissue, tumor tissue and ER-positive and negative subsets. ANOVA was used to test the RB-loss signature and these key pathway genes for differential expression in comparisons of tumor versus normal and for ER-negative versus ER-positive breast cancers. An additional dataset of 180 breast cancer samples was used to compare key members of the RB RB/p16/cyclin-D1 pathway at the protein level. ANOVA was used to test for differential expression of cyclin-D1, p16, Ki67 and RB proteins, based on log2 AQUA scores, in comparisons of tumor versus normal and ER-negative versus ER-positive breast cancer.
The RB-loss signature was compared against gene signatures representative of tumor proliferation and differentiation to identify similarities among their gene composition and expression profiles. A set of proliferation genes was compiled from multiple studiesCitation30,Citation44–Citation46 and includes genes of prognostic significance. Genes representative of tumor differentiation and histological grade have been described using the Genomic Grade Index (GGI) and also demonstrate prognostic significance.Citation47 Overlap among the three signatures was identified based on gene symbol. Pearson's correlation coefficient was computed for the proliferation and GGI expression signatures versus the RB-loss signature within healthy breast samples and all tumor samples in addition to ER-positive and ER-negative subsets. To further establish the proliferative capacity of the RB-loss signature, the proportion of metastasis events among samples having high RB-loss signature expression was tested for significance using Pearson's chi-square test.
Survival analysis.
Survival analysis was performed using Kaplan-Meier curves for relapse-free survival among all tumor samples, as well as ER-positive and ER-negative subsets. X-Tile softwareCitation48 was used to define optimal cut points for low, intermediate and high expression based on ten year relapse-free survival within each subset. Log-rank test p-values were generated based on ten year survival data. Comparisons between Kaplan- Meier survival curves for ER-positive and ER-negative disease were performed by comparing subpopulations based on the low and high cut points defined in the ER-negative analysis, where samples below 0.25 expression cut point were classified as low and samples above the expression cut point of 0.73 were classified as high. Survival analysis was also performed on subpopulations with known treatment regimens. Within the ER-positive samples, Kaplan-Meier curves were used to evaluate response to endocrine (tamoxifen) therapy in subpopulations with high RB-loss signature expression versus subpopulations with low RB-loss signature expression. Within the ER-negative samples, Kaplan-Meier curves were used to evaluate survival dependent on the RB-loss signature in subpopulations with and without chemotherapy. Recurrence intervals were available for only one ER-negative cohort (ArrayExpress accession E-TABM-158) among the unified microarray datasets. Due to this limited number of ER-negative samples with available treatment data, the low RB-loss signature expression population was expanded to include samples below the 0.73 expression cut point, while samples above that cut point were still considered as high RB-loss signature expression. Survival analysis among these subpopulations was used to evaluate the outcome in untreated patients and response to chemotherapy dependent on the RB-loss signature. To provide additional strength to the analysis of the RB-loss signature in ER-negative samples, two independent cohort datasets, identified with accession numbers MDA133,Citation49 and GSE18864,Citation50 that included pathological response for ER-negative patients treated with chemotherapy were analyzed separately. These two datasets were used to evaluate the degree of pathological response in high RB-loss versus low RB-loss signature patients. Within each of these datasets, the average RB-loss signature was used to group patients into the lower quartile, interquartile and upper quartile ranges. The proportion of patients showing pathological complete response (pCR), pathological partial response (pPR) and recurrent or progressive disease (PD) was compared between the upper quartile and lower quartile expression groups. The p values for the observed differences between upper and lower quartile groups were computed using a Pearson's chi-square test.
Figures and Tables
Figure 1 Relative expression of the RB target signature and correlated genes in normal and tumor samples. Correlation between genes in the RB/CCND1 pathway and the RB-loss signature magnitude are shown along side their expression level heat maps in (A) normal breast, (B) all tumor samples and subsets for (C) ER-positive and (D) ER-negative samples. Boxplots show the (E–H) relative transcript expression levels of RB target signature and RB/CCND1 pathway genes. (I) ER-positive and ER-negative human breast cancer cell lysates were subjected to immunoblotting with the indicated antibodies for RB pathway members. (J) AQUA immunohistochemical co-staining of tumor specimens for indicated proteins. Cytokeratin (green) was used to detect epithelial cells. Dapi (blue) staining was utilized to detect all nuclei in the section. Target proteins are stained in red. (K–M) relative protein levels of the RB target signature and RB/CCND1 pathway genes for healthy breast, all tumor samples and tumor samples stratified by ER status.
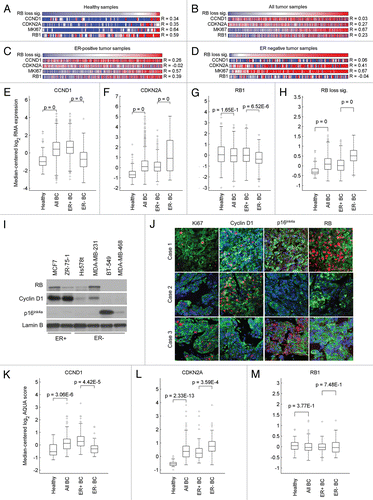
Figure 2 Correlation between the RB target signature and signatures representing cell proliferation and histological tumor grade. (A) A Venn diagram representing overlap between the RB target signature, the proliferation signature and GGI signature. (B–E) The expression of RB-loss, proliferation and GGI signatures is highly correlated.
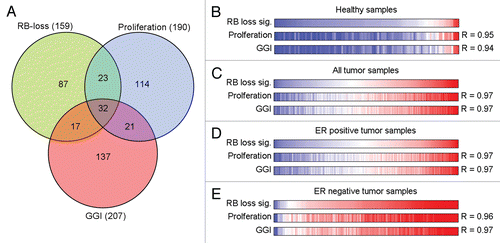
Figure 3 Relapse-free survival in subpopulations defined by RB-loss signature expression. Survival curves are shown for low, intermediate and high RB-loss signature magnitude for (A) all tumor samples, (B) the ER-positive subset and (C) the ER-negative subset. A comparison of ER-positive and ERnegative survival curves is shown in (D), using cut offs established in the ER-negative population.
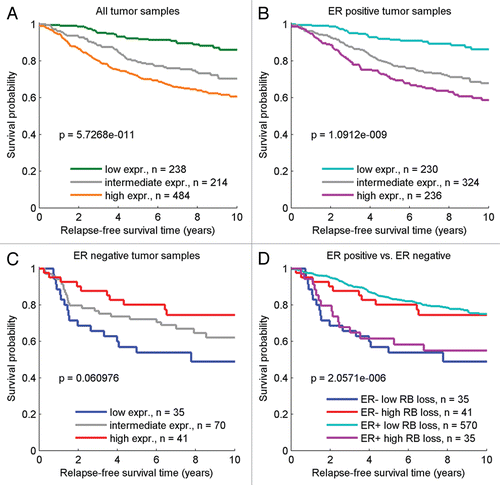
Figure 4 Relapse-free survival in the ER-positive population, stratified by treatment status and RB-loss signature. (A) In ER-positive samples with surgery alone, poor outcome is associated with high RB-loss signature [51% 10 yr relapse-free survival (rfs)] versus low RB-loss signature (95% 10 yr rfs). (B) In ER-positive patients treated with Tamoxifen, outcome in high RB-loss signature samples is improved (66% 10 yr rfs) relative to low RB-loss signature samples (92% 10 yr rfs).
![Figure 4 Relapse-free survival in the ER-positive population, stratified by treatment status and RB-loss signature. (A) In ER-positive samples with surgery alone, poor outcome is associated with high RB-loss signature [51% 10 yr relapse-free survival (rfs)] versus low RB-loss signature (95% 10 yr rfs). (B) In ER-positive patients treated with Tamoxifen, outcome in high RB-loss signature samples is improved (66% 10 yr rfs) relative to low RB-loss signature samples (92% 10 yr rfs).](/cms/asset/0c65dc4c-b5e0-4d2a-8450-e051ed2c73bd/kccy_a_10913454_f0004.gif)
Figure 5 Disease outcomes related to the RB-loss signature and treatment regimens in the ER-negative population. (A) High RB-loss signature expression is associated with different outcomes in ER-positive vs. ER-negative. (B) High RB-loss signature expression is associated with similar survival profiles between ER-positive and untreated ER-negative samples. (C) Chemotherapy is associated with better outcome in ER-negative samples with high RB-loss signature expression. (D) The proportion of pCR and PD in high versus low RB-loss signature groups of ER-positive patients receiving FAC therapy. (E) The proportion of pCR and PD in high versus low RB-loss signature groups of ER-negative patients receiving FAC therapy. (F) The proportion of pCR, pPR and PD in high versus low RB-loss signature groups of ER-negative patients receiving cisplatin therapy.
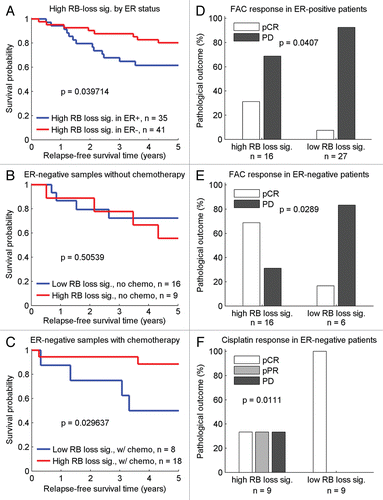
Figure 6 Candidate markers for targeted therapy. (A) Human breast cancer cell lines were treated with 500 nM PD-0332991 or DMSO control for 24 hours. Percentage BrdU positive (of total) is shown. (B) Human breast cancer cell lines were treated with 500 nM PD-0332991 or DMSO control for 24 hours. Cell lysates were subjected to immunoblot analysis for indicated proteins. (C) Relapse-free survival curves for breast cancer populations stratified by individual gene transcript levels.
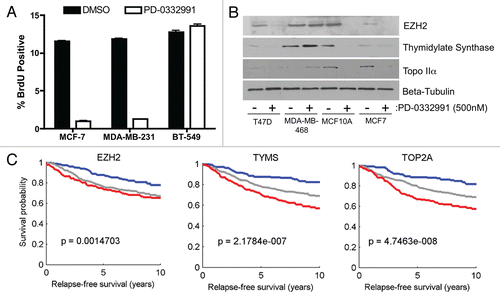
Additional material
Download Zip (2 MB)Acknowledgements
National Cancer Institute grants CA129134 (E.S. Knudsen) and CA118740 (H. Rui) and Komen for the Cure Promise Grant KG091116 (H. Rui).
References
- American Cancer Society. Cancer facts & figures 2009 2009; Atlanta, GA The American Cancer Society
- Musgrove EA, Sutherland RL. Biological determinants of endocrine resistance in breast cancer. Nat Rev Cancer 2009; 9:631 - 643
- Schneider BP, Winer EP, Foulkes WD, Garber J, Perou CM, Richardson A, et al. Triple-negative breast cancer: risk factors to potential targets. Clin Cancer Res 2008; 14:8010 - 8018
- Reis-Filho JS, Tutt AN. Triple negative tumours: a critical review. Histopathology 2008; 52:108 - 118
- Miller TW, Perez-Torres M, Narasanna A, Guix M, Stal O, Perez-Tenorio G, et al. Loss of Phosphatase and Tensin homologue deleted on chromosome 10 engages ErbB3 and insulin-like growth factor-I receptor signaling to promote antiestrogen resistance in breast cancer. Cancer Res 2009; 69:4192 - 4201
- Osborne CK, Bardou V, Hopp TA, Chamness GC, Hilsenbeck SG, Fuqua SA, et al. Role of the estrogen receptor coactivator AIB1 (SRC-3) and HER-2/neu in tamoxifen resistance in breast cancer. J Natl Cancer Inst 2003; 95:353 - 361
- Creighton CJ, Massarweh S, Huang S, Tsimelzon A, Hilsenbeck SG, Osborne CK, et al. Development of resistance to targeted therapies transforms the clinically associated molecular profile subtype of breast tumor xenografts. Cancer Res 2008; 68:7493 - 7501
- Massarweh S, Schiff R. Resistance to endocrine therapy in breast cancer: exploiting estrogen receptor/growth factor signaling crosstalk. Endocr Relat Cancer 2006; 1:15 - 24
- Burkhart DL, Sage J. Cellular mechanisms of tumour suppression by the retinoblastoma gene. Nat Rev Cancer 2008; 8:671 - 682
- Knudsen ES, Knudsen KE. Tailoring to RB: tumour suppressor status and therapeutic response. Nat Rev Cancer 2008; 8:714 - 724
- Cobrinik D. Pocket proteins and cell cycle control. Oncogene 2005; 24:2796 - 2809
- Gladden AB, Diehl JA. Location, location, location: The role of cyclin D1 nuclear localization in cancer. J Cell Biochem 2005; 96:906 - 913
- Sutherland RL, Musgrove EA. Cyclins and breast cancer. J Mammary Gland Biol Neoplasia 2004; 9:95 - 104
- Mittnacht S. Control of pRB phosphorylation. Curr Opin Genet Dev 1998; 8:21 - 27
- Markey MP, Bergseid J, Bosco EE, Stengel K, Xu H, Mayhew CN, et al. Loss of the retinoblastoma tumor suppressor: differential action on transcriptional programs related to cell cycle control and immune function. Oncogene 2007; 26:6307 - 6318
- Arnold A, Papanikolaou A. Cyclin D1 in breast cancer pathogenesis. J Clin Oncol 2005; 23:4215 - 4224
- Dublin EA, Patel NK, Gillett CE, Smith P, Peters G, Barnes DM. Retinoblastoma and p16 proteins in mammary carcinoma: their relationship to cyclin D1 and histopathological parameters. Int J Cancer 1998; 79:71 - 75
- Hui R, Macmillan RD, Kenny FS, Musgrove EA, Blamey RW, Nicholson RI, et al. INK4a gene expression and methylation in primary breast cancer: overexpression of p16INK4a messenger RNA is a marker of poor prognosis. Clin Cancer Res 2000; 6:2777 - 2787
- Oliveira AM, Ross JS, Fletcher JA. Tumor suppressor genes in breast cancer: the gatekeepers and the caretakers. Am J Clin Pathol 2005; 124:16 - 28
- Bosco EE, Knudsen ES. RB in breast cancer: at the crossroads of tumorigenesis and treatment. Cell Cycle 2007; 6:667 - 671
- Markey MP, Angus SP, Strobeck MW, Williams SL, Gunawardena RW, Aronow BJ, et al. Unbiased analysis of RB-mediated transcriptional repression identifies novel targets and distinctions from E2F action. Cancer Res 2002; 62:6587 - 6597
- Derenzini M, Donati G, Mazzini G, Montanaro L, Vici M, Ceccarelli C, et al. Loss of retinoblastoma tumor suppressor protein makes human breast cancer cells more sensitive to antimetabolite exposure. Clin Cancer Res 2008; 14:2199 - 2209
- Treré D, Brighenti E, Donati G, Ceccarelli C, Santini D, Taffurelli M, et al. High prevalence of retinoblastoma protein loss in triple-negative breast cancers and its association with a good prognosis in patients treated with adjuvant chemotherapy. Annals of Oncology 2009; 20:1818 - 1823
- Burkhart DL, Ngai LK, Roake CM, Viatour P, Thangavel C, Ho VM, et al. Regulation of RB transcription in vivo by RB family members. Mol Cell Biol 30:1729 - 1745
- Ren B, Cam H, Takahashi Y, Volkert T, Terragni J, Young RA, et al. E2F integrates cell cycle progression with DNA repair, replication and G(2)/M checkpoints. Genes Dev 2002; 16:245 - 256
- Ishida S, Huang E, Zuzan H, Spang R, Leone G, West M, et al. Role for E2F in control of both DNA replication and mitotic functions as revealed from DNA microarray analysis. Mol Cell Biol 2001; 21:4684 - 4699
- Vernell R, Helin K, Muller H. Identification of target genes of the p16INK4A-pRB-E2F pathway. J Biol Chem 2003; 278:46124 - 46137
- Subhawong AP, Subhawong T, Nassar H, Kouprina N, Begum S, Vang R, et al. Most basal-like breast carcinomas demonstrate the same Rb−/p16+ immunophenotype as the HPV-related poorly differentiated squamous cell carcinomas which they resemble morphologically. Am J Surg Pathol 2009; 33:163 - 175
- Nielsen NH, Loden M, Cajander J, Emdin SO, Landberg G. G1-S transition defects occur in most breast cancers and predict outcome. Breast Cancer Res Treat 1999; 56:105 - 112
- Herschkowitz JI, He X, Fan C, Perou CM. The functional loss of the retinoblastoma tumour suppressor is a common event in basal-like and luminal B breast carcinomas. Breast Cancer Res 2008; 10:75
- Bosco EE, Wang Y, Xu H, Zilfou JT, Knudsen KE, Aronow BJ, et al. The retinoblastoma tumor suppressor modifies the therapeutic response of breast cancer. J Clin Invest 2007; 117:218 - 228
- Stengel KR, Dean JL, Seeley SL, Mayhew CN, Knudsen ES. RB status governs differential sensitivity to cytotoxic and molecularly-targeted therapeutic agents. Cell Cycle 2008; 7:1095 - 1103
- Dean JL, Thangavel C, McClendon AK, Reed CA, Knudsen ES. Therapeutic CDK4/6 inhibition in breast cancer: key mechanisms of response and failure. Oncogene 2010; 29:4018 - 4032
- Edgar R, Domrachev M, Lash AE. Gene Expression Omnibus: NCBI gene expression and hybridization array data repository. Nucleic Acids Res 2002; 30:207 - 210
- Brazma A, Parkinson H, Sarkans U, Shojatalab M, Vilo J, Abeygunawardena N, et al. ArrayExpress—a public repository for microarray gene expression data at the EBI. Nucleic Acids Res 2003; 31:68 - 71
- Ertel A, Tozeren A. Human and mouse switch-like genes share common transcriptional regulatory mechanisms for bimodality. BMC Genomics 2008; 9:628
- Irizarry RA, Hobbs B, Collin F, Beazer-Barclay YD, Antonellis KJ, Scherf U, et al. Exploration, normalization and summaries of high density oligonucleotide array probe level data. Biostatistics 2003; 4:249 - 264
- Katz S, Irizarry RA, Lin X, Tripputi M, Porter MW. A summarization approach for Affymetrix GeneChip data using a reference training set from a large, biologically diverse database. BMC Bioinformatics 2006; 7:464
- Gong Y, Yan K, Lin F, Anderson K, Sotiriou C, Andre F, et al. Determination of oestrogen-receptor status and ERBB2 status of breast carcinoma: a gene-expression profiling study. Lancet Oncol 2007; 8:203 - 211
- Ashburner M, Ball CA, Blake JA, Botstein D, Butler H, Cherry JM, et al. Gene Ontology: tool for the unification of biology. Nat Genet 2000; 25:25 - 29
- Dennis G Jr, Sherman BT, Hosack DA, Yang J, Gao W, Lane HC, et al. DAVID: Database for Annotation, Visualization and Integrated Discovery. Genome Biol 2003; 4:3
- Zagorski WA, Knudsen ES, Reed MF. Retinoblastoma deficiency increases chemosensitivity in lung cancer. Cancer Res 2007; 67:8264 - 8273
- Tran TH, Utama FE, Lin J, Yang N, Sjolund AB, Ryder A, et al. Prolactin inhibits BCL6 expression in breast cancer through a Stat5a-dependent mechanism. Cancer Res 2010; 70:1711 - 1721
- Dai H, van't Veer L, Lamb J, He YD, Mao M, Fine BM, et al. A cell proliferation signature is a marker of extremely poor outcome in a subpopulation of breast cancer patients. Cancer Res 2005; 65:4059 - 4066
- Hu Z, Fan C, Oh DS, Marron JS, He X, Qaqish BF, et al. The molecular portraits of breast tumors are conserved across microarray platforms. BMC Genomics 2006; 7:96
- Whitfield ML, George LK, Grant GD, Perou CM. Common markers of proliferation. Nat Rev Cancer 2006; 6:99 - 106
- Sotiriou C, Wirapati P, Loi S, Harris A, Fox S, Smeds J, et al. Gene expression profiling in breast cancer: understanding the molecular basis of histologic grade to improve prognosis. J Natl Cancer Inst 2006; 98:262 - 272
- Camp RL, Dolled-Filhart M, Rimm DL. X-tile: a new bio-informatics tool for biomarker assessment and outcome-based cut-point optimization. Clin Cancer Res 2004; 10:7252 - 7259
- Hess KR, Anderson K, Symmans WF, Valero V, Ibrahim N, Mejia JA, et al. Pharmacogenomic predictor of sensitivity to preoperative chemotherapy with paclitaxel and fluorouracil, doxorubicin and cyclophosphamide in breast cancer. J Clin Oncol 2006; 24:4236 - 4244
- Li Y, Zou L, Li Q, Haibe-Kains B, Tian R, Desmedt C, et al. Amplification of LAPTM4B and YWHAZ contributes to chemotherapy resistance and recurrence of breast cancer. Nat Med 2010; 16:214 - 218